Measuring Protein-Protein Interactions and Quantifying Their Dissociation Constants with Mass Photometry
Zornitsa Kofinova, Zornitsa Kofinova, Gogulan Karunanithy, Gogulan Karunanithy, Ana Sofia Ferreira, Ana Sofia Ferreira, Weston B. Struwe, Weston B. Struwe
antibodies
binding affinity
complex formation
dissociation constant
KD
protein A
protein-protein interactions
Abstract
Protein-protein interactions underlie most biological processes, and determining the affinity and abundance of binding partners for each interaction is often a challenging task because these interactions often involve multiple ligands and binding sites. Standard methods for determining the affinity of protein interactions often require a large amount of starting material in addition to potentially disruptive labeling or immobilization of the binding partners. Mass photometry is a bioanalytical technique that measures the mass of single biomolecules in solution, quickly and with minimal sample requirements. This article describes how mass photometry can be used to determine the mass distribution of binding partners, the complexes they form, the relative abundance of each species, and, accordingly, the dissociation constant (KD) of their interactions. © 2024 Refeyn Ltd. Current Protocols published by Wiley Periodicals LLC.
Basic Protocol : Using mass photometry to measure protein-protein binding and quantify the KD of this interaction
INTRODUCTION
Most biological processes are performed and regulated by protein-protein interactions and their wider networks. To gain a mechanistic understanding of these processes, determining the affinity of interactions is pivotal. This can be a challenging task because protein interactions often involve the binding of multivalent ligands to multiple binding sites, which cannot be determined by ensemble-based methods (Soltermann et al., 2020).
Standard methods for determining the affinity of protein interactions include isothermal titration calorimetry (ITC), microscale thermophoresis (MST), surface plasmon resonance (SPR), and biolayer interferometry (BLI) (Jerabek-Willemsen et al., 2014; Khalili et al., 2018; Noy-Porat et al., 2021; Wu & Piszczek, 2020). ITC measures the heat changes that occur when two molecules bind. This provides valuable insights into binding thermodynamics, yielding parameters such as dissociation constants (KD), stoichiometry (n), enthalpy (∆H), and entropy (∆S). However, it is a laborious technique that requires a large amount of starting material (in the μM range) (Atri et al., 2015). MST can determine binding kinetics by detecting the diffusion of molecules in a temperature gradient. It requires lower sample consumption than ITC (typically in the nM range) but involves the use of hydrophobic fluorescent labels that can cause nonspecific binding effects (Wienken et al., 2010). SPR is highly used for measuring binding interactions by monitoring changes in the refractive index at the sensor surface. It provides valuable information on association (kon) and dissociation (koff) rates, as well as KD measurements. SPR requires less starting material than ITC (typically in the nM range) but involves the immobilization of one of the binding partners (Helmerhorst et al., 2012). Similarly to SPR, BLI is a label-free optical biosensing technology that can also be used to measure binding kinetics. Like SPR, it necessitates the immobilization of one of the binding partners but boasts high-throughput capabilities. Additionally, in comparison to SPR and MST, it has relatively high sample consumption, typically in the μM range (Dubrow et al., 2022).
This article describes how to use an alternative technology, termed mass photometry, to quantify the affinity of protein-protein interactions in a simple and rapid approach that does not require protein immobilization or extensive sample preparation. Mass photometry is a label-free bioanalytical technique that measures the mass of single biomolecules in solution (Young et al., 2018). Specifically, it reports the mass distribution of species that can be used to quantify the relative concentration of binding partners and complexes by molecular counting (Soltermann et al., 2020; Wu & Piszczek, 2021). By knowing the number of bound and unbound species, one can easily calculate interaction affinities in a so-called single-shot KD. This approach is also attractive because each measurement takes just a few minutes and uses little sample (in the concentration range of 100 pM to 100 nM), making it straightforward to run repeat measurements, test different conditions, and vary the ratios and concentrations of the binding partners.
Our simple protocol describes the use of mass photometry to characterize and quantify the affinity of two protein-protein interaction systems, specifically protein A with two immunoglobulin G (IgG) antibodies from different species (human and bovine). The approach is founded on the accurate measurement of the molecular weights (MWs) of binding partners, their stoichiometries, and the relative abundances of the bound and unbound species. The data intrinsically reveal the complexity of the interactions in each system (e.g., multivalent binding), with multiple complexes forming in each case. The Basic Protocol describes how to calculate the dissociation constants (KD) for each interaction studied using mass photometry, confirming that protein A has a stronger affinity to human IgG than to bovine IgG, in accordance to published data (Hage & Cazes, 2005).
Basic Protocol: USING MASS PHOTOMETRY TO MEASURE PROTEIN-PROTEIN BINDING AND QUANTIFY THE KD OF THIS INTERACTION
This protocol outlines the steps to perform an experiment for measuring protein-protein interactions with mass photometry. Here, the example system is the interaction between two IgG antibodies, one human and one bovine, and protein A.
Antibody-binding proteins, such as protein A, are pivotal in antibody purification processes and diverse applications like immunoprecipitation (IP) and chromatin IP (ChIP). Protein A is reported to have a strong affinity to human IgG but weaker affinity to other animal-derived immunoglobulins (Drikic et al., 2019).
Mass photometry has been used to investigate the binding affinity of human and bovine IgG to protein A and whether it confirms previously published affinity chromatography data, indicating weaker affinity of the bovine IgG (Hage & Cazes, 2005). Indeed, mass photometry data show clear differences in the formation of antibody–protein A complexes when IgGs of different origins are tested. More specifically, due to the stronger interaction of human IgG with protein A, the results not only show a higher relative concentration of the human IgG–protein A complex but also allow the identification of higher-order oligomeric complexes compared to experiments using bovine IgG (Fig. 1). To identify the mass of the measured species, β-amylase is used as a calibrant. From these measurements, it is possible to calculate the equilibrium KD for each interaction (Fig. 2). Each sample is measured in triplicate, and data acquisition and analysis are performed using AcquireMP and DiscoverMP software, respectively. The KD calculations are performed using Microsoft Excel (see Supporting Information, Data 2).
