Mass Spectrometry-Based Untargeted Plant Metabolomics
Saleh Alseekh, Saleh Alseekh, Leonardo Perez de Souza, Leonardo Perez de Souza, Thomas Naake, Thomas Naake, Alisdair Fernie, Alisdair Fernie
Abstract
Metabolomics has grown into one of the major approaches for systems biology studies, in part driven by developments in mass spectrometry (MS), providing high sensitivity and coverage of the metabolome at high throughput. Untargeted metabolomics allows for the investigation of metabolic phenotypes involving several hundreds to thousands of metabolites. In this approach, all signals in a mass chromatogram are processed in an unbiased way, allowing for a deeper investigation of metabolic phenotypes, but also resulting in significantly more complex data processing and post-processing steps. In this article, we discuss all the intricacies involved in extracting and analyzing metabolites by chromatography coupled to MS, as well as the processing and analysis of such datasets. © 2019 The Authors.
Basic Protocol 1 : Metabolite extraction for LC-MS
Alternate Protocol : Methyl tert -butyl ether (MTBE) extraction for multiple mass spectrometry platforms (GC-polar, LC-polar, LC-lipid)
Basic Protocol 2 : LC-MS analysis
Support Protocol 1 : GC-MS derivatization and analysis
Support Protocol 2 : Lipid analysis
Basic Protocol 3 : LC-MS data processing
Basic Protocol 4 : Data analysis
Basic Protocol 5 : Metabolite annotation
Support Protocol 3 : Molecular networking using MetNet
Support Protocol 4 : Co-injection of authentic standards
INTRODUCTION
The past decades witnessed an exponential increase in our ability to attain information of the molecular mechanisms regulating biological systems, driven by technological advances in the analytical capabilities of measuring genes, transcripts, proteins, and metabolites. Such advances culminated in the coining of the term “omics,” referring to the capacity of describing an organism, as its whole, at a certain level of complexity. The metabolome, in this sense, comprises the entire complement of small molecules an organism accumulates at a certain point in time. An inherent complexity of the metabolome in relation to the genome, transcriptome, and proteome is a profound lack of regularity among metabolite structures, particularly when compared to these other molecules. Metabolites cover a much broader range of different physico-chemical properties, making it difficult to envision a single technique capable of measuring their entirety (Cajka & Fiehn, 2016). Moreover, it also imposes a significant challenge to identify measured metabolites (Fernie et al., 2011). Currently, the most promising technique in terms of metabolome coverage is liquid chromatography coupled to mass spectrometry (LC-MS). Compared to other alternatives, LC-MS is more versatile in that sample preparation and elution systems (chromatographic separation) can be adapted to nearly every class of compound, while being extremely sensitive and additionally providing rich structural information. These features make LC-MS a particularly interesting choice for untargeted metabolomics, i.e., the unbiased measurement of all metabolites detected in a particular analysis. This article will provide protocols for performing basic comparative untargeted metabolomics analysis in plant material from different sources. It includes basic protocols for sample extraction for polar/medium polarity metabolites (Basic Protocol 1), conditions for reversed-phase LC-MS analysis (Basic Protocol 2), initial data processing for peak picking chromatogram alignment and compound mass spectra extraction from data-independent acquisition (DIA) experiments (Basic Protocol 3), data analysis to identify differentially accumulated metabolites (Basic Protocol 4), and LC-MS-based metabolite annotation (Basic Protocol 5). Additionally, we include an alternate protocol to include the extraction of metabolites for gas chromatography mass spectrometry (GC-MS) and lipidomics analysis (Alternate Protocol) as well as support protocols with conditions for GC-MS analysis (Support Protocol 1), lipidomics analysis (Support Protocol 2), and molecular networking using the MetNet tool (Support Protocol 3).
STRATEGIC PLANNING
Several factors should be considered before starting any untargeted metabolomics experiment that otherwise could compromise the whole experiment. First, it is important to have in mind that absolute quantification is not a possibility in such experiments; therefore, one should consider the best strategies for the relative quantification of metabolites within the samples (Cajka & Fiehn, 2016). A common approach is to obtain relative quantification in relation to internal standards (ISs) added during extraction. The choice of an IS requires some previous knowledge about the sample. Ideally, they should be structurally similar to compounds of interest and not be present naturally within the sample.
Metabolomics experiments usually require the comparison of multiple samples analyzed over the span of several days. One should always consider that GC-MS and LC-MS systems are both (although LC-MS more than GC-MS) prone to exhibiting intensity drifts over such periods which could compromise sample comparison (Livera et al., 2015). There are many different strategies to avoid this particular issue, but in general, it is a good practice to run all samples belonging to the same experiment sequentially (separated in smaller batches when necessary), in a fully randomized order. Additionally, it is strongly advised that several technical replicates of a quality control (QC) are included and distributed across every batch of samples. QCs are not only an excellent way of accessing and normalizing for such intensity drifts but are also very useful for facilitating further data cleanup procedures. The results of untargeted metabolomics data processing are highly dimensional, often comprising a high number of features (chromatographic peaks) in relation to observations (samples; Schiffman et al., 2019). QCs can be used to remove unstable features; therefore, their composition should ideally reflect those of the samples. A pool of all samples should be considered whenever this is a possibility. Finally, following the same reasoning with regard to data post-processing, it is useful to include extraction blanks for which no plant material is extracted in order to identify and remove signals arising from contaminates introduced by the extraction process.
Basic Protocol 1: METABOLITE EXTRACTION FOR LC-MS
Extraction is a vital step in determining which metabolites are present in a sample. A multitude of different protocols are available that allow for the enrichment of specific classes of metabolites such as lipids and phytohormones (Giavalisco et al., 2011; Lisec, Schauer, Kopka, Willmitzer, & Fernie, 2006; Tohge & Fernie, 2010). Extraction protocols should ideally be as simple as possible to avoid introduction of unnecessary error while being selective to the classes of metabolites of interest, thus reducing sample complexity to the minimum necessary. Sample composition will also have a significant impact on system reproducibility and robustness, which are of great relevance for the high-throughput analysis of the large datasets often performed in metabolomics. Plants produce a particularly rich repertoire of polar to medium polarity metabolites, including amino acids and secondary metabolites. Here, we provide a simple protocol for the extraction of such classes of metabolites that can be easily applied in a high-throughput pipeline as required for metabolomics.
Materials
-
Plant material
-
Liquid nitrogen
-
Methanol ULC/MS grade (Biosolve, cat. no. 13684102)
-
Water, ULC/MS grade (Biosolve, cat. no. 23214102)
-
Extraction buffer 1 (see recipe)
-
Mixer mill (Retsch, MM 300)
-
1.5- and 2.0-ml, safe-lock, polypropylene microcentrifuge tubes, round bottom
-
Vortex
-
Centrifuge (Eppendorf, cat. no. 5409000535)
-
Glass vial (Chromacol, cat. no. 02-CTVG)
-
Screw cap for glass vial (Chromacol, cat. no. 8-SC-ST15)
-
VA 5-mm steel balls, (Geyer Berlin, cat. no. 9738870)
1.Harvest leaves from the plants, place inside a 2-ml tube, and freeze in liquid nitrogen.
2.Grind frozen materials to a fine powder using the mixer mill for 2 min at 25 Hz.
3.Aliquot frozen powder (50 mg) into a 2-ml tube and keep in liquid nitrogen.
4.Add 250 µl extraction buffer 1 to each sample, vortex, and keep on ice before proceeding to the next step.
5.Homogenize sample at room temperature using mixer mill, 4 min at 25 Hz.
6.Centrifuge samples at room temperature 10 min at 20,000 × g.
7.Transfer as much of the supernatant as possible, keeping the volume constant across all samples, to a new 1.5-ml tube.
8.Centrifuge new sample tube 5 min at 20,000 × g.
9.Transfer supernatant to a glass vial for LC-MS analysis (Basic Protocol 2).
Alternate Protocol: METHYL TERT-BUTYL ETHER (MTBE) EXTRACTION FOR MULTIPLE MASS SPECTROMETRY PLATFORMS (GC-POLAR, LC-POLAR, LC-LIPID)
As mentioned in Basic Protocol 1, the extraction procedure is crucial in determining the classes of compounds that can be analyzed within a sample. As such, we provide an alternative protocol that despite being more complex than Basic Protocol 1, allows for a broader coverage of metabolites from a single sample (Giavalisco et al., 2011). The protocol results in additional samples to be analyzed by LC-MS-based lipidomics as well as GC-MS platforms.
Additional Materials (also see Basic Protocol 1)
-
Liquid nitrogen
-
Extraction buffer 2 (see recipe)
-
Extraction buffer 3 (see recipe)
-
Resuspension buffer 1 (see recipe)
-
Resuspension buffer 2 (see recipe)
-
Mixer mill (Retsch, MM 300)
-
1.5- and 2.0-ml, safe-lock, polypropylene microcentrifuge tubes, round bottom
-
Spatula
-
Centrifuge (Eppendorf, cat. no. 5409000535)
-
Speed vac
-
Glass vial (Chromacol)
-
Screw cap for glass vial (Chromacol)
-
VA 5-mm steel balls (Geyer Berlin)
-
Tips for pipet, fine quality
-
Ultrasonic bath (Bandelin Sonorex RK100, cat. no. 301)
1.Prepare samples following Basic Protocol 1, steps 1-3.
2.Add 1 ml pre-cooled (−20°C) extraction buffer 2, vortex, and keep samples on ice throughout steps 2-9.
3.Shake 10 min at 4 °C.
4.Sonicate for 10 min.
5.Add 500 µl extraction buffer 3, pre-cooled on ice, and vortex well.
6.Centrifuge 5 min at room temperature and 15,000 × g.
7.Transfer as much as possible (∼500 µl) from upper phase (lipophilic phase) into a fresh 1.5-ml tube.
8.Remove the rest of upper phase by pipetting.
9.Take two aliquots of 150 µl each (for GC-polar phase and a back up) and 300 µl (for LC-polar phase) from the lower phase (polar phase) and place in fresh 1.5-ml tubes.
10.Dry samples from both aliquots in a speed vac (∼3 hr to overnight) and store at −80°C or proceed to the next step.
11.Dissolve upper phase (lipophilic phase) in 200 µl resuspension buffer 1 and transfer to a glass vial for LC-MS analysis.
12.For the LC-MS lower dry phase (polar phase), dissolve in 200 µl resuspension buffer 2 and transfer to a glass vial for LC-MS analysis (Basic Protocol 2).
Basic Protocol 2: LC-MS ANALYSIS
A variety of systems for LC-MS are available, each with particularities that make them more suitable for some applications compared to others. Untargeted metabolomics will often rely on systems providing high resolution, and MS2 capabilities, for metabolite annotation together with relatively fast scan rates to provide enough data points within the short spans of ultra performance liquid chromatography (UPLC) peak elution. Currently, Orbitrap and QTOF-based systems are by far the most popular choices for such applications (Glauser, Veyrat, Rochat, Wolfender, & Turlings, 2013). We describe here a general method used for analyzing polar to medium polarity metabolites within a Waters ACQUITY UPLC (Waters) coupled to a Q-Exactive Orbitrap-focus system (Thermo Finnigan) via an electrospray ionization (ESI) interface. Samples are run in both positive and negative ionization modes to provide the broadest coverage of the metabolome.
Materials
-
Acetonitrile
-
Elution buffer A (see recipe)
-
Elution buffer B (see recipe)
-
Ultra performance liquid chromatography (UPLC; Waters ACQUITY UPLC system)
-
Reverse phase, analytical column, HSS T3 C18 (100 × 2.1 mm i.d., 1.8-µm particle size; Waters)
-
Q-Exactive Orbitrap-focus mass analyzer (Thermo Finnigan)
-
PC machine with Windows operating system
1.Set machine parameters according to Table 1.
Parameter | Values |
---|---|
Injection volume | 2 μl |
Column temperature | 40°C |
Flow rate | 0.4 ml/min |
Elution gradient | 1 min: 99% A, 1% B |
11 min: 60% A, 40% B | |
13 min: 30% A, 70% B | |
15 min: 1% A, 100% B | |
16 min: 1% A, 100% B | |
17 min: 99% A, 55% B | |
20 min: 99% A, 1% B | |
Spectra acquisition time in MS | 1-19 min |
MS mode | All ion fragmentation (AIF) |
Resolution | 25,000 |
Maximum injection time | 100 ms |
Automatic gain control value | 1.00E+06 |
HCD energy | 30.0 eV |
Scan range MS | 100-1500 m/z |
Scan range MS2 | 100-1000 m/z |
Capillary voltage | 3 kV |
Sheath gas flow | 60 au |
Auxiliary gas flow | 20 au |
Capillary temperature | 200°C |
Drying gas temperature | 350°C |
Skimmer voltage | 25 V |
Tube lens voltage | 130 V |
2.Start equilibrating system by injecting 3-5 blank samples (vial with MeOH).
