Perspectives on the Drosophila melanogaster Model for Advances in Toxicological Science
Matthew D. Rand, Matthew D. Rand, Jason M. Tennessen, Jason M. Tennessen, Trudy F. C. Mackay, Trudy F. C. Mackay, Robert R. H. Anholt, Robert R. H. Anholt
Abstract
The use of Drosophila melanogaster for studies of toxicology has grown considerably in the last decade. The Drosophila model has long been appreciated as a versatile and powerful model for developmental biology and genetics because of its ease of handling, short life cycle, low cost of maintenance, molecular genetic accessibility, and availability of a wide range of publicly available strains and data resources. These features, together with recent unique developments in genomics and metabolomics, make the fly model especially relevant and timely for the development of new approach methodologies and movements toward precision toxicology. Here, we offer a perspective on how flies can be leveraged to identify risk factors relevant to environmental exposures and human health. First, we review and discuss fundamental toxicologic principles for experimental design with Drosophila. Next, we describe quantitative and systems genetics approaches to resolve the genetic architecture and candidate pathways controlling susceptibility to toxicants. Finally, we summarize the current state and future promise of the emerging field of Drosophila metabolomics for elaborating toxic mechanisms. © 2023 The Authors. Current Protocols published by Wiley Periodicals LLC.
INTRODUCTION
The multitude of toxic chemicals in our environment, such as metals (e.g., mercury, arsenic, and lead) and natural and synthetic organics (e.g., plant, fungal, and microbial toxicants and polyfluoroalkyl substances) continues to drive the need to develop new approaches and methodologies for obtaining a deeper knowledge of toxicity mechanisms. The Drosophila model has long been appreciated as a versatile and powerful model because of its ease of handling, low costs of maintenance in the laboratory, molecular genetic accessibility with availability of a wide range of publicly available resources, and short life cycle. The broad conservation of genes encoding fundamental developmental and physiologic pathways between Drosophila and mammals supports the translatability of study results from flies to people. Conventional forward genetic approaches are essential for revealing toxicity targets via unbiased screening methods, whereas reverse genetic approaches are highly effective for dissecting and validating potential adverse outcome pathways underlying toxic actions of agents of interest. Advances in deep sequencing technologies and high-resolution mass spectrometry in the last decade have propelled an unprecedented expansion in -omics capabilities, particularly relevant to understanding genetic regulation (genomics and transcriptomics) and metabolic and physiologic function (metabolomics) when applied to the fly.
Here, we revisit fundamental aspects of toxicology and how they apply when constructing experimental study designs in the fly model. We then offer examples of advanced approaches using genomics and metabolomics that are pushing the capabilities of the model forward, expanding the utility of the fly into the arena of new approach methodologies.
DROSOPHILA USE IN TOXICOLOGY
Use of the Drosophila model for toxicology studies has expanded immensely in the past decade (Abolaji et al., 2013; Chifiriuc et al., 2016; Demir, 2020; Rand, 2010, 2019; Tiwari et al., 2011). Toxicology is the science of poisons. A poison is any agent capable of producing a deleterious effect on a biological system (Klaassen, 2013). Yet, even beneficial nutrients can elicit toxicity when experienced in excess, and conversely, several toxicants elicit marginal to significant health or performance benefits at very low doses. Accordingly, much of toxicology is concerned with understanding the dose-response relationship. Deleterious effects can also arise from environmental stressors, such as temperature or physical demands. Thus, toxicology today is taking on new dimensions focused on understanding how a substance or environmental stressor, independently or in combination, will perturb a biological system.
THE DROSOPHILA MODEL SUPPORTS MECHANISTIC AND DESCRIPTIVE TOXICOLOGY STUDIES
Three fundamental areas of toxicology are: (1) mechanistic toxicology , which concerns cellular, molecular, and biochemical characteristics; (2) descriptive toxicology , which concerns toxicity testing for safety and risk assessment; and (3) regulatory toxicology , which encompasses the decision-making process to create advisories and policy that nonetheless rely on mechanistic and descriptive toxicity evidence (Klaassen, 2013). The Drosophila model best supports mechanistic and, to a limited extent, descriptive toxicology studies. Mechanistic and descriptive toxicity data overlap in that toxic phenotypes will inform hypotheses regarding underlying mechanisms. For example, molecular approaches, particularly the -omics (genomics, transcriptomics, metabolomics, proteomics), yield data that are essentially descriptive while also being informative for elucidating mechanisms. The application of flies to regulatory toxicology for human and mammalian health is limited. Yet the Drosophila model is naturally aligned with the sub-disciplines of environmental toxicology and ecotoxicology. The ability to investigate gene-by-environment interactions in controlled genetic backgrounds and to perform selection experiments with a population in a standardized laboratory condition is what makes the Drosophila model especially robust. Here, population genetics can be leveraged to elucidate toxicity mechanisms. Quantitative and systems genetics approaches to identifying risk factors for toxic exposure are discussed below (Quantitative and Systems Genetics Approaches to Identifying Risk Factors for Toxic Exposure).
Mechanistic toxicology seeks to understand how toxins affect biological systems at multiple levels from molecular to organismal. Three aspects of toxicology to understand mechanism are: (1) characteristics of the toxicity effect (e.g., are the effects immediate or delayed, reversible or irreversible, or acting locally or systemically?); (2) characteristics of the exposure (e.g., what is the nature of the dose-response relationship? Is it monotonic or non-monotonic [hormetic]?); and (3) variation in response (e.g., what are the underlying genetics and metabolic activities explaining individual resistant, susceptible or idiosyncratic responses?). Toxicology encompasses several sub-disciplines defined by the organ system (e.g., neurotoxicology, reproductive toxicology, and immunotoxicology). The fly model, with its long history of discovering fundamental principles of genetics and developmental biology—including tissue morphogenesis, complex behaviors and human disease modeling—presents a comprehensive system for studies on mechanistic toxicology at multiple levels.
TOXICOLOGY PRINCIPLES AS THEY APPLY TO STUDIES IN THE FLY
The effects of toxicants are categorized in terms of absorption, distribution, metabolism, and elimination (ADME). Each of these processes has an associated rate, which can be quantified in terms of toxicokinetics. The toxic effect is dependent upon the interaction of the toxicant and the target molecule (Klaassen, 2013). For instance, a target of an enzyme in the mitochondrial electron transport chain versus an ion channel for nerve conduction or a structural microtubule component necessary for mitotic events will elicit starkly different effects on the cell and the system. The efficacy of the interaction in disrupting the target's function, together with the affinity of the interaction, ultimately comprises the toxicodynamic characteristics of the compound. Aspects of ADME as they apply to studies in Drosophila are discussed below.
