deltaTE: Detection of Translationally Regulated Genes by Integrative Analysis of Ribo-seq and RNA-seq Data
Sivakumar Viswanathan, Sivakumar Viswanathan, Stuart A. Cook, Stuart A. Cook, Sebastian Schafer, Sebastian Schafer, Sonia Chothani, Sonia Chothani, Eleonora Adami, Eleonora Adami, John F. Ouyang, John F. Ouyang, Norbert Hubner, Norbert Hubner, Owen J. L. Rackham, Owen J. L. Rackham
Abstract
Ribosome profiling quantifies the genome-wide ribosome occupancy of transcripts. With the integration of matched RNA sequencing data, the translation efficiency (TE) of genes can be calculated to reveal translational regulation. This layer of gene-expression regulation is otherwise difficult to assess on a global scale and generally not well understood in the context of human disease. Current statistical methods to calculate differences in TE have low accuracy, cannot accommodate complex experimental designs or confounding factors, and do not categorize genes into buffered, intensified, or exclusively translationally regulated genes. This article outlines a method [referred to as deltaTE (ΔTE), standing for change in TE] to identify translationally regulated genes, which addresses the shortcomings of previous methods. In an extensive benchmarking analysis, ΔTE outperforms all methods tested. Furthermore, applying ΔTE on data from human primary cells allows detection of substantially more translationally regulated genes, providing a clearer understanding of translational regulation in pathogenic processes. In this article, we describe protocols for data preparation, normalization, analysis, and visualization, starting from raw sequencing files. © 2019 The Authors.
Basic Protocol : One-step detection and classification of differential translation efficiency genes using DTEG.R
Alternate Protocol : Step-wise detection and classification of differential translation efficiency genes using R
Support Protocol : Workflow from raw data to read counts
INTRODUCTION
Next-generation sequencing methods have become commonplace tools in the life sciences, allowing researchers to understand the molecular mechanisms underpinning cellular processes, shaping phenotypic differences, and ultimately modifying disease susceptibility. While it is evident that mining every layer of gene expression would be required for a thorough understanding of gene regulation, expression profiling studies most commonly focus on the abundance of RNA molecules.
RNA sequencing (RNA-seq) is a methodology that quantifies fragments of RNA molecules to assess the level of gene transcription. To achieve this, sequencing reads are mapped to the genome and counted to quantify the expression of each gene. Significant changes in these counts between conditions identify genes undergoing transcriptional regulation. However, RNA-seq alone does not capture the full picture. While transcription serves to generate a broad collection of transcripts, the final expression of a gene is refined, and its fate determined, in the downstream stages of gene expression regulation, such as translational regulation, protein stability, protein degradation, and others.
Ribosome profiling (Ribo-seq) offers a quantitative approach to study translational regulation, a post-transcriptional process affecting protein levels. Transcriptome-wide translation is quantified via the capture of ribosome-protected RNA fragments (RPFs; Ingolia, Ghaemmaghami, Newman, & Weissman, 2009; also see Fig. 1A). Changes in the number of RPFs between conditions for a given gene can be used as a proxy for a change in the translation of the encoded protein. However, reliably identifying differences in translational regulation is complicated by the fact that the mRNA abundance of the transcript directly affects the probability of ribosome occupancy.
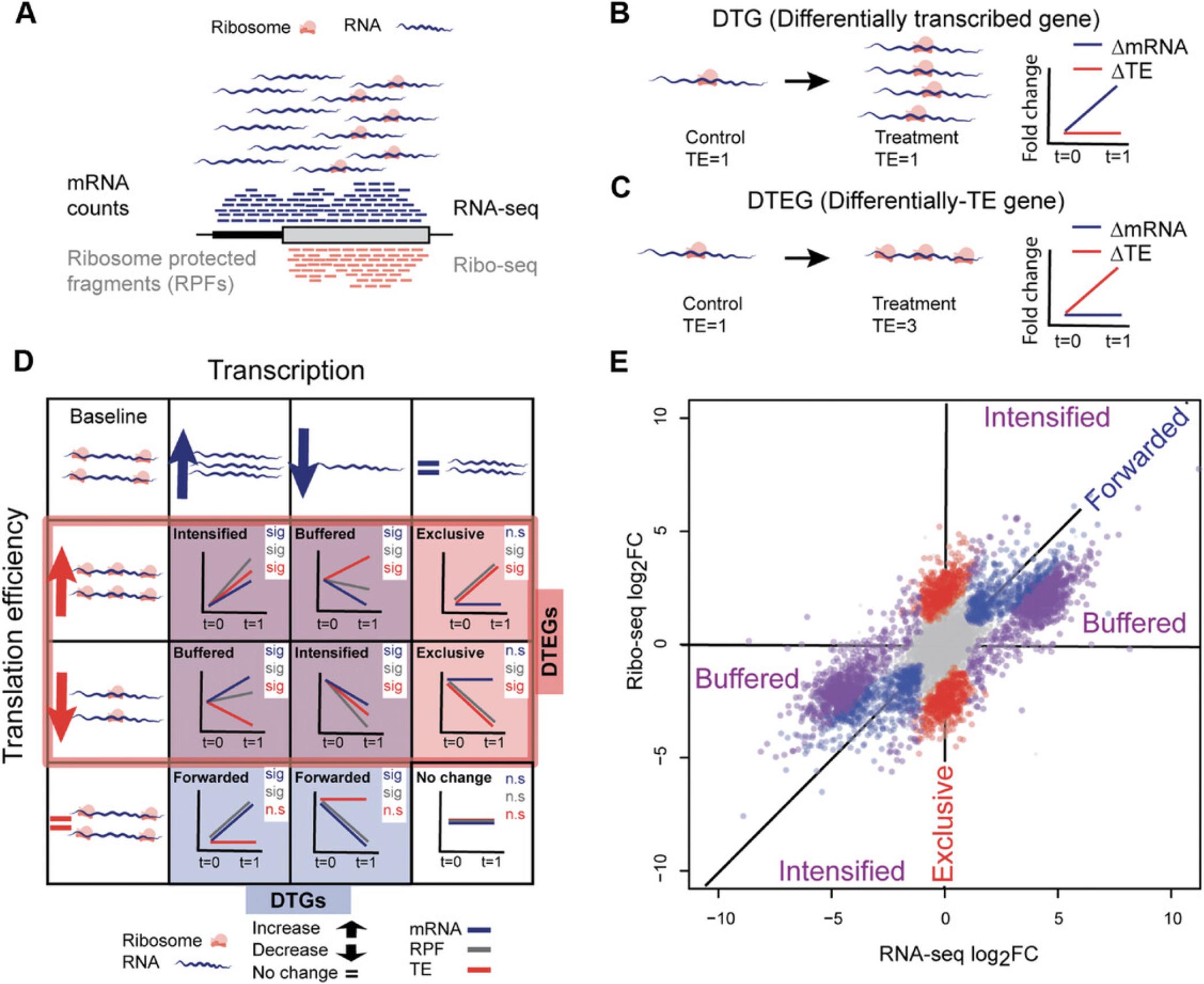
The number of ribosomes per transcript can be estimated by integrating RNA-seq and Ribo-seq to calculate translation efficiency (TE), the ratio of the RPFs over mRNA counts within a gene's coding sequence (CDS). TE is essentially the number of ribosomes per gene, normalized to transcript abundance. Genes with changes in TE between conditions are considered to undergo translational regulation [differential translation efficiency genes (DTEGs)]. Specifically, a gene is classified as DTEG if the changes in the number of RPFs cannot be explained by variation in mRNA read counts. A gene with a significant change in its mRNA counts and a concordant change in RPFs is transcriptionally, but not translationally, regulated [differentially transcribed gene (DTG); Fig. 1B]. Conversely, genes that have significant changes in RPFs independent of changes in mRNA counts are considered DTEGs (Fig. 1C).
A gene can be regulated transcriptionally and/or translationally, resulting in several different regulatory profiles. For example, if a gene is not a DTG, but is a DTEG, then it is exclusively regulated at the translational level. On the contrary, if a gene is both a DTG and DTEG, it is categorized as translationally intensified or buffered depending on the direction of the regulation (see Figure 1D, E for details).
There are a number of existing approaches to detect DTEGs by combining Ribo-seq and RNA-seq data, with the earliest report based on differences in TE (Ingolia et al., 2009). However, this approach does not take into account the variance, low expression of RPFs or mRNA counts, or batch effects, severely compromising the accuracy of detection. Several other approaches to detect DTEGs by modeling changes in TE have been developed subsequently: Ribodiff (Zhong et al., 2017), Xtail (Xiao, Zou, Liu, & Yang, 2016), Riborex (Li, Wang, Uren, Penalva, & Smith, 2017), and Anota2Seq (Oertlin et al., 2019). At their core, all of these approaches either utilize existing differential expression programs [e.g., DEseq2 (Love, Huber, & Anders, 2014) or EdgeR (Robinson, McCarthy, & Smyth, 2010)], or apply similar statistical assumptions to model the data. Unfortunately, these methods mostly miss essential functionalities of the underlying tools, vastly reducing their effectiveness. For instance, none of these methods, with the exception of Anota2Seq, allow for complex experimental design (i.e., with more than two conditions) or the use of alternative statistical setups (such as likelihood ratio tests for comparisons across time). Crucially, they do not account for the widespread batch effects in next-generation sequencing datasets. Although stand-alone tools for batch correction of sequencing data exist (Leek et al., 2010), differential expression tools require raw read counts to accurately model sample-to-sample variation (Anders et al., 2013; also see Table 1).
Tool | Xtail | RiboDiff | RiboRex | Anota2Seq | ΔTE (our approach) |
---|---|---|---|---|---|
Sample-to-sample variance | Yes | Yes | Yes | Yes | Yes |
Based on established statistical frameworks partly/or completely | Yes | Yes | Yes | ||
Allows for complicated experimental design | Yes | Yes | |||
Allows for covariates like batch effects | Yes | Yes | |||
Classifies regulatory layers | Yes, but not all | Yes | |||
Runtime (for primary human fibroblast dataset (Chothani et al., 2019), four pair-wise comparisons) | ∼120 min | ∼60 min | ∼5 min | ∼20 min | ∼5 min |
This article outlines detection of DTEGs by introducing an interaction term into the statistical model of DESeq2, an approach that we refer to as ΔTE. We show that the fold change of the interaction term is equivalent to changes in TE, which detect DTEGs more accurately compared to all existing methods. When combining RNA-seq and Ribo-seq from two conditions, the interaction term can be used to model condition (untreated/treated) and sequencing methodology (Ribo-seq/RNA-seq). This allows the identification of significant differences between conditions that are discordant between sequencing methodologies. In order to do this, we design our generalized linear model with three components: the condition (c), the sequencing type (s), and an interaction term containing both (c:s); refer to the Commentary for details. The result is a ΔTE fold change and an associated false discovery rate (FDR) for significant changes of this fold change, which quantify the extent of translational regulation between conditions.