3.Place samples from Basic Protocol 1 (step 10) or Alternate Protocol (step 12) in the autosampler.
4.Make sure to add samples in a randomized order and include QCs at regular intervals (∼every 5-8 samples).
5.Analyze each sample in positive ionization mode, immediately followed by the same sample analyzed in negative ionization mode.
6.Start batch analysis.
Support Protocol 1: GC-MS DERIVATIZATION AND ANALYSIS
GC-MS systems show significantly higher chromatographic resolution in comparison to LC-MS and a much more stable ionization provided by the interface with mass spectrometry (MS) via electron ionization (EI; Lisec et al., 2006). Consequently, deconvolution of the highly fragmented spectra signals into analyte mass spectra is facilitated and high scores can be achieved for spectral matching against standard libraries. Compound identification is facilitated even further with the use of retention time indexes to compare compound retention across different runs. The great limitation, however, is that GC-MS can only be applied to compounds that are stable under the conditions necessary to volatilize or derivatize them. That said, GC-MS represents an invaluable complement to LC-MS in order to expand metabolome coverage. In the following protocol, we provide the steps necessary for the derivatization and analysis of plant samples by GC-MS.
Materials
-
Plant samples
-
Reagent 1 (see recipe)
-
Reagent 2 (see recipe)
-
Helium
-
Gas chromatograph coupled to a time-of-flight mass spectrometer (Leco Pegasus HT TOF-MS)
-
Autosampler, GERSTEL MultiPurpose
-
30 m DB-35 column
-
PC with Chroma TOF 4.5 software (Leco)
1.Add 40 µl reagent 1 and shake 2 hr at 37°C.
2.Spin down quickly the drops on the cover, add 70 µl reagent 2, and shake 30 min at 37°C.
3.Spin down quickly the drops on the cover and transfer derivatized sample into a glass vial for GC-MS.
4.Set machine parameters according to Table 2.
Parameter | Value |
---|---|
Carrier gas | Helium, 2 ml/s |
Injection temperature (T) | 230°C |
Transfer line T | 250°C |
Ion source | 250°C |
Oven T0 | 85°C |
Oven gradient rate | 15°C/min |
Oven Tfinal | 360°C |
Solvent delay | 180 s |
Scan rate | 20 scans/s |
Scan range | 70-600 m/z |
5.Start equilibrating system by injecting 3-5 blank samples.
6.Place samples from step 3 in the autosampler.
7.Make sure to add samples in a randomized order and include QCs at regular intervals (approximately every 5-8 samples).
8.Start batch analysis.
Support Protocol 2: LIPID ANALYSIS
Lipidomics is often treated as a special type of metabolomics, in part due to the greater regularity across metabolites belonging to these classes, which allows for the application of specialized post-processing steps for the identification of these metabolites (Hummel et al., 2011). Despite using the same equipment used for polar metabolites, lipidomics analysis represents a notable example of LC-MS system flexibility. By simply changing the chromatographic system stationary phase (column) and mobile phase (buffers), it is possible to analyze a completely different set of metabolites than with traditional C18-based elution systems.
Additional Materials (also see Alternate Protocol)
-
Acetic acid, ULC/MS grade (Biosolve, cat. no. 01074131)
-
Ammonium acetate, ULC/MS grade (Biosolve, cat. no. 01244156)
-
Elution buffer C (see recipe)
-
Elution buffer D (see recipe)
-
Waters ACQUITY UPLC system
-
Analytical column, Waters BEH C8 (100 mm length × 2.1 mm i.d. × 1.7-µm particle size)
-
Q-Exactive Orbitrap-focus mass analyzer (Thermo Finnigan)
-
PC with Windows operating system
1.Set machine parameters according to Table 3.
Parameter | Values |
---|---|
Column temperature | 60°C |
Flow rate | 0.4 ml/min |
Gradient | 1 min: 45% C, 55% D |
4 min: 25% C, 75% D | |
12 min: 11% C, 89% D | |
15 min: 0% C, 100% D | |
19.5 min: 45% C, 100% D | |
24 min: 45% C, 55% D | |
Spectra acquisition time in MS | 1-20 min |
MS mode | MS |
Resolution | 50,000 |
Maximum injection time | 100 ms |
Automatic gain control value | 1.00E+06 |
HCD energy | 30.0 eV |
Scan range | 150-1500 m/z |
Capillary voltage | 3 kV |
Sheath gas flow | 60 au |
Auxiliary gas flow | 20 au |
Capillary temperature | 200°C |
Drying gas temperature | 350°C |
Skimmer voltage | 25 V |
Tube lens voltage | 130 V |
2.Start equilibrating system by injecting 3-5 blank samples (vial with MeOH).
3.Place samples from Alternate Protocol (step 11) in the autosampler.
4.Make sure to add samples in a randomized order and include QCs at regular intervals (approximately every 5-8 samples).
5.Start batch analysis.
Basic Protocol 3: LC-MS DATA PROCESSING
Data processing consists of several procedures to identify mass features, integrate those features across the chromatographic dimension, and align them across different samples. As such, there are several tools available for performing one or a combination of several of these steps (Perez de Souza, Naake, Tohge, & Fernie, 2017). This protocol focuses on the use of some procedures we consider among the most user friendly, namely processing data independent tandem MS experiments with the MS-DIAL software (Tsugawa et al., 2015) and analyzing the results within the MetaboAnalyst web platform (Chong et al., 2018). These by no means represent the only or best options; we chose to use these tools because they both provide an easy user interface that can be readily learned by the novice and they require no prior knowledge of programming, unlike other popular alternatives.
Materials
- Standard computer with Internet access
- Raw data files (see Basic Protocol 2)
- Software Abf converter
- Mass spectrometry-data independent analysis (MS-DIAL) software
Feature detection and alignment with MS-DIAL
1.Convert vendor format files to .abf using Abf Converter (http://prime.psc.riken.jp/Metabolomics_Software/MS-DIAL/index.html) and transfer all files into a project folder.
2.Start MS-DIAL software and a new processing batch in Menu → File → New project.
3.Add path for the project folder and select Soft ionization. In Method type, you will have to select between the multiple options of data-dependent and independent MS/MS acquisition. For our exemplary dataset, samples were run using a conventional All-ions method. The Experiment file is a tab-delimited file containing MS1 scan range and precursor window (Supplementary dataset 1).
4.Select appropriate data type for MS1 and MS/MS, which can be easily verified by zooming in on some of the signals on the raw MS spectra, ion mode, and experiment type.
5.Load and identify all samples. It is useful to keep a sample list with all necessary metadata in a spreadsheet (Supplementary dataset 2). We recommend that only a small subset of representative samples be processed initially in order to optimize parameters.
6.On the Data collection tab, set mass accuracy according to the machine. For our training dataset, run on an Exactive Orbitrap (Thermo Fisher Scientific); we leave the default values of 0.01 (MS1) and 0.025 (MS2).
7.In the same tab (Advanced), remove initial and final portions of the chromatogram in case of a high concentration of co-eluting peaks and to eliminate possible monitoring during column cleaning and stabilization steps often included in a run. For the training dataset, we set the retention time to begin at 2.5 min and end at 12 min. Additionally, set the appropriate mass range of the experiment to be processed (e.g., 100-1,500), and the number of threads you want to use according to your processor.
8.Check the intensity of a chromatogram/mass spectra region without any eluting peaks to select an appropriate intensity threshold that should be set on the Peak detection tab.
9.MS-DIAL automatically matches deconvoluted spectra to an .msp library that should be loaded in the identification tab.
10.Check raw data with sample preparation and analysis information in mind (e.g., extraction procedures, mobile phase composition) and check which are the relevant adducts to be searched for; this will potentially facilitate further interpretation of detected features.
11.We recommend initially performing peak spotting without aligning the samples by unchecking the box in the bottom left, Together with Alignment.
12.In case you run a subset of representative samples to optimize processing parameters, MS-DIAL allows you to plot all extracted ion chromatograms for a sample.
Select the sample in the File navigator and click Show ion table on the top right of the peak spot viewer.
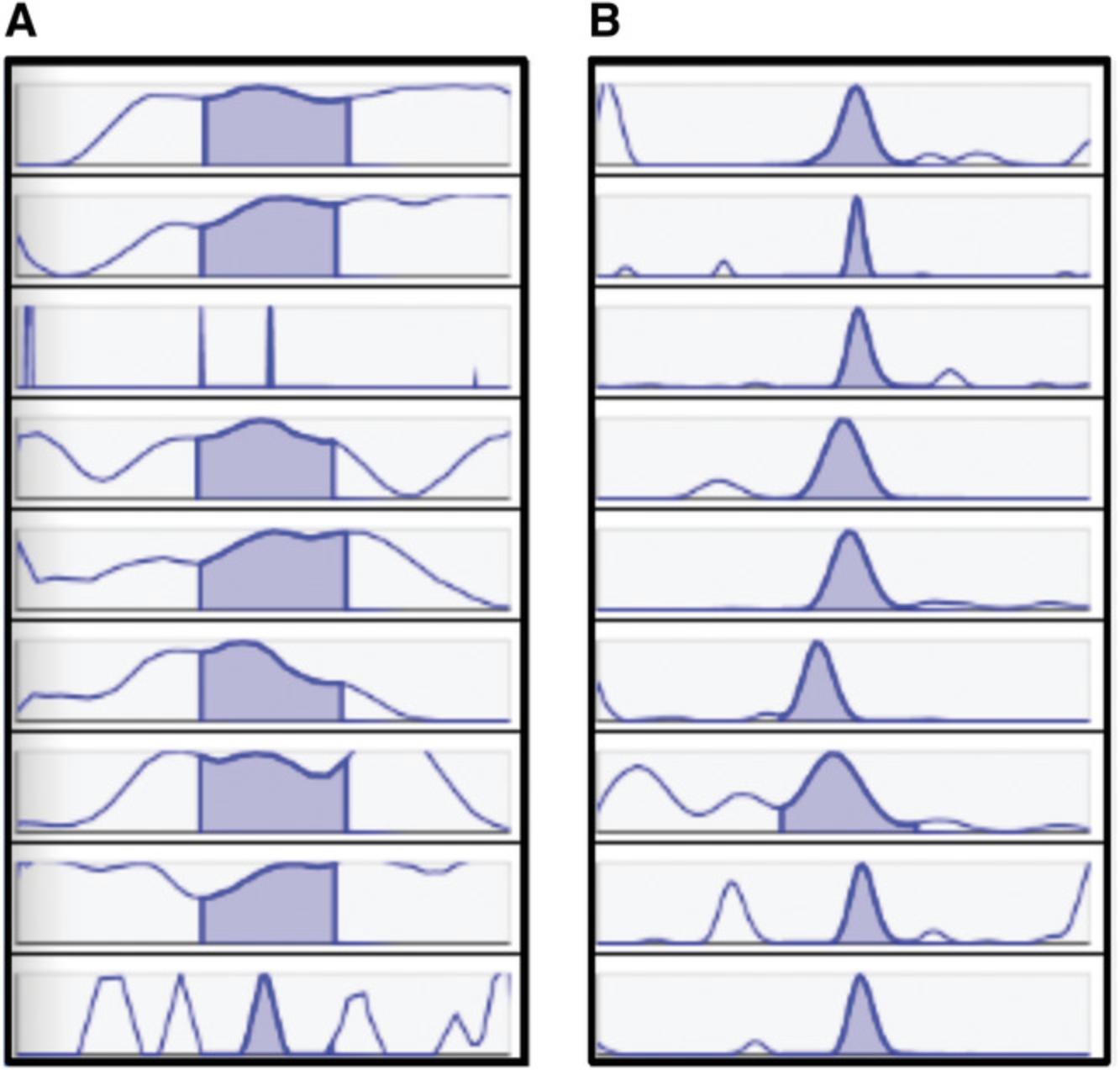
13.Once feature detection results are acceptable, align all chromatograms in Menu → Data processing → Alignment.
Quality check
14.Before starting the quality assessment, remove blank samples to facilitate interpretation. Go to Menu → Option → File property setting and uncheck boxes in the column Included for the blank samples.
15.Normalize data according to your dataset.
16.Plot a principal component analysis (PCA) in Menu → Data visualization → Principal component analysis, as a quick way of checking data quality.
17.Within our exemplary data, observe sample 23_neg (IL 10-3) is a clear outlier(Fig. 2A).
