Identifying Genetic Dependencies in Cancer by Analyzing siRNA Screens in Tumor Cell Line Panels
James Campbell, Colm J Ryan, Christopher J. Lord
Abstract
Loss-of-function screening using RNA interference or CRISPR approaches can be used to identify genes that specific tumor cell lines depend upon for survival. By integrating the results from screens in multiple cell lines with molecular profiling data, it is possible to associate the dependence upon specific genes with particular molecular features (e.g., the mutation of a cancer driver gene, or transcriptional or proteomic signature). Here, using a panel of kinome-wide siRNA screens in osteosarcoma cell lines as an example, we describe a computational protocol for analyzing loss-of-function screens to identify genetic dependencies associated with particular molecular features. We describe the steps required to process the siRNA screen data, integrate the results with genotypic information to identify genetic dependencies, and finally the integration of protein-protein interaction data to interpret these dependencies.
Before start
Introduction
Recent large-scale sequencing projects and decades of small-scale studies have led to the identification of hundreds of “driver” genes in cancer—genes whose alteration through genetic or epigenetic means provides a growth or survival advantage for tumor cells [1, 2]. A key remaining challenge is to understand how these driver mutations alter cellular states to promote tumor progression and how this altered state may be exploited for the development of targeted therapeutics [3]. Identifying the set of genes that are required for growth in a given tumor cell line provides both an insight into the cellular state and suggests genes whose products may be targeted therapeutically. Toward this end, a number of laboratories have used loss-of-function screening to generate resources describing the genetic requirements of panels of tumor cell lines [4-11]. The majority of these resources use either genome-scale shRNA screens carried out in a pooled format [6, 7, 10] or siRNA screens carried out in an arrayed format [4, 5, 11] to identify genetic dependencies. In the near future CRISPR-based approaches will likely be used for similar purposes, although to date the number of cell lines profiled by genome-wide CRISPR libraries remains small (e.g., five cell lines in [8]). Regardless of the experimental methodology used, the goal of loss-of-function screens is largely the same—the identification of genes required for growth in specific cancer cell lines. By integrating the results of these screens with genotypic data, it is possible to identify genes that appear specifically required for growth in the presence of a particular driver gene mutation. In some cases the driver gene mutation results in an increased dependency upon the gene itself, a phenomenon known as “oncogene addiction” [12]. Examples of this include an increased sensitivity of ERBB2 -amplified breast cancer cell lines to siRNA reagents targeting ERBB2 [4], and an increased sensitivity of KRAS mutant cell lines to shRNA reagents targeting KRAS [7]. More frequent are instances where the driver gene and the resulting dependency gene are different, often termed non-oncogene addictions or synthetic lethalities [12, 13]. Examples of non-oncogene addictions identified from loss-of-function screens include a dependence of ARID1A mutant cell lines upon the ARID1A paralog ARID1B [14], an increased sensitivity of PTEN mutant breast cancer cell lines to inhibition of the mitotic kinase TTK [4], and an increased sensitivity of MYC amplified breast cancer cell lines to inhibition of multiple spliceosome component coding genes [15]. Ultimately both oncogene addictions and synthetic lethalities identified in these screens may be exploited for the development of novel targeted therapeutics in cancer [13].
When these screens are analyzed, statistical approaches are used to identify significant associations between the mutation of a driver gene and an increased sensitivity to the inhibition of another gene. The interpretation of the resulting associations remains challenging—the statistical tests provide information on which genes are required in the presence of specific driver genes, but not the mechanistic explanation as to why these dependencies exist. Inspired by approaches initially developed for the interpretation of genetic interactions in yeast [16], we have recently used the integration of functional interaction networks to aid the interpretation of dependencies identified in loss-of-function screens in cancer cell lines [5]. For instance in ERBB2 -amplified cell lines we see an increased dependency upon ERBB2 itself and also the ERBB2 protein-interaction partners ERBB3 and PIK3CA [5]. This suggests that ERBB2 amplified cell lines are frequently “addicted” to the functionality of ERBB2 , the binding of ERBB2 to its interaction partner ERBB3 , and the function of the downstream effector PIK3CA.
Here, we describe a protocol for the analysis of loss-of-function screens in a panel of cancer cell lines. We use as example data a recent kinome-wide siRNA screen performed in a panel of osteosarcoma cell lines [5]. Our analysis protocol involves three main steps:
1.The conversion of siRNA screening results into gene-sensitivity scores.
2.The integration of these sensitivity scores with genotypic data to identify statistical associations between driver
genes and sensitivity to the inhibition of particular genes.