The protocols require the installation of R and basic familiarity with R or a Unix-like environment. The workflow in the Basic Protocol includes a script, DTEG.R, which can be run in one step. This script implements two processes: (a) detection of DTEGs and (b) classification of genes into regulatory classes. An Alternate Protocol is included that carries out the same functions step-by-step in R, allowing flexibility in the case of complex experimental designs. Lastly, a Support Protocol is provided that outlines the workflow of obtaining count matrices from raw sequencing files, including a quality check of the data.
The Basic Protocol and example results are provided in our github repository: https://github.com/SGDDNB/translational_regulation.git.
STRATEGIC PLANNING
Ribo-seq can be carried out as described in the Current Protocols article Ingolia, Brar, Rouskin, McGeachy, & Weissman (2013). Similar to RNA-seq analysis, careful experimental design is crucial. At least three biological replicates per condition or group are recommended for robust analysis of differential transcription, translation, and translational efficiency. The sample processing and library preparation should be carried out together for different conditions and sequenced on the same lane of a sequencing machine, or in a randomized order across lanes, to avoid batch effects. It is not possible to account for batch effects that are completely confounded by any other covariate. For instance, if all the control samples were prepared in one batch and the treatment samples in another batch, it would not be possible to distinguish differences due to treatment versus control from differences arising due to separate preparation batches. Thus, it is recommended to prepare control and treatment samples together. Alternatively, when there are large sample sizes, it is important to split the samples in such a way that the conditions are randomized. Samples should be sequenced to sufficient depth both in RNA-seq and Ribo-seq. Despite the presence of an experimental step to remove ribosomal RNA (rRNA) fragments from the input RNA, sequenced Ribo-seq reads still include a fraction of rRNA sequences, which should be discarded before ΔTE analysis. Thus, it is recommended to sequence at least 20 million reads per sample. Single-end 50-bp read sequencing is sufficient, since ribosome footprints are expected to be 29 bp in length. After sequencing and processing the data, the fastq and alignment files should be checked for several quality measures, as described in the Support Protocol.
Basic Protocol: ONE-STEP DETECTION AND CLASSIFICATION OF DIFFERENTIAL TRANSLATION EFFICIENCY GENES (DTEG) USING DTEG.R
The RNA-seq and Ribo-seq data should be processed first as described in the Support Protocol, in order to determine translationally regulated genes. In the following steps, we quantify the change in TE of each gene, calculate an FDR value for this change, and categorize genes into regulation classes using the ΔTE approach. A DTEG is determined based on significant change in TE (FDR < 0.05). This protocol describes a wrapper script, DTEG.R, to detect and classify DTEGs. It also includes a script to visualize the transcriptional, translational, and TE changes for a gene of interest. Alternatively, the protocol can also be carried out step-by-step in R, allowing flexibility for complex experimental designs (see Alternate Protocol).
Materials
Hardware
- Computer running Unix, Linux or Mac OS X
- Administrative privileges and internet connection to install packages
Software
- DTEG.R and goi_viz.R script: These scripts can be downloaded from our github page by typing the following command in the terminal window:
- R: https://cran.r-project.org/bin/windows/base/
- Rstudio: https://www.rstudio.com/products/rstudio/download/
- DESeq2: https://bioconductor.org/packages/release/bioc/html/DESeq2.html
- DESeq2 can also be installed in R by typing the following command:
-
if(!requireNamespace("BiocManager", quietly = TRUE)) install.packages("BiocManager")
-
BiocManager::install("DESeq2")
-
Input files
- ribo_counts.txt: RPF count matrix including genes as rows and samples as columns
- rna_counts.txt: mRNA count matrix including genes as rows and samples as columns
- sample_info.txt: Sample-wise information about experimental condition, type of sample (RNA- or Ribo-seq), and sample batch where applicable
Preparing input files for DTEG.R
1.Generate read count matrices for both Ribo-seq and RNA-seq, as described in the Support Protocol.
Gene ID | Sample 1 | Sample 2 | Sample 3 | Sample 4 |
ENSG0000XX | 1290 | 130 | 2 | 1000 |
ENSG0000XY | 0 | 2 | 10 | 5 |
…. | .. | .. | .. | .. |
ENSG0000ZZ | 0 | 2 | 10 | 5 |
Gene ID | Sample 5 | Sample 6 | Sample 7 | Sample 8 |
ENSG0000XX | 4000 | 2000 | 200 | 1200 |
ENSG0000XY | 10 | 20 | 0 | 40 |
…. | .. | .. | .. | .. |
ENSG0000ZZ | 0 | 2 | 10 | 5 |
2.Create a tab-separated sample information file with rows as samples and columns as condition and sequencing methodology.
Sample ID | Condition | SeqType | Batch |
Sample 1 | 1 | RIBO | 1 |
Sample 2 | 1 | RIBO | 2 |
Sample 3 | 2 | RIBO | 1 |
Sample 4 | 2 | RIBO | 2 |
Sample 5 | 1 | RNA | 1 |
Sample 6 | 1 | RNA | 2 |
Sample 7 | 2 | RNA | 1 |
Sample 8 | 2 | RNA | 2 |
Detecting and categorizing differentially transcribed genes and differential translation efficiency genes
3.Open a Unix/Linux command line environment (“Terminal” application in a Linux operating system or Mac OS). Run script DTEG.R using the following command line:
- $ Rscript DTEG.R arg1 arg2 arg3 arg4 arg5 arg6
- where command arguments arg1-6 are as follows:
- Argument 1 (arg1): Ribo-seq count matrix file path
- Argument 2 (arg2): RNA-seq count matrix file path
- Argument 3 (arg3): Sample information file path
- Argument 4 (arg4): Batch effect covariate: yes=1, or no=0
- Argument 5 (arg5): Save Rdata file as a record for future use (optional, Default = 1)
- Argument 6 (arg6): Verbose mode (optional, Default = 0)
Example:
- $ Rscript DTEG.R ./ribo_counts.txt ./rna_counts.txt ./sample_info.txt 1
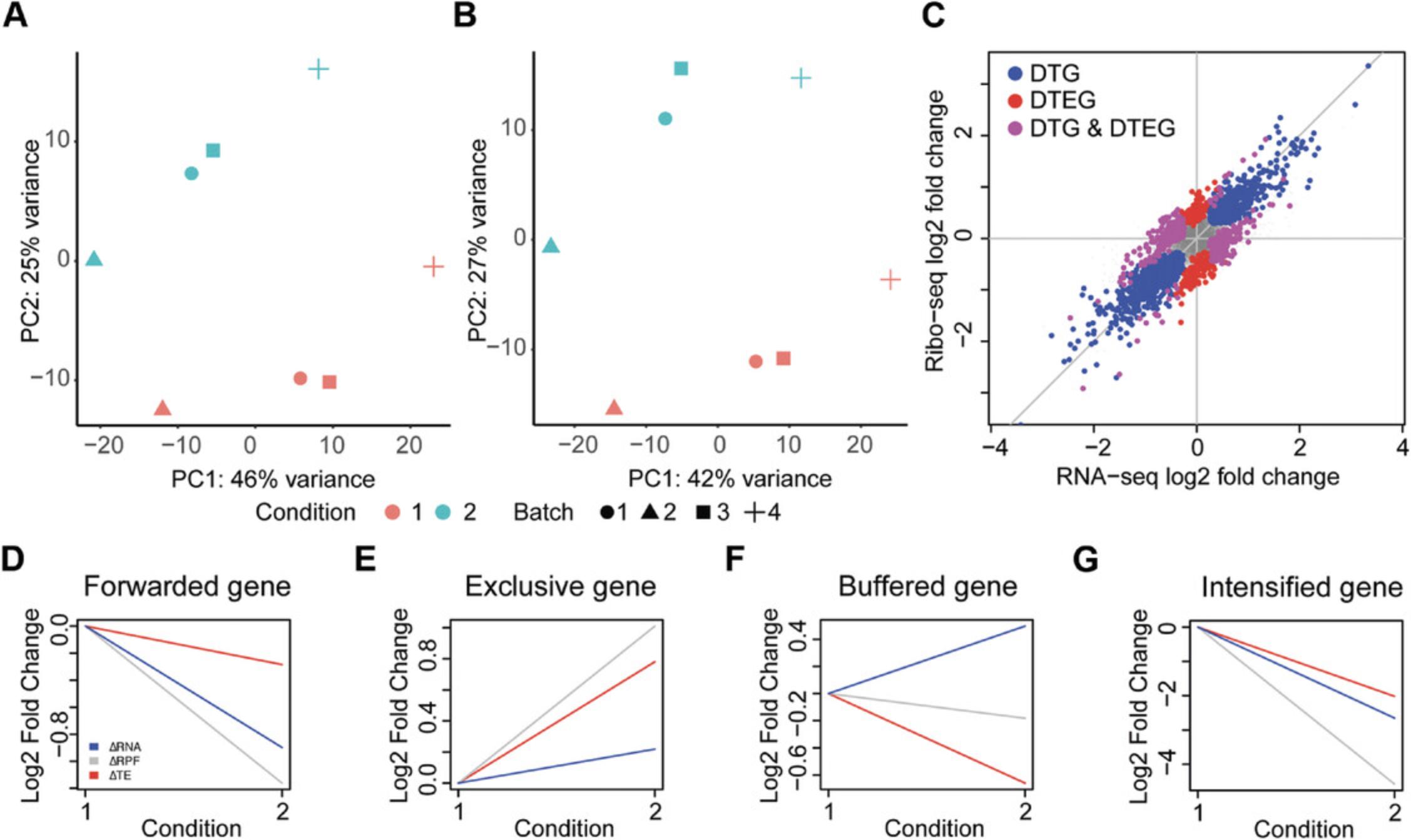
Visualizing changes in mRNA counts, RPFs, and TE for a gene of interest
4.Run goi_vis.R.
This step includes a one-step script to visualize the fold changes across the condition given in the study for a gene of interest as shown in Figure 2D-G:
- $ Rscript goi_viz.R arg1 arg2 arg3 arg4
- where command arguments arg1-6 are as follows:
- Argument 1 (arg1): Ribo-seq fold change file path.
- Argument 2 (arg2): RNA-seq fold change file path.
- Argument 3 (arg3): TE fold change file path.