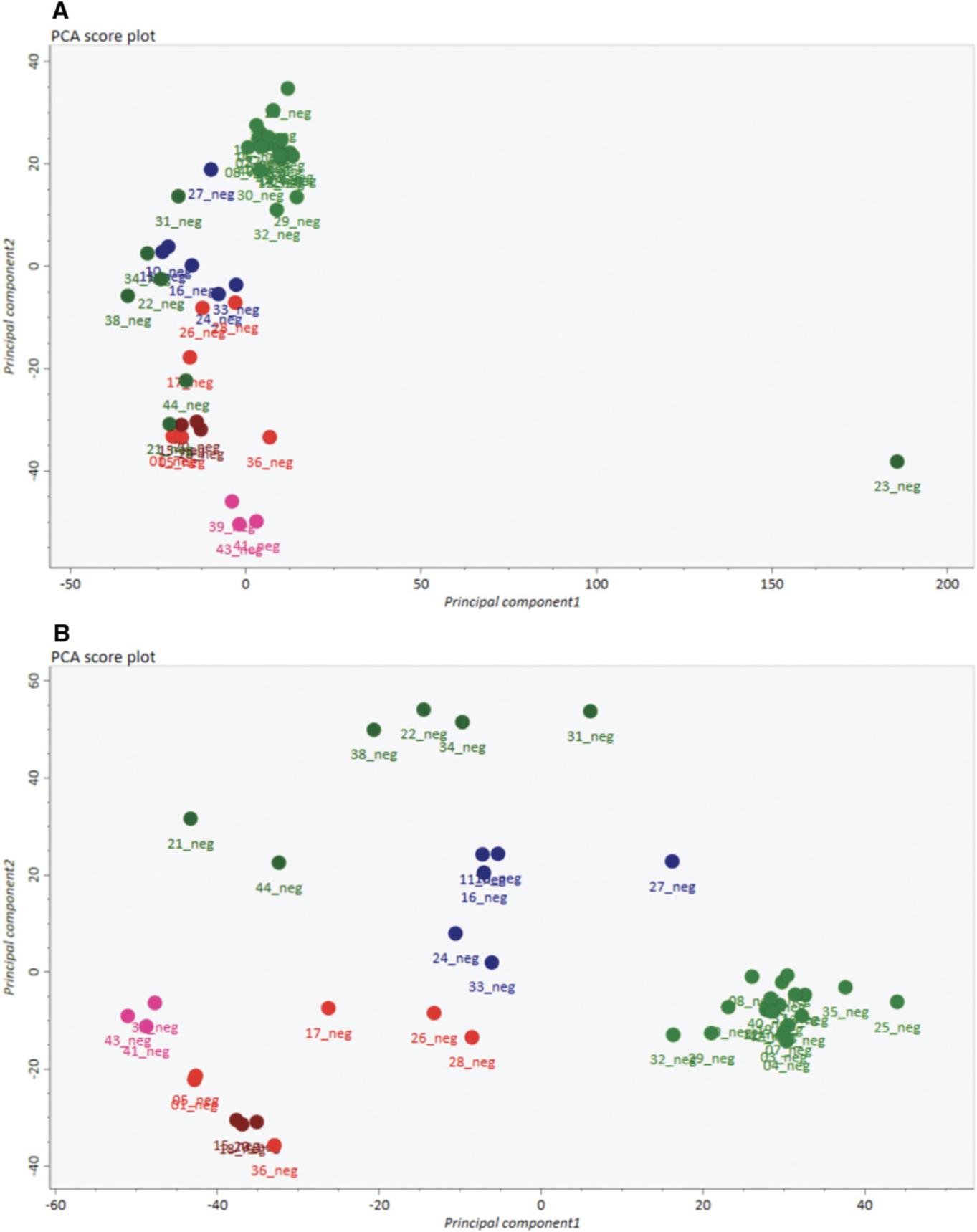
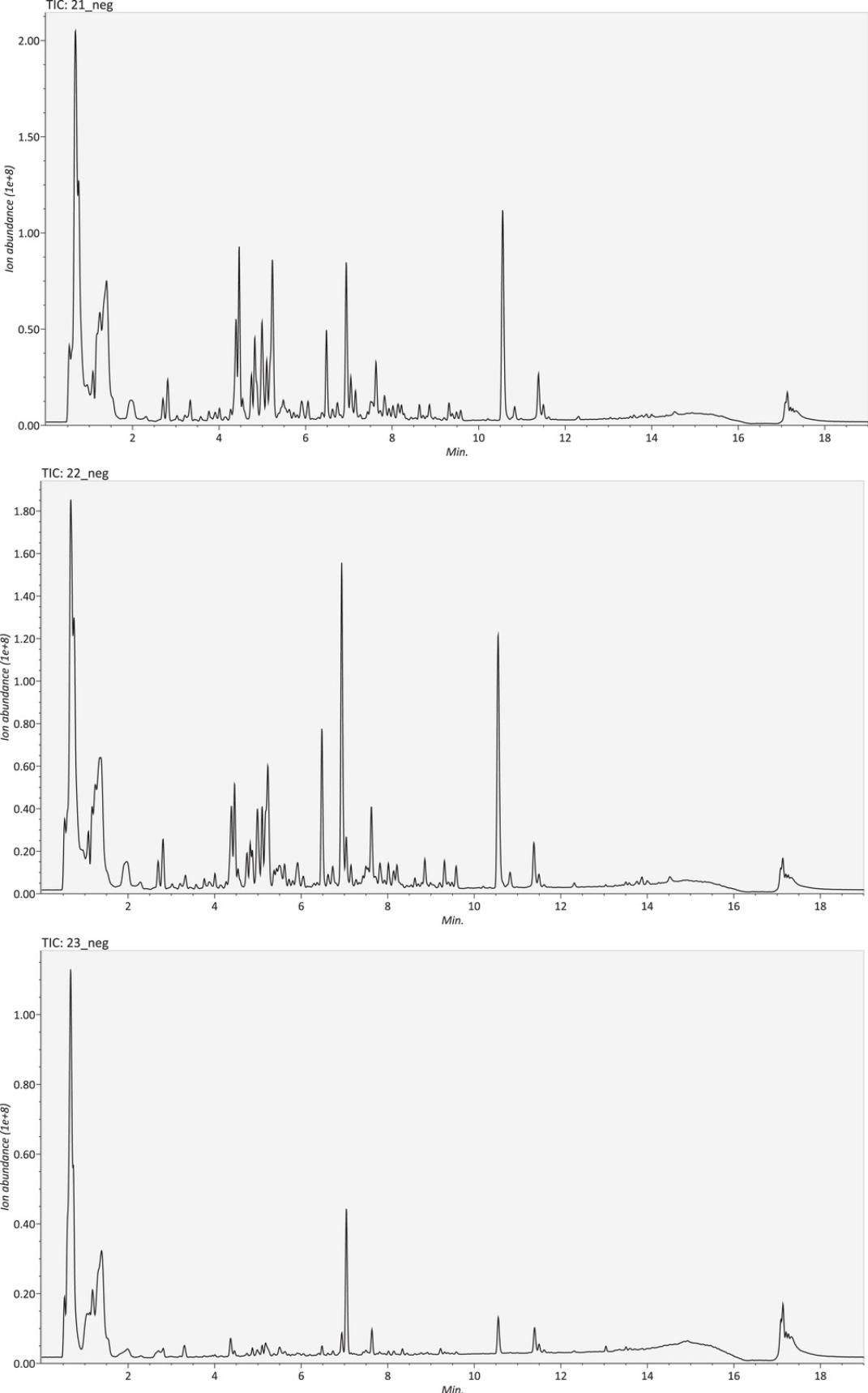
18.Observe that a new PCA after exclusion of sample 23_neg (Fig. 2B) displays a clear separation between different genotypes represented by different colors.
19.Export raw data for peak areas in Menu → Export → Alignment result. Be sure to select options for filtering data based on blank samples and replacing zero values by one tenth of the minimum. Change export format to text and Spectra type to centroid.
Basic Protocol 4: DATA ANALYSIS
Data analysis varies significantly according to the biological question of the experiment. Several traditional statistical models are commonly used to characterize metabolic changes and to identify variables relevant to differentiate treatments. On top of that, heteroscedasticity, high dimensionality, and a large range of concentrations characterize most untargeted metabolomics datasets. Therefore, data transformation and feature selection are as important as the choice of appropriate statistical models. In this protocol, we describe how to upload data exported from MS-DIAL into the MetaboAnalyst web platform and how to perform some general steps of data analysis including data transformation, feature selection, normalization, and identification of important variables by partial least square discriminant analysis (PLS-DA). MetaboAnalyst provides a rich set of utilities in a user friendly platform that can be applied to data obtained from any platform with little adjustment (Chong et al., 2018; Current Protocols article: Chong, Wishart, & Xia, 2019; Chong, Yamamoto, & Xia, 2019).
Materials
- Standard computer with Internet access
- Processed data (see Basic Protocol 3)
Feature filtering and data normalization with MetaboAnalyst
1.Format data matrix exported from MS-DIAL for MetaboAnalyst input format by deleting all columns except for alignment ID and the sample areas. Also delete rows 2-4, leaving only sample names in the first row and sample classes in the second row (Supplementary dataset 3).
2.Open MetaboAnalyst in your browser (https://www.metaboanalyst.ca/MetaboAnalyst/faces/home.xhtml) and select Welcome >> click here to start << → Statistical Analysis.
3.Upload and submit your data matrix: For Data Type, select Peak intensity table; for Format, select Samples in columns (unpaired).
4.Skip missing values estimation.
5.Initial data processing steps usually result in an overwhelming number of features, many of which are uninformative, and may jeopardize the integrity of the statistical models. MetaboAnalyst provides several (cumulative) options for data filtering that are quickly performed in series for testing the most appropriate filtering preferences. For the training dataset, filtering features based on high QC relative standard deviation (RSD; >25%) and low standard deviation (SD) proved to be the most effective. Fig. 4 shows a PCA comparison of unfiltered versus filtered data.
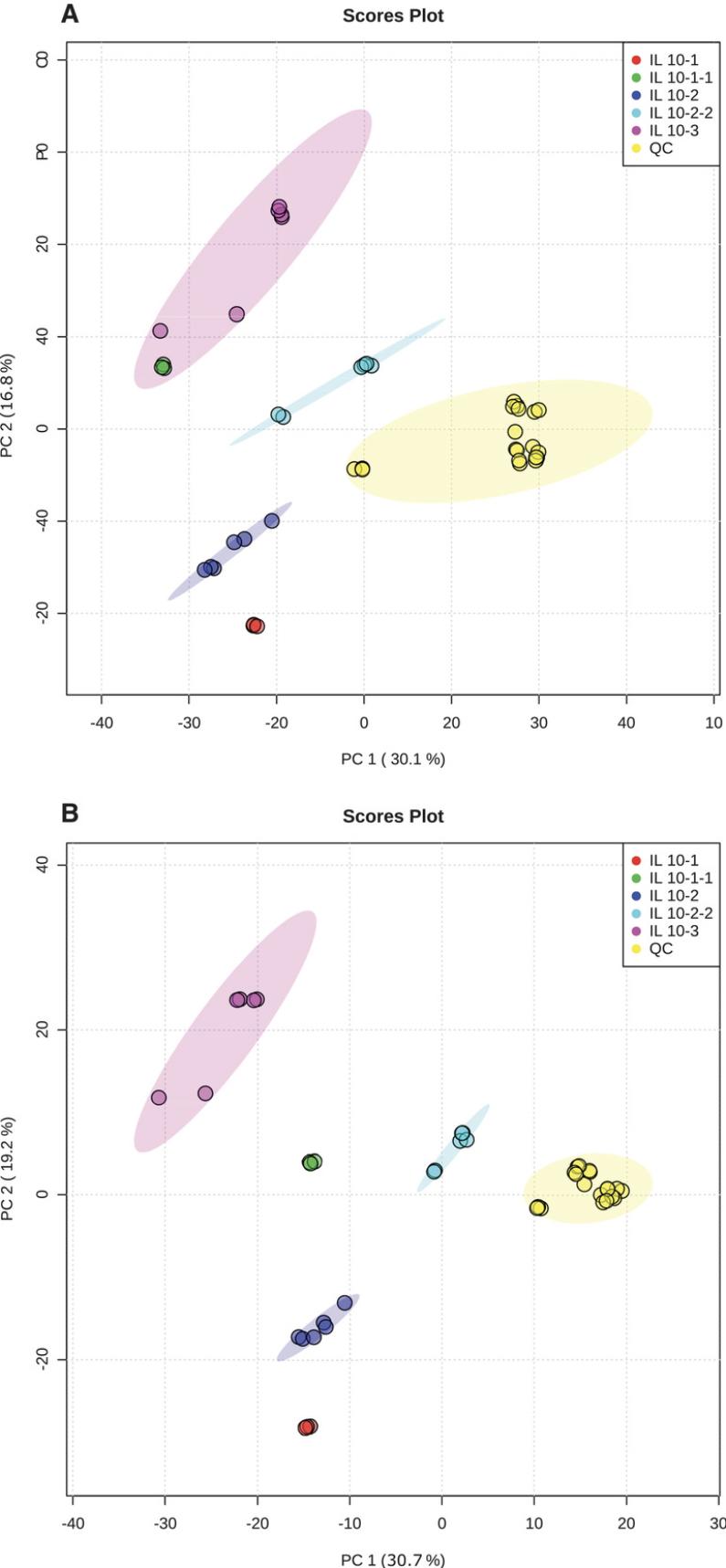
6.Several options for data normalization, transformation, and scaling are available under the Normalization tab.