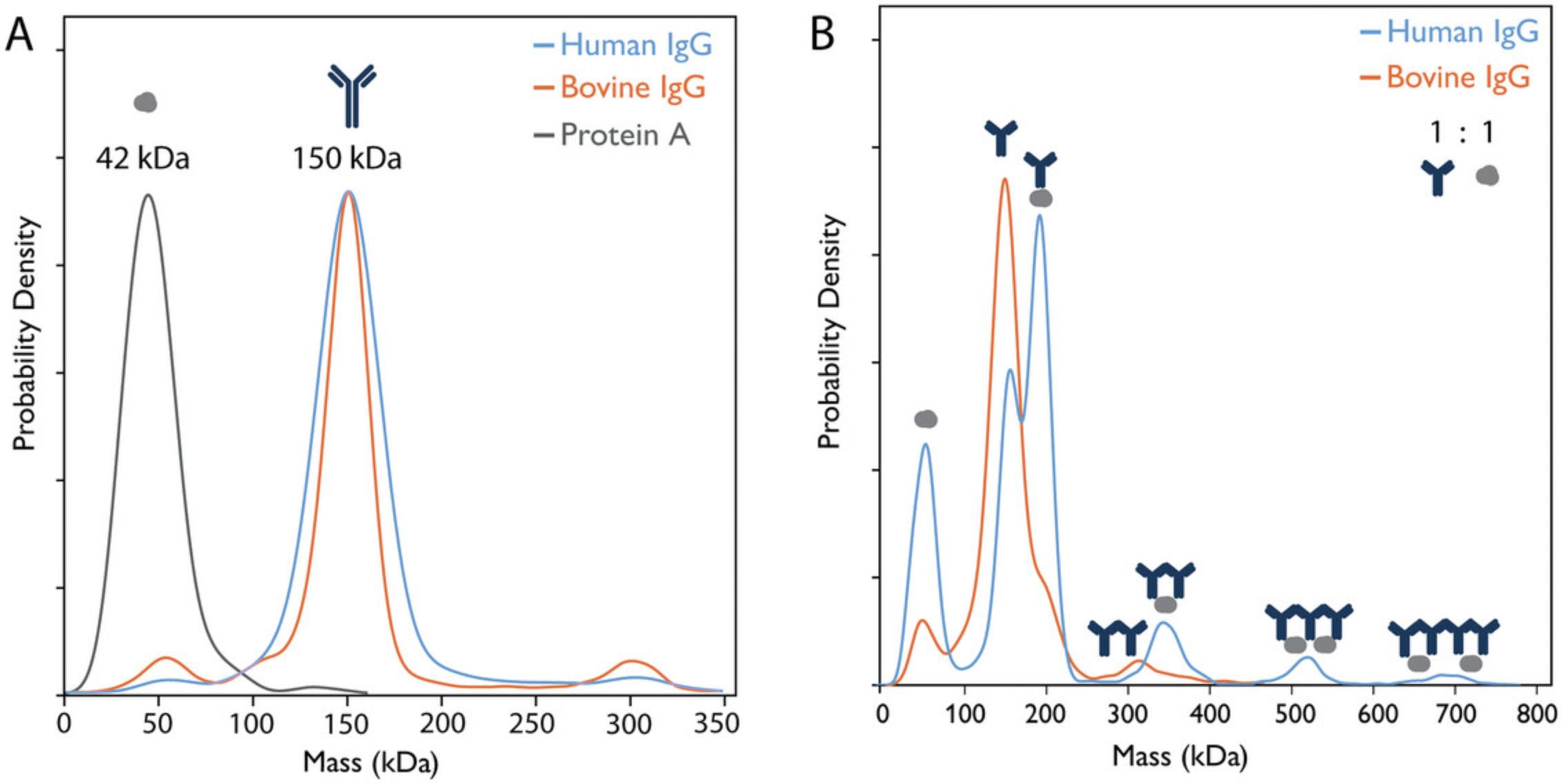
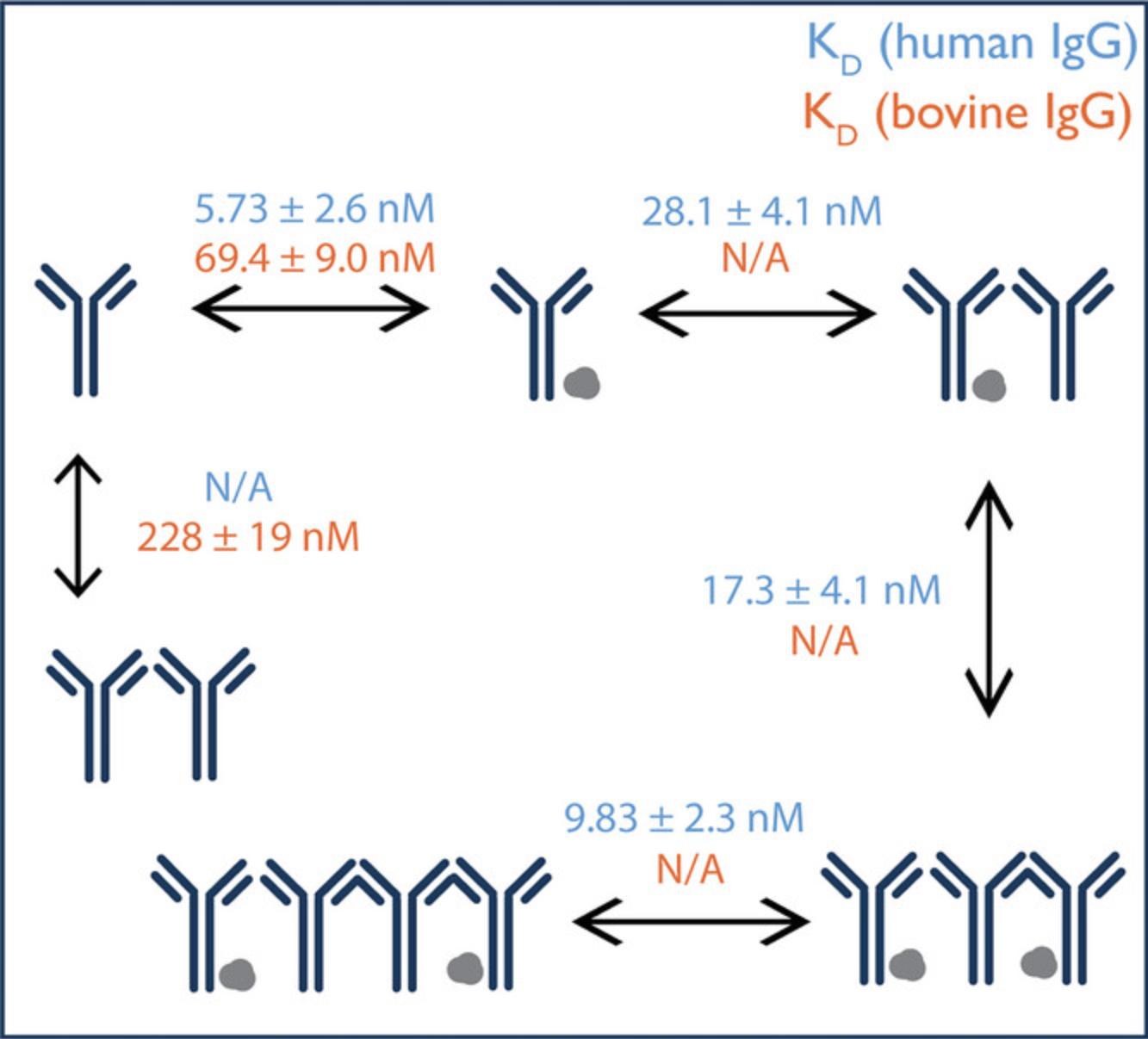
Materials
-
Immersion oil (Zeiss Immersol 518F, cat. no. 444960-0000-000)
-
β-amylase, from sweet potato (Sigma-Aldrich, cat. no. A8781-1VL)
-
Phosphate-buffered saline (PBS), 1× solution (VWR Life Science, cat. no. K812-500ML)
-
Protein A, from Staphylococcus aureus (Sigma-Aldrich, cat. no. P6031-.5MG)
-
IgG from bovine serum (Sigma-Aldrich, cat. no. I5506-10MG)
-
IgG from human serum (Sigma-Aldrich, cat. no. I8640-10MG)
-
Isopropanol
-
TwoMP mass photometer (Refeyn)
-
AcquireMP v2022 R1 software
-
Sample well cassettes, alignment tool, and sample carrier slides (MP Starter Kit for TwoMP and SamuxMP, Refeyn, cat. no. MP-CON-21001)
-
Tweezers
-
Eppendorf tubes (Merck, cat. no. EP0030120086)
-
Lens-cleaning tissues
-
DiscoverMP v2022 R1 software
NOTE : The steps listed below provide a complete picture of the procedure followed to collect the data presented. The procedure follows Refeyn's current recommendations, but it is recommended to consult the manufacturer's current guidance for any updates to the method.