- Argument 4 (arg4): ENSEMBL gene ID
Example:
-
$ Rscript goi_viz.R
-
path/to/Results/directory/fold_changes/deltaRibo.txt
-
path/to/Results/directory/fold_changes/deltaRNA.txt
-
path/to/Results/directory/fold_changes/deltaTE.txt
-
ENSG00000095752
Alternate Protocol: STEP-WISE DETECTION AND CLASSIFICATION OF DIFFERENTIAL TRANSLATION EFFICIENCY GENES USING R
This protocol performs the same task as the Basic Protocol, but step-wise in R, describing each step allowing flexibility to users for complex experimental designs.
Materials
Hardware
- Computer running Unix, Linux or Mac OS X
- Administrative privileges and internet connection to install packages
Software
- R: https://cran.r-project.org/bin/windows/base/
- Rstudio: https://www.rstudio.com/products/rstudio/download/
- DESeq2: https://bioconductor.org/packages/release/bioc/html/DESeq2.html
- DESeq2 can also be installed in R by typing the following command:
-
if(!requireNamespace("BiocManager", quietly =TRUE)) install.packages("BiocManager")
-
BiocManager::install("DESeq2")
-
Input files
- ribo_counts.txt: RPF count matrix including genes as rows and samples as columns
- rna_counts.txt: mRNA count matrix including genes as rows and samples as columns
- sample_info.txt: Sample-wise information on sequencing methodology used, condition and batch
1.Prepare input files as described in steps 1 and 2 of the Basic Protocol.
2.Open Rstudio and load count matrices and sample information file:
-
ribo_counts = read.delim(“ribo_counts.txt”)
-
rna_counts = read.delim(“rna_counts.txt”)
-
sample_info = read.delim(“sample_info.txt”)
3.Create DESeq2 object for the combined dataset of Ribo-seq and RNA-seq counts. The interaction term should be included in the linear model design as follows:
-
ddsMat = DESeqDataSetFromMatrix(
- countData=cbind(ribo_counts,rna_counts),
- colData=sample_info,
- design=∼ Condition+SeqType+Condition:SeqType)
4.Run DESeq2:
-
ddsMat = DESeq(ddsMat)
5.Obtain fold changes for TE:
-
res = results(ddsMat, name=“Condition2.SeqTypeRIBO”)
Detecting differential translation efficiency genes
6.Store the list of DTEGs in a file:
-
write.table(res[which(res$padj<0.05), ], “DTEGs.txt”, quote=F)
7.Run DESeq2 for mRNA counts in order to obtain DTGs:
-
ddsMat_rna = DESeqDataSetFromMatrix(
- countData=rna_counts,
- colData=sample_info[which(samples_info$SeqType == “RNA”),],
- design=∼Condition)
-
ddsMat_rna = DESeq(ddsMat_rna)
-
res_rna = results(ddsMat_rna, name="Condition_2_vs_1")
-
res_rna = lfcShrink(ddsMat_rna,name="Condition_2_vs_1",res=res_rna)
-
write.table(res_rna[which(res_rna$padj<0.05), ],“DTGs.txt”, quote=F)
Categorizing genes into different regulation groups
8.Run DESeq2 for RPFs (Ribo-seq counts):
-
ddsMat_ribo = DESeqDataSetFromMatrix(
- countData=ribo_counts,
- colData=sample_info[which(samples_info$SeqType == “RIBO”),],
- design=∼Condition)
-
ddsMat_ribo = DESeq(ddsMat_ribo)
-
res_ribo = results(ddsMat_ribo,name="Condition_2_vs_1")
-
res_ribo =
-
lfcShrink(ddsMat_ribo,name="Condition_2_vs_1"),res=res_ribo)
9.Obtain genes for each regulation class described in Figure 1D, E.
-
Forwarded: Genes driven by transcriptional regulation. These genes do not have a change in TE, and the change in RNA drives the change in RPFs. Hence, genes that have significant ΔRPF and ΔRNA but that do not have a significant ΔTE fall into this class.> forwarded = rownames(res)[which(res$padj > 0.05 & res_ribo$padj < 0.05 & res_rna$padj < 0.05)]
-
Exclusive: Genes regulated exclusively by translation. This means that the change in TE is driven by change in RPFs exclusively, and there is no change in mRNA counts. Hence, genes with significant ΔTE and ΔRPFs but no significant change in mRNA counts belong to this group.> exclusive = rownames(res)[which(res$padj < 0.05 & res_ribo$padj < 0.05 & res_rna$padj > 0.05)]
-
Intensified and buffered: Genes regulated both by transcriptional and translational regulation (significant ΔRNA, ΔRPFs, and ΔTE) include intensified and buffered genes. These genes are both DTGs and DTEGs.> both = rownames(res)[which(res$padj < 0.05 & res_ribo$padj < 0.05 & res_rna$padj < 0.05)]
In order to further categorize these genes into intensified and buffered genes, the direction of the transcriptional change (ΔRNA) and translational efficiency change (ΔTE) are compared.
Intensified : Genes for which the translational regulation acts with the transcriptional regulation change. These genes have the translational change in the same direction as their transcriptional change:
-
intensified = rownames(res)[both[which(res[both,2]*res_rna[both,2] > 0)]]
Buffered : Genes for which the translational regulation counteracts the transcriptional regulation change. In these genes, the transcriptional change (ΔRNA) and translational efficiency change (ΔTE) are in the opposite direction:
-
buffered = rownames(res)[both[which(res[both,2]*res_rna[both,2] < 0)]]
There is also a special case of buffered genes wherein the transcriptional change is cancelled out by the change in TE to the point of no significant change in RPFs. Hence, genes with significant ΔTE and ΔRNA but that do not have a significant ΔRPF are also considered as translationally buffered.
-
buffered = c(rownames(res)[which(res
padj > 0.05 & res_rna$padj < 0.05)], buffered)
- a
DTG, differentially transcribed gene; DTEG, differential translation efficiency gene; n.s., not significant; sig, significant.
- b
Genes with any other combinations, i.e., 1 RPF: n.s.; RNA: sig, TE: n.s.; (2) RPF: sig; RNA: n.s., TE: n.s.; and (3) RPF: n.s.; RNA: n.s., TE: sig; are considered as undetermined as they cannot be grouped into any of the classes.
10.Visualize the global translational and transcriptional regulation as in Figure 1E.
-
max_val = max(res_ribo[,2],res_rna[,2],na.rm = T)
-
plot(y=res_ribo[,2],x=res_rna[,2],
- xlab="RNA-seq log2 fold change",
- ylab = "Ribo-seq log2 fold change", asp=1, pch=16,
- col=rgb(128/255,128/255,128/255,0.1), ylim=c(-
- max_val,max_val), xlim=c(-max_val,max_val),cex=0.4)
-
abline(a=0,b=1,col="gray")
-
abline(h=0,v=0,col="gray")
-
points(y=res_ribo[forwarded,2], x=res_rna[forwarded,2],
- pch=16,col=rgb(0,0,1,1))
-
points(y=res_ribo[exclusive,2], x=res_rna[exclusive,2],
- pch=16,col=rgb(1,0,0,1))
-
points(y=res_ribo[intensified,2], x=res_rna[intensified,2],
- pch=16,col=rgb(1,0,1,1))
-
points(y=res_ribo[buffered,2], x=res_rna[buffered,2],
- pch=16,col=rgb(1,0,1,1))
Visualizing changes in mRNA counts, RPFs, and TE for a gene of interest
11.Visualize the transcriptional, translational, and TE changes of a given gene id [id] using a line plot.
-
ymax=max(res_ribo[id ,2],res_rna[id ,2],res[id ,2],0)
-
ymin=min(res_ribo[id ,2],res_rna[id ,2],res[id ,2],0)
-
plot(c(0,1), c(0,res_ribo[id ,2]), type="l",col="gray",
- ylim=c(ymin,ymax), ylab="Log2 fold change",xlab="",xaxt="n")
-
lines(c(0,1), c(0,res_rna[id ,2]),type="l",col="blue")
-
lines(c(0,1), c(0,res[id ,2]), type="l",col="red")
-
legend("bottomleft",c("RNA","Ribo","TE"),fill=c("blue","gray","red"),
- cex=1, border = NA, bty="n")
-
axis(1,at=c(0,1),labels=c(1,2),las=1)
Support Protocol: WORKFLOW FROM RAW DATA TO READ COUNTS
The raw sequencing data should be processed prior to the Basic Protocol or Alternate Protocol, as shown below. It is also strongly recommended to carry out quality check for the raw and processed data as described in the following steps.
Materials
Hardware
- Computer running Unix, Linux or Mac OS X
Software
- Trimmomatic: http://www.usadellab.org/cms/?page=trimmomatic
- Bowtie2: http://bowtie-bio.sourceforge.net/bowtie2/index.shtml
- STAR: https://github.com/alexdobin/STAR
- subread: http://subread.sourceforge.net/
- FastQC: https://www.bioinformatics.babraham.ac.uk/projects/download.html
- MultiQC: https://multiqc.info
- Ribo-TISH: https://github.com/zhpn1024/ribotish/blob/master/INSTALL.rst
Input files
- seq.fastq.gz: Raw sequencing files for both Ribo-seq and RNA-seq
- adaptors.fa: List of adaptor sequences in a fasta format
- abundant.fa: List of abundant sequences (rRNA, transfer RNA (tRNA), and mitochondrial RNA (mtRNA)) in fasta format
- organism.fa: Genome sequence in fasta format for the organism used in the study
- organism.gtf: Genome-wide transcript annotations in gene transfer format (GTF) for the organism used in the study
Processing the raw sequencing data to generate gene expression count matrix files
1.Trim adaptor sequences from reads:
- $ java -jar trimmomatic-0.36.jar SE -phred33 seq.fastq.gz outfile ILLUMINACLIP:adaptors.fa:2:30:10 MAXINFO:20:0.5 MINLEN:20
where:
- seq.fastq.gz is the raw sequencing file;
- outfile is the output file prefix;
- adaptors.fa is the list of sequences of adaptors used for sequencing in fasta format;
- MINLEN is the minimum length of reads required to retain.
2.Remove reads mapping to abundant sequences.
- $ bowtie2-build abundant.fa index
Where:
- abundant.fa is the list of abundant sequences (rRNA, tRNA, and mtRNA) in fasta format;
- index is the prefix for the bowtie index output files.
- $ bowtie2 -L 20 -x index --un-gz outfile -U infile -S samfile
Where:
- infile is the trimmed sequencing fastq.gz file, which was the outfile obtained in step 1;
- outfile is the output filename for unmapped reads in fastq.gz format;
- samfile is the output filename for mapped reads in SAM format;
- index is the prefix used for the bowtie index.
3.Align reads to the genome file using the transcriptome index.