7.Visualize sample distribution on the PCA tab → 2D Scores Plot.
Identify variables important for projection in PLS-DA
8.Select the PLSDA tab.
9.Verify that group separation is consistent in the 2D Scores Plot.
10.In the Imp. Features tab, visualize features exhibiting the top variable importance in projection (VIP) scores as well as their relative values across different groups.
11.Alternatively, plot a heatmap of these variables in the tab Heatmap by selecting the Use top box with the PLS-DA VIP option.
Basic Protocol 5: METABOLITE ANNOTATION
Metabolite annotation is one of the major challenges in MS-based metabolomics with the vast majority of metabolite features remaining unannotated in most experiments (da Silva, Dorrestein, & Quinn, 2015; Fernie et al., 2011). Mass spectra provide relevant structural information through molecular masses, fragmentation patterns, and isotopic composition. Despite that, the unambiguous identification of analytes requires the comparison with authentic standards or the analysis of a purified compound by other techniques, most commonly nuclear magnetic resonance (NMR). There are, however, an ever-growing repertoire of strategies to obtain putative structures or compound classes that can significantly assist data analysis and hypothesis generation (Perez de Souza et al., 2017). MS-DIAL offers a practical interface with some of these commonly used strategies. MS-FINDER provides functionalities for database matching and molecular formula calculation based on stable isotope patterns and can be queried directly from MS-DIAL. Additionally, molecular networking can be applied to the dataset, generating a network based on spectra similarity and feature correlation to identify structurally related compounds.
Materials
- Standard computer with Internet access
- Mass spectrometry-data independent analysis (MS-DIAL; see Tsugawa et al., 2015)
- MS-FINDER (see Tsugawa et al., 2016)
Spectra data curation and standard matching
1.Repeat Basic Protocol 3 and Basic Protocol 4 for both positive and negative ionization modes.
2.Combine results by opening one analysis in MS-DIAL and merging both peak lists in Menu → Post processing → Amalgamation of different polarity peak list.
3.Check which is the actual molecular ion for metabolites of interest.
4.MS-DIAL will automatically match the second order spectra against the uploaded library. The results can be observed in the top right corner of the graphical user interface (GUI) in the Peak and compound information window.
Molecular networking
5.To visualize the network in the browser, set Firefox as the default browser.
6.Proceed with generating the network in Menu → Data visualization → Molecular spectrum networking.
7.To include correlation across multiple samples, select Alignment spots on Search viewer and select the option Export ion abundance correlation among samples.
Support Protocol 3: MOLECULAR NETWORKING USING MetNet
Following data normalization, a major task is to (putatively) annotate mass features as metabolites in the dataset. Many software packages exist that guide the annotation of mass features (Perez de Souza et al., 2017). Here, we will describe the approach from Breitling, Ritchie, Goodenowe, Stewart, and Barrett (2006) using metabolic transformation that was recently integrated as the R/Bioconductor package MetNet (Naake & Fernie, 2019). In addition to the chemical transformation approach, MetNet includes information from statistical association to construct metabolic networks based on the guilt-by-association principle. The protocol here describes the functionality from MetNet (version 1.3.0). As an example, we will describe the functionality of MetNet using a dataset from Solanum pennellii introgression lines (Alseekh et al., 2015) obtained in negative ionization mode. The analysis will focus on the selection of mass features from the dataset relating to hydroxycinnamates and their derivatives and their putative annotation.
Materials
- Computer with Internet access
- R (available for download at https://www.r-project.org/)
- MetNet (see Naake & Fernie, 2019)
1.MetNet is written in the R programming language; ensure that R (version ≥3.5.0) is installed. Install MetNet from Bioconductor and load to the R environment by entering:
- if (!requireNamespace(“BiocManager”, quietly = TRUE))
- install.packages(“BiocManager”)
- BiocManager::install(“MetNet”)
- library(MetNet)
2.Read the table with normalized values (Supplementary dataset 4) into the R environment and convert it to a matrix in mode numeric. Assign row names to a vector derived from m/z (column mz) and retention time values (column rt).
- peaklist <- read.csv(“./path_to_data_normalized.csv/data_normalized.csv”, stringsAsFactors = FALSE, header=TRUE, row.names=1)
- ## convert to matrix and set mode to numeric
- peaklist <- as.matrix(peaklist)
- mode(peaklist) <- “numeric”
- ## set rownames to mz/rt, use rounded m/z and retention time values
- rownames(peaklist) <- paste(round(peaklist[, “mz”], 2), round(peaklist[, “rt”], 1), sep=“/”)
3.Define the transformations that are queried for in the dataset.
-
transformation <- rbind(c(“Hydroxylation (-H)”, “O”, 15.9949146221, “-”),
-
c(“Malonyl group (-H2O)”, “C3H2O3”, 86.0003939305, “+”) ,
-
c(“Rhamnose (-H2O)”, “C6H10O4”, 146.0579088094, “-”) ,
-
c(“Glucose (-H2O)”, “C6H10O5”, 162.0528234315, “-”) ,
-
c(“Disaccharide (-H2O) #1”, “C12H2OO10”, 324.105649, “-”) ,
-
c(“Disaccharide (-H2O) #2”, “C12H2OO11”, 340.1005614851, “-”) ,
-
c(“Trisaccharide (-H2O)”, “C18H30O15”, 486.1584702945, “-”))
-
transformation <- data.frame(group=transformation[, 1],
-
formula=transformation[, 2], mass=as.numeric(transformation[, 3]), rt=transformation[, 4])
-
adj_struct <- createStructuralAdjacency(peaklist, transformation, ppm=20)
4.Optionally, query the adjacency matrix created in step 3 if the predicted transformation aligns with the expected change in polarity. Links between instances that do not agree with the expected polarity change (column rt in transformation) will be removed from the adjacency matrix. The function rtCorrection will correct for the expected changes in retention time.
- adj_struct_rt <- rtCorrection(struct_adj=adj_struct, peaklist, transformation=transformation)
5.Combine information from mass differences and changes in retention time with statistical associations between mass features based on the guilt-by-association principle.
- peaklist_cut <- peaklist[, !colnames(peaklist) %in% c(“mz”, “rt”)]
- adj_stat <- createStatisticalAdjacency(peaklist_cut,
- model = c(“pearson”, “spearman”, “clr”, “aracne”), correlation_adjust = “BH”)
6.Combine the two data sources (structural and statistical information) to an adjacency matrix that only contains those links that are reported by both sources.
- adj_comb <- combineStructuralStatistical(adj_struct_rt[[1]], adj_stat)
7.Further analyze the adjacency matrix of step 6: We will use the igraph framework for analysis and visualization. The function graph_from_adjacency_matrix creates an igraph network from the adjacency matrix of step 6.In the following, the components of the network are retrieved and components that have five or more vertices (corresponding to mass features) will be used for visualization.
- library(igraph)
- net <- graph_from_adjacency_matrix(adj_comb, mode=“undirected”)
- comp <- components(net)
- ## retain only components which have 5 or more vertices for visualization
- comp_red <- names (comp
membership %in% which(comp$csize >= 5)]) - adj_comb_red <- adj_comb[comp_red, comp_red]
- net <- graph_from_adjacency_matrix(adj_comb_red, mode=“undirected”)
8.In our study, we use coumaric acid (C9H8O3, exact mass: 164.047345 u, in negative ionization mode: 163.039520 m/z) as bait to find co-regulated metabolites that share structural similarity based on the metabolic transformation. In the dataset, four mass features correspond to coumaric acid that located to the same component. Highly connected features in the component might correspond to caffeic acid (C9H8O4, exact mass: 180.042260 u, negative ionization mode: 179.034435 m/z), coumaric acid glucoside (C15H18O8, exact mass: 326.100170 u, negative ionization mode: 325.092345 m/z), caffeic acid glucoside (C15H18O9, exact mass: 342.095085 u, negative ionization mode: 341.087260 m/z), and other derivatives that can be inferred by mass difference calculation (Fig. 5).
![Details are in the caption following the image MetNet: Network obtained by MetNet targeting the metabolic transformations hydroxylation, malonylation, rhamnosylation, glucosylation, and addition of two or three glycosyl units. In total, 16 components with ≥5 mass features were obtained. The whole network contained 154,528 undirected links (derived from statistical association) and 536 undirected links (derived from structural mass differences) of which 343 links were shared. Highlighted is a component (component #6) that contains (putative) hydroxycinnamates and derivatives. The m/z value of cinnamic acid, [M − H<sup>+</sup>]<sup>−</sup> 163.039520 m/z, was used as bait to find putative hydroxycinnamates. Component #6 contained 301 and 172 undirected links derived from statistical association and structural mass differences, respectively. The heatmap shows the normalized intensities of mass features of component #6, indicating strong co-regulation between the mass features of component #6.](https://static.yanyin.tech/literature_test/cppb20100-fig-0005-m.jpg)
Support Protocol 4: CO-INJECTION OF AUTHENTIC STANDARDS
All metabolite annotation approaches described so far can at best provide strong evidence of metabolite identities, which can be enough depending on the context but for ultimately determining compound structure by MS, experiments of co-injection should be performed using the authentic standards for the proposed compound. This also can serve as an approach to verify the quantification of a protocol because the standard plus the extract should be quantitatively equal to the sum of both of their own concentrations. Alternatively, well-characterized samples can be mixed with less well-characterized samples and the quantitative recovery assessed.
Additional Materials (also see Basic Protocols 1 and 2)
- Original standard of proposed compound
1.Prepare a stock solution of the authentic standard of the expected metabolite at a concentration of 100 µM.
2.Prepare samples according to Basic Protocol 1 (steps 1-9).
3.Following Basic Protocol 1, step 9, pipet two 100 µl aliquots of the samples and one of resuspension buffer to new 1.5-ml tubes.
4.Spike one sample and resuspension buffer with 10 µl standard stock solution and the other sample with 10 µl resuspension buffer.
5.Transfer all samples to a glass vial for LC-MS analysis and analyze samples according to Basic Protocol 2.
REAGENTS AND SOLUTIONS
Elution buffer A
Using a clean glass pipet, prepare a 0.1% solution (v/v) of ULC/MS grade formic acid (for liquid chromatography; Biosolve) and ultrapure water. Store at room temperature for up to 1 week.
Elution buffer B
Using a clean glass pipet, prepare a 0.1% solution (v/v) of ULC/MS grade formic acid (for liquid chromatography; Biosolve) and ultrapure acetonitrile (ULC/MS grade; Biosolve, cat. no. 01204102). Store at room temperature for up to 2 months.
Elution buffer C
Using a clean glass pipet, prepare a 0.1% solution (v/v) of ULC/MS grade acetic acid and 1% (v/v) 1 M ammonium acetate in ultrapure water. Store at room temperature for up to 1 week.
Elution buffer D
Using a clean graduated cylinder, prepare a 7:3 (v/v) mixture of ultrapure acetonitrile and isopropanol. With a clean glass pipet, add to the mixture 0.1% (v/v) ULC/MS grade acetic acid and 1% (v/v) 1 M ammonium acetate. Store at room temperature for up to 2 months.
Extraction buffer 1
Using a clean graduated cylinder, prepare an 8:2 (v/v) mixture of ultrapure methanol and water. Add the IS (e.g., naringenin-7-O -glucoside) at a final concentration of 5 µg/ml. Store at room temperature for up to 2 months.
Extraction buffer 2
Prepare a stock solution of IS corticosterone (Biosolve UPLC grade) at a concentration of 1 mg/ml in ultrapure methanol (ULC/MS grade; Biosolve, cat. no. 13684102). Add 50 μl stock solution to 25 ml ultrapure methanol.
Prepare a stock solution of IS 1,2-diheptadecanoyl-sn -glycero-3-phosphocholine (Avanti Polar Lipids) at a concentration of 1 mg/ml in chloroform. Add 50 μl stock solution to 75 ml methyl tert -butyl ether (MTBE; HPLC grade, Biosolve, cat. no. 13890602).