3.The integration of additional data such as protein-protein interactions to interpret these associations.
Only the first step is specific to arrayed siRNA screens—we have successfully applied the latter analysis scripts to data resulting from additional screen types (e.g., pooled shRNA screens) (Fig. 1).
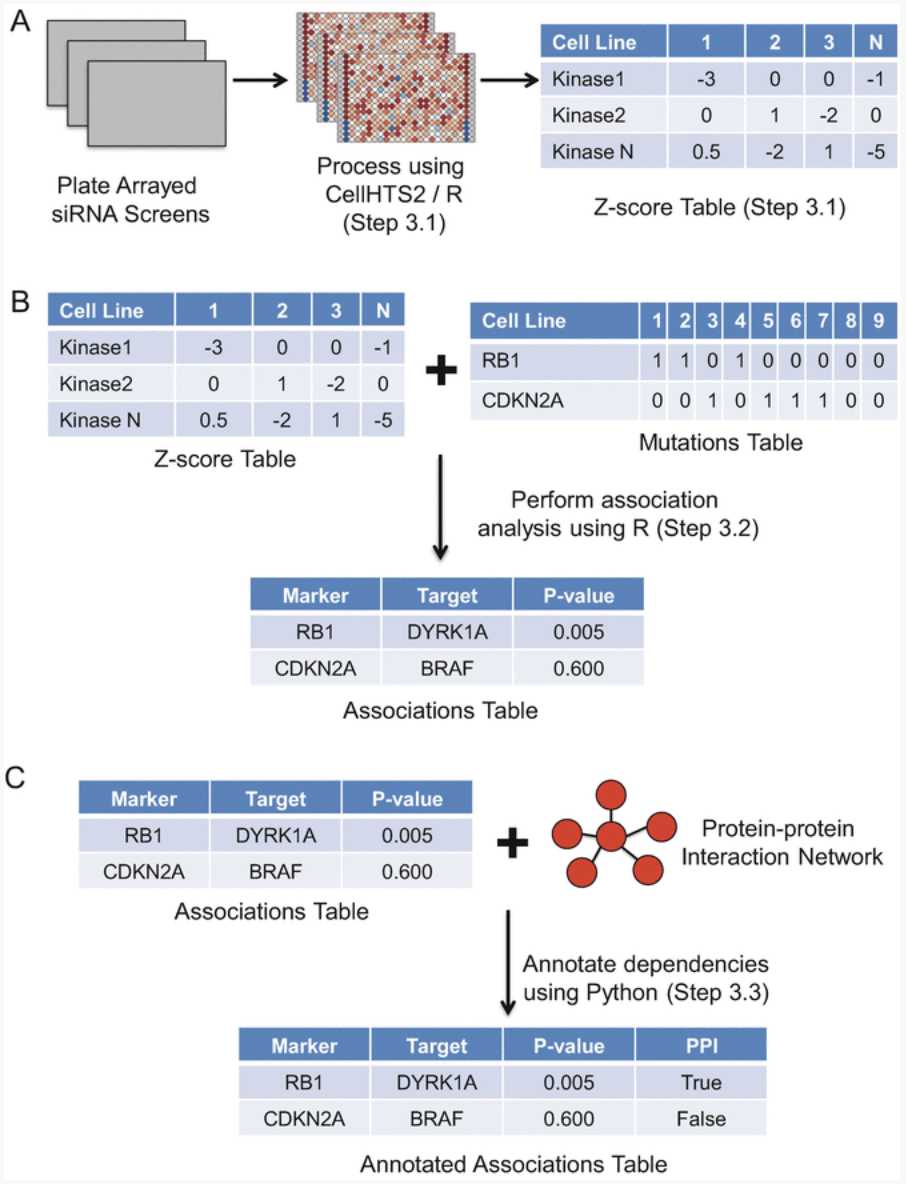
Attachments
Steps
3.1 Processing siRNA Screen Data Using CellHTS2
Typically, siRNA screens are conducted in multiwell tissue culture plates. The process of transfecting a cancer cell line with siRNAs is optimized prior to screening and once optimal conditions have been selected (described in [17]), cells are dispensed into multiwell plates containing growth media, transfection reagents, and siRNAs. The data in the example provided represent a screen of a single osteosarcoma tumor cell line using an siRNA library targeting 714 kinase and kinase-related genes. Positive and negative controls are included on each plate—typically non-targeting siRNA as a negative control and an siRNA pool targeting PLK1 as a positive control. The full experimental protocol for this screen has been described elsewhere [4, 5]. Briefly, following siRNA transfection, the cells were cultured for 5 days, after which a luminescence assay measuring cellular ATP was used to estimate cell viability. A Victor X5 platereader was used to read luminescence values, resulting in data files in Microsoft Excel format. Prior to the analysis in R, these data files were converted to plain text plate files . Each plate file contains the luminescence reading from each well in one 96 or 384 multiwell plate. Where an siRNA library is larger than the plate format used in the screen, several plates are required for a single screen. Additionally, multiple replicate screens are typically conducted for a given cell line and siRNA library. The organization of plates into segments of an siRNA library and replicate screens is described in a plate list file . A plate list file contains the file names of the plate files, the replicate numbers, and plate numbers in a multiplate screen. Annotations indicating the genes targeted by siRNAs in the library across multiple plates as well as the positions of control wells are provided in separate plain text files. The analysis protocol set out below uses the cellHTS2 [18] R package developed by Huber and Boutros to combine data from the plate files, the plate list file, the plate configuration file, and the annotation file. The luminescence data are normalized to produce Z -scores by first log2transforming the values and subtracting the median log luminescence value on a plate-by-plate basis. The plate-centered data are then scaled to the median absolute deviation (MAD) value calculated across the entire siRNA library to produce Z -scores.