NOTE : All measurements described are done in PBS, but experiments can be performed in any buffer chosen by the user.
Start the system
1.Switch on the TwoMP mass photometer and leave it on for ≥1 hr before starting to measure the samples.
2.Launch the AcquireMP v2022 R1 software (AMP, blue icon) and set the name of the project and the location where the data will be saved.
3.Turn on the antivibration table by switching on the power and press the isolation button (under the mass photometry instrument).
Assemble cassette and clean slide
4.Using tweezers, place a sample well cassette in the middle groove of the alignment tool (Fig. 3A).
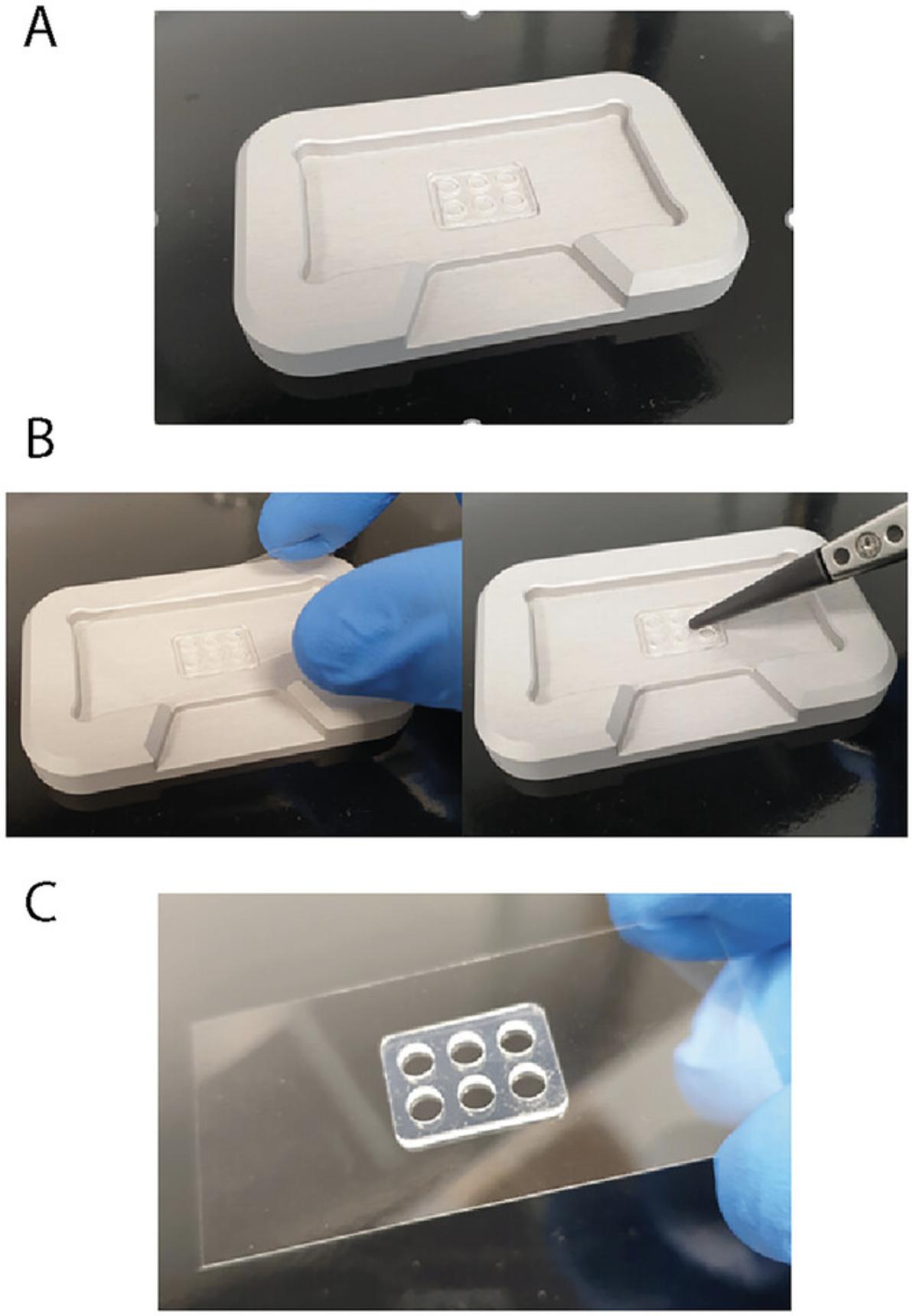
5.Place a clean sample carrier slide on top of the cassette. Press gently with the tweezers to ensure firm attachment (Fig. 3B).
6.Put a drop of immersion oil on the mass photometer objective.
7.Carefully transfer the slide with the attached cassette (Fig. 3C) to the mass photometer stage.
8.Place the magnets in the corners of the slide to hold it firmly to the stage.
9.Position the middle of the first well above the objective using the AMP software controls.
Prepare samples, buffer, and calibrant
10.In an Eppendorf tube, prepare 20 µl β-amylase at 40 nM concentration in PBS (Calibrant).
11.In a second tube, prepare 20 µl protein A at 40 nM concentration in PBS (Control A).