- $ STAR --runMode genomeGenerate --genomeDir --genomeFastaFiles organism.fa --sjdbGTFfile organism.gtf
Where:
- organism.fa is the genome sequence in fasta format;
- organism.gtf is the genome-wide transcript information;
- genomeDir is the directory name for the output STAR index files.
- $ STAR --runThreadN 16 --alignSJDBoverhangMin 1 --alignSJoverhangMin 51 --outFilterMismatchNmax 2 --alignEndsType EndToEnd --genomeDir star2.5.2b_genome_index --readFilesIn infile --readFilesCommand gunzip -c --outFileNamePrefix outPrefix --quantMode GeneCounts --outSAMtype BAM SortedByCoordinate --limitBAMsortRAM 31532137230 --outSAMattributes All
Where:
- genomeDir is the directory name for the STAR index files generated in the previous step;
- infile is the cleaned fastq.gz file, which was the outfile in step 2;
- outPrefix is the prefix for the output filenames.
4.Count reads mapped to coding regions of genes:
- $ featureCounts -t CDS -g gene_id -O -s 1 -J -R -G organism.fa -a organism.gtf -o outfile infile_path/*bam
Where:
- organism.fa is the genome sequence in fasta format;
- organism.gtf is the genome-wide transcript information;
- outfile is the output file name for the count matrix;
- infile_path is the path to the directory containing all bam files obtained in step 3.
Quality check of the raw sequencing data and processed files
5.Run FastQC:
- $ fastqc [filename].fastq.gz
6.Run MultiQC to summarize QC for all the steps in Support Protocol:
- $ multiqc/path/to/parent/directory/of/all/log/files/
7.Calculate and visualize periodicity of Ribo-seq dataset:
- $ samtools index [bam_file_prefix].bam
- $ ribotish quality -b [bam_file_prefix].bam -g ensemble.gtf
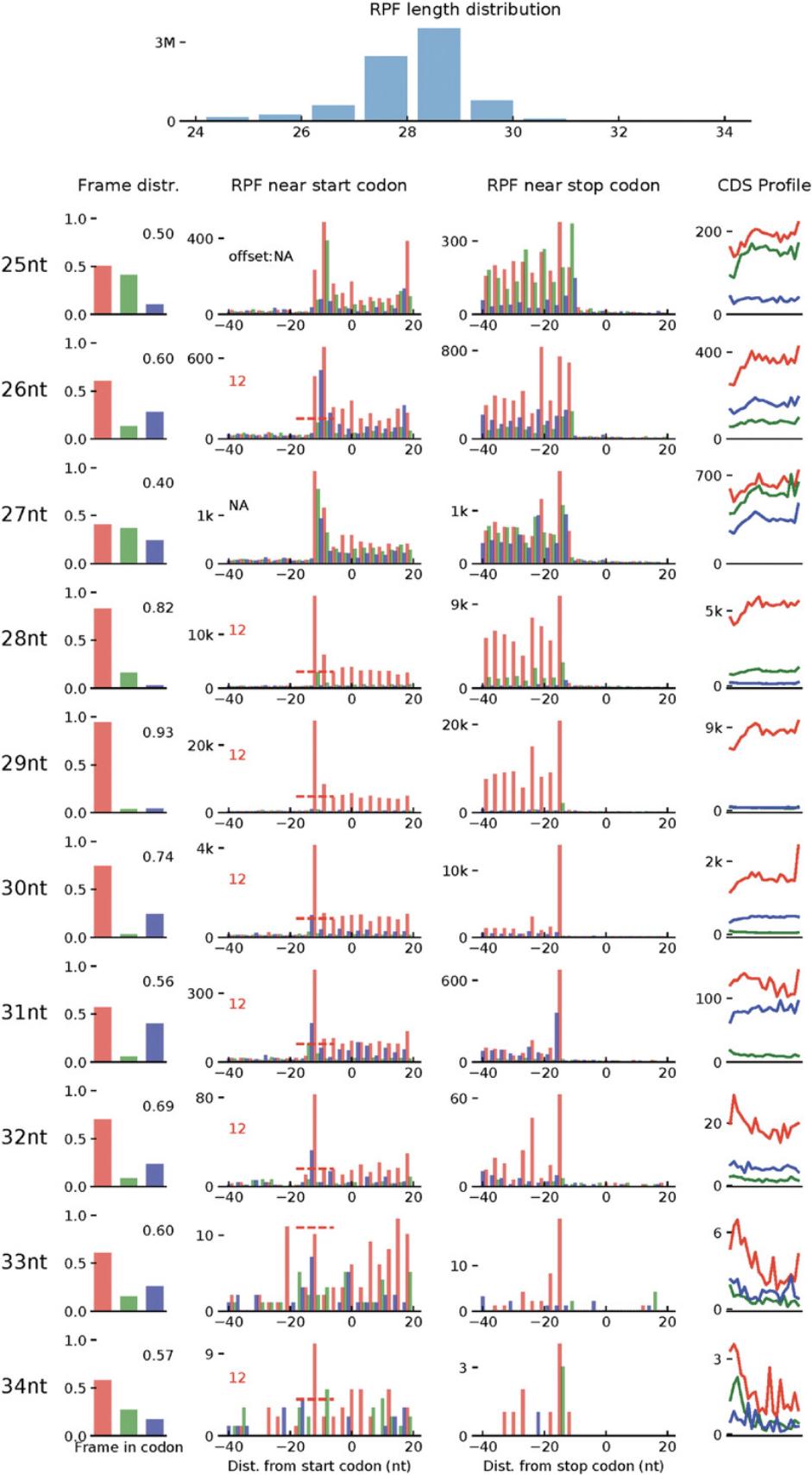
COMMENTARY
Background Information
Several methods have been developed for read alignment and read counting since the advent of RNA-seq (see Current Protocols article Ji & Sadreyev, 2018). In the Support Protocol, we use STAR, bowtie2, and feature counts for both Ribo-seq and RNA-seq datasets. These tools can be chosen based on user preferences. Due to the slightly different nature of Ribo-seq reads, it is important to modify parameters accordingly. For instance, since the RPFs are expected to be around 29 bp, soft clipping of reads can be quite detrimental to alignment pipelines and is not recommended. Furthermore, RNA-seq pipelines use six to eight allowed mismatches, but this can be quite large in a 29-bp read. We recommend one to two allowed mismatches for a robust downstream analysis.
In this protocol, we describe an interaction term–based TE analysis using DESeq2, but a similar model can also be incorporated in other generalized linear model–based differential expression tools such as edgeR. Previously, several publications have used DESeq2 to identify DTEGs, but in a suboptimal manner. For instance, these tools are used to calculate ΔRPF and ΔRNA, following which changes in TE are calculated using the ratio ΔRPF/ΔRNA. The translationally regulated genes are then identified using |z -score| > 1.5 (Xu et al., 2017). This approach is referred to as the Ratio method in the benchmarking analyses. Another approach used previously also involves quantification of ΔRPF and ΔRNA using DESeq2. However, in this case, the translationally regulated genes are defined as genes with significant changes in either RPF or mRNA levels, but not both (Schafer et al., 2015). This approach falsely calls genes as translationally exclusive or buffered in cases where counts have a large variance across samples or are very low in either sequencing methodology. It would be unable to differentiate between a case where a gene is translationally regulated and a case where a gene has low counts/high variation in one of the sequencing methodologies. This is referred to as the Overlap method in the benchmarking analyses.
In order to benchmark the performance of our approach, we use three independent simulation datasets, two derived from previous publications (Oertlin et al., 2019, Xiao et al., 2016) and a third that was newly generated to evaluate the performance of the tools in the presence of a batch effect. Despite DESeq2 being a key component of many existing approaches, it was either not included or not used correctly in previous benchmarks.
Figure 4A-C shows accuracy curves for detection of DTEGs in each of these benchmarking datasets across typically used FDR thresholds. A full receiver operating characteristic (ROC) and area under the curve (AUC) analysis can be found in the associated web resource. Our benchmarking shows that ΔTE has a superior accuracy in comparison to existing methods, especially in the presence of a batch effect. The only method that performs at a similar level to ΔTE is RiboDiff, in the case of the data from Oertlin et al. (2019) (Fig. 4A). However, in the presence of a batch effect or based on the data from Xiao et al. (2016), ΔTE is superior.
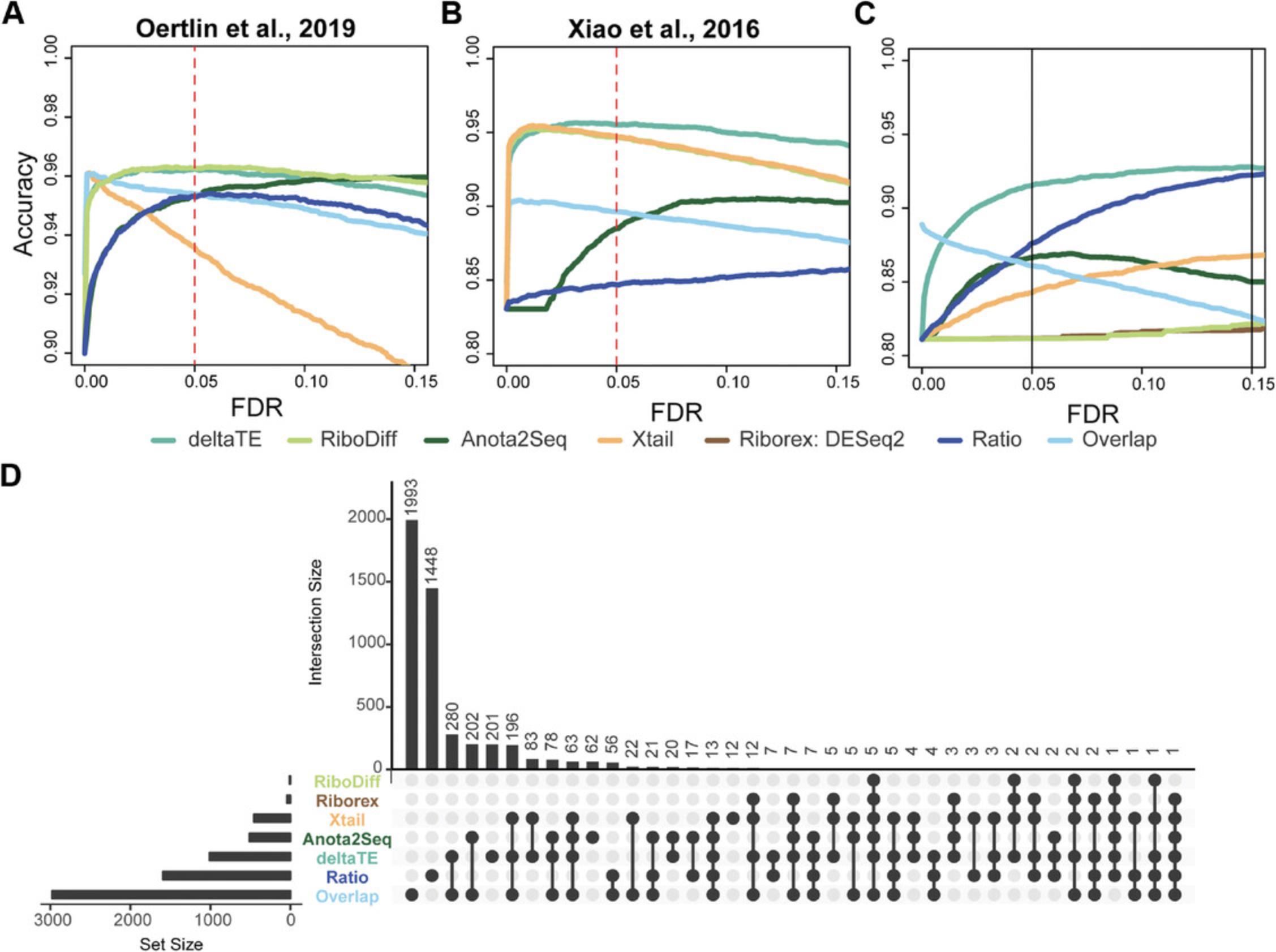
To further verify that this effect is not confined to simulated data, we analyzed RNA-seq and Ribo-seq data derived from our recent study on cardiac fibrosis (Chothani et al., 2019). This experiment contained cardiac fibroblasts from four different individuals, and, as a result, has a pronounced patient-related batch effect accounting for roughly 25% of the variance within the data. While it is not possible to quantify the accuracy of these real data, it is consistent with the benchmark results. For instance, the overlap and ratio methods predict the highest number of DTEGs, but were shown to have high FP rates in the benchmarking. Conversely, other existing tools that detect very few genes consistently showed the worst accuracy in the benchmark containing batch effects.