Administration and Distribution
It is critical to consider the means of introducing the toxicant into the fly, as well as the biological factors that regulate its entry and distribution (Fig. 1), which can vary widely across developmental stages. The embryo and pupal stages pose an impediment to administration with their respective cuticular protective coatings (the eggshell and pupal case, respectively). The introduction of toxicants to the embryo can be achieved by maternal feeding, micropipette injection, or soaking (Ghaemi et al., 2017; Rand et al., 2008, 2009, 2010), whereas injection is the only practical way to introduce water soluble chemicals into the pupa. With these approaches there are difficulties with attaining consistent dosages, both in terms of amounts being delivered and the subsequent distribution of the compound to peripheral organs and tissues. Maternal feeding can yield variable levels of compound being transmitted to the embryo due to the circadian nature of egg laying and the numerous eggs per female that are laid. Soaking the embryo, even with the assistance of a permeabilization reagent, also yields variable introduction and distribution of small molecules, which requires an additional step of sorting equivalently dosed flies (Rand, 2014; Rand et al., 2010). Injection, on the other hand, produces a more reliable level of dosing, yet can elicit a gradient of effects from the injection site (Ghaemi et al., 2017).
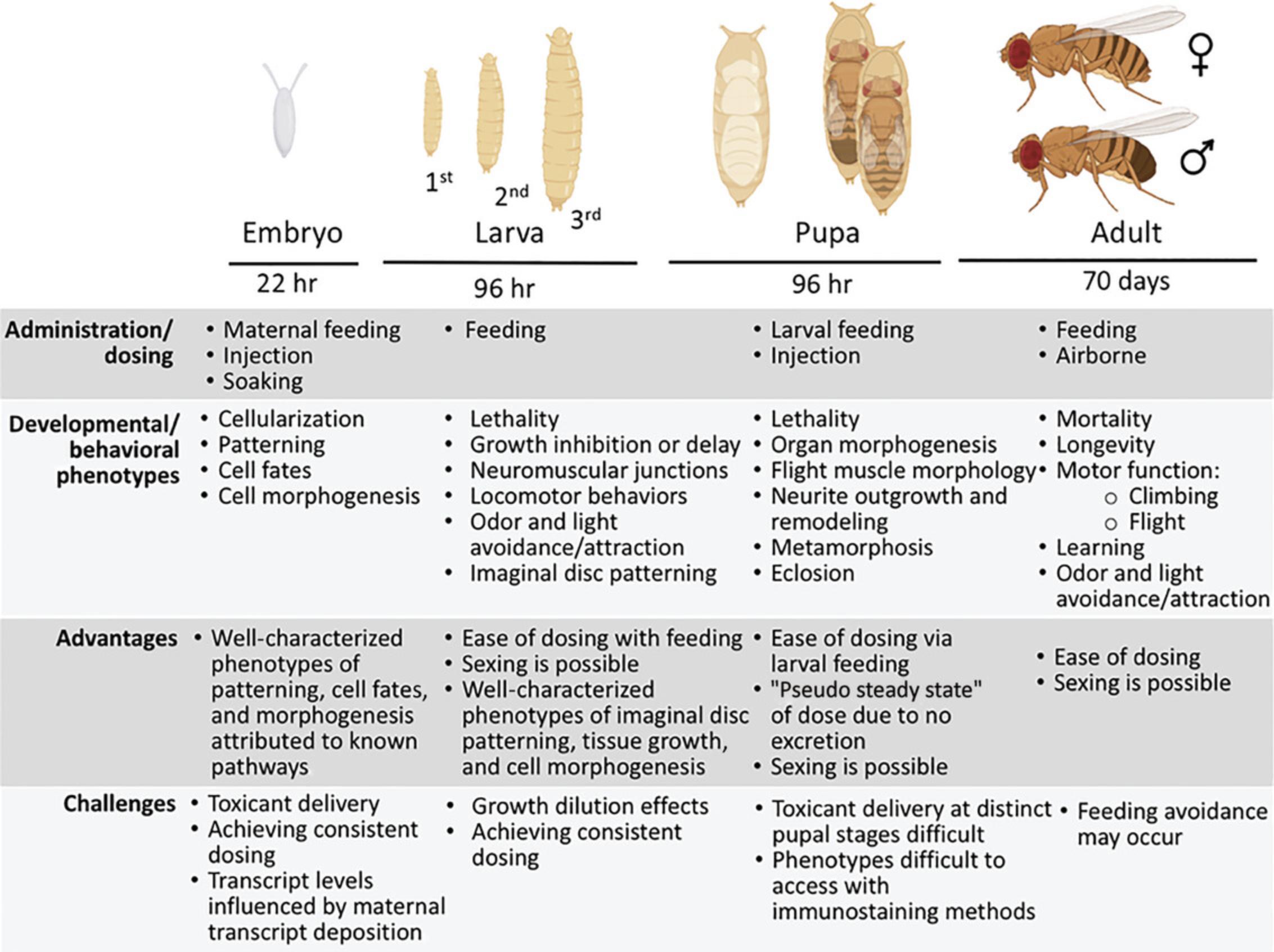
Administration via the feeding of larvae and adults is a preferred method in toxicologic assay design. Larval and adult feeding are convenient since compound concentrations in the food can be carefully controlled. Yet, flies are prone to variability in feeding behavior (Garlapow et al., 2017). Importantly, larvae and adult flies can exhibit long periods of feeding avoidance, for example, to metal ions in the food (Xiao et al., 2022). However, adding dye to the food can ensure selection of flies with nearly equivalent exposures based on coloration of the gut (Shell et al., 2021). In adults, a period of starvation (e.g., overnight without food) before dosing can prompt a more consistent feeding behavior on food with toxic agents. Furthermore, methods of measuring food intake, such as the capillary feeder assay (Diegelmann et al., 2017; Ja et al., 2007) in adult flies, have been optimized to quantitatively account for food uptake.
Administration of volatile toxicants has technical challenges, yet has been executed in a number of studies with definitive findings for the effects and gene candidates mediating ethanol and benzene toxicity and other volatile organics (Guarnieri & Heberlein, 2003; Inamdar et al., 2014; Li et al., 2022; Morozova et al., 2015; Sass et al., 2020). This mode of delivery is feasible and easier to implement for airborne delivery of toxicants in Drosophila than it is for other model organisms (e.g., Caenorhabditis elegans and zebrafish).