Prepare a 75:25 (v/v) mixture of MTBE solution and MeOH solution. Add to 100 ml of that mixture 25 µl of a stock solution of ampicillin [1 mg/ml in water (ULC/MS grade, Biosolve, cat. no. 23214102)] and 50 µl of a stock solution of 13C sorbitol [1 mg/ml in water (ULC/MS grade, Biosolve, cat. no. 23214102)]. Store at 4°C for up to 1 month.
Extraction buffer 3
Using a clean graduated cylinder, prepare a 1:9 (v/v) mixture of ultrapure methanol (ULC/MS grade; Biosolve, cat. no. 13684102) and water (ULC/MS grade; Biosolve, cat. no. 23214102). Store at room temperature for up to 1 week.
Fatty acid methyl esters (FAMEs) mix
- Dissolve the following methyl esters in chloroform (Uvasol® for spectroscopy; Merk, CAS Registry Number 67-66-3) at a final concentration of 0.4 ml/ml for liquid standards or 0.8 mg/ml for solid standards:
- Methyl caprylate (MilliporeSigma, CAS Registry Number 111-11-5)
- Methyl pelargonate (MilliporeSigma, CAS Registry Number 1731-84-6)
- Methyl caprate (MilliporeSigma, CAS Registry Number 110-42-9)
- Methyl laurate (MilliporeSigma, CAS Registry Number 111-82-0)
- Methyl myristate (MilliporeSigma, CAS Registry Number 124-10-7)
- Methyl palmitate (MilliporeSigma, CAS Registry Number 112-39-0)
- Methyl stearate (MilliporeSigma, CAS Registry Number 112-61-8)
- Methyl eicosanoate (MilliporeSigma, CAS Registry Number 1120-28-1)
- Methyl docosanoate (MilliporeSigma, CAS Registry Number 929-77-1)
- Lignoceric acid methyl ester (MilliporeSigma, CAS Registry Number 2442-49-1)
- Methyl hexacosanoate (MilliporeSigma, CAS Registry Number 5802-82-4)
- Methyl octacosanoate (MilliporeSigma, CAS Registry Number 55682-92-3)
- Triacontanoic acid methyl ester (MilliporeSigma, CAS Registry Number 629-83-4)
- Store in 1-ml vials at −20°C for up to 1 year.
Reagent 1
Dissolve methoxyamine hydrochloride (98%; MilliporeSigma, CAS Registry Number 593-56-6) in pyridine (ACS; MilliporeSigma, CAS Registry Number 110-86-1) to a final concentration of 20 mg/ml.
Prepare and use immediately.
Reagent 2
Add FAMEs mix (see recipe) in N -methyl-N -(trimethylsilyl)trifluoroacetamide (MSTFA; Macherey-Nagel, CAS Registry Number 24589-78-4) to a final concentration of 20 µl/ml.
Resuspension buffer 1
Using a clean graduated cylinder, prepare a 7:3 (v/v) mixture of ultrapure acetonitrile (ULC/MS grade; Biosolve, cat. no. 01204102) and isopropanol (ULC/MS grade; Biosolve, cat. no. 16264102). Store at room temperature for up to 2 months.
Resuspension buffer 2
Using a clean graduated cylinder, prepare a 1:1 (v/v) mixture of ultrapure methanol and water. Store at room temperature for up to 2 months.
COMMENTARY
Background Information
Metabolomics platforms
Metabolomics developed in the late 1990s as a companion to other systems biology approaches such as transcriptomics and proteomics (Alseekh & Fernie, 2018). Two major approaches emerged based on the techniques utilized for small molecule characterization: MS and NMR. NMR is considered the gold standard for providing structural elucidation information (Fernie et al., 2011), it can be applied to any class of molecule, and it is an inherently quantitative technique (Markley et al., 2017). It is limited, however, by the complexity in interfacing it with other separation techniques such as chromatography, limiting its application to highly complex mixtures such as the samples usually obtained from plant extracts. MS, on the other hand, has a very well-established interface with both gas and liquid chromatography (GC-MS and LC-MS), providing the means to break down sample complexity on the run and allowing for a much higher throughput in the analytical pipeline (Cajka & Fiehn, 2016). Even though the structural information obtained from MS is significantly less powerful than that obtained from NMR, mass spectra fragmentation patterns still provide important clues about analyte structure, and together with the retention time obtained from the chromatographic dimension, provide a fingerprint that can assist compound identification. Overall, both techniques have different limitations and thereby complement each other. The flexibility of MS is, however, reflected in a much wider application of MS-based metabolomics platforms.
Targeted and untargeted metabolomics
As well as being distinguished on the basis of the technique utilized for metabolite detection and characterization, metabolomics can also be differentiated into targeted and untargeted approaches, with important consequences for data acquisition and analysis (Cajka & Fiehn, 2016; Perez de Souza et al., 2017). As the name implies, targeted approaches focus on detecting previously known compounds expected within the sample. This can be a handful of specific metabolites or many metabolites in a well-characterized sample (e.g., primary metabolites in Arabidopsis). It is particularly common in the first situation to obtain the absolute quantification of these targets, for instance with the use of their respective isotopically labeled standard in MS. When the analysis increases in metabolite coverage, such an approach may become unfeasible, in which case it is more common to aim at relative quantification of all compounds, relative to one or a few ISs, allowing for the comparison of samples run within a single experiment.
Untargeted metabolomics experiments, on the other hand, aim at processing every signal and trying to identify relevant signals from within these much larger datasets that can reach up to several thousand signals. In such experiments, only relative quantification is achieved and a great computational challenge is involved in the exclusion of noise from contaminants and artifacts and combination of redundant signals in compound mass spectra to reduce the number of uninformative/correlated variables and identification of compounds.
Mass spectrometry-based metabolomics
Currently, there are several MS-based systems available, each one with particularities that make them more suitable for some applications than others. Within this article, we focus on those coupled to some chromatographic system, as they are usually the choice for metabolomics analysis. Hence, the first important distinction comes in the chromatographic system that can be either gas or liquid chromatography. GC-MS is a robust system with great resolution in the chromatographic dimension, most often followed by coupling with one of the most reproducible ionization sources, electron ionization (EI; Lisec et al., 2006). Such features make GC-MS systems excellent for the identification of compounds by matching their mass spectra and chromatographic retention indexes, calculated based on internal standards added to the sample against information from standard databases. The greatest limitation of GC-MS is the necessity of analytes to be volatile, either naturally or, more commonly, following a derivatization reaction. It is precisely in this regard that one of the main advantages of LC-MS systems becomes evident. Despite representing a system with lower chromatographic resolution, and significantly lower reproducibility of both the chromatography and the ionization source, often interfaced via electrospray ionization (ESI), LC-MS is extremely versatile and can be adjusted to analyze a broader spectrum of different compounds than other available techniques.
The choice of chromatography method and ionization source (which essentially defines what compounds can be analyzed) are crucial but the mass spectrometer analyzer is also an essential component of the system and it often defines the application. There are a few options of analyzers working upon different physical principles as well as several subtle improvements by different vendors providing specific advantages. We focus here on three of the most generally used in metabolomics and their interesting features. Targeted analysis focuses mainly on achieving high selectivity and sensitivity to quantify even those compounds present at trace levels. For such trace amounts, triple quadrupoles (QQQ) are often used. QQQs are relatively cheap and simple to operate, providing great sensitivity and excellent selectivity in single reaction monitoring (SRM) mode. Untargeted metabolomics, in contrast, represent a big challenge in terms of chromatographic separation and metabolite identification. Therefore, an analyzer capable of providing high scan speed for sampling enough data on sharp UPLC peaks (∼1 to 10 s wide), together with high resolution and MS/MS capabilities for metabolite identification, is highly desirable. Most current untargeted metabolomics platforms rely on one of two different analyzers that provide such features, the Q-Exactive family of Orbitrap and quadrupole time-of-flight (QTofs) systems.
Data processing and analysis
A multitude of tools are available for handling data obtained from metabolomics experiments, focusing on the many different steps following data acquisition. There are also several examples of community efforts in developing pipelines combining several tools in a user friendly way. These approaches can be broken down in a few general steps: Data processing, data analysis, and metabolite annotation. Data processing includes the necessary procedures for extracting the relevant information from raw data and combining all samples for further data analysis. This usually comprises a feature detection and integration step over each sample followed by feature alignment across the different samples. Several different algorithms have been developed to perform such tasks and a few of them are available in some popular freeware such as XCMS (Smith, Want, O'Maille, Abagyan, & Siuzdak, 2006; Tautenhahn, Böttcher, & Neumann, 2008) and MZmine 2 (Pluskal, Castillo, Villar-Briones, & Orešič, 2010).
A recent trend that has been developing in the last few years and is particularly useful in untargeted metabolomics is the data independent acquisition (DIA) of tandem mass spectra. In traditional data-dependent acquisition, specific fragments are selected and fragmented providing a link between parental ions and daughter fragments. DIA experiments, on the other hand, use much broader windows for the selection of parental ions, resulting in a second order spectra containing fragments from all ions in the first order spectra, potentially providing richer structural information but with a disadvantage: Loss of the parental/daughter ion relationship. Processing such data requires extra steps to correlate features and try to re-establish this connection. A few groups have developed extensions to previous methods developed for traditional MS data and MS/MS data but it is worth mentioning here the recent development of mass spectrometry-data independent analysis (MS-DIAL; Tsugawa et al., 2015), with a particular focus on processing DIA MS data. This tool also provides an intuitive graphical user interface with extensions to several other functionalities that facilitate the annotation process.
A common feature of results obtained from processing metabolomics data in an untargeted manner is the overwhelming number of mass features detected, for most cases reaching the order of tens of thousands (Schiffman et al., 2019). A first important consideration in this regard is that these numbers do not represent different metabolites but rather include several fragments and adducts of the same analyte. Identifying and removing these features is often a challenge; while it can lead to some loss of information, the excessive number of correlated variables may compromise statistical models utilized to further analyze data. Some tools, including MS-DIAL (Tsugawa et al., 2015) and CAMERA (Kuhl, Tautenhahn, Böttcher, Larson, & Neumann, 2012), an extension to XCMS, include a step for the identification of possible adducts and fragments. The identification of putative molecular ions by these tools can significantly reduce data complexity and facilitate further metabolite annotation. Additionally, many signals in a mass chromatogram arise from different sources of contamination and/or represent unstable and uninformative signals. It is a crucial step in data analysis to identify and remove such signals. The inclusion of blanks (i.e., extraction without sample, injection of resuspension buffer) and QCs among samples is a valuable way of identifying such signals. Using the coefficient of variation in QC samples or the interclass correlation coefficient as a cut off are a few examples of procedures that can be applied to exclude highly variable signals (Schiffman et al., 2019).
After all cleanup procedures, most datasets still comprise several hundred to a few thousand mass features. The use of traditional statistics such as Student's t -test and analysis of variance (ANOVA) to identify differently accumulated metabolites can still prove useful for data analysis; however, it is often unpractical for the initial steps to generate a hypothesis and draw conclusions based on individual metabolites. Such large datasets usually rely on multivariate statistical analysis such as principal component analysis (PCA) and partial least square discriminant analysis (PLS-DA) to visualize data distribution and identify variables of interest. Many of the software packages available combine common procedures that can be applied for a variety of situations. MetaboAnalyst is a user friendly web platform developed for providing many of these functionalities organized in multiple pipelines commonly used for metabolomics studies (Chong et al., 2018; Current Protocols article: Chong, Wishart, et al., 2019; Chong, Yamamoto, et al., 2019).
Metabolite annotation
It is often stated that the bottleneck in metabolomics is compound identification. Not surprisingly, there is a great effort in trying to constantly improve and develop new strategies for the identification of compounds in a high-throughput way (da Silva et al., 2015). Most traditional MS-based approaches rely on matching spectral features to standard databases or the use of high resolution mass information to calculate putative molecular masses. However, this represents a big issue in terms of limiting the technique to the identification of known previously characterized compounds. It is also important to keep in mind the relevance of the queried databases for the analyzed samples. Using larger databases such as Metlin (Guijas et al., 2018) and MassBank (Horai et al., 2010) will certainly increase the chances of getting more matches. However, opting for plant-specific databases, such as ReSpect (Sawada et al., 2012) and Golm Metabolome Database (GMD; Hummel, Selbig, Walther, & Kopka, 2007), may significantly reduce the number of false positive matches, facilitating further data analysis.