12.In a third tube, prepare 20 µl IgG from bovine serum at 40 nM concentration in PBS (Control B).
13.In a fourth tube, prepare 20 µl IgG from human serum at 40 nM concentration in PBS (Control C).
14.In a fifth tube, mix human IgG (80 nM) and protein A (80 nM) at a 1:1 ratio, for a final volume of 30 μl, so that each component has a concentration of 40 nM before interaction (Sample mix A).
15.In a sixth tube, mix bovine IgG (80 nM) and protein A (80 nM) at a 1:1 ratio, for a final volume of 30 μl, so that each component has a concentration of 40 nM before interaction (Sample mix B).
16.In a seventh tube, aliquot 60 µl PBS.
17.Incubate all the tubes at room temperature for ≥15 min.
Measure calibrant
18.Add 10 µl PBS to the cassette well above the objective and then use the software to find focus.
19.After focusing, ensure the area of focus is clean and free of big dust particles and that the native images show the typical glass roughness pattern (Fig. 4A).
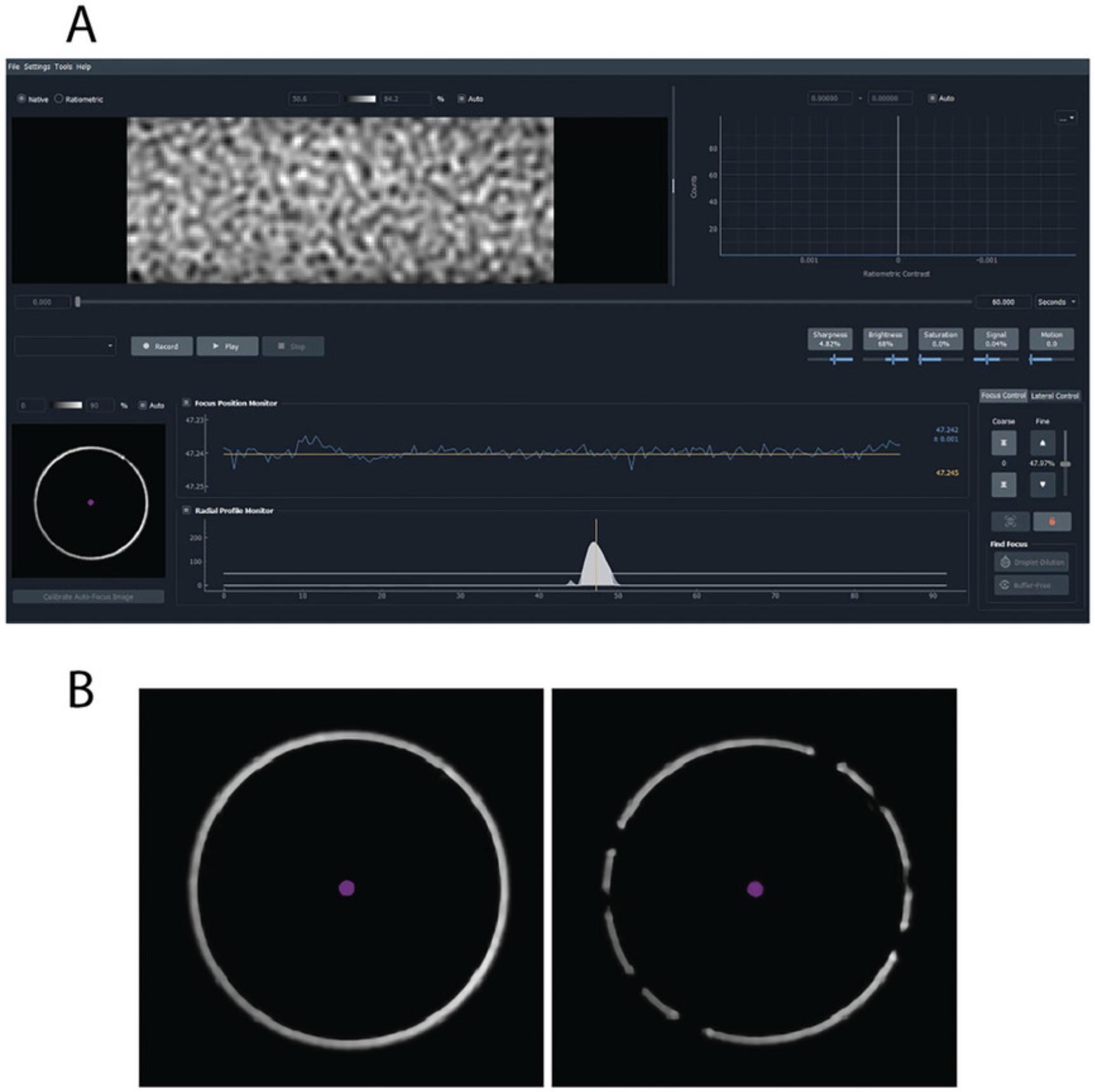
20.Ensure that the white focus ring in the bottom left corner of the AMP screen is clear and unbroken (Fig. 4B).
21.Check the data quality monitors (referring to Sharpness, Brightness, Saturation, Signal, and Motion) to ensure the parameters are all at acceptable levels.
22.Press “Record” to take a 60-s measurement of the buffer with no sample added.
23.Find focus again, add 10 µl Calibrant (see step 10) to the buffer, and then pipet up and down three times to mix.
24.Close the lid. Press “Record” to acquire data.
25.When the measurement finishes, save the data when prompted.
Measure controls
26.Move to a new well using the AMP software “Lateral Control” menu.
27.Repeat steps 18 to 22 to find focus (recording a buffer measurement is optional at this stage).
28.Repeat steps 23 to 25, but instead of the Calibrant, perform the measurements with protein A (Control A, step 11) (Fig. 1A).
29.Repeat this process for bovine and human IgG (Controls B and C, steps 12 and 13), using one sample at a time until all samples have been measured (Fig. 1A).
Measure Sample mixes A and B
30.Repeat steps 26 to 28 for Sample mix A (see step 14) and then Sample mix B (see step 15). Acquire three independent measurements for each sample mix.
Change slides in between measurements
31.When all the wells in the gasket are used, discard the glass slide.
32.Using a lens-cleaning tissue, wipe away the old oil from the objective. Using a second lens-cleaning tissue wet with isopropanol, wipe away the oil residue from the objective with gentle circular motions. Repeat with new tissue if necessary.
33.Using a new clean slide, repeat the “Measure calibrant” section (steps 18 to 25) before proceeding to any other measurements.
Data analysis and visualization in DiscoverMP
34.Launch the DiscoverMP v2022 R1 software (DMP, orange icon).
35.Click on the plus icon at the top left and select all .mp files.
36.Ensure that the software starts to analyze the loaded files automatically.