Another potentially confounding event for toxicant distribution is growth dilution at the larval stage. Larvae increase in size more than 20-fold over the three larval instars. Growth dilution of the toxicant must be considered in instances where exposures are restricted to the first and second instars (Rand et al., 2019). For example, a presumed toxic dose administered only within the first instar stage can be reduced to innocuous levels in the fly once the third instar is reached. These effects can be seen where toxic levels of methylmercury exposure introduced to first and second larval instars show no downstream effect on eclosion due to growth dilution at the third instar stage (Rand et al., 2019). Exposure via feeding across all larval stages is a practical and more common practice. Here, it is important to recognize that an internal steady state of the toxicant can be reached in the third instar larvae, which will be retained in the fly through pupal stages (Rand et al., 2019).
Metabolism
Flies are endowed with an extensive array of genes encoding xenobiotic metabolizing enzymes (Misra et al., 2011). Flies carry highly conserved members of phase I (e.g., cytochrome p450 enzymes [CYPs]; Nelson et al., 1996), phase II (e.g., glutathione-S-transferases [GSTs]; UDP-glucoronyl transferases [UGTs] Mackenzie et al., 1997; Tu & Akgul, 2005), and phase III (e.g., ABC ATP-dependent transporters; Dermauw & Van Leeuwen, 2014) enzymes. Studies of these families of enzymes are numerous and have been reviewed elsewhere (Daborn et al., 2007; Le Goff et al., 2006; Willoughby et al., 2006). The knowledge base of these enzymes and transporters has benefitted greatly from the study of insecticides (Willoughby et al., 2006). An important lesson from these studies, and comparative studies with mammals and humans, is that conservation of enzyme activity across species does not always follow sequence homology. In addition, the classification of large enzyme families based on sequence homology (e.g., CYP enzymes) has revealed considerable divergence between fly and mouse and human, such that explicit homologs cannot be identified with certainty in large multigene families that have undergone repeated gene duplication with subfunctionalization and neofunctionalization over the course of evolution. Thus, for translational inferences from mechanistic studies where the presumed toxic activity requires metabolic biotransformation of the agent, it is critical to document that the appropriate bioactivating or bioinactivating step(s) are occurring, especially in instances where the enzyme(s) responsible shows low homology between flies and mammals.
Despite the divergence of xenobiotic metabolizing enzymes, flies have proven highly effective for resolving metabolic mechanisms of toxicity with "humanized" modeling. A salient example is an analysis of human GSTP1 (hGSTP1) polymorphisms for their protective activity toward methylmercury toxicity. Transgene ectopic expression of wild-type and two single-nucleotide polymorphism (SNP) mutant hGSTP1 isoforms (I105V, A114V) revealed that the mutants failed to rescue methylmercury inhibition of eclosion behavior with the same efficacy as the wild-type hGSTP1 (Vorojeikina et al., 2017). Interestingly, this outcome occurred without a significant change in methylmercury body burden. Hence, the mechanism of resistance conferred by GSTP1 can be inferred to be one of toxicodynamics rather than toxicokinetics. This distinction is fundamental for guiding hypotheses for more in depth mechanistic studies, as we describe in more detail below.
Elimination
The internal dose, or body burden, is an important consideration for interpreting mechanism. Variation in internal dose between individuals can be a consequence of differences in the bioavailability, which is influenced primarily by absorption (e.g., uptake by the gut). Yet, toxicokinetic principles dictate that the amount of toxicant retained in the body after intermittent or continuous dosing is predominantly influenced by the rate of elimination (e.g., half-life [t1/2]). Thus, the body burden itself can be considered a toxicologic endpoint, reflective of innate differences in the kinetics of absorption and elimination. Elimination rates of compounds are rarely investigated in fly toxicology studies. However, variation in elimination rate may explain resistance traits, as is seen between the Canton S (CS) strain and the more mercury-resistant Hikone R strain (Rand et al., 2019).
RESOLVING MECHANISMS OF TOXICITY: KINETICS VERSUS DYNAMICS
A first step in mapping a toxic mechanism is establishing a dose-dependent effect on an interpretable and quantifiable endpoint. Next, accounting for the relationship of external dose and internal dose (body burden) will distinguish where fundamental mechanisms of resistance and susceptibility are operating. This can be illustrated with an example of various fly strains that exhibit different resistance to methylmercury toxicity during development (Fig. 2). Larval feeding of methylmercury results in dose-dependent inhibition of eclosion behavior (Magnusson & Ramel, 1986; Mahapatra et al., 2010), which has been used to characterize quantitative genetic variation in methylmercury resistance and susceptibility (Montgomery et al., 2014). A wide variation in dose response can be seen between five representative strains of flies that exhibit a range of resistance (Res) and susceptibility (Sus) to methylmercury exposure (compare CS to Res20.3 to Res20.1, Fig. 2A,C). However, comparison of eclosion to mercury body burden (internal dose) reveals that Sus0.2, Res20.3, and CS all show similar inhibition profiles with respect to internal dose (Fig. 2B). This demonstrates that these strains vary in their uptake and/or elimination of methylmercury; hence the variation in their resistance is a toxicokinetic trait. By contrast, the Res20.1 strain shows a much greater eclosion rate with higher levels of mercury internally compared with the Sus0.1 strain (Fig. 2D). This profile reveals that resistance in the Res20.1 strain is consistent with a toxicodynamic trait where methylmercury has less efficacy on internal targets. It can be hypothesized that the difference between CS, Sus0.2, and Res20.3 resistance may track to genetic variation in transporters in the gut, whereas the Res20.1 strain resistance is more likely to have a genetic variant in, for example, a mitochondrial enzyme that exhibits lower affinity for methylmercury thiol modification and averts inhibition.