Taken together, the three benchmark studies and real data analysis strongly suggest that the ΔTE method is the most suitable for any integrative analysis of Ribo-seq and RNA-seq data, being both accurate and robust regardless of the data being analyzed.
Critical Parameters and Troubleshooting
Experimental design is one of the most important factors for efficient detection of DTEGs. In best-case scenarios, designs should avoid batch effects. Unavoidable batch effects should not be completely confounded with the groups of interest. This would lead to a non-full-rank design in DESeq2, which makes correction of the batch effect impossible within the model. It is recommended to evaluate samples for batch effects or outliers using PCA prior to analysis. Batch effects can be checked by visualizing PC1 and PC2, which account for most variance, and the remaining PCs can also be explored to identify minor batch effects.
Installation of tools can be quite cumbersome due to different platforms and versions. Apart from the standard installation procedures provided in the protocols, the required tools can also be installed using the Anaconda software package https://docs.anaconda.com/anaconda/install/. For instance, some of the tools used in Support Protocol can be installed with the following commands:
conda install -c bioconda trimmomatic conda install -c bioconda bowtie2 conda install -c bioconda subread conda install -c bioconda star
Statistical Analysis
DESeq2 utilizes the Wald test for differential expression analysis in pair-wise data (i.e., two conditions). If the experimental design includes a time-series, each time point can be compared pair-wise using the Wald test. Alternatively, the likelihood ratio test within DESeq2 can be used, which is more suitable to identify differences across a time-series.
Mathematical proof: Interaction term coefficient is equivalent to the changes in translation efficiency
The interaction term in a generalized linear model provides a coefficient that models the non-additive effects of two variables. The design described in the protocol corresponds to the following linear equation (Equation 1):
where c = condition and s = sequencing methodology. When this is used to model changes in the gene expression between conditions, it is possible to disentangle the transcriptional and translational contributions. For example, in an experimental setup with Ribo-seq (s = 1) and RNA-seq (s = 0) carried out over two conditions (c = 0 or 1), the gene-wise transcriptional and translational changes are calculated as follows.
First, the coefficients contributing towards the mRNA levels (s = 0) are identified for each condition (c = 0 or 1) separately. We then compute the difference of the identified coefficients to obtain the change in transcription.
mRNA levels given condition (c = 1) and sequencing methodology (s = 0) using Equation 1:
mRNA levels given condition (c = 0) and sequencing methodology (s = 0):
Change in mRNA levels between the two conditions:
Similarly, the coefficients contributing towards the RPF counts (s = 1) can be quantified, and the differences signify the change in RPFs of a gene across conditions.
RPFs given condition (c = 1) and sequencing methodology (s = 0) using Equation 1:
RPFs given condition (c = 0) and sequencing methodology (s = 0) using Equation 1.
Changes in RPFs between the two conditions:
In order to obtain the translational changes that are independent of transcriptional changes, we subtract the changes in mRNA from the change in RPFs. This is equivalent to the interaction term coefficient β 3 as follows:
Changes in RPFs – Changes in mRNA levels:
Thus, β3, which is the interaction term coefficient, is equal to translational changes that are independent of transcriptional changes. Importantly, it is also possible to show that this interaction term coefficient is equivalent to the fold change in TE:
Further, since TE is defined as the ratio of mean normalized Ribo-seq counts (RPFs) over RNA-seq counts,
where TE is the translation efficiency.
As a result, the fold change (and associated adjusted p -value) obtained using the interaction term coefficient β3 describes, for each gene, the change in TE. Genes with a significant adjusted p -value for ΔTE are considered as DTEGs. Since this is a linear model, the design can also be extended to facilitate more complex experimental designs, such as batch effects or other covariates, making it a powerful tool for identifying DTEGs.
Understanding Results
The Basic Protocol implements the script DTEG.R from our github repository (https://github.com/SGDDNB/translational_regulation). This generates a results directory which includes two subdirectories (fold_changes/, gene_lists/) and one file (Results_figures.pdf).
In order to demonstrate the usage and output of DTEG.R, we utilized Ribo-seq and RNA-seq count data from our recent study (Chothani et al., 2019) on primary human fibroblasts stimulated with TGFB1. We obtained a subset of this dataset using four patients and two conditions (unstimulated, stimulated). The results directory generated after following the Basic Protocol on this dataset is also saved in the github repository.
The subdirectory fold_changes/ contains three files, namely: deltaRibo.txt, deltaRNA.txt, and deltaTE.txt. These files store gene-wise expression changes across the given conditions in RPF, RNA, and TE, respectively. The results are obtained using DESeq2 and are saved in its standard output format. The two important columns, gene-wise log fold changes and the associated adjusted p -values, are used to determine gene expression changes between the two conditions. Generally, p adj < 0.05 is used as a threshold for determining genes that are changing significantly. A threshold for the absolute log fold change can also be used to select only high-effect sizes. The genes obtained using these thresholds are considered as significantly changing across the given condition or treatment. Genes passing these thresholds in deltaRNA.txt are those with a significant change in RNA and are considered DTGs, and genes passing these thresholds in deltaTE.txt are considered DTEGs.
Furthermore, the combination of changes in RPF, RNA, and TE are used to determine a gene's regulatory class, as shown in Figure 1D. A subdirectory, gene_lists/, contains files that list genes from each regulatory class. These include genes that have been identified as either DTG or DTEG and then further classified into translationally forwarded, buffered, exclusive, or intensified (see details in Table 2). Genes that are classified as forwarded are transcriptionally driven and exhibit no change in TE. On the contrary, translationally exclusive genes exhibit changes in TE but no change in transcription, which implies that these genes are only regulated translationally. Buffered and intensified genes have changes in TE as well as changes in RNA. If these changes in RNA counteract the change in TE, we consider them as translationally buffered, while if RNA changes act with changes TE, we consider them intensified. In each case, these genes are under both transcriptional and translational regulation.
Beyond what is described in these protocols, to understand the potential functions of the different gene regulatory classes, a gene set enrichment analysis (GSEA) or gene ontology (GO) overrepresentation analysis is recommended. Furthermore, hierarchical clustering of the gene-wise fold changes can also be performed to identify subgroups of genes that have a similar regulatory profile.
Lastly, the script generates a file, Results_figures.pdf, which includes three main visualizations of 1 a PCA, (2) global fold changes, and (3) gene-wise fold changes.
The PCA is conducted for both the Ribo-seq and RNA-seq count data. A PCA transforms the data in such a way that each component captures a different source of variation within the data, with the first component (PC1) capturing the largest source of variance in the data. Thus, a PCA can be used to determine any batch effect that is a source of variation in the data. In the example data, it shows that PC1 accounts for 42% of the variance in the Ribo-seq and 46% of the variance in the RNA-seq data. Importantly, PC1 separates the individual patients in both the datasets, indicating that the largest variance in these data is due to the difference between patients in the study. Since these datasets were generated to study the changes in different conditions (unstimulated/stimulated), it is important to remove this patient effect (Fig. 2A, B). Therefore, in this case, the DTEG.R script should be run with the batch effect parameter (Argument 4) set to 1.
The .pdf file also includes a visualization of the global fold changes, as shown in Figure 2C. This is drawn using a scatter plot of the fold changes in RNA and RPFs. The plot also highlights whether the gene is a DTG and/or a DTEG. This plot gives an overview of the overall impact of translational regulation in the system. As such, it can be used to determine the dominant mode of regulation in the dataset and visualize the overall effect sizes of the different regulation types. For instance, if there were very few DTEGs and many DTGs found, it would imply that there is very little translational regulation in the system, and most of the changes occur via transcriptional regulation.
In order to look at individual examples, the file further visualizes the gene-wise fold changes for the genes with the strongest effect in each category (Fig. 2D-G). A line plot is used for visualizing the changes from unstimulated to stimulated in this study. These line plots can be generated for any gene of interest using step 4 in the Basic Protocol or step 11 in the Alternate Protocol.
Time Considerations
The protocol takes a couple of minutes on a standard computer for the example dataset, which includes four samples and two conditions. This could vary based on the number of samples and conditions to be tested.