37.Delete the automatic Gaussians if they do not fit the data well (for example, if two adjacent peaks have been combined into a single skewed peak). In that case, fit the Gaussians manually by double-clicking near the center of the desired peak.
38.Once all movies have been analyzed, select the calibration movie and press the “Create mass calibration” button (bottom right).
39.Add the MW values to the respective ratiometric values loaded automatically in the window open (for β-amylase, these are 56, 112, and 224 kDa). Press “Save.”
40.To apply the mass calibration to a single measurement, select the .mp sample file (e.g., Sample mix A) and double-click on the .mc calibration file in the bottom left. To apply the same calibration to all files in the workspace, left click on the .mc calibration file and select “apply to all.” Once a calibration is loaded, the data will be displayed as a mass histogram, rather than the default ratiometric-contrast histogram.
41.Adjust the histogram if needed using the “Plot configuration options” under the histogram in the analysis menu.
42.To plot multiple measurements together and/or export histograms, use the “Figures” tab, press “+ Add Figure,” and select “Stacked Histograms.” Next, drag the measurements to be plotted across to the middle panel. Modify the plot further, if needed, in the “Figures” tab before exporting figures.
43.Save data analysis as a workspace, which will create a .dmp file.
44.Examine the data from the control measurements. Assess the purity and quality of the control samples, ensuring that any aggregation or protein oligomerization is identified and considered when interpreting results (Fig. 1A).
45.Examine the data from the sample mix measurements. Identify differences in complex formation between protein A and the IgG antibodies of differing origin (Sample mixes A and B) (Fig. 1B; Table 1; Supporting Information, Data 1). Make sure to analyze the data of the repeats, as differences in the interaction profile of the repeats can be an indication that the interaction is not at equilibrium, which will affect the KD measured (see Troubleshooting section).
Human IgG (n=4) | ||
Species | Expected mass (kDa) | Observed mass (kDa), µ ± σ |
Protein A | 42 | 44 ± 1.0 |
IgG | 150 | 153 ± 1.5 |
IgG–protein A | 192 | 192 ± 1.0 |
IgG2–protein A | 342 | 352 ± 1.0 |
IgG3–protein A2 | 534 | 536 ± 4.8 |
IgG4–protein A2 | 684 | 700 ± 5.3 |
Bovine IgG (n=3) | ||
Species | Expected mass (kDa) | Observed mass (kDa), µ ± σ |
Protein A | 42 | 51 ± 2.7 |
IgG | 150 | 147 ± 1.0 |
IgG–protein A | 192 | 187 ± 1.2 |
IgG2 | 300 | 304 ± 3.2 |
-
aUncertainty is estimated by taking the standard deviation (σ) of the central mass from n independent measurements.
KD calculation
46.Find all species that contain the IgG and note the number of IgG monomers within each species.
47.Using the known concentration of IgG, calculate the counts-to-concentration conversion factor:
where [IgG]tot is the known total concentration of IgG in the sample droplet that was measured, Countsspecies is the counts of a particular species from a Gaussian fit to a histogram, and αspecies is an integer that accounts for the number of IgG monomers within each species (i.e., it would be 0 if a complex contained no IgG, 1 for an IgG monomer or IgG–protein A complex, and 2 for an IgG dimer or IgG2–protein A complex).
48.From the conversion factor, calculate the concentration for all species except protein A:
where again Countsspecies is obtained from a Gaussian fit.
49.Due to the variable noise contributions and protein A being close to the detection limit of the instrument (Soltermann et al., 2020), calculate the equilibrium concentration of protein A using mass balance with the formula
where [A]tot is the total concentration of protein A added to the droplet, βspecies is an integer that accounts for the number of protein A monomers in a complex, and the sum is made over all species except unbound protein A.
50.Once the concentration has been calculated for each species, determine the KD for each complex using the following standard equation:
where it is assumed equilibrium is reached as
51.To calculate the error for the KD measurement, take the standard deviation of calculated KD values from repeated measurements (as was done for the values given in Fig. 2).
COMMENTARY
Background Information
Determining the affinity of protein-protein interactions can give mechanistic insights into many biological processes (Soltermann et al., 2020). Standard techniques for measuring binding affinities often involve protein labeling and immobilization, which can perturb the interactions under observation and therefore their calculated values, which may not represent in vivo binding. These methods are often time consuming and require large amounts of sample and consumables as well as extensive instrument or acquisition optimization (Wu & Piszczek, 2020).
Mass photometry is a single-molecule bioanalytical technique that can quantify the affinity of protein interactions in solution, without labels, and so represents an attractive alternative approach. Mass photometry measures the light scattered by individual proteins and other biomolecules, using this signal to count the molecules and determine their mass, with the aid of a mass calibration step (Young et al., 2018). It provides the mass distribution of biomolecules in a sample, revealing the binding partners present, the complexes formed, and the relative abundance of each species. From this information, the affinity of the interactions can be readily calculated, as shown using the Basic Protocol described here as well as in previous studies (Soltermann et al., 2020; Wu & Piszczek, 2021). The technique offers further practical advantages in that a typical mass photometry experiment requires little sample (∼10 μl) at a low concentration (∼20 nM) and takes <5 min from data acquisition to data analysis.
The data from a mass photometry measurement can be used to calculate KD values for any interactions where both bound and unbound species are observable at the concentrations used (100 pM to 100 nM). For this reason, affinities outside of this range cannot be reliably determined by mass photometry. Weaker interactions, for example, are challenging as these complexes are liable to dissociate at such low concentrations. However, a microfluidics-based approach has recently been developed that enables the measurement of weak interactions by mass photometry (Claasen et al., 2023). Mass photometry allows detection of biomolecules with MWs ranging from 30 kDa to 5 MDa, and the equations presented are valid for species detected within this mass range (for the TwoMP mass photometer; Refeyn Ltd., 2022). For the KD determination, it is also important that the populations measured are resolved with distinct mass (Refeyn Ltd., 2021).
The simple protocol presented here describes the use of mass photometry to study two related protein-antibody interactions possessing differing affinities. It conveys how the contrast-mass calibration is performed for proteins, how to collect data, and how to determine the mass distributions of the binding species and complexes. The Basic Protocol goes on to explain how, once a user has determined the relative abundance of the binding partners and the multiple complexes they form in solution, to calculate the KD values for each interaction.
The protocol is simple and quick and can be performed by users who are new to mass photometry. Furthermore, it can provide valuable information that is difficult if not impossible to obtain with other ensemble-based methods. With this protocol, mass photometry can be used to characterize and quantify complex protein interactions.