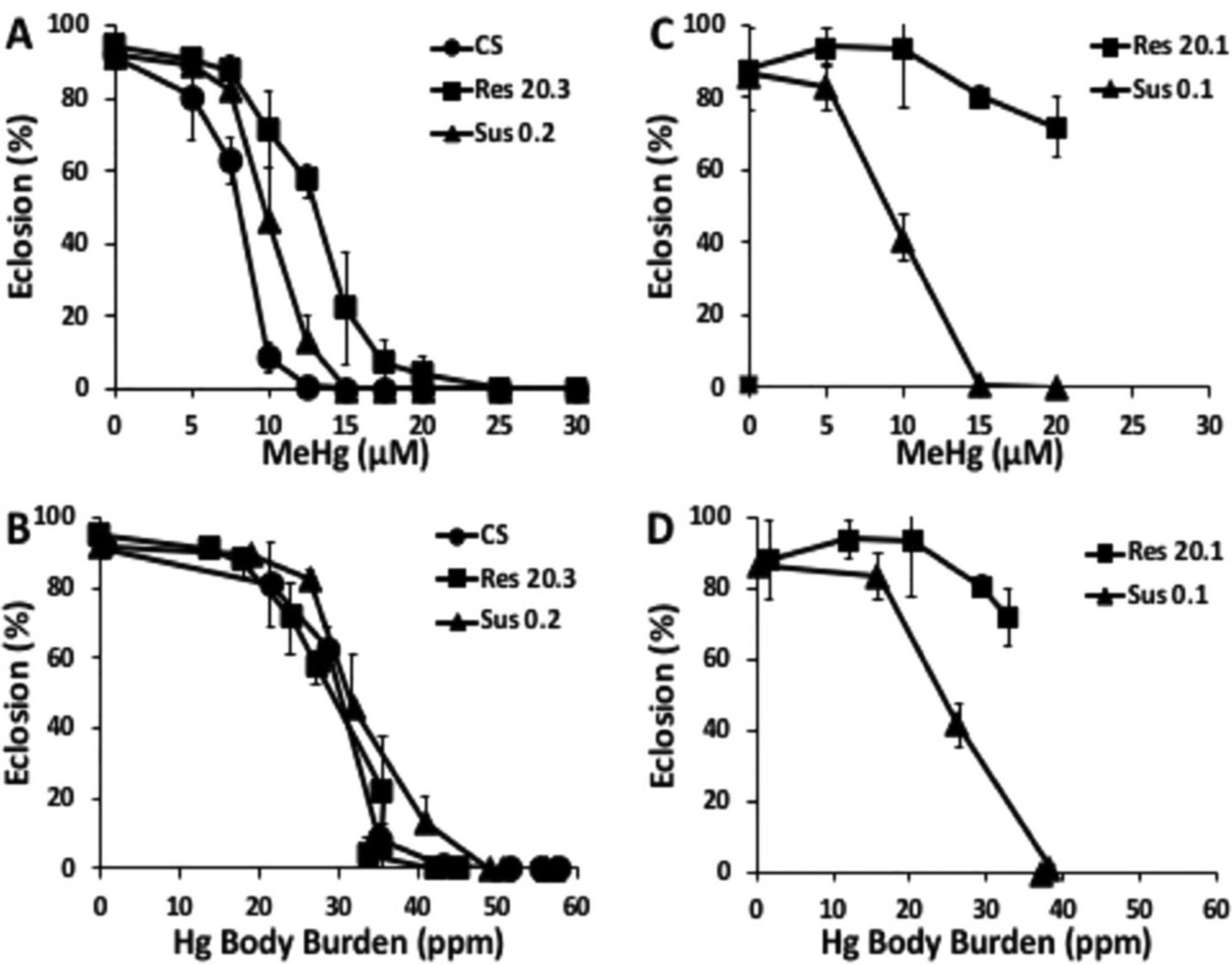
CONSIDERATIONS OF THE DEVELOPMENTAL STAGE FOR ASSESSING TOXICOLOGIC MECHANISMS
Drosophila, being holometabolous, offer a variety of developmental stage-specific phenotypic endpoints that are readily assayed. To zero in on potential toxicity targets and pathways, it is helpful to consider the morphogenetic programs that are active during each stage of the life cycle.
Embryogenesis is marked by a broad array of fundamental cellular and developmental processes, including cellularization (assembly of chromatin in a nuclear envelope and plasma membrane), proliferation (expansion of the blastoderm and subsequent progenitor cells of differentiated lineages), tissue patterning (e.g., axial determination, dorsal/ventral, anterior/posterior), differentiation and determination of cell fates (e.g., establishment of neurectoderm and neuroblasts), and cellular morphogenesis (e.g., neurite outgrowth and targeting). Embryo morphogenesis can readily be monitored with standard immunohistologic methods and genetically encoded reporter genes. A multitude of signaling pathways operate simultaneously across multiple cell types and developmental time points. When a specific toxicant interaction occurs, it may phenocopy a mutation in a morphogenetic pathway. For example, a hypertrophic neurogenic phenotype could implicate Notch pathway inhibition (Rand et al., 2009). However, pleiotropic actions arising from toxic exposure at the embryo stage may be less immediately interpretable. In addition, transcript levels are influenced by maternally deposited mRNAs (Johnstone & Lasko, 2001). Therefore, toxicant action on mRNA levels may not solely reflect effects on zygotic transcription.
The larval stages are prominent for growth. Larvae increase in size more than 20-fold over the first to third instars in a period of ∼4 days. Thus, adversely affected growth can give a first approximation for a toxic endpoint of exposures during the larval stage. This can be easily scored in terms of body length or mass, as well as delays in developmental timing. Larval stages progress through two molts of the exoskeletal cuticle (Fig. 1), which requires pulses of elevated ecdysone hormone signaling prior to each molt. Thus, the ecdysone pathway is an immediate toxicity target candidate when larval growth is diminished and/or delayed. Although growth-regulating pathways are highly active in larval stages, signaling mechanisms affecting cell fate and differentiation can also be revealed with toxic larval exposures, notably in the development of imaginal tissues within the soma of the larva. Perhaps most extensively studied are the imaginal discs of the wing and eye, the primordial tissues that will form the corresponding adult structures subsequent to pupal metamorphosis. Genetic insults occurring in the imaginal tissue at the larval stage (e.g., induction of Notch-/- clones in wing or eye discs) can yield abnormal morphogenesis of the corresponding adult structure (“Notched” wing margins and loss of ommatidia; Kurata et al., 2000). Thus, larval imaginal discs offer the potential to reveal toxicants with targeted specificity toward a prominent developmental signaling pathway. Larval exposure can also model a developmental origin of health and disease paradigm, where early life exposure results in a late life disease or deficiency. The larval nervous system undergoes rapid proliferation of neuroblasts and dynamic establishment of neuron-neuron and neuron-muscle synapses in stereotypic patterns on body wall muscles (neuromuscular junctions). Innervation of somatic muscle in Drosophila larvae are mapped in detail (Menon et al., 2013). Thus, the larval model offers a robust platform to assess toxicant effects on neurite outgrowth and targeting via standard immunostaining protocols or expression of vital fluorescent dyes, such as green fluorescent protein (GFP). At a finer resolution, the larval neuromuscular junction has been used extensively as a model system to investigate developmental programs for neuromuscular junction assembly (Banerjee et al., 2017; Menon et al., 2013), which has yet to be fully exploited for toxicologic studies.