An R script named “run_cellHTS.R” in the R-scripts directory contains the following commands. The first command loads the cellHTS2 R package that provides the functions required for the analysis.
require(cellHTS2)
With cellHTS2 loaded, we then use the readPlateList() function to read the plate list file which in turn creates a cellHTS object containing the luminescence data from the plate files ( see Note 7 ).
x <- readPlateList(
filename=" platelist_p3r3.txt",
name="CGDsExample"
path="./"
)
We next use the configure() function to add information from the plate configuration file and (optionally) the screen log and description file to the cellHTS object. The plate configuration defines the locations of samples, controls and empty wells.
x <- configure(
x,
descripFile="screen_description.txt",
confFile="plateconf_384.txt",
logFile="Screenlog.txt",
path="./"
)
We use the annotate() function to define the genes targeted by siRNAs in each well of the plate. This information is located in the “kinome_library.txt” file.
x <- annotate(
x,
geneIDFile="kinome_library.txt",
path="./"
)
We now process the luminescence data in the cellHTS object to normalize data values across the plates in the screen. This is done by log2transforming the luminescence values and subtracting the median value within a plate from all the values of wells in that plate. The parameters passed to the normalizePlates() function are described in Note 8 . The original cellHTS object “x” is passed to the normalizePlates() function and the result is saved into a new cellHTS object called “xn.”
xn <- normalizePlates(
x,
scale="multiplicative",
log=TRUE,
method="median",
varianceAdjust = "none",
negControls="neg",
posControls="pos"
)
The normalized data stored in “xn” are then scaled by dividing each well’s value by the median absolute deviation (MAD) calculated from the normalized values across the whole siRNA library. Control wells are excluded from the estimation of the MAD. Scaling the plate median centered normalized data by the MAD produces the robust equivalent of Studentized values or Z -scores ( see Note 9 ).
xsc <- scoreReplicates(
xn,
method="zscore",
sign="+"
)
For later statistical analyses, it may be preferable to summarize the values of replicate wells targeting a specific gene as a median or some other summary statistic. This can be performed using the summarizeReplicates() function in cellHTS2.
xsc <- summarizeReplicates(
xsc,
summary="median"
)
CellHTS2 also provides a function called getTopTable() that writes a plain text file containing the well annotation data as well as the luminescence data at each stage of processing. Here, we write this information to a file called “TopTable.txt.”
summary_info <- getTopTable(
list(
"raw"=x,
"normalized"=xn,
"scored"=xsc
),
file="TopTable.txt"
)
An HTML formatted report can also be generated describing the screen and the processing steps applied to it using the commands below. This HTML report provides information on the positive and negative controls included, the distribution of the resulting scores, and details of the quality of the screen ( Z ′ scores, see below).