Critical Parameters
Experimental error
The measurement error for a mass photometry measurement (using the TwoMP mass photometer) is ±5%, meaning that the mass determined in a single measurement can deviate from the expected mass by that percentage (Refeyn Ltd., 2022). Taking repeated measurements can reduce this error, with the expected deviation of MW between repeats being <2%. A common factor contributing to the discrepancy between the measured and the expected MWs is the existence of post-translational modifications, which can significantly alter the mass of the proteins (e.g., heavily glycosylated proteins). Because mass photometry measures mass, the contribution of such modifications will contribute to the MW measured, although it will not be included in any mass calculations based on the amino acid sequence. Further sources of experimental error include human errors in the sample preparation or pipetting.
Concentration range
Mass photometry is a single-particle method based on interference signals created by particles as they land on a glass surface. Accordingly, it is important to prepare samples at the appropriate concentration. For the TwoMP mass photometer, the optimal sample concentration ranges from 100 pM to 100 nM (Refeyn Ltd., 2021). If the landing event density is too low, there are not enough species (i.e., protein landing events) to provide an accurate statistical analysis of the populations. These events are still detected and will be displayed in the acquisition software data, but the data points will be insufficient for meaningful analysis. Conversely, if the concentration is too high, the landing molecules will spatially overlap, resulting in poor data quality. In such cases, the mass photometry histograms would have broader distributions (higher sigma values), indicating worse resolution (Fig. 5).
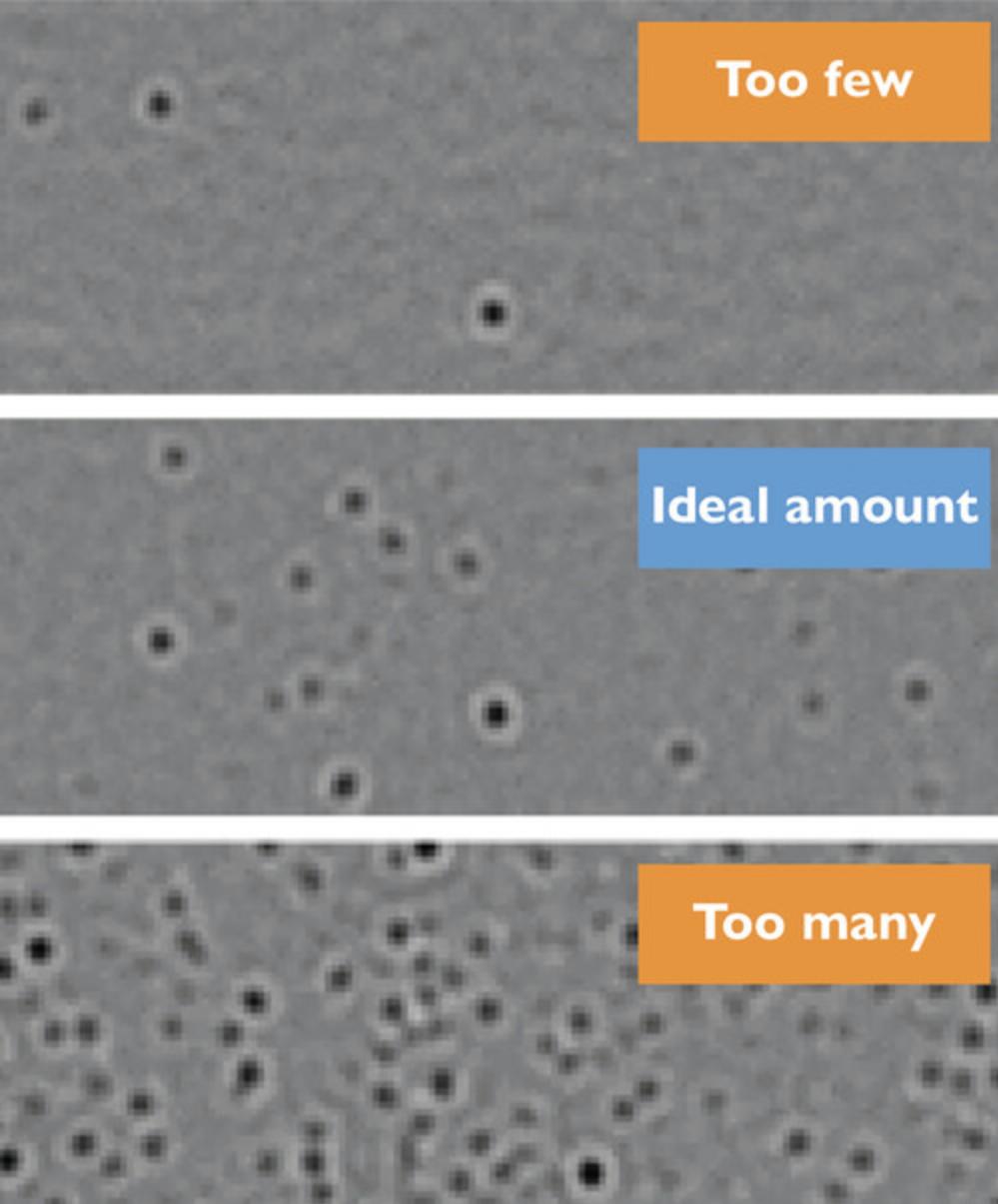
Buffer, impurities, and background noise
In the Basic Protocol, PBS is utilized for all mass photometry measurements. It is worth noting that there is a wide range of biological buffers that are compatible with mass photometry. Importantly, the calibration measurement should be performed using the same buffer as the subsequent measurements.
Impurities in the buffer or protein samples can detrimentally affect the measurement. To minimize this, it is recommended to use buffers filtrated through 0.2-µm filters and to perform a calibration measurement with the same buffer as the buffer used to run the samples, where possible.
Additionally, certain components, like detergents, can introduce background noise. In aqueous solution, detergents tend to form micelles, which scatter light, affecting the measurement of proteins. By measuring the buffer alone, users can detect the presence of those micelles and their contribution to the measurement. When measuring buffers with detergent, during the measurement, the signal monitor will turn orange, and larger micelles can be visualized directly in the AcquireMP software (ratiometric view).
Troubleshooting
See Table 2 for a summary of the most common sources of errors that might impact the data quality and how to solve them. It is recommended to consult the manufacturer's latest guidance for any updates.