The recent advances in tandem MS acquisition, data processing, and computational power have yielded several more sophisticated approaches aimed at de novo identification of structures based on mass spectral data. One simple approach that has become very popular in the last half decade is the use of molecular networking (Wang et al., 2016). The rationale behind molecular networking is that structurally related compounds originate similar MS2 fragmentation patterns. Hence, it is possible to generate spectra similarity networks, in which clusters represent structurally related compounds. Several works have shown the power of this approach in significantly increasing the number of putatively annotated metabolites by expanding the knowledge from previously identified compounds through these networks. Use of correlation filters is also a good strategy, particularly to reveal co-regulated metabolites, which is often the case for plant-specialized metabolites. MS-DIAL provides a simple interface for both spectra matching and molecular networking directly from its processed data. A less explored variation of molecular networking, available through the MetNet R package (Naake & Fernie, 2019), is based on identifying putative biological reactions using solely differences in mass rather than the whole spectra and has also proven useful in identifying related metabolites within a dataset.
Critical Parameters
Experimental design is the first crucial point to consider when performing a metabolomics experiment. It is essential to evaluate what is the biological question and the effect of sizes expected to be observed, in order to assess the number of replications necessary for obtaining statically significant results. Pilot experiments are not always a possibility but combining them with power analysis is a practical way of observing the effect of size and estimating the number of replications necessary for obtaining statistically significant results for more complex experiments. Moreover, it is important to reduce to a minimum every possible undesirable source of variation that can be introduced in the experiment, such as variations in light irradiance, watering, and substrate composition just to mention a few. Following experimental design, sample extraction is another point in which introduction of error can easily compromise samples. Sample aliquoting of fresh frozen material must be performed quickly, using pre-cooled tubes and spatulas to avoid material from thawing. Small errors introduced by pipetting can be mitigated by correction based on internal standards within extraction buffers. It is strongly recommended that the number of samples extracted be limited; split the experiment into several batches if necessary. Data acquisition in MS-based metabolomics, particularly LC-MS, is prone to fluctuations in intensity and retention time due to factors affecting separation, ionization, and system response. It is crucial for large experiments to include technical replicates of QCs that can be used to normalize such fluctuations. Moreover, the injection of randomized samples can avoid introducing any bias that could affect conclusions.
Understanding Results
An important aim that one should have in mind with data processing is to select optimal parameters in order to integrate as many true signals as possible, while avoiding the integration of excessive noise and peaks with unusual shapes. These signals result in uninformative features after data processing, and therefore, avoiding them will significantly improve further data analysis. Figure 1 is a good example of what the analyst should be checking for. If an excessive number of such bad peaks is present in the results, as represented in Figure 1A, the parameters for peak picking should be optimized.
If data processing and filtering is performed adequately, it should be possible to visualize group separation using data reduction approaches such as PCA. Often the differences between groups will be strong enough that they can be observed even with suboptimal selection of features and normalization procedures. Therefore, it is important to evaluate the improvement of separation following multiple processing steps as represented in Figure 4. Once the optimal feature selection and data normalization procedures are selected, the use of supervised data clustering approaches such as PLS-DA provide a practical visualization of relevant variables based on the VIP scores. Moreover, the observation of such variable distribution across different samples is an effective approach to identify the variables for further analysis.
The initial steps in metabolite annotation rely mostly in spectra matching against databases. It is, however, often the case that no matches can be observed for the interesting variables previously identified. Molecular networking, using both strategies presented in this article, should therefore be used to try establishing putative relationships between multiple metabolites. Such networks can reveal connections to other known metabolites, facilitating the interpretation of the unknowns.
Time Considerations
All protocols may vary significantly depending on the number of samples. Therefore, estimations presented here consider a batch of 30 samples. Basic Protocol 1 takes ∼2.5 hr for the extraction of one batch of samples. Alternate Protocol takes ∼3.5 hr, excluding the time necessary for drying the samples. This can vary depending on the system available but it should be close to 3 hr. Leaving samples to dry overnight is also a possibility and often more convenient. Basic Protocol 2 takes 21 min per sample per ionization mode, resulting in ∼1 day per batch of samples. Support Protocol 2 requires 4 hr for preparing and 12 hr for running one batch of samples. Support Protocol 2 requires ∼16 hr for one batch of samples. Basic Protocol 3 can also vary depending on the final total number of samples, processing power of the computer available, complexity of the chromatogram, and parameters choice. For our training dataset running on a DELL PC with an Intel Core i7-7700K CPU and 16 GB of RAM, it takes ∼1 min per file for data conversion and 30 min to process and align all samples. Including the necessary time to check data quality and adjust any necessary parameters, estimate ∼1 day for working on this protocol. Uploading and analyzing data in the MetaboAnalyst platform for Basic Protocol 4 takes only a few minutes, working on interpreting the data, however, may take another full day. Finally, metabolite annotation is a process that takes several days.
Acknowledgements
L.P.S gratefully acknowledges the European Union project TomGEM: A holistic multi-actor approach towards the design of new tomato varieties and management practices to improve yield and quality in the face of climate change. Project No 679796.
Supporting Information
Filename | Description |
---|---|
Supplementarydataset1dictionaryfile.txt151 B | Supporting Information |
Supplementarydataset2SamplelistILs.xlsx9 KB | Supporting Information |
Supplementarydataset3Metaboanalystdata.csv881.6 KB | Supporting Information |
Supplementarydataset4inputMetNet.csv422.4 KB | Supporting Information |
Please note: The publisher is not responsible for the content or functionality of any supporting information supplied by the authors. Any queries (other than missing content) should be directed to the corresponding author for the article.
Literature Cited
- Alseekh, S., & Fernie, A. R. (2018). Metabolomics 20 years on: What have we learned and what hurdles remain? The Plant Journal , 94(6), 933–942. doi: 10.1111/tpj.13950.
- Alseekh, S., Tohge, T., Wendenberg, R., Scossa, F., Omranian, N., Li, J., … Fernie, A. R. (2015). Identification and mode of inheritance of quantitative trait loci for secondary metabolite abundance in tomato. The Plant Cell , 27(3), 485–512. doi: 10.1105/tpc.114.132266.
- Breitling, R., Ritchie, S., Goodenowe, D., Stewart, M. L., & Barrett, M. P. (2006). Ab initio prediction of metabolic networks using Fourier transform mass spectrometry data. Metabolomics , 2(3), 155–164. doi: 10.1007/s11306-006-0029-z.
- Cajka, T., & Fiehn, O. (2016). Toward merging untargeted and targeted methods in mass spectrometry-based metabolomics and lipidomics. Analytical Chemistry , 88(1), 524–545. doi: 10.1021/acs.analchem.5b04491.
- Chong, J., Soufan, O., Li, C., Caraus, I., Li, S., Bourque, G., … Xia, J. (2018). MetaboAnalyst 4.0: Towards more transparent and integrative metabolomics analysis. Nucleic Acids Research , 46(W1), W486–W494. doi: 10.1093/nar/gky310.
- Chong, J., Wishart, D. S., & Xia, J. (2019). Using MetaboAnalyst 4.0 for comprehensive and integrative metabolomics data analysis. Current Protocols in Bioinformatics , 68(1), e86. doi: 10.1002/cpbi.86.
- Chong, J., Yamamoto, M., & Xia, J. (2019). MetaboAnalystR 2.0: From raw spectra to biological insights. Metabolites , 9(3), 57.
- da Silva, R. R., Dorrestein, P. C., & Quinn, R. A. (2015). Illuminating the dark matter in metabolomics. Proceedings of the National Academy of Sciences , 112(41), 12549–12550. doi: 10.1073/pnas.1516878112.
- Faith, J. J., Hayete, B., Thaden, J. T., Mogno, I., Wierzbowski, J., Cottarel, G., … Gardner, T. S. (2007). Large-scale mapping and validation of Escherichia coli transcriptional regulation from a compendium of expression profiles. PLoS Biology , 5(1), e8. doi: 10.1371/journal.pbio.0050008.
- Fernie, A. R., Aharoni, A., Willmitzer, L., Stitt, M., Tohge, T., Kopka, J., … DeLuca, V. (2011). Recommendations for reporting metabolite data. The Plant Cell , 23(7), 2477–2482. doi: 10.1105/tpc.111.086272.
- Giavalisco, P., Li, Y., Matthes, A., Eckhardt, A., Hubberten, H.-M., Hesse, H., … Willmitzer, L. (2011). Elemental formula annotation of polar and lipophilic metabolites using 13C, 15N and 34S isotope labelling, in combination with high-resolution mass spectrometry. The Plant Journal , 68(2), 364–376. doi: 10.1111/j.1365-313X.2011.04682.x.
- Glauser, G., Veyrat, N., Rochat, B., Wolfender, J.-L., & Turlings, T. C. J. (2013). Ultra-high pressure liquid chromatography–mass spectrometry for plant metabolomics: A systematic comparison of high-resolution quadrupole-time-of-flight and single stage Orbitrap mass spectrometers. Journal of Chromatography A , 1292, 151–159. doi: 10.1016/j.chroma.2012.12.009.
- Guijas, C., Montenegro-Burke, J. R., Domingo-Almenara, X., Palermo, A., Warth, B., Hermann, G., … Siuzdak, G. (2018). METLIN: A technology platform for identifying knowns and unknowns. Analytical Chemistry , 90(5), 3156–3164. doi: 10.1021/acs.analchem.7b04424.
- Horai, H., Arita, M., Kanaya, S., Nihei, Y., Ikeda, T., Suwa, K., … Nishioka, T. (2010). MassBank: A public repository for sharing mass spectral data for life sciences. Journal of Mass Spectrometry , 45(7), 703–714. doi: 10.1002/jms.1777.
- Hummel, J., Segu, S., Li, Y., Irgang, S., Jueppner, J., & Giavalisco, P. (2011). Ultra performance liquid chromatography and high resolution mass spectrometry for the analysis of plant lipids. Frontiers in Plant Science , 2, 54. doi: 10.3389/fpls.2011.00054.
- Hummel, J., Selbig, J., Walther, D., & Kopka, J. (2007). The Golm metabolome database: A database for GC-MS based metabolite profiling. In J. Nielsen & M. C. Jewett (Eds.), Metabolomics: A powerful tool in systems biology (pp. 75–95). Berlin, Heidelberg: Springer Berlin Heidelberg.
- Kuhl, C., Tautenhahn, R., Böttcher, C., Larson, T. R., & Neumann, S. (2012). CAMERA: An integrated strategy for compound spectra extraction and annotation of liquid chromatography/mass spectrometry data sets. Analytical Chemistry , 84(1), 283–289. doi: 10.1021/ac202450g.
- Lisec, J., Schauer, N., Kopka, J., Willmitzer, L., & Fernie, A. R. (2006). Gas chromatography mass spectrometry–based metabolite profiling in plants. Nature Protocols , 1(1), 387–396. doi: 10.1038/nprot.2006.59.
- Livera, A. M. D., Sysi-Aho, M., Jacob, L., Gagnon-Bartsch, J. A., Castillo, S., Simpson, J. A., & Speed, T. P. (2015). Statistical methods for handling unwanted variation in metabolomics data. Analytical Chemistry , 87(7), 3606–3615. doi: 10.1021/ac502439y.
- Margolin, A. A., Nemenman, I., Basso, K., Wiggins, C., Stolovitzky, G., Favera, R. D., & Califano, A. (2006). ARACNE: An algorithm for the reconstruction of gene regulatory networks in a mammalian cellular context. BMC Bioinformatics , 7(1), S7. doi: 10.1186/1471-2105-7-S1-S7.
- Markley, J. L., Brüschweiler, R., Edison, A. S., Eghbalnia, H. R., Powers, R., Raftery, D., & Wishart, D. S. (2017). The future of NMR-based metabolomics. Current Opinion in Biotechnology , 43, 34–40. doi: 10.1016/j.copbio.2016.08.001.
- Naake, T., & Fernie, A. R. (2019). MetNet: Metabolite network prediction from high-resolution mass spectrometry data in R aiding metabolite annotation. Analytical Chemistry , 91(3), 1768–1772. doi: 10.1021/acs.analchem.8b04096.
- Perez de Souza, L., Naake, T., Tohge, T., & Fernie, A. R. (2017). From chromatogram to analyte to metabolite. How to pick horses for courses from the massive web resources for mass spectral plant metabolomics. GigaScience , 6(7), 1–20. doi: 10.1093/gigascience/gix037.
- Pluskal, T., Castillo, S., Villar-Briones, A., & Orešič, M. (2010). MZmine 2: Modular framework for processing, visualizing, and analyzing mass spectrometry-based molecular profile data. BMC Bioinformatics , 11(1), 395. doi: 10.1186/1471-2105-11-395.