Developmental programs at the pupal stage involve histolysis and tissue breakdown (autophagic and apoptotic), which precede and overlap with morphogenesis and assembly of adult structures. The pupal stage comprises complex events of metamorphosis occurring over ∼4 days. Ecdysone hormone signaling is essential for inducing the transition from larva to pupa and for the progression through metamorphosis. Several attributes of the pupal stage make it robust for toxicity assays. First, histolysis of larval organs and morphogenesis of adult organs can be monitored in real time. Notably, morphogenesis of adult flight muscles are readily imaged with expression of a muscle-targeted GFP reporter (Barthmaier & Fyrberg, 1995). Likewise, elaboration and branching of neurites can be imaged and quantified (Williams & Truman, 2004). An additional attribute of the pupa to consider for toxicity assays is that excretion does not occur. Thus, toxicant accumulated via larval feeding will persist in the fly through metamorphosis, effectively giving a pseudosteady-state internal dose (Fig. 1). Eclosion can easily be quantified and is the first adult behavior that requires neuromuscular integrity. Lastly, the pupal stage can be factored into modeling late-onset deleterious effects. Low-dose exposures at the larval stage can be accounted for by whole-body measurements of the toxicant in the pupae. Where the dose does not affect the pupal-adult transition, later-life onset of decline in activity, motor function, and shortening of lifespan, among several other neurologic behaviors, can be readily assayed in the adult well after the toxicant has left the system (Peppriell et al., 2021).
Once the adult is fully formed, developmental programs give way to metabolic programs, as well as programs of stress response that are needed to sustain homeostasis of the adult. Lethality and mortality are fundamental toxicity assays that are straightforward. Here, toxicity is commonly ascribed to events of oxidative stress that overwhelm the stress response systems and compromise essential metabolic or physiologic functions. The adult fly is ideal for assays of complex behaviors, as elaborated in the section on quantitative and systems genetics, below. Pioneering studies of Seymour Benzer led to paradigms to test for neuromuscular function of locomotor behaviors of climbing (negative geotaxis), phototaxis, and flight (Benzer, 1967, 1973). Even complex behaviors of associative learning and memory can be assayed using olfactory cues and food sources (Dubnau & Tully, 1998; El Kholy & Al Naggar, 2023; Zamberlan et al., 2020). Flies exhibit stereotypic courtship, mating, and aggressive behaviors, as well as circadian rhythm behaviors driven by highly conserved genes (e.g., Clock and Period genes; Ferveur, 2010; Konopka & Benzer, 1971; Manoli et al., 2005). Thus, the plethora of complex adult behaviors, and known genetic pathways regulating them, presents a robust platform for dissecting toxicological mechanisms.
MEASURING TOXIC EXPOSURE IN THE FLY
Flies are a rich resource for descriptive toxicology with the wide variety of endpoints available ranging from molecular to developmental to behavioral. However, each of these endpoints has important considerations for confounding factors that might alter the interpretation of the toxicant's activity.
Molecular Effects
Typical toxicologic endpoints include standard biochemical and molecular assays of gene and protein expression levels. Standard controls for assay validity apply (e.g., a housekeeping gene in qPCR [RP49] and a protein standard in immunochemical assays [actin]) that are not expected to show a response to the toxicant. It is critical for the interpretation of changes in expression levels to account for circadian rhythms and developmental timing. For example, the Notch target gene, E(spl)mDelta , undergoes a more than four-fold reduction in expression across the pupal stages of p5 to p6 (Prince & Rand, 2017), which spans a mere 13 of the 96 hr of total time for metamorphosis. Thus, even a slight developmental delay due to toxicant exposure could result in significantly different levels of E(spl)mDelta gene expression attributed to the toxicant compared with control flies. Comparative analyses of gene/protein expression are therefore best done across several developmental time points (Engel & Rand, 2014; Prince & Rand, 2017), and samples should be collected at the same time of day.
It is useful to consider how the fly/tissues are harvested for gene/protein expression analyses. For example, collecting head, thorax, and abdomen of adult flies separately via transection with a scalpel or razor blade is simple and straightforward and can enable a crude assessment of whether or not expression levels vary between neural versus muscle versus reproductive/intestinal tissues, respectively. Also critical for analyses are sex-dependent effects. Sexing adults is straightforward, whereas sexing larvae and pupae is more difficult but feasible for the trained eye.
Flies carrying reporter transgenes can be used for a toxicity readout. For example, flies engineered to express the antioxidant response element fused to GFP are effective as a reporter of Nrf2 transcription factor activation in response to specific oxidants, metals, and drugs (Gunderson et al., 2020; Sykiotis & Bohmann, 2008). Other strategies have used the promoter of heat shock protein 70 (HSP70) linked to reporter genes (e.g., lacZ or GFP) to report a toxic response to even crude mixtures of toxicants (Siddique et al., 2008). Such a reporter gene strategy can be used not only to reveal global toxicity across the fly but also to localize toxicity to specific organs and cells through microscopy (Gunderson et al., 2020; Siddique et al., 2007).
Developmental Effects
Among typical developmental phenotypes affected by toxic exposure are developmental delay and stage-specific developmental stalling or mortality. These endpoints include time to pupariation, length of metamorphosis, and successful eclosion. A comparison of all three endpoints can yield information on the relative efficacy of various toxicants at distinct developmental windows. A salient example of this is seen with an examination of lead, arsenic, and two mercury isoforms, where all of these metals can delay time to pupariation. However, methylmercury selectively inhibits eclosion at lower doses (Beamish et al., 2021). Other toxic effects can alter wings, eyes, bristles, and body size. Longevity is an informative endpoint directly applicable to toxicity effects. The lifespan of Drosophila is ∼70 days yet can vary widely due to genetic background and culture conditions (Huang et al., 2020; Mackay, 2002; Piper & Partridge, 2018). It is therefore critical to establish consistent culture conditions (i.e., temperature, food composition, number of flies per vial) to obtain reproducible results. Longevity is easy to score by tallying death events among replicate cultures of 50 or more same sex flies (>200 flies) over the course of 100 days or until all the flies are dead. Dietary restriction or starvation paradigms can accelerate the lethal effects of toxicants and yield endpoints on the scale of hours or days (Arking et al., 1991).
Behavioral Effects
Behavioral assays for toxicity in larvae include crawling speed, chemotaxis, phototaxis, and avoidance behavior (Aleman-Meza et al., 2015; Gerber & Stocker, 2007; Gerber et al., 2009). Tests of adult fly behaviors are continually being developed to assess complex behaviors (Moulin et al., 2021) for toxicity testing including locomotor activity, climbing, flight, and longevity, all of which are quantifiable (Gunderson et al., 2021; Peppriell et al., 2021). More elaborate behaviors such as mating and fecundity (Tiwari et al., 2011), food choice (El Kholy & Al Naggar, 2023), and even associative learning and memory (Zamberlan et al., 2020) are available to assess toxicity. As with any fly, behaviors are complex to interpret and can reflect perturbation at one of several biological levels ranging from energetics to neural morphogenesis and connectivity. Fortunately, the fly enables molecular genetic dissection to relate behavior to underlying genetics and physiology, as described below.