Acknowledgments
S.S., St.C., and O.J.L.R. conceived and designed the study. So.C. analyzed the data and produced the pipeline with support from J.F.O., O.J.L.R., and S.S. St.C., E.A., O.J.L.R, So.C., and S.S. prepared the manuscript with input from St.C., N.H., J.F.O., and E.A., and with data produced by E.A. and S.V. The research was supported by the National Medical Research Council–Singapore Translational Research investigator awards to St.C. (NMRC/STaR/0029/2017), the National Medical Research Council Central Grant to the National Heart Centre Singapore, Goh Foundation, Tanoto Foundation, National Institute for Health Research Cardiovascular Biomedical Research Unit of Royal Brompton and Harefield NHS Foundation Trust UK, Heart Research UK (RG2657/17/19), and a grant from the Fondation Leducq. S.S. is supported by the Goh Foundation and Charles Toh Cardiovascular Fellowship. O.J.L.R is supported by National Medical Research Council Young Individual Research Grant (NMRC/OFYIRG/0022/2016) and Singapore National Research Foundation Competitive Research Programme (NRF-CRP20-2017-0002).
Literature Cited
- Anders, S., McCarthy, D. J., Chen, Y., Okoniewski, M., Smyth, G. K., Huber, W., & Robinson, M. D. (2013). Count-based differential expression analysis of RNA sequencing data using R and Bioconductor. Nature Protocols , 8(9), 1765–1786. doi: 10.1038/nprot.2013.099.
- Chothani, S., Schäfer, S., Adami, E., Viswanathan, S., Widjaja, A. A., Langley, S. R., … Rackham, O. J. L. (2019). Widespread translational control of fibrosis in the human heart by RNA-binding proteins. Circulation , 140(11), 937–951. doi: 10.1161/circulationaha.119.039596.
- Ingolia, N. T., Brar, G. A., Rouskin, S., McGeachy, A. M., & Weissman, J. S. (2013). Genome-wide annotation and quantitation of translation by ribosome profiling. Current Protocols in Molecular Biology , 103(1), 4.18.1–4.18.19. doi: 10.1002/0471142727.mb0418s103.
- Ingolia, N. T., Ghaemmaghami, S., Newman, J. R. S., & Weissman, J. S. (2009). Genome-wide analysis in vivo of translation with nucleotide resolution using ribosome profiling. Science , 324(5924), 218–223. doi: 10.1126/science.1168978.
- Ji, F., & Sadreyev, R. I. (2018). RNA-seq: Basic bioinformatics analysis. Current Protocols in Molecular Biology , 124, e68. doi: 10.1002/cpmb.68.
- Leek, J. T., Scharpf, R. B., Bravo, H. C., Simcha, D., Langmead, B., Johnson, W. E., … Irizarry, R. A. (2010). Tackling the widespread and critical impact of batch effects in high-throughput data. Nature Reviews Genetics , 11(10), 733–739. doi: 10.1038/nrg2825.
- Li, W., Wang, W., Uren, P. J., Penalva, L. O. F., & Smith, A. D. (2017). Riborex: Fast and flexible identification of differential translation from Ribo-seq data. Bioinformatics , 33(11), 1735–1737. doi: 10.1093/bioinformatics/btx047.
- Love, M. I., Huber, W., & Anders, S. (2014). Moderated estimation of fold change and dispersion for RNA-seq data with DESeq2. Genome Biology , 15(12), 550. doi: 10.1186/s13059-014-0550-8.
- Oertlin, C., Lorent, J., Murie, C., Furic, L., Topisirovic, I., & Larsson, O. (2019). Generally applicable transcriptome-wide analysis of translation using anota2seq. Nucleic Acids Research , 47(12), e70. doi: 10.1093/nar/gkz223.
- Robinson, M. D., McCarthy, D. J., & Smyth, G. K. (2010). edgeR: A Bioconductor package for differential expression analysis of digital gene expression data. Bioinformatics , 26(1), 139–140. doi: 10.1093/bioinformatics/btp616.
- Schafer, S., Adami, E., Heinig, M., Rodrigues, K. E. C., Kreuchwig, F., Silhavy, J., … Hubner, N. (2015). Translational regulation shapes the molecular landscape of complex disease phenotypes. Nature Communications , 6, 7200. doi: 10.1038/ncomms8200.
- Xiao, Z., Zou, Q., Liu, Y., & Yang, X. (2016). Genome-wide assessment of differential translations with ribosome profiling data. Nature Communications , 7, 11194. doi: 10.1038/ncomms11194.
- Xu, G., Greene, G. H., Yoo, H., Liu, L., Marqués, J., Motley, J., & Dong, X. (2017). Global translational reprogramming is a fundamental layer of immune regulation in plants. Nature , 545(7655), 487–490. doi: 10.1038/nature22371.
- Zhong, Y., Karaletsos, T., Drewe, P., Sreedharan, V. T., Kuo, D., Singh, K., … Rätsch, G. (2017). RiboDiff: Detecting changes of mRNA translation efficiency from ribosome footprints. Bioinformatics , 33(1), 139–141. doi: 10.1093/bioinformatics/btw585.
Internet Resources
Github page with scripts for Basic Protocol.
Manual for Trimmomatic.
Manual for bowtie2.
Manual for STAR aligner.
Manual for subread; Refer FeatureCounts.
Manual for RiboTISH.
Citing Literature
Number of times cited according to CrossRef: 63
- Jesslyn Park, Hetvee Desai, José M Liboy-Lugo, Sohyun Gu, Ziad Jowhar, Albert Xu, Stephen N Floor, IGHMBP2 deletion suppresses translation and activates the integrated stress response, Life Science Alliance, 10.26508/lsa.202302554, 7 , 8, (e202302554), (2024).
- Roswitha Dolcemascolo, Raúl Ruiz, Sara Baldanta, Lucas Goiriz, María Heras-Hernández, Roser Montagud-Martínez, Guillermo Rodrigo, Probing the orthogonality and robustness of the mammalian RNA-binding protein Musashi-1 in Escherichia coli, Journal of Biological Engineering, 10.1186/s13036-024-00448-x, 18 , 1, (2024).
- Xingzhuo Yang, Xianguo Zhao, Zhangwu Zhao, Juan Du, Genome-wide analysis reveals transcriptional and translational changes during diapause of the Asian corn borer (Ostrinia furnacalis), BMC Biology, 10.1186/s12915-024-02000-1, 22 , 1, (2024).
- Ruitang Chai, Cong Xiao, Zhuliang Yang, Wenya Du, Ke Lv, Jiayi Zhang, Xiurong Yang, Identification of genes associated with sperm storage capacity in hens at different times after insemination by RNA-seq and Ribo-seq, BMC Genomics, 10.1186/s12864-024-10472-2, 25 , 1, (2024).
- Patrick B. F. O’Connor, Jennifer Mahony, Eoghan Casey, Pavel V. Baranov, Douwe van Sinderen, Martina M. Yordanova, Ribosome profiling reveals downregulation of UMP biosynthesis as the major early response to phage infection, Microbiology Spectrum, 10.1128/spectrum.03989-23, 12 , 4, (2024).
- Arka Banerjee, Meric Ataman, Maciej Jerzy Smialek, Debdatto Mookherjee, Julius Rabl, Aleksei Mironov, Lea Mues, Ludovic Enkler, Mairene Coto-Llerena, Alexander Schmidt, Daniel Boehringer, Salvatore Piscuoglio, Anne Spang, Nitish Mittal, Mihaela Zavolan, Ribosomal protein RPL39L is an efficiency factor in the cotranslational folding of a subset of proteins with alpha helical domains, Nucleic Acids Research, 10.1093/nar/gkae630, 52 , 15, (9028-9048), (2024).
- Nikita A Verheyden, Melina Klostermann, Mirko Brüggemann, Hanna M Steede, Anica Scholz, Shady Amr, Chiara Lichtenthaeler, Christian Münch, Tobias Schmid, Kathi Zarnack, Andreas Krueger, A high-resolution map of functional miR-181 response elements in the thymus reveals the role of coding sequence targeting and an alternative seed match, Nucleic Acids Research, 10.1093/nar/gkae416, 52 , 14, (8515-8533), (2024).
- Tamsin J Samuels, Jinghua Gui, Daniel Gebert, Felipe Karam Teixeira, Two distinct waves of transcriptome and translatome changes drive Drosophila germline stem cell differentiation, The EMBO Journal, 10.1038/s44318-024-00070-z, 43 , 8, (1591-1617), (2024).
- Nitish Mittal, Meric Ataman, Lionel Tintignac, Daniel J. Ham, Lena Jörin, Alexander Schmidt, Michael Sinnreich, Markus A. Ruegg, Mihaela Zavolan, Calorie restriction and rapamycin distinctly restore non-canonical ORF translation in the muscles of aging mice, npj Regenerative Medicine, 10.1038/s41536-024-00369-9, 9 , 1, (2024).
- Muhammad Aammar Tufail, Britta Jordan, Lydia Hadjeras, Rick Gelhausen, Liam Cassidy, Tim Habenicht, Miriam Gutt, Lisa Hellwig, Rolf Backofen, Andreas Tholey, Cynthia M. Sharma, Ruth A. Schmitz, Uncovering the small proteome of Methanosarcina mazei using Ribo-seq and peptidomics under different nitrogen conditions, Nature Communications, 10.1038/s41467-024-53008-8, 15 , 1, (2024).
- Ioanna Tzani, Marina Castro-Rivadeneyra, Paul Kelly, Lisa Strasser, Lin Zhang, Martin Clynes, Barry L. Karger, Niall Barron, Jonathan Bones, Colin Clarke, Detection of host cell microprotein impurities in antibody drug products, Nature Communications, 10.1038/s41467-024-51870-0, 15 , 1, (2024).
- Yudong Fu, Fan Jiang, Xiao Zhang, Yingyi Pan, Rui Xu, Xiu Liang, Xiaofen Wu, Xingqiang Li, Kaixuan Lin, Ruona Shi, Xiaofei Zhang, Dominique Ferrandon, Jing Liu, Duanqing Pei, Jie Wang, Tao Wang, Perturbation of METTL1-mediated tRNA N7- methylguanosine modification induces senescence and aging, Nature Communications, 10.1038/s41467-024-49796-8, 15 , 1, (2024).
- Qiang Zhang, Hanzhe Ye, Cong Liu, Haiwu Zhou, Mingbin He, Xiaodong Liang, Yu Zhou, Kun Wang, Yali Qin, Zhifei Li, Mingzhou Chen, PABP-driven secondary condensed phase within RSV inclusion bodies activates viral mRNAs for ribosomal recruitment, Virologica Sinica, 10.1016/j.virs.2023.12.001, 39 , 2, (235-250), (2024).
- Mingso Sherma Limbu, Tianze Xiong, Sufang Wang, A review of Ribosome profiling and tools used in Ribo-seq data analysis, Computational and Structural Biotechnology Journal, 10.1016/j.csbj.2024.04.051, 23 , (1912-1918), (2024).