- Sawada, Y., Nakabayashi, R., Yamada, Y., Suzuki, M., Sato, M., Sakata, A., … Saito, K. (2012). RIKEN tandem mass spectral database (ReSpect) for phytochemicals: A plant-specific MS/MS-based data resource and database. Phytochemistry , 82, 38–45. doi: 10.1016/j.phytochem.2012.07.007.
- Schiffman, C., Petrick, L., Perttula, K., Yano, Y., Carlsson, H., Whitehead, T., … Dudoit, S. (2019). Filtering procedures for untargeted LC-MS metabolomics data. BMC Bioinformatics , 20(1), 334. doi: 10.1186/s12859-019-2871-9.
- Smith, C. A., Want, E. J., O'Maille, G., Abagyan, R., & Siuzdak, G. (2006). XCMS: Processing mass spectrometry data for metabolite profiling using nonlinear peak alignment, matching, and identification. Analytical Chemistry , 78(3), 779–787. doi: 10.1021/ac051437y.
- Tautenhahn, R., Böttcher, C., & Neumann, S. (2008). Highly sensitive feature detection for high resolution LC/MS. BMC Bioinformatics , 9(1), 504. doi: 10.1186/1471-2105-9-504.
- Tohge, T., & Fernie, A. R. (2010). Combining genetic diversity, informatics and metabolomics to facilitate annotation of plant gene function. Nature Protocols , 5, 1210–1227. doi: 10.1038/nprot.2010.82.
- Tsugawa, H., Cajka, T., Kind, T., Ma, Y., Higgins, B., Ikeda, K., … Arita, M. (2015). MS-DIAL: Data-independent MS/MS deconvolution for comprehensive metabolome analysis. Nature Methods , 12, 523–526. doi: 10.1038/nmeth.3393. Retrieved from https://www.nature.com/articles/nmeth.3393#supplementary-information.
- Tsugawa, H., Kind, T., Nakabayashi, R., Yukihira, D., Tanaka, W., Cajka, T., … Arita, M. (2016). Hydrogen rearrangement rules: Computational MS/MS fragmentation and structure elucidation using MS-FINDER software. Analytical Chemistry , 88(16), 7946–7958. doi: 10.1021/acs.analchem.6b00770.
- Wang, M., Carver, J. J., Phelan, V. V., Sanchez, L. M., Garg, N., Peng, Y., … Bandeira, N. (2016). Sharing and community curation of mass spectrometry data with Global Natural Products Social Molecular Networking. Nature Biotechnology , 34, 828–837. doi: 10.1038/nbt.3597. Retrieved from https://www.nature.com/articles/nbt.3597#supplementary-information.
Citing Literature
Number of times cited according to CrossRef: 63
- Rafael Paiva, Manuela Correia, Cristina Delerue-Matos, Joana S. Amaral, Adulteration of Brain Health (Cognitive, Mood, and Sleep Enhancement) Food Supplements by the Addition of Pharmaceutical Drugs: A Comprehensive Review of Analytical Approaches and Trends, Foods, 10.3390/foods13060908, 13 , 6, (908), (2024).
- Anastasia Apodiakou, Saleh Alseekh, Rainer Hoefgen, Sarah J. Whitcomb, Overexpression of SLIM1 transcription factor accelerates vegetative development in Arabidopsis thaliana, Frontiers in Plant Science, 10.3389/fpls.2024.1327152, 15 , (2024).
- Pratibha Demiwal, Sumedh Tayade, Shri Ram Yadav, Debabrata Sircar, A metabolomics perspective on root‐derived plant immunity and phytohormone interaction, Physiologia Plantarum, 10.1111/ppl.14150, 176 , 1, (2024).
- Arthur Gessler, Thomas Wieloch, Matthias Saurer, Marco M. Lehmann, Roland A. Werner, Bernd Kammerer, The marriage between stable isotope ecology and plant metabolomics – new perspectives for metabolic flux analysis and the interpretation of ecological archives, New Phytologist, 10.1111/nph.19973, 244 , 1, (21-31), (2024).
- Abdullah R. Alzahrani, Nora Hosny, Doaa I. Mohamed, Hebatallah H. Abo Nahas, Abdulaziz Albogami, Tahani Mohamed Ibrahim Al-Hazani, Ibrahim Abdel Aziz Ibrahim, Alaa Hisham Falemban, Ghazi A. Bamagous, Essa M. Saied, Unveiling the multifaceted antiproliferative efficacy of Cichorium endivia root extract by dual modulation of apoptotic and inflammatory genes, inducing cell cycle arrest, and targeting COX-2 , RSC Advances, 10.1039/D4RA02131B, 14 , 27, (19400-19427), (2024).
- Anna Mascellani Bergo, Kirsten Leiss, Jaroslav Havlik, Twenty Years of 1 H NMR Plant Metabolomics: A Way Forward toward Assessment of Plant Metabolites for Constitutive and Inducible Defenses to Biotic Stress , Journal of Agricultural and Food Chemistry, 10.1021/acs.jafc.3c09362, 72 , 15, (8332-8346), (2024).
- Jeanaflor Crystal T. Concepcion, Shiv S. Kaundun, James A. Morris, Autumn N. Brandenburg, Dean E. Riechers, Metabolism of the 4-Hydroxyphenylpyruvate Dioxygenase Inhibitor, Mesotrione, in Multiple-Herbicide-Resistant Palmer amaranth ( Amaranthus palmeri ) , Journal of Agricultural and Food Chemistry, 10.1021/acs.jafc.3c06903, 72 , 11, (5595-5608), (2024).
- Pinki Kumari Singh, Nagasathiya Krishnan, Pachaiappan Raman, Quantification of hormones and profiling of metabolites from vegetative to reproductive growth phase shift in Lagenaria siceraria (Molina) Standl, Scientia Horticulturae, 10.1016/j.scienta.2023.112464, 323 , (112464), (2024).
- Nuti Hutasingh, Apinya Tubtimrattana, Pornkanok Pongpamorn, Putthamas Pewlong, Atchara Paemanee, Nat Tansrisawad, Ubonrat Siripatrawan, Supaart Sirikantaramas, Unraveling the effects of drying techniques on chaya leaves: Metabolomics analysis of nonvolatile and volatile metabolites, umami taste, and antioxidant capacity, Food Chemistry, 10.1016/j.foodchem.2024.138769, 446 , (138769), (2024).
- Sajedeh Rezaei Cherati, Mariya V. Khodakovskaya, Identification of Stress-Responsive Metabolites in Plants Using an Untargeted Metabolomics Approach, Plant Stress Tolerance, 10.1007/978-1-0716-3973-3_12, (171-182), (2024).
- Youjun Zhang, Kezhen Qin, Alisdair R. Fernie, Plant Tissue Culture and Metabolite Profiling for High-Value Natural Product Synthesis, Plant Cell Culture Protocols, 10.1007/978-1-0716-3954-2_27, (405-416), (2024).
- Anish Kaachra, Anish Tamang, Vipin Hallan, An Expedited Qualitative Profiling of Free Amino Acids in Plant Tissues Using Liquid Chromatography‐Mass Spectrometry (LC–MS) in Conjunction With MS‐DIAL, Journal of Mass Spectrometry, 10.1002/jms.5094, 59 , 10, (2024).
- Zili Guo, Jiangxuan Lai, Yiwen Wu, Sheng Fang, Xianrui Liang, Investigation on Antioxidant Activity and Different Metabolites of Mulberry (Morus spp.) Leaves Depending on the Harvest Months by UPLC–Q-TOF-MS with Multivariate Tools, Molecules, 10.3390/molecules28041947, 28 , 4, (1947), (2023).
- Ewart Smith, Ainsely Lewis, Suresh S. Narine, R. J. Neil Emery, Unlocking Potentially Therapeutic Phytochemicals in Capadulla (Doliocarpus dentatus) from Guyana Using Untargeted Mass Spectrometry-Based Metabolomics, Metabolites, 10.3390/metabo13101050, 13 , 10, (1050), (2023).
- Pierluigi Reveglia, Francisco J. Agudo-Jurado, Eleonora Barilli, Marco Masi, Antonio Evidente, Diego Rubiales, Uncovering Phytotoxic Compounds Produced by Colletotrichum spp. Involved in Legume Diseases Using an OSMAC–Metabolomics Approach, Journal of Fungi, 10.3390/jof9060610, 9 , 6, (610), (2023).
- Seon-Woo Oh, Muhammad Imran, Eun-Ha Kim, Soo-Yun Park, Sang-Gu Lee, Hyoun-Min Park, Jung-Won Jung, Tae-Hun Ryu, Approach strategies and application of metabolomics to biotechnology in plants, Frontiers in Plant Science, 10.3389/fpls.2023.1192235, 14 , (2023).
- Fernanda R. Castro-Moretti, Jean-Christophe Cocuron, Humberto Castillo-Gonzalez, Efrain Escudero-Leyva, Priscila Chaverri, Oliveiro Guerreiro-Filho, Jason C. Slot, Ana Paula Alonso, A metabolomic platform to identify and quantify polyphenols in coffee and related species using liquid chromatography mass spectrometry, Frontiers in Plant Science, 10.3389/fpls.2022.1057645, 13 , (2023).
- Leonardo Perez de Souza, Elena Bitocchi, Roberto Papa, Takayuki Tohge, Alisdair R. Fernie, Decreased metabolic diversity in common beans associated with domestication revealed by untargeted metabolomics, information theory, and molecular networking, The Plant Journal, 10.1111/tpj.16277, 115 , 4, (1021-1036), (2023).
- Liliana Grazina, Isabel Mafra, Linda Monaci, Joana S. Amaral, Mass spectrometry‐based approaches to assess the botanical authenticity of dietary supplements, Comprehensive Reviews in Food Science and Food Safety, 10.1111/1541-4337.13222, 22 , 5, (3870-3909), (2023).
- Marko Bertić, Ina Zimmer, David Andrés-Montaner, Maaria Rosenkranz, Jaakko Kangasjärvi, Jörg-Peter Schnitzler, Andrea Ghirardo, Automatization of metabolite extraction for high-throughput metabolomics: case study on transgenic isoprene-emitting birch, Tree Physiology, 10.1093/treephys/tpad087, 43 , 10, (1855-1869), (2023).
- Philipp Westhoff, Andreas P M Weber, The role of metabolomics in informing strategies for improving photosynthesis, Journal of Experimental Botany, 10.1093/jxb/erad508, 75 , 6, (1696-1713), (2023).
- Ahmed Zayed, Mohamed A. Farag, Alexander Mehring, Mohamed A. Salem, Rana M. Ibrahim, Saleh Alseekh, Alisdair R. Fernie, Roland Ulber, Methyl jasmonate elicitation effect on the metabolic profile of cambial meristematic cells culture derived from sweet basil (Ocimum basilicum L.) in relation to antioxidant activity: Untargeted metabolomics study in a time-based approach, Phytochemistry, 10.1016/j.phytochem.2023.113777, 213 , (113777), (2023).
- Youjun Zhang, Lorenz Wiese, Hao Fang, Saleh Alseekh, Leonardo Perez de Souza, Federico Scossa, John J. Molloy, Mathias Christmann, Alisdair R. Fernie, Synthetic biology identifies the minimal gene set required for paclitaxel biosynthesis in a plant chassis, Molecular Plant, 10.1016/j.molp.2023.10.016, 16 , 12, (1951-1961), (2023).
- Shuangqian Shen, Chuansong Zhan, Chenkun Yang, Alisdair R. Fernie, Jie Luo, Metabolomics-centered mining of plant metabolic diversity and function: Past decade and future perspectives, Molecular Plant, 10.1016/j.molp.2022.09.007, 16 , 1, (43-63), (2023).
- Eric T. Dziekonski, Gregory S. Eakins, R. Graham Cooks, Enabling one- and two-dimensional mass spectrometry in a linear quadrupole ion trap, International Journal of Mass Spectrometry, 10.1016/j.ijms.2023.117099, 491 , (117099), (2023).
- Syed Muhammad Zaki Shah, Muhammad Ramzan, Muhammad Noman Khan, Hamna Shadab, Muhammad Usman, Saeedur Rahman, Arslan Ali, Jalal Uddin, Mufarreh Asmari, Syed Ghulam Musharraf, Untargeted screening of plant metabolites based on data-independent and data-dependent acquisition modes using LC-ESI-QTOF-MS: Tribulus terrestris L. as a case study, Arabian Journal of Chemistry, 10.1016/j.arabjc.2023.104978, 16 , 8, (104978), (2023).
- Dicky Aldian, Laila Dini Harisa, Hiroki Mitsuishi, Ke Tian, Atsushi Iwasawa, Masato Yayota, Diverse forage improves lipid metabolism and antioxidant capacity in goats, as revealed by metabolomics, animal, 10.1016/j.animal.2023.100981, 17 , 10, (100981), (2023).