Limitations
Despite considerable conservation of fundamental developmental pathways, flies differ from mammals in several aspects of neuronal cell composition, neurotransmission, immune and cytokine signaling, and metabolism. For example, the mammalian brain comprises a glia to neuron ratio of ∼10:1, whereas that of Drosophila lies at 1:10 (Pfeiffer et al., 2008; Yildirim et al., 2019). At the neuromuscular junction, unlike the mammalian system, which releases acetylcholine, the fly system uses glutamate (Martin & Krantz, 2014; Nassel, 2018; Shin et al., 2018). Drosophila do not possess an adaptive immune system and instead rely on an innate immune response. This gap in a significant arm of immune signaling poses challenges for translating toxicity studies performed in a Drosophila model to mammalian toxicity pathways that may be even partially mediated via immune responses (Hoffmann, 2003; Salminen & Vale, 2020; Tassetto et al., 2017). It is important to acknowledge these shortcomings in the fly model, especially when interpreting the translatability of findings in fly toxicology studies.
MOLECULAR GENETIC DISSECTION OF TOXICITY MECHANISMS
A preferred strategy for determining mechanism is to interrogate how the action of the toxicant can be phenocopied, or alternatively, exacerbated or rescued by a change in expression of a gene that is within a pathway being targeted by the toxicant. Here, use of the Gal4>UAS (upstream activating sequence) transgene expression system is a standard practice (Brand & Perrimon, 1993). When a toxicant is presumed to inhibit a function of a protein, transgenic overexpression of the protein would be expected to rescue the phenotype, whereas knockdown of the protein expression would exacerbate susceptibility to the toxicant. The molecular "toolbox" of Drosophila is extensive and is supported by databases (http://flybase.org/; see Internet Resources) and repositories for fly strains (Bloomington Drosophila Stock Center) and reagents (Drosophila Genomics Resource Center), which provide UAS-RNA interference (RNAi) lines, CRISPR lines, and well-characterized gene deletion and disruption lines that effectively cover a majority of the coding genes across the genome. The utility of these resources, when coupled with quantitative and systems genetic approaches, is expanded upon in the section below.
Two strategies worth emphasizing for determining toxicity mechanisms are to (1) determine target organ specificity and (2) determine developmental windows of susceptibility. The strategy in the first instance is a targeted rescue approach, whereby a gene with a known "resistance" function toward the toxicity is systematically expressed in select tissues (e.g., neurons, muscle, fat body, or gut) in combination with the toxicant exposure. Resistance genes could include a CYP enzyme, a GST, or an ABCC transporter, which will either biotransform the toxicant to an inactive species or export it and thus spare the target organ. If targeted expression rescues the mortality or behavioral phenotype, it can be inferred that the respective organ is a central target. The strategy in the second instance is to determine windows of susceptibility using the temperature-inducible Gal80 construct in combination with Gal4>UAS, which enables a targeted turning on and off of gene expression within a narrow time frame. By systematically upregulating the expression of a resistance gene in sequential 12-hr windows across pupal development, the toxic effects on an adult phenotype can be monitored and tracked to a developmentally susceptible window.
A SUMMARY OF PRINCIPLES FOR TOXICOLOGICAL STUDIES ON DROSOPHILA
As more investigators are turning to Drosophila as a complementary whole-fly alternative model to toxicology work in cells or mice, it is important that the special attributes of fly development, genetics, metabolism, and behavior are integrated with fundamental principles of toxicology.
- 1. Evaluate More Than One Strain
A genetic basis for the variation in resistance and susceptibility to a toxicant can be quickly established by surveying several strains of flies for their dose response profile. The evaluation of multiple strains opens the door to the power of genomics and metabolomics for resolving toxicity pathways.
- 2. Consider Dosing Modality
Guide the choice of toxicant administration mode around the life stage that will yield the most relevant endpoint. Consider the steps needed for consistent controlled dosing together with the need to achieve assay throughput.
- 3. Account for Internal Dose Together with the External Dose
Where genetic variation exists in a dose response, accounting for the body burden is the first step in deducing whether it is a result of a toxicokinetic effect or a toxicodynamic effect. Distinguishing between these two effects will guide the rationale for interrogating relevant target pathways.
- 4. Validate Expression Pattern of the Gal4 Construct Being Used
To establish tissue specificity for a toxicant action, the pattern of expression using the Gal4>UAS rescue strategy must be verified. Gal4 drivers that are tissue specific at one stage of development may vary in expression at a later stage. For instance, the conventional pan-neural Gal4 driver, Elav C155, actually expresses in flight muscles during pupal metamorphosis (Gunderson et al., 2020). Driver expression can be easily validated with a standard UAS-GFP reporter.
- 5. Account for Biotransformation of the Toxicant
Flies are equipped with phase I and II xenobiotic metabolizing enzymes, yet the preferential metabolite of the parent compound may vary from that seen in mammalian systems. Knowing the xenobiotic transformation is key for assessing the ultimate toxicant in the system.
- 6. Account for Distribution of the Toxic Effect
The small size of flies makes it quick and easy to sample the whole fly for readout of gene and protein expression. However, information on targeted effects will be masked unless sampling is refined to body regions and, optimally, to microdissected tissues or even individual cell types using single cell RNA sequencing methods.
- 7. Use Available Information to Choose the Toxicity Assay Endpoint
As an example, longevity is a robust Drosophila assay and a possible indicator of aging mechanisms, nutritional status, and delayed effects due to early toxic exposure. However, factors that can extend and shorten lifespan are numerous (Huang et al., 2020; Piper & Partridge, 2018).
QUANTITATIVE AND SYSTEMS GENETICS APPROACHES TO IDENTIFYING RISK FACTORS FOR TOXIC EXPOSURE
The Drosophila Model for Quantitative Genetic Studies of Toxic Exposure
The sensitivity to toxic exposure and severity of toxicant-induced phenotypes differs among individuals in a population. Variation in sensitivity to toxic exposure arises from interactions among multiple environmentally sensitive genetic variants that segregate in the population. This underlying genetic architecture composed of additive, dominant, genotype-by-environment, and epistatic interactions determines individual variation in the threshold for adverse toxic effects. Thus, sensitivity to toxic exposure is a quantitative trait, and understanding—and ultimately predicting—the genetic factors that predispose to sensitivity to toxicants requires quantitative and systems genetics analyses.