- Thorsten Schaefer, Nitish Mittal, Hui Wang, Meric Ataman, Silvia Candido, Jonas Lötscher, Sergiy Velychko, Lionel Tintignac, Thomas Bock, Anastasiya Börsch, Jochen Baßler, Tata Nageswara Rao, Jakub Zmajkovic, Sarah Roffeis, Jordan Löliger, Francis Jacob, Alain Dumlin, Christoph Schürch, Alexander Schmidt, Radek C. Skoda, Matthias P. Wymann, Christoph Hess, Hans R. Schöler, Holm Zaehres, Ed Hurt, Mihaela Zavolan, Claudia Lengerke, Nuclear and cytosolic fractions of SOX2 synergize as transcriptional and translational co-regulators of cell fate, Cell Reports, 10.1016/j.celrep.2024.114807, 43 , 10, (114807), (2024).
- K. Shanmugha Rajan, Saurav Aryal, Disha-Gajanan Hiregange, Anat Bashan, Hava Madmoni, Mika Olami, Tirza Doniger, Smadar Cohen-Chalamish, Pascal Pescher, Masato Taoka, Yuko Nobe, Aliza Fedorenko, Tanaya Bose, Ella Zimermann, Eric Prina, Noa Aharon-Hefetz, Yitzhak Pilpel, Toshiaki Isobe, Ron Unger, Gerald F. Späth, Ada Yonath, Shulamit Michaeli, Structural and mechanistic insights into the function of Leishmania ribosome lacking a single pseudouridine modification, Cell Reports, 10.1016/j.celrep.2024.114203, 43 , 5, (114203), (2024).
- Archana Bairavasundaram Prusty, Anja Hirmer, Julieth Andrea Sierra-Delgado, Hannes Huber, Ulf-Peter Guenther, Andreas Schlosser, Olexandr Dybkov, Ezgi Yildirim, Henning Urlaub, Kathrin C. Meyer, Sibylle Jablonka, Florian Erhard, Utz Fischer, RNA helicase IGHMBP2 regulates THO complex to ensure cellular mRNA homeostasis, Cell Reports, 10.1016/j.celrep.2024.113802, 43 , 2, (113802), (2024).
- Mehdi Amiri, Stephen J. Kiniry, Anthony P. Possemato, Niaz Mahmood, Tayebeh Basiri, Catherine R. Dufour, Negar Tabatabaei, Qiyun Deng, Michael A. Bellucci, Keerthana Harwalkar, Matthew P. Stokes, Vincent Giguère, Randal J. Kaufman, Yojiro Yamanaka, Pavel V. Baranov, Soroush Tahmasebi, Nahum Sonenberg, Impact of eIF2α phosphorylation on the translational landscape of mouse embryonic stem cells, Cell Reports, 10.1016/j.celrep.2023.113615, 43 , 1, (113615), (2024).
- Maximilian P. Kohl, Béatrice Chane-Woon-Ming, Roberto Bahena-Ceron, Jose Jaramillo-Ponce, Laura Antoine, Lucas Herrgott, Pascale Romby, Stefano Marzi, Ribosome Profiling Methods Adapted to the Study of RNA-Dependent Translation Regulation in Staphylococcus aureus, Bacterial Regulatory RNA, 10.1007/978-1-0716-3565-0_5, (73-100), (2024).
- Xiao Zhang, Shihao Hong, Chengying Yu, Xiaozhong Shen, Fangying Sun, Jianhua Yang, Comparative analysis between high-grade serous ovarian cancer and healthy ovarian tissues using single-cell RNA sequencing, Frontiers in Oncology, 10.3389/fonc.2023.1148628, 13 , (2023).
- Anna K. Goldkamp, Darren E. Hagen, Implications of tRNA abundance on translation elongation across bovine tissues, Frontiers in Genetics, 10.3389/fgene.2023.1308048, 14 , (2023).
- Kilian Schumacher, Rick Gelhausen, Willow Kion-Crosby, Lars Barquist, Rolf Backofen, Kirsten Jung, Ribosome profiling reveals the fine-tuned response of Escherichia coli to mild and severe acid stress , mSystems, 10.1128/msystems.01037-23, 8 , 6, (2023).
- Yulia Gonskikh, Julian Stoute, Hui Shen, Krista Budinich, Bianca Pingul, Kollin Schultz, Heidi Elashal, Ronen Marmorstein, Junwei Shi, Kathy Fange Liu, Noncatalytic regulation of 18 S rRNA methyltransferase DIMT1 in acute myeloid leukemia , Genes & Development, 10.1101/gad.350298.122, 37 , 7-8, (321-335), (2023).
- Hirotatsu Imai, Daisuke Utsumi, Hidetsugu Torihara, Kenzo Takahashi, Hidehito Kuroyanagi, Akio Yamashita, Simultaneous measurement of nascent transcriptome and translatome using 4-thiouridine metabolic RNA labeling and translating ribosome affinity purification, Nucleic Acids Research, 10.1093/nar/gkad545, 51 , 14, (e76-e76), (2023).
- Shir Mandelboum, Melisa Herrero, Andrea Atzmon, Marcelo Ehrlich, Orna Elroy-Stein, Effective extraction of polyribosomes exposes gene expression strategies in primary astrocytes, Nucleic Acids Research, 10.1093/nar/gkad131, 51 , 7, (3375-3390), (2023).
- Ivan Milenkovic, Helaine Graziele Santos Vieira, Morghan C Lucas, Jorge Ruiz-Orera, Giannino Patone, Scott Kesteven, Jianxin Wu, Michael Feneley, Guadalupe Espadas, Eduard Sabidó, Norbert Hübner, Sebastiaan van Heesch, Mirko Völkers, Eva Maria Novoa, Dynamic interplay between RPL3- and RPL3L-containing ribosomes modulates mitochondrial activity in the mammalian heart, Nucleic Acids Research, 10.1093/nar/gkad121, 51 , 11, (5301-5324), (2023).
- Sonia Chothani, Lena Ho, Sebastian Schafer, Owen Rackham, Discovering microproteins: making the most of ribosome profiling data, RNA Biology, 10.1080/15476286.2023.2279845, 20 , 1, (943-954), (2023).
- Yifang Xie, Qiaozi Wang, Yuchen Yang, David Near, Haofei Wang, Marazzano Colon, Christopher Nguyen, Conor Slattery, Benjamin Keepers, Gregory Farber, Tzu-Wen Wang, Sung-Ho Lee, Yen-Yu Ian Shih, Jiandong Liu, Li Qian, Translational landscape of direct cardiac reprogramming reveals a role of Ybx1 in repressing cardiac fate acquisition, Nature Cardiovascular Research, 10.1038/s44161-023-00344-5, 2 , 11, (1060-1077), (2023).
- Lucas Ferguson, Heather E. Upton, Sydney C. Pimentel, Amanda Mok, Liana F. Lareau, Kathleen Collins, Nicholas T. Ingolia, Streamlined and sensitive mono- and di-ribosome profiling in yeast and human cells, Nature Methods, 10.1038/s41592-023-02028-1, 20 , 11, (1704-1715), (2023).
- Yezi Xiang, Wenze Huang, Lianmei Tan, Tianyuan Chen, Yang He, Patrick S. Irving, Kevin M. Weeks, Qiangfeng Cliff Zhang, Xinnian Dong, Pervasive downstream RNA hairpins dynamically dictate start-codon selection, Nature, 10.1038/s41586-023-06500-y, 621 , 7978, (423-430), (2023).
- Toman Borteçen, Torsten Müller, Jeroen Krijgsveld, An integrated workflow for quantitative analysis of the newly synthesized proteome, Nature Communications, 10.1038/s41467-023-43919-3, 14 , 1, (2023).
- Yi-Hua Huang, Jia-Qi Han, Biao Ma, Wu-Qiang Cao, Xin-Kai Li, Qing Xiong, He Zhao, Rui Zhao, Xun Zhang, Yang Zhou, Wei Wei, Jian-Jun Tao, Wan-Ke Zhang, Wenfeng Qian, Shou-Yi Chen, Chao Yang, Cui-Cui Yin, Jin-Song Zhang, A translational regulator MHZ9 modulates ethylene signaling in rice, Nature Communications, 10.1038/s41467-023-40429-0, 14 , 1, (2023).
- Xi-Tong Zhu, Run Zhou, Jian Che, Yu-Yu Zheng, Muhammad Tahir ul Qamar, Jia-Wu Feng, Jianwei Zhang, Junxiang Gao, Ling-Ling Chen, Ribosome profiling reveals the translational landscape and allele-specific translational efficiency in rice, Plant Communications, 10.1016/j.xplc.2022.100457, 4 , 2, (100457), (2023).
- Angela Re, Translational efficiency in gas-fermenting bacteria: Adding a new layer of regulation to gene expression in acetogens, iScience, 10.1016/j.isci.2023.108383, 26 , 12, (108383), (2023).
- John E. Froberg, Omer Durak, Jeffrey D. Macklis, Development of nanoRibo-seq enables study of regulated translation by cortical neuron subtypes, showing uORF translation in synaptic-axonal genes, Cell Reports, 10.1016/j.celrep.2023.112995, 42 , 9, (112995), (2023).
- Amy E. Campbell, Michael C. Dyle, Roberto Albanese, Tyler Matheny, Kavitha Sudheendran, Michael A. Cortázar, Thomas Forman, Rui Fu, Austin E. Gillen, Marvin H. Caruthers, Stephen N. Floor, Lorenzo Calviello, Sujatha Jagannathan, Compromised nonsense-mediated RNA decay results in truncated RNA-binding protein production upon DUX4 expression, Cell Reports, 10.1016/j.celrep.2023.112642, 42 , 6, (112642), (2023).
- Weiqian Li, Yue Huo, Yue Ren, Chenxi Han, Shuo Li, Kangning Wang, Manman He, Yiying Chen, Yanran Wang, Lingjie Xu, Yuehong Guo, Yanmin Si, Yufeng Gao, Jiayue Xu, Xiaoshuang Wang, Yanni Ma, Jia Yu, Fang Wang, Deciphering the Functional Long Non‐Coding RNAs Derived from MicroRNA Loci, Advanced Science, 10.1002/advs.202203987, 10 , 33, (2023).
- Atefeh Bagheri, Artem Astafev, Tara Al-Hashimy, Peng Jiang, Tracing Translational Footprint by Ribo-Seq: Principle, Workflow, and Applications to Understand the Mechanism of Human Diseases, Cells, 10.3390/cells11192966, 11 , 19, (2966), (2022).
- Katerina Douka, Michaela Agapiou, Isabel Birds, Julie L. Aspden, Optimization of Ribosome Footprinting Conditions for Ribo-Seq in Human and Drosophila melanogaster Tissue Culture Cells, Frontiers in Molecular Biosciences, 10.3389/fmolb.2021.791455, 8 , (2022).