- Domenico Montesano, Monica Gallo, Sustainable Approaches for the Extraction and Characterization of Phytochemicals from Food Matrices, Sustainable Food Science - A Comprehensive Approach, 10.1016/B978-0-12-823960-5.00055-X, (103-118), (2023).
- Jeffrey D. Weidenhamer, Don Cipollini, Kathryn Morris, Saliya Gurusinghe, Leslie A. Weston, Ecological realism and rigor in the study of plant-plant allelopathic interactions, Plant and Soil, 10.1007/s11104-023-06022-6, 489 , 1-2, (1-39), (2023).
- Marisa Maia, Stefania Savoi, Andreia Figueiredo, Deciphering Plant-Pathogen Interactions Through Plant Metabolomics: From Technical Advances to Applied Research, Metabolomics, 10.1007/978-3-031-39094-4_11, (363-385), (2023).
- Deepu Pandita, Orchestration of Omics Technologies for Crop Improvement, Climate-Resilient Agriculture, Vol 2, 10.1007/978-3-031-37428-9_34, (771-791), (2023).
- Miriam Pérez-Cova, Romà Tauler, Joaquim Jaumot, Adverse Effects of Arsenic Uptake in Rice Metabolome and Lipidome Revealed by Untargeted Liquid Chromatography Coupled to Mass Spectrometry (LC-MS) and Regions of Interest Multivariate Curve Resolution, Separations, 10.3390/separations9030079, 9 , 3, (79), (2022).
- Edcyl Lee O. Salac, Michael Russelle Alvarez, Rnie Shayne Gaurana, Sheryl Joyce B. Grijaldo, Luster Mae Serrano, Florence de Juan, Rowell Abogado, Isagani Padolina Jr., Froila Marie Deniega, Kimberly Delica, Kimberly Fernandez, Carlito B. Lebrilla, Marlon N. Manalo, Francisco M. Heralde III, Gladys Cherisse J. Completo, Ruel C. Nacario, Biological Assay-Guided Fractionation and Mass Spectrometry-Based Metabolite Profiling of Annona muricata L. Cytotoxic Compounds against Lung Cancer A549 Cell Line, Plants, 10.3390/plants11182380, 11 , 18, (2380), (2022).
- Christoph Seger, Sonja Sturm, NMR-Based Chromatography Readouts: Indispensable Tools to “Translate” Analytical Features into Molecular Structures, Cells, 10.3390/cells11213526, 11 , 21, (3526), (2022).
- Thorsten Stefan, Xu Na Wu, Youjun Zhang, Alisdair Fernie, Waltraud X. Schulze, Regulatory Modules of Metabolites and Protein Phosphorylation in Arabidopsis Genotypes With Altered Sucrose Allocation, Frontiers in Plant Science, 10.3389/fpls.2022.891405, 13 , (2022).
- Vassya Bankova, Innovative Approaches to Phytochemical Analysis, The Natural Products Journal, 10.2174/2210315511666211119122945, 12 , 5, (2022).
- Cyril Abadie, Julie Lalande, Guillaume Tcherkez, Exact mass GC‐MS analysis: Protocol, database, advantages and application to plant metabolic profiling, Plant, Cell & EnvironmentPlant, Cell & EnvironmentPlant, Cell & Environment, 10.1111/pce.14407, 45 , 10, (3171-3183), (2022).
- Wadzani Palnam Dauda, Virendra Singh Rana, Amolkumar U. Solanke, Gopala Krishnan, Bishnu Maya Bashya, Rashmi Aggarwal, Veerubommu Shanmugam, Metabolomic analysis of sheath blight disease of rice (Oryza sativa L.) induced by Rhizoctonia solani phytotoxin, Journal of Applied Microbiology, 10.1111/jam.15776, 133 , 5, (3215-3227), (2022).
- Yang Gao, Wolfram Thiele, Omar Saleh, Federico Scossa, Fayezeh Arabi, Hongmou Zhang, Arun Sampathkumar, Kristina Kühn, Alisdair Fernie, Ralph Bock, Mark A Schöttler, Reimo Zoschke, Chloroplast translational regulation uncovers nonessential photosynthesis genes as key players in plant cold acclimation, The Plant Cell, 10.1093/plcell/koac056, 34 , 5, (2056-2079), (2022).
- Abner Herbert Lim, Zhen Jie Low, Prashant Narendra Shingate, Jing Han Hong, Shu Chen Chong, Cedric Chuan Young Ng, Wei Liu, Robert Vaser, Mile Šikić, Wing-Kin Ken Sung, Niranjan Nagarajan, Patrick Tan, Bin Tean Teh, Genome assembly and chemogenomic profiling of National Flower of Singapore Papilionanthe Miss Joaquim ‘Agnes’ reveals metabolic pathways regulating floral traits, Communications Biology, 10.1038/s42003-022-03940-6, 5 , 1, (2022).
- Youjun Zhang, Alisdair R. Fernie, Metabolite profiling of Arabidopsis mutants of lower glycolysis, Scientific Data, 10.1038/s41597-022-01673-z, 9 , 1, (2022).
- Zhihao Liu, Mengliang Zhang, Pei Chen, James M. Harnly, Jianghao Sun, Mass Spectrometry-Based Nontargeted and Targeted Analytical Approaches in Fingerprinting and Metabolomics of Food and Agricultural Research, Journal of Agricultural and Food Chemistry, 10.1021/acs.jafc.2c01878, 70 , 36, (11138-11153), (2022).
- Sidratul Nur Muntaha, Xiaoping Li, Julia Compart, Ardha Apriyanto, Joerg Fettke, Carbon pathways during transitory starch degradation in Arabidopsis differentially affect the starch granule number and morphology in the dpe2/phs1 mutant background, Plant Physiology and Biochemistry, 10.1016/j.plaphy.2022.03.033, 180 , (35-41), (2022).
- Chenkun Yang, Shuangqian Shen, Shen Zhou, Yufei Li, Yuyuan Mao, Junjie Zhou, Yuheng Shi, Longxu An, Qianqian Zhou, Wenju Peng, Yuanyuan Lyu, Xuemei Liu, Wei Chen, Shouchuang Wang, Lianghuan Qu, Xianqing Liu, Alisdair R. Fernie, Jie Luo, Rice metabolic regulatory network spanning the entire life cycle, Molecular Plant, 10.1016/j.molp.2021.10.005, 15 , 2, (258-275), (2022).
- Dina A. Selim, Eman Shawky, Rasha M. Abu El-Khair, Identification of the discriminatory chemical markers of different grades of Sri Lankan white, green and black tea (Camellia sinenesis L.) via metabolomics combined to chemometrics, Journal of Food Composition and Analysis, 10.1016/j.jfca.2022.104473, 109 , (104473), (2022).
- Jeffrey Simpson, Clint Chapple, Tag you're it: Application of stable isotope labeling and LC-MS to identify the precursors of specialized metabolites in plants, Biochemical Pathways and Environmental Responses in Plants: Part A, 10.1016/bs.mie.2022.07.039, (279-303), (2022).
- Valentina Calabrese, Isabelle Schmitz-Afonso, Candice Prevost, Carlos Afonso, Abdelhakim Elomri, Molecular networking and collision cross section prediction for structural isomer and unknown compound identification in plant metabolomics: a case study applied to Zhanthoxylum heitzii extracts, Analytical and Bioanalytical Chemistry, 10.1007/s00216-022-04059-7, 414 , 14, (4103-4118), (2022).
- Feng Zhu, Alisdair R. Fernie, Federico Scossa, Preparation and Curation of Omics Data for Genome-Wide Association Studies, Genome-Wide Association Studies, 10.1007/978-1-0716-2237-7_8, (127-150), (2022).
- Luisa Mattoli, Mattia Gianni, Michela Burico, Mass spectrometry‐based metabolomic analysis as a tool for quality control of natural complex products, Mass Spectrometry Reviews, 10.1002/mas.21773, 42 , 4, (1358-1396), (2022).
- Hyung Min Kim, Jong Seong Kang, Metabolomic Studies for the Evaluation of Toxicity Induced by Environmental Toxicants on Model Organisms, Metabolites, 10.3390/metabo11080485, 11 , 8, (485), (2021).
- Junjie Xia, Zili Guo, Sheng Fang, Jinping Gu, Xianrui Liang, Effect of Drying Methods on Volatile Compounds of Burdock (Arctium lappa L.) Root Tea as Revealed by Gas Chromatography Mass Spectrometry-Based Metabolomics, Foods, 10.3390/foods10040868, 10 , 4, (868), (2021).
- Hui Yan, Zong-Jin Pu, Zhen-Yu Zhang, Gui-Sheng Zhou, Dong-Qian Zou, Sheng Guo, Chao Li, Zhi-Lai Zhan, Jin-Ao Duan, Research on Biomarkers of Different Growth Periods and Different Drying Processes of Citrus wilsonii Tanaka Based on Plant Metabolomics, Frontiers in Plant Science, 10.3389/fpls.2021.700367, 12 , (2021).
- Hongxu Zhou, Yi Zhang, Hui Liang, Huijie Song, Jiaming Zhao, Li Liu, Jun Zeng, Lei Sun, Shuangcheng Ma, Dali Meng, A novel multidimensional strategy to evaluate Belamcanda chinensis (L) DC and Iris tectorum Maxim based on plant metabolomics, digital reference standard analyzer and biological activities evaluation, Chinese Medicine, 10.1186/s13020-021-00494-3, 16 , 1, (2021).
- Silvio A. Cândido‐Sobrinho, Valéria F. Lima, Francisco B. S. Freire, Leonardo P. Souza, Jorge Gago, Alisdair R. Fernie, Danilo M. Daloso, Metabolism‐mediated mechanisms underpin the differential stomatal speediness regulation among ferns and angiosperms, Plant, Cell & EnvironmentPlant, Cell & EnvironmentPlant, Cell & Environment, 10.1111/pce.14232, 45 , 2, (296-311), (2021).
- Federico Scossa, Saleh Alseekh, Alisdair R. Fernie, Integrating multi-omics data for crop improvement, Journal of Plant Physiology, 10.1016/j.jplph.2020.153352, 257 , (153352), (2021).
- Dominique Rolin, Catherine Bennetau-Pelissero, Sarada D. Tetali, Pierre Pétriacq, When dietary supplements meet metabolomics: A fast-evolving field—A follow-up of ABR volume 67: Metabolomics coming of age with its technological diversity, Past, Current and Future Topics, 10.1016/bs.abr.2021.01.009, (329-354), (2021).
- Alex Williams, Jordi Gamir, Antoine Gravot, Pierre Pétriacq, Untangling plant immune responses through metabolomics, Plant Metabolomics in full swing, 10.1016/bs.abr.2020.09.017, (73-105), (2021).
- Zong‐Jin Pu, Shuo Zhang, Yu‐Ping Tang, Xu‐Qin Shi, Hui‐Juan Tao, Hui Yan, Jia‐Qian Chen, Shi‐Jun Yue, Yan‐Yan Chen, Zhen‐Hua Zhu, Gui‐Sheng Zhou, Shu‐Lan Su, Jin‐Ao Duan, Study on changes in pigment composition during the blooming period of safflower based on plant metabolomics and semi‐quantitative analysis, Journal of Separation Science, 10.1002/jssc.202100439, 44 , 22, (4082-4091), (2021).
- Gerjen H. Tinnevelt, Udo F.H. Engelke, Ron A. Wevers, Stefanie Veenhuis, Michel A. Willemsen, Karlien L.M. Coene, Purva Kulkarni, Jeroen J. Jansen, Variable Selection in Untargeted Metabolomics and the Danger of Sparsity, Metabolites, 10.3390/metabo10110470, 10 , 11, (470), (2020).
- Sándor Gonda, Special Issue: Plant Metabolomics, Metabolites, 10.3390/metabo10110467, 10 , 11, (467), (2020).
- Sanghamitra Majumdar, Arturo A. Keller, Omics to address the opportunities and challenges of nanotechnology in agriculture, Critical Reviews in Environmental Science and Technology, 10.1080/10643389.2020.1785264, 51 , 22, (2595-2636), (2020).
- Brijesh Kumar, K. P. Madhusudanan, Role of Mass Spectrometry in Modern Herbal Drug Research, Biophysical and Computational Tools in Drug Discovery, 10.1007/7355_2020_107, (33-64), (2020).
- Taly Lapidot‐Cohen, Leah Rosental, Yariv Brotman, Liquid Chromatography–Mass Spectrometry (LC‐MS)‐Based Analysis for Lipophilic Compound Profiling in Plants, Current Protocols in Plant Biology, 10.1002/cppb.20109, 5 , 2, (2020).