The contents of the HTML report can be modified using the setSettings() function. Here, we turn on the reproducibility and intensities reports (producing heatmap visualizations of well values) and set the range of heatmap colors for the screen summary scores report.
setSettings(
list(
plateList=list(
reproducibility=list(
include=TRUE,
map=TRUE
),
intensities=list(
include=TRUE,
map=TRUE)
),
screenSummary=list(
scores=list(
range=c(-20, 10),
map=TRUE
)
)
)
)
We then use the writeReport() function to generate the HTML report.
writeReport(
raw=x,
normalized=xn,
scored=xsc,
outdir=./report,
force=TRUE,
posControls="pos",
negControls="neg",
mainScriptFile="../R-scripts/run_cellHTS.R"
)
The outputs from this cellHTS2 analysis so far have been a TopTable plain text file and a folder containing an HTML report. It is possible to extract any data in the cellHTS objects using accessor methods in order to produce customized outputs. Here, we extract information on the targeted genes, the plate numbers, well numbers, and median Z -scores and combine this into a data frame (“combinedz”) containing four columns (compound, plate, well, and zscore).
genes <- geneAnno(xsc)
plates <-plate(xsc)
wells <- well(xsc)
scores <- Data(xsc)[,1,1]
combinedz <- data.frame(
compound=compounds,
plate=plates,
well=wells,
zscore=scores
)
We can then write out the “combined” data frame to a text file. A use case for this is to enable joining data from multiple screens into a single file for analysis.
write.table(
combinedz,
"zscore.txt",
sep="\t",
quote=FALSE,
row.names=FALSE
)
This analysis needs to be performed for each screen in the experiment. Typically, multiple distinct screens would represent multiple tumor cell lines screened with a specific library of siRNAs. Quality control steps need to be applied on a screen-by-screen basis. We expect siRNA screen replicates to be strongly correlated and reject screens where no pairs of replicates have a correlation coefficient greater than 0.7 ( see Note 10 ).
In an earlier step, we saved the output from the getTopTable() function to a data frame called “summary_info.” We can extract the replicate normalized luminescence values from this data frame and calculate the Pearson correlation coefficients for each pair of replicates using the following command.
cor(
summary_info[,c(
"normalized_r1_ch1",
"normalized_r2_ch1",
"normalized_r3_ch1"
)],
use="pairwise.complete.obs"
)
A further quality control step that is recommended is to examine the Z -prime ( Z ′) values for each screen [19]. Z ′ scores provide a measure of the separation of the positive and negative control siRNAs included in a screen and so can be considered an estimate of how much it is possible for the individual “sample” wells to vary in Z -scores. Larger values of Z ′ indicate better screens. Screens with Z ′ values ≥0.5 are considered excellent. Those with Z ′ values ≤0 are considered unusable and should be rejected and the experiments should be repeated. CellHTS2 calculates Z ′ scores for each replicate and these can be found in the HTML report under the “plate summaries” section.
3.2 Identification of Kinase Dependencies Associated with Driver Gene Mutation or Copy Number Alteration
We next integrate the processed results from multiple siRNA screens with data describing the genetic alterations present in each sample. For this tutorial we use the siRNA data from 18 osteosarcoma tumor cell lines and a mutations file that describes the presence or absence of genetic alterations in different members of the Retinoblastoma (RB1) pathway. In the git repository downloaded, there is a set of directories containing pre-formatted siRNA and mutation datasets as well as R scripts to process the data. Open the script R-scripts/identifying_CGDs_RB1_osteosarcoma.R and examine its contents. The first command sets the working directory to the top level of the git repository we cloned/downloaded earlier. Modification of the path given to the setwd() function is required to point to the appropriate location on your local system.
setwd("~/software/identifying-genetic-dependencies")
The next command runs R code contained in a second file in the R-scripts directory. The dot at the beginning of the path indicates that the path is relative to the current working directory. The file “identifying_CGDs_library.R” contains a set of functions that abstract the process of loading mutation and siRNA datasets as well as running a set of statistical tests. Readers familiar with R can examine the code in this file to understand the individual analysis steps in more detail.
source("./R-scripts/identifying_CGDs_library.R")
We next define the paths to the siRNA and mutation data files used in the analysis. It is a helpful to define this kind of information near the top of scripts so that in the future the files can be changed without having to find the commands where these values are used.