Problem | Possible cause | Solution |
---|---|---|
Signal reported by the AcquireMP software is in the orange range when measuring the buffer only | Sample carrier slides are not clean | Always use clean sample carrier slides following the manufacturer's recommendations |
Buffer contains particles (e.g., dust, large undissolved particles, or detergent) | Prepare fresh buffer, make sure all components are well dissolved, and filter the buffer to 0.2 µm | |
Fewer events detected than expected for a sample at an acceptable concentration | Sample is not mixed properly | After adding the sample to the buffer, mix well by pipetting up and down 3 to 4 times. Use a pipet with a larger volume if needed. |
Particles may form higher-order assemblies, reducing the effective concentration and number of particles in solution | Increase the sample concentration or add more sample to the glass coverslip | |
Sample is aggregated, which reduces the effective concentration and number of particles in solution | Use a fresh sample preparation | |
Optimize buffer conditions to decrease aggregation propensity (e.g., pH, salt, additives). | ||
Particles could have been depleted from the stock solution due to adhesion to the Eppendorf tube | Try to bring the protein into solution again by mixing with the pipet. If this does not help, prepare a fresh pre-dilution of the sample. Use of low-protein-binding tubes is generally recommended for protein samples; however, their performance may vary based on the system under study. | |
Particles appear already depleted from the solution before starting the recording | Follow the recommended loading procedure: first, load the buffer and find focus, and then immediately add the sample to the buffer droplet, mix well, close the lid, and start the recording | |
Particles are not adhering to the bare glass on the measurement surface | Consider coating the glass surface to counteract charge effects. This issue tends to occur more frequently with nucleic acids than with proteins. An example of such coating is APTES (Li et al., 2020), but you may also explore other coating options. | |
Measured particles do not show the expected mass | Problem with the calibration and/or sample focus | Repeat the calibration measurement and double-check its accuracy with proteins of known MW, e.g., bovine serum albumin. Ensure that the sample is in focus. Repeat the measurement of your sample in triplicate. |
Calibration was performed under different conditions | Ensure consistent buffer composition, temperature, and size of the field of view (FOV), which impact the ratiometric contrast | |
Ensure that samples, calibrants, and buffers are all at room temperature | ||
It is recommended to do calibrations at the beginning and at the end of the experiments as well as when sample/buffer conditions change | ||
It is not recommended to use a calibration from a different day. Make sure buffer composition matches between the calibration standard and sample. | ||
Sample and calibration need to be measured in the same FOV | ||
Calibration was performed with particles of a different refractive index | Different biomolecules (e.g., proteins, nucleic acids) possess different refractive indices, which affects the ratiometric contrast. Use the same type of biomolecules for calibration and sampling. | |
Sample is not as expected | If the mass continuously differs from what is expected, there may be a problem with the sample. For example, the interaction might not be in equilibrium condition, or the sample might be more heterogenous than expected due to the presence of impurities or post-translational modifications such as glycosylation. | |
High level of uncertainty in data due to poorly resolved peaks or high level of background noise | Reconsider whether data interpretation has been appropriate. Control measurements, including buffer measurements and interaction partners measured alone, will give insights about what to expect. | |
Samples are not in equilibrium, and the complexes are still assembling or disassembling. The broadening of the peaks might be due the presence of transient species. | ||
Cannot measure consistent KD values from mass photometry data | Sample is not at equilibrium | Ensure that the sample has equilibrated for sufficient time (and at room temperature) before starting experiments |
Species are adsorbing to tubes | If species are adsorbing to containers to a specific extent, the concentrations added in experiments will not be consistent, and hence, KD values will appear to change. To mitigate this, low-binding tubes can be used, and the amount of time that samples are left in tubes between repeats should be minimized. However, performance may vary based on the system under study. |
Understanding Results
Interpreting mass photometry data involves examining the histograms produced in mass photometry measurements. During a measurement, the biomolecules and complexes are diffusing in the sample droplet. They randomly land on the measurement surface (the sample carrier slide), which results in the interferometric scattering signal that the mass photometer detects. During a typical mass photometry measurement (lasting ∼1 min), hundreds to thousands of landing events can be detected. Histograms are a helpful way to visualize that data.
The contrast signal must be converted to mass via a mass calibration step using a biomolecule of known MW. The measured contrast value is negative when it corresponds to an event where a particle landed on the measurement surface and positive when a particle moved away from the surface.
In mass photometry histograms (Supporting Information, Figure 1), the data from the many single-particle events are grouped into narrow mass ranges (“binned”) to make the data easier to interpret. Each bin is represented by a vertical bar, and the height of the bar (the “counts”) tells you how many particles fell into that particular range. If a bar is tall, it means that the mass photometer counted many events (corresponding to many molecules or complexes) in that mass range.
As for most biological data, repeated measurements will produce data with some variability that is centered on the true value, often following a normal or Gaussian probability distribution. In a mass photometry histogram, this means that the data appear as peaks made up of several bars. Each peak can be fit by a Gaussian curve, and this fitting is implemented in the DiscoverMP software. From the Gaussian fitting, the peak's mean gives the central mass value of a species, whereas the peak's standard deviation (σ) measures the peak's variability or spread.
Mass photometry histograms often have multiple peaks, indicating the presence of multiple species in the sample. When multiple peaks are present (as in the experiment described here), a third key parameter is obtained from the mass histograms: the relative abundance of each species. Integrating the number of counts under each peak provides a relative measure of the abundance of the corresponding species. As described in the Basic Protocol, these relative measures can be converted to concentration values and used to calculate the affinity constants for the complexes observed (Supporting Information, Data 1).
Another thing to note is that in mass photometry experiments, we observe both binding and unbinding events between molecules of interest. Ideally, all proteins involved in these interactions should exhibit similar binding and unbinding ratios. When the interaction complex demonstrates a higher binding-to-unbinding ratio than its individual components, it is likely that the concentration of the interaction species will gradually decrease over time in the solution. Meanwhile, the individual components, which tend to unbind more frequently, will be maintained at relatively higher concentrations. This dynamic can have an impact on the measured KD, which may appear lower than the actual KD due to the reduced concentration of the interaction species. Conversely, if the interaction complex has a lower binding-to-unbinding ratio, the KD measured in mass photometry is likely to be higher. In such cases, the interaction species is more prone to unbinding from the surface, leading to a relatively higher concentration of this species over time. It is important to note that at this stage, there is no straightforward mathematical or software-based correction for this effect in mass photometry, and addressing this challenge may require further investigation.
Time Considerations
The Basic Protocol can be completed within 2 hr. The mass photometer needs to be switched on 1 hr prior to measurements. Preparing the samples and equilibrating them at room temperature can be achieved in 30 min, although the time required for the interactions to reach equilibrium is sample dependent. Each sample and calibrant measurement can be completed within 5 min. Primary data analysis in the DiscoverMP software and calculation of KD values can be also performed within 30 min.
Acknowledgments
W.B.S. is supported by a UKRI Future Leaders Fellowship [MR/V02213X/1]. The article text and graphics were prepared with support from members of Refeyn's scientific communications team (Panagiota Paganopoulou, Neus Torres Tamarit, and Catherine Lichten). The authors also acknowledge valuable feedback from Matthias Langhorst.
Author Contributions
Zornitsa Kofinova : Formal analysis; investigation; validation; writing—original draft; writing—review and editing. Gogulan Karunanithy : Data curation; formal analysis; software; validation; writing—original draft; writing—review and editing. Ana Sofia Ferreira : Conceptualization; data curation; project administration; supervision; writing—original draft; writing—review and editing. Weston B. Struwe : Conceptualization; methodology; writing—original draft; writing—review and editing.