Identifying genetic risk factors for sensitivity to toxicants is challenging in human populations because of uncontrolled genetic backgrounds, lifestyles, and developmental histories. In addition, the dose and duration of exposure are often unknown. Adverse toxic effects can emerge long after exposure, and simultaneous exposure to multiple toxicants is a confounding factor. Experiments on rodent models are feasible but limited in sample sizes and diversity of genetic backgrounds. In addition, cost considerations and increasing awareness of ethics concerns limit the utility of traditional rodent models. The haplotype structure in both the human genome (Daly et al., 2001; Reich et al., 2001) and rodents (Guryev et al., 2006), which consists of large linkage blocks, makes it difficult to assign causality of associations with toxicity risk phenotypes to variants within individual genes based on tagging SNPs.
Drosophila melanogaster has emerged as a powerful model for systems genetics analyses of toxic exposure. Flies can be inbred, which enables precise control over the genetic background, environmental growth conditions, and dose and time frame of toxic exposure. Replicated genotypes can be grown in large numbers, rapidly, at low cost, and without regulatory restrictions. A wealth of publicly available genetic resources from national stock centers enables sophisticated functional validations. Multiple behavioral and physiologic phenotypes can be quantified accurately at different concentrations of toxicants. In addition, whole-genome transcriptional analyses can identify differentially expressed transcripts in response to toxicant exposure, enabling comprehensive systems genetics analyses. The power of the Drosophila model is further enhanced by the D. melanogaster Genetic Reference Panel (DGRP), a community resource that enables genome-wide association studies (Huang et al., 2014; Mackay et al., 2012).
The Drosophila Genetic Reference Panel
The original DGRP Freeze 2 is a population of 205 sequenced inbred Drosophila lines derived from a natural population (Mackay et al., 2012) and has recently been expanded to ∼1000 lines (unpublished). Gravid females were collected from peaches at the Raleigh Farmers’ Market in North Carolina, and offspring of individual females were subjected to 20 generations of full-sibling inbreeding (Fig. 3). The original 205 lines were sequenced to high coverage (average ∼27 fold) and extensively annotated. They contain 4,565,215 naturally occurring molecular variants, including 3,976,011 high-quality SNPs or multiple nucleotide polymorphisms, 169,053 polymorphic insertions, 293,363 polymorphic deletions, and 125,788 polymorphic microsatellites. Common inverted and standard karyotypes are genetically divergent and account for most of the variation in relatedness among the DGRP lines (Huang et al., 2014; Mackay et al., 2012). Approximately 50% of the DGRP lines are infected with the endosymbiont Wolbachia pipientis , but effects of Wolbachia infection on quantitative traits are rarely significant. The vast majority of the DGRP lines are genetically unrelated and linkage disequilibrium (LD), which determines the precision of mapping causal variants, decays rapidly within ∼100 bp, facilitating assessment of causality of associated polymorphisms in genome-wide association studies (Huang et al., 2014). The DGRP provides a living library of natural variants that have survived the sieve of natural selection, and all traits measured in the DGRP to date show extensive phenotypic variation that far exceeds variation observed among standard laboratory inbred strains or recombinant inbred lines. Since individuals within each line are virtually genetically identical, the same genotypes can be measured repeatedly, and different laboratories can measure and correlate phenotypes on the same genotypes.
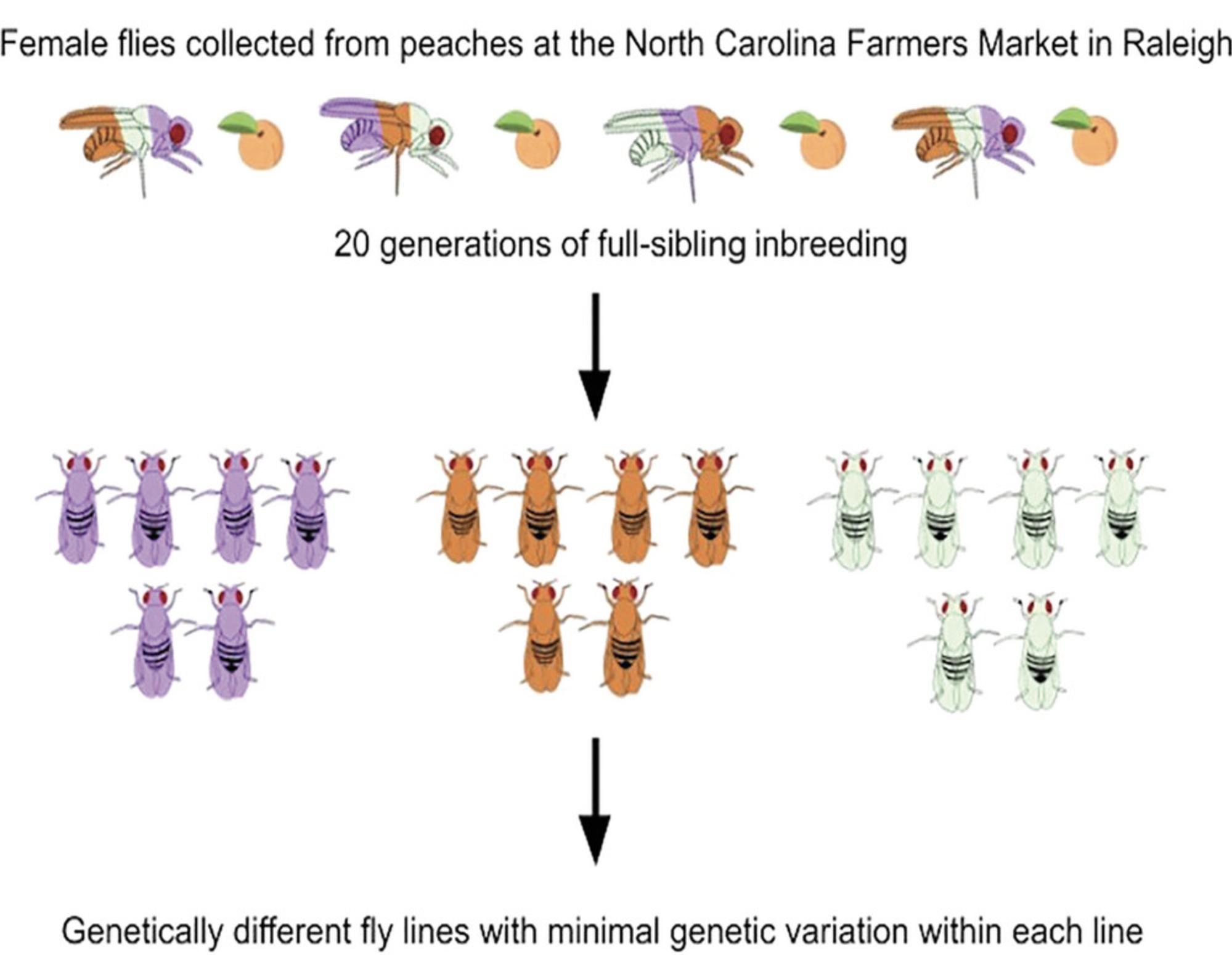
The quantification of genome-wide variation in gene expression in the DGRP increased the annotated Drosophila transcriptome by 11%, including thousands of novel transcribed regions (NTRs; Everett et al., 2020). It is noteworthy that these NTRs are predominantly located in heterochromatic regions of the genome, traditionally thought to be transcriptionally silent. They are often negatively correlated with the expression of protein-coding genes and likely have regulatory functions. About 42% of the Drosophila transcriptome, including the NTRs, is genetically variable in males and females and is organized into modules of genetically correlated transcripts (Boyd et al., 2011; Everett et al., 2020; Huang et al., 2015).