sirna_screens_file <- "./siRNA-data/Osteosarcoma_kinome_screens.txt"
rb_pathway_func_muts_file <- "./mutation-data/combined_exome_cnv_func_muts_RBpathway_160418.txt"
rb_pathway_all_muts_file <- "./mutation-data/combined_exome_cnv_all_muts_RBpathway_160418.txt"
The next command reads the siRNA and mutation datasets, identifies cell lines in common between each dataset, and returns an R list object containing analysis-ready tables. The input files comprise tab-separated data where the first row and first column represent column and row names respectively. Aside from the first row (column headings), each row contains data for a single-cell line. Each column represents a property measured across each cell line. In the “sirna_screens_file,” these properties are the Z -scores representing the relative viability of cells treated with siRNAs targeting specific genes. In the case of the mutation datasets (rb_pathway_func_muts_file and rb_pathway_all_muts_file), these properties represent the presence or absence of a driver gene alteration. The file rb_pathway_func_muts_file contains a “1” where a cell line is considered to contain a likely functional cancer driver gene alteration (mutation or copy number alteration) and a “0” where such a change is absent. Similarly, the file rb_pathway_all_muts_file contains a “1” or “0” to indicate the presence of any driver gene alteration found in a cell line irrespective of presumed functional impact. These two files are used to identify sets of cell lines where a driver gene is considered to be functionally altered (the mutant group) or where alterations to the driver gene are entirely absent (the wild-type group) ( see Note 11 ).
kinome_rb_muts <- read_rnai_mutations(
rnai_file=sirna_screens_file,
func_muts_file=rb_pathway_func_muts_file,
all_muts_file=rb_pathway_all_muts_file
)
With the siRNA and mutation data tables organized within kinome_rb_muts, we now run association tests between mutations or copy number alterations in RB1 pathway genes and test dependency on each gene targeted in the kinome siRNA library. The function run_univariate_tests() performs Wilcoxon Rank Sum tests between siRNA Z -scores of cell lines in the mutant and wild-type groups and returns a table of these test results as well as other information such as descriptive statistics (including the median Z -score of the mutant and wild-type group and the difference between those two values).
kinome_rb_mut_associations <- run_univariate_tests(
zscores=kinome_rb_muts$rnai,
mutations=kinome_rb_muts$func_muts,
all_variants=kinome_rb_muts$all_muts,
alt="less"
)
We write out the results of the association tests to a text file that can be opened in a spreadsheet application or used as input for other programs such as the annotate_dependencies.py python program described in Section 3.3.
write.table(
kinome_rb_mut_associations,
"./results/kinome_rb_mut_associations.txt",
sep="\t",
col.names=TRUE,
row.names=FALSE,
quote=FALSE
)
3.3 Annotating Molecular Dependencies According to Known Functional Relationships
In the absence of additional information, interpreting an association between the mutation of a driver gene and sensitivity to RNAi reagents targeting another gene can be difficult. One approach to aiding the interpretation of these associations is the integration of orthogonal data, including known functional relationships between genes or their protein products. We provide a simple Python script (annotate_dependencies.py) that can be used to integrate known functional relationships (e.g., protein-protein, kinase-substrate, or gene-regulatory interactions) with the associations generated by the R scripts described in Section 3.2. This script adds an additional column to the associations file indicating whether or not the marker-target gene pair has a known functional relationship according to a user-supplied source of interactions.
Create a file containing functional relationships between genes ( see Note 6 for potential sources of these relationships). Each line of this file should contain two gene symbols (HUGO gene names) separated by a tab. Alternatively, files in the BioGRID Tab 2.0 Format, such as those downloaded from the BioGRID database [20], can be used as input.
Open a command prompt/terminal and run the script as follows:
python annotate_dependencies.py -a <associations> -o <output> -i <interactions> -n <column_name>
where
View the resulting output in a text editor or spread sheet application. There should be an additional column in the file named using the <column_name> argument, with True or False values indicating whether each marker-target association involves a gene pair with a known functional relationships according to the
Additional columns can be added (e.g., to annotate the associations according to a different source of interactions) by running the script again using the output file (
The end result of this analysis is a file containing an annotated list of associations between a particular genomic feature (indicated in the “marker” column) and increased sensitivity to siRNA reagents targeting a particular gene (indicated in the “target” column). The column titled “PPI” in this file indicates whether the marker gene and the target have a known functional relationship (e.g., protein-protein interaction) while the column “wilcox.p” gives an indication of the statistical significance of the association. These p -values, together with the annotation of known functional relationships, may be used to prioritize candidate genetic dependencies (synthetic lethalities) for follow-up experiments. At a minimum these follow-up experiments should involve using orthogonal means to test the observed association (e.g., alternative siRNA reagents or a small molecule targeting the protein product of the gene of interest) [21]. Ideally, the follow-up validation would test the association in additional cell lines harboring the mutation of interest. In the example provided, we found that RB1 mutation is associated with increased sensitivity to siRNA targeting the kinase DYRK1A , a known RB1 binding partner. In Campbell et al. [5] we validated this in a larger panel of osteosarcoma cell lines using four distinct siRNA reagents targeting the DYRK1A gene suggesting that the initial observation represents a real dependency.