Conflict of Interest
Z.K., G.K., and A.S.F. are employees of the company Refeyn Ltd., which produces the mass photometer used in the Basic Protocol. W.B.S. is a shareholder in and consultant for Refeyn Ltd.
Open Research
Data Availability Statement
The data necessary to repeat the KD calculations are available in the Supporting Information of this article. The complete raw data are available (as .mp files, which require DiscoverMP software to open) from the corresponding authors upon reasonable request.
Supporting Information
Filename | Description |
---|---|
cpz1962-sup-0001-SuppMat.xlsx23.4 KB | Figure 1. Mass photometry mass-to-counts histogram exported directly from the DiscoverMP software. This histogram illustrates a single mixed measurement of bovine IgG and protein A along with the complexes they form in solution. Gaussian fitting was conducted manually. Using this histogram, users can access information regarding the resultant populations, including mass, standard deviation (σ), skewness, and counts. |
cpz1962-sup-0002-SuppMat.pdf109.3 KB | Data 1. Mass and counts of IgG–protein A complexes. |
cpz1962-sup-0003-FigureS1.png105.7 KB | Data 2. KD measurements. |
Please note: The publisher is not responsible for the content or functionality of any supporting information supplied by the authors. Any queries (other than missing content) should be directed to the corresponding author for the article.
Literature Cited
- Atri, M. S., Saboury, A. A., & Ahmad, F. (2015). Biological applications of isothermal titration calorimetry. Physical Chemistry Research , 3(4), 319–330. https://doi.org/10.22036/pcr.2015.11066
- Claasen, M., Kofinova, Z., Contino, M., & Struwe, W. B. (2023). Analysis of protein complex formation at micromolar concentrations by coupling microfluidics with mass photometry. JoVE (Journal of Visualized Experiments), in press.
- Hage, D. S., & Cazes, J. (2005). Affinity chromatography in antibody and antigen purification. In Handbook of affinity chromatography ( 2nd ed.). Taylor & Francis. https://doi.org/10.1201/9780824751982
- Drikic, M., Olsen, S., & De Buck, J. (2019). Detecting total immunoglobulins in diverse animal species with a novel split enzymatic assay. BMC Veterinary Research , 15(1), 374. https://doi.org/10.1186/s12917-019-2126-z
- Dubrow, A., Zuniga, B., Topo, E., & Cho, J.-H. (2022). Suppressing nonspecific binding in biolayer interferometry experiments for weak ligand–analyte Interactions. ACS Omega , 7(11), 9206–9211. https://doi.org/10.1021/acsomega.1c05659
- Helmerhorst, E., Chandler, D. J., Nussio, M., & Mamotte, C. D. (2012). Real-time and label-free bio-sensing of molecular interactions by surface plasmon resonance: A laboratory medicine perspective. The Clinical Biochemist Reviews , 33(4), 161–173.
- Jerabek-Willemsen, M., André, T., Wanner, R., Roth, H. M., Duhr, S., Baaske, P., & Breitsprecher, D. (2014). MicroScale Thermophoresis: Interaction analysis and beyond. Journal of Molecular Structure , 1077, 101–113. https://doi.org/10.1016/j.molstruc.2014.03.009
- Khalili, H., Brocchini, S., Khaw, P. T., & Filippov, S. K. (2018). Comparative thermodynamic analysis in solution of a next generation antibody mimetic to VEGF. RSC Advances , 8(62), 35787–35793. https://doi.org/10.1039/C8RA07059H
- Li, Y., Struwe, W. B., & Kukura, P. (2020). Single molecule mass photometry of nucleic acids. Nucleic Acids Research , 48(17), e97. https://doi.org/10.1093/nar/gkaa632
- Noy-Porat, T., Alcalay, R., Mechaly, A., Peretz, E., Makdasi, E., Rosenfeld, R., & Mazor, O. (2021). Characterization of antibody-antigen interactions using biolayer interferometry. STAR Protocols , 2(4), 100836. https://doi.org/10.1016/j.xpro.2021.100836
- Refeyn Ltd. (2021). Mass range, resolution and accuracy: Understanding the strengths and boundaries of mass photometry. https://www.refeyn.com/post/benefits-and-limitations-of-mass-photometry
- Refeyn Ltd. (2022). Quantifying protein binding affinities using mass photometry. https://www.refeyn.com/mass-photometry-application-notes
- Refeyn Ltd. (2022). TwoMP: Transforming biomolecular characterisation. https://www.refeyn.com/twomp-mass-photometer
- Soltermann, F., Foley, E. D. B., Pagnoni, V., Galpin, M., Benesch, J. L. P., Kukura, P., & Struwe, W. B. (2020). Quantifying protein–protein interactions by molecular counting with mass photometry. Angewandte Chemie International Edition , 59(27), 10774–10779. https://doi.org/10.1002/anie.202001578
- Wienken, C. J., Baaske, P., Rothbauer, U., Braun, D., & Duhr, S. (2010). Protein-binding assays in biological liquids using microscale thermophoresis. Nature Communications , 1(1), 1. https://doi.org/10.1038/ncomms1093
- Wu, D., & Piszczek, G. (2020). Measuring the affinity of protein-protein interactions on a single-molecule level by mass photometry. Analytical Biochemistry , 592, 113575. https://doi.org/10.1016/j.ab.2020.113575
- Wu, D., & Piszczek, G. (2021). Rapid determination of antibody-antigen affinity by mass photometry. JoVE (Journal of Visualized Experiments) , 15, https://doi.org/10.3791/61784
- Young, G., Hundt, N., Cole, D., Fineberg, A., Andrecka, J., Tyler, A., Olerinyova, A., Ansari, A., Marklund, E. G., Collier, M. P., Chandler, S. A., Tkachenko, O., Allen, J., Crispin, M., Billington, N., Takagi, Y., Sellers, J. R., Eichmann, C., Selenko, P., … Kukura, P. (2018). Quantitative mass imaging of single biological macromolecules. Science , 360(6387), 423–427. https://doi.org/10.1126/science.aar5839
Citing Literature
Number of times cited according to CrossRef: 2
- Jiří Kratochvíl, Roi Asor, Seham Helmi, Weston B. Struwe, Philipp Kukura, Lifting the Concentration Limit of Mass Photometry by PEG Nanopatterning, Nano Letters, 10.1021/acs.nanolett.4c01667, 24 , 33, (10032-10039), (2024).
- Constantinos Chatzicharalampous, Herwig Schüler, A multidomain PARP14 construct suitable for bacterial expression, Protein Expression and Purification, 10.1016/j.pep.2024.106580, 224 , (106580), (2024).