- Chunxia Zhang, Meng Wang, Yisi Li, Yi Zhang, Profiling and functional characterization of maternal mRNA translation during mouse maternal-to-zygotic transition, Science Advances, 10.1126/sciadv.abj3967, 8 , 5, (2022).
- Naoyuki Sotta, Yukako Chiba, Haruka Aoyama, Seidai Takamatsu, Takamasa Suzuki, Kyoko Miwa, Yui Yamashita, Satoshi Naito, Toru Fujiwara, Translational Landscape of a C4 Plant, Sorghum bicolor , Under Normal and Sulfur-Deficient Conditions , Plant and Cell Physiology, 10.1093/pcp/pcac023, 63 , 5, (592-604), (2022).
- Emeline I J Lelong, Gabriel Khelifi, Pauline Adjibade, France-Hélène Joncas, Valérie Grenier St-Sauveur, Virginie Paquette, Typhaine Gris, Amina Zoubeidi, Etienne Audet-Walsh, Jean-Philippe Lambert, Paul Toren, Rachid Mazroui, Samer M I Hussein, Prostate cancer resistance leads to a global deregulation of translation factors and unconventional translation, NAR Cancer, 10.1093/narcan/zcac034, 4 , 4, (2022).
- Caia Deborah Suzanne Duncan, Juan Mata, Translation-complex profiling of fission yeast cells reveals dynamic rearrangements of scanning ribosomal subunits upon nutritional stress, Nucleic Acids Research, 10.1093/nar/gkac1140, 50 , 22, (13011-13025), (2022).
- Shihao Hong, Xiaozhong Shen, Chunhai Luo, Fei Sun, Comparative analysis of the testes from wild-type and Alkbh5 -knockout mice using single-cell RNA sequencing , G3 Genes|Genomes|Genetics, 10.1093/g3journal/jkac130, 12 , 8, (2022).
- Baptiste Kerouanton, Sebastian Schäfer, Lena Ho, Sonia Chothani, Owen J L Rackham, CLIPreg: constructing translational regulatory networks from CLIP-, Ribo- and RNA-seq, Bioinformatics, 10.1093/bioinformatics/btac363, 38 , 14, (3651-3653), (2022).
- Erin E. Duffy, Benjamin Finander, GiHun Choi, Ava C. Carter, Iva Pritisanac, Aqsa Alam, Victor Luria, Amir Karger, William Phu, Maxwell A. Sherman, Elena G. Assad, Naomi Pajarillo, Alexandra Khitun, Elizabeth E. Crouch, Sanika Ganesh, Jin Chen, Bonnie Berger, Nenad Sestan, Anne O’Donnell-Luria, Eric J. Huang, Eric C. Griffith, Julie D. Forman-Kay, Alan M. Moses, Brian T. Kalish, Michael E. Greenberg, Developmental dynamics of RNA translation in the human brain, Nature Neuroscience, 10.1038/s41593-022-01164-9, 25 , 10, (1353-1365), (2022).
- Zhipeng Li, Lucas Ferguson, Kirandeep K. Deol, Melissa A. Roberts, Leslie Magtanong, Joseph M. Hendricks, Gergey Alzaem Mousa, Seda Kilinc, Kaitlin Schaefer, James A. Wells, Michael C. Bassik, Andrei Goga, Scott J. Dixon, Nicholas T. Ingolia, James A. Olzmann, Ribosome stalling during selenoprotein translation exposes a ferroptosis vulnerability, Nature Chemical Biology, 10.1038/s41589-022-01033-3, 18 , 7, (751-761), (2022).
- Ulrike Zinnall, Miha Milek, Igor Minia, Carlos H. Vieira-Vieira, Simon Müller, Guido Mastrobuoni, Orsalia-Georgia Hazapis, Simone Del Giudice, David Schwefel, Nadine Bley, Franka Voigt, Jeffrey A. Chao, Stefan Kempa, Stefan Hüttelmaier, Matthias Selbach, Markus Landthaler, HDLBP binds ER-targeted mRNAs by multivalent interactions to promote protein synthesis of transmembrane and secreted proteins, Nature Communications, 10.1038/s41467-022-30322-7, 13 , 1, (2022).
- K. Pimkova, M. Jassinskaja, R. Munita, M. Ciesla, N. Guzzi, P. Cao Thi Ngoc, M. Vajrychova, E. Johansson, C. Bellodi, J. Hansson, Quantitative analysis of redox proteome reveals oxidation-sensitive protein thiols acting in fundamental processes of developmental hematopoiesis, Redox Biology, 10.1016/j.redox.2022.102343, 53 , (102343), (2022).
- Sonia P. Chothani, Eleonora Adami, Anissa A. Widjaja, Sarah R. Langley, Sivakumar Viswanathan, Chee Jian Pua, Nevin Tham Zhihao, Nathan Harmston, Giuseppe D’Agostino, Nicola Whiffin, Wang Mao, John F. Ouyang, Wei Wen Lim, Shiqi Lim, Cheryl Q.E. Lee, Alexandra Grubman, Joseph Chen, J.P. Kovalik, Karl Tryggvason, Jose M. Polo, Lena Ho, Stuart A. Cook, Owen J.L. Rackham, Sebastian Schafer, A high-resolution map of human RNA translation, Molecular Cell, 10.1016/j.molcel.2022.06.023, 82 , 15, (2885-2899.e8), (2022).
- Shitao Chen, Geng An, Hanshu Wang, Xiaolong Wu, Ping Ping, Longfei Hu, Yunmei Chen, Jue Fan, C. Yan Cheng, Fei Sun, Human obstructive (postvasectomy) and nonobstructive azoospermia – Insights from scRNA-Seq and transcriptome analysis, Genes & Diseases, 10.1016/j.gendis.2020.09.004, 9 , 3, (766-776), (2022).
- Suhail A. Ansari, Widad Dantoft, Jorge Ruiz-Orera, Afzal P. Syed, Susanne Blachut, Sebastiaan van Heesch, Norbert Hübner, Nina Henriette Uhlenhaut, Integrative analysis of macrophage ribo-Seq and RNA-Seq data define glucocorticoid receptor regulated inflammatory response genes into distinct regulatory classes, Computational and Structural Biotechnology Journal, 10.1016/j.csbj.2022.09.042, 20 , (5622-5638), (2022).
- Danielle Minichino, Kaosheng Lv, Niansheng Chu, Wei Tong, Edward M Behrens, BRAF‐V600E utilizes posttranscriptional mechanisms to amplify LPS‐induced TNFα production in dendritic cells in a mouse model of Langerhans cell histiocytosis, Journal of Leukocyte Biology, 10.1002/JLB.3A0122-075RR, 112 , 5, (1089-1104), (2022).
- Håkon Tjeldnes, Kornel Labun, Yamila Torres Cleuren, Katarzyna Chyżyńska, Michał Świrski, Eivind Valen, ORFik: a comprehensive R toolkit for the analysis of translation, BMC Bioinformatics, 10.1186/s12859-021-04254-w, 22 , 1, (2021).
- Ameya Sinha, Sebastian Baumgarten, Amy Distiller, Emma McHugh, Patty Chen, Meetali Singh, Jessica M. Bryant, Jiaqi Liang, Germano Cecere, Peter C. Dedon, Peter R. Preiser, Stuart A. Ralph, Artur Scherf, Functional Characterization of the m 6 A-Dependent Translational Modulator PfYTH.2 in the Human Malaria Parasite , mBio, 10.1128/mBio.00661-21, 12 , 2, (2021).
- Dimitris C. Kanellis, Jaime A. Espinoza, Asimina Zisi, Elpidoforos Sakkas, Jirina Bartkova, Anna-Maria Katsori, Johan Boström, Lars Dyrskjøt, Helle Broholm, Mikael Altun, Simon J. Elsässer, Mikael S. Lindström, Jiri Bartek, The exon-junction complex helicase eIF4A3 controls cell fate via coordinated regulation of ribosome biogenesis and translational output, Science Advances, 10.1126/sciadv.abf7561, 7 , 32, (2021).
- Kaining Chen, Congying Chen, Huihui Li, Jiaqi Yang, Mengqing Xiang, Hongwei Wang, Zhi Xie, Widespread translational control regulates retinal development in mouse, Nucleic Acids Research, 10.1093/nar/gkab749, 49 , 17, (9648-9664), (2021).
- Lorenzo Calviello, Srivats Venkataramanan, Karol J Rogowski, Emanuel Wyler, Kevin Wilkins, Malvika Tejura, Bao Thai, Jacek Krol, Witold Filipowicz, Markus Landthaler, Stephen N Floor, DDX3 depletion represses translation of mRNAs with complex 5′ UTRs, Nucleic Acids Research, 10.1093/nar/gkab287, 49 , 9, (5336-5350), (2021).
- Agnes Karasik, Grant D Jones, Andrew V DePass, Nicholas R Guydosh, Activation of the antiviral factor RNase L triggers translation of non-coding mRNA sequences, Nucleic Acids Research, 10.1093/nar/gkab036, 49 , 11, (6007-6026), (2021).
- Nikolay E. Shirokikh, Translation complex stabilization on messenger RNA and footprint profiling to study the RNA responses and dynamics of protein biosynthesis in the cells, Critical Reviews in Biochemistry and Molecular Biology, 10.1080/10409238.2021.2006599, 57 , 3, (261-304), (2021).
- Caspar Glock, Anne Biever, Georgi Tushev, Belquis Nassim-Assir, Allison Kao, Ina Bartnik, Susanne tom Dieck, Erin M. Schuman, The translatome of neuronal cell bodies, dendrites, and axons, Proceedings of the National Academy of Sciences, 10.1073/pnas.2113929118, 118 , 43, (2021).
- Tamara Fernández-Calero, Marcos Davyt, Karen Perelmuter, Cora Chalar, Giovana Bampi, Helena Persson, Juan Pablo Tosar, Völundur Hafstað, Hugo Naya, Carlos Rovira, Mariela Bollati-Fogolín, Ricardo Ehrlich, Gilles Flouriot, Zoya Ignatova, Mónica Marín, Fine-tuning the metabolic rewiring and adaptation of translational machinery during an epithelial-mesenchymal transition in breast cancer cells, Cancer & Metabolism, 10.1186/s40170-020-00216-7, 8 , 1, (2020).
- Kyle A. Cottrell, Ryan C. Chiou, Jason D. Weber, Upregulation of 5′-terminal oligopyrimidine mRNA translation upon loss of the ARF tumor suppressor, Scientific Reports, 10.1038/s41598-020-79379-8, 10 , 1, (2020).