DGRP-Derived Advanced Intercross Populations for Extreme Quantitative Trait Locus Mapping
The DGRP has been used to identify variants associated with variation in a wide range of morphologic (Özsoy et al., 2021; Zwarts et al., 2015), physiologic (Carbone et al., 2016; Dembeck et al., 2015; Huang et al., 2012; Huang et al., 2020; Jordan et al., 2012; Olivares et al., 2023; Weber et al., 2012; Zhou et al., 2012), and behavioral (Arya et al., 2015; Baker, Carbone, et al., 2021; Chi et al., 2021; Harbison et al., 2013; Harbison et al., 2019; Highfill et al., 2019; Shorter et al., 2015; Spierer et al., 2021; Swarup et al., 2013) phenotypes. Genome-wide associations seldom reach genome-wide significance based on Bonferroni corrections for multiple tests. However, the Bonferroni correction assumes independence of tests, whereas the Drosophila transcriptome is highly intercorrelated (Ayroles et al., 2009; Willingham et al., 2006; Zhou et al., 2012). Thus, applying a Bonferroni-corrected threshold for significance is overly restrictive and would prevent the discovery of many true positive associations. Most genome-wide association studies on the DGRP have used a significance threshold of p < 10–5. This lenient threshold, which is several orders of magnitude below the Bonferroni threshold, is justified based on deviations from linearity of quantile-quantile plots and generally results in the identification of several hundred polymorphisms associated with phenotypic variation in the trait under study.
The statistical power of the DGRP can be enhanced by generating DGRP-derived advanced intercross populations (AIP; Anholt & Mackay, 2018; Baker, Carbone, et al., 2021; Carbone et al., 2016; Huang et al., 2012; Morozova et al., 2015). In this design, a subset of DGRP lines, either chosen at random or selected from lines that are free of Wolbachia or karyotypic inversions, are crossed in a round-robin design to generate a base population in which all selected line variants are initially represented at equal frequency. Thus, variants that are unique to any line are present at frequency 1/N , where N is the number of parental lines of the AIP. Intercrossing this population for many generations while maintaining them in large population sizes to minimize genetic drift will result in the breakdown of LD due to recombination and increased genetic diversity within the population. Association analyses can be performed on the AIP by measuring individual phenotypes in replicates for sexes separately and pooling individuals at the extremes of the phenotypic distribution for subsequent bulk DNA sequencing. Since the advanced intercross population is derived from a base population of a subset of DGRP lines, it captures a smaller portion of the genetic variance represented in the entire DGRP. However, most common variants will be represented in this population, and this design also captures rare variants in the AIP base population that are refractory to genome-wide association analysis using the DGRP.
Association analyses can be performed on the advanced intercross population by measuring individual phenotypes in replicates for sexes separately and pooling individuals at the extremes of the phenotypic distribution for subsequent bulk DNA sequencing and extreme quantitative trait locus (QTL) mapping (Anholt & Mackay, 2018; Baker, Carbone, et al., 2021; Carbone et al., 2016; Huang et al., 2012; Morozova et al., 2015). Alternatively, the 10% of surviving flies after toxic exposure can be collected and contrasted with a pool of randomly selected individuals from the population. Associations are inferred from significant differences in allele frequencies between the high and low pools. The larger the number of individuals is in each pool, the greater will be the resolution of differences in allele frequencies. Increased statistical power in this design can give rise to associations that reach Bonferroni significance.
One of the advantages of the Drosophila model is that associations can be confirmed both at the gene level and the SNP level. Causal validation of associations of candidate genes that harbor variants associated with phenotypic variation can be achieved by targeted disruption of gene expression either through publicly available transposon-insertion lines or RNAi with appropriately controlled genetic backgrounds. Although one needs to take care to select RNAi lines without predicted off-target effects, it is recommended to use multiple RNAi lines targeting the same gene to alleviate potential concerns of off-target effects. Similar concerns arise with CRISPR gene editing, which is still challenging in Drosophila. Studies on many traits have indicated empirical false positive discovery rates of ∼20% or less (Arya et al., 2015; Carbone et al., 2016; Jordan et al., 2012; Shorter et al., 2015; Swarup et al., 2013). The validation rate is higher for candidate genes that can be grouped into genetic interaction networks.
Higher resolution validation at the SNP level can be performed by comparing the phenotypic effects of alternative SNPs in multiple randomized genetic backgrounds by crossing lines with each alternative SNP inter se for several generations and analyzing the effects of replicate SNP-specific advanced intercross populations on the trait (Baker, Carbone, et al., 2021). This procedure is, however, time and labor intensive and limited to a subset of candidate SNPs. The future availability of ∼1000 DGRP lines will provide increased power to enable out-of-sample validation by replicating associations observed in a subset of the population through measurements on individuals from the same population or by contrasting phenotypic measurements on DGRP lines with alternative SNPs since rare SNPs in the original DGRP may have greater representation in the expanded DGRP3 collection.
High-Throughput Behavioral Screens for Toxicity
Most studies measure lethality (e.g., LD50) as a measure for toxicity. A simple and rapid screen for toxicity in flies has been developed that uses toxin-impregnated filter paper discs to assess lethality (Holsopple et al., 2023). Toxic effects at sublethal doses of toxicants can be quantified using a variety of behavioral assays (see Measuring Toxic Exposure in the Fly and Fig. 1). Since sexual dimorphism is characteristic of virtually every quantitative trait that has been measured in Drosophila, it is essential to conduct behavioral measurements on sexes separately under conditions of controlled genetic background and rearing conditions and during standardized times to prevent confounding circadian effects. Standard analysis of variance models can be used for the assessment of the statistical significance of behavioral data.
Locomotor activity is central to the expression of most behaviors, and the effects of toxic exposure can be readily quantified using drosophila activity monitors (DAMs). In this system, a single fly is placed in a narrow tube, and an activity count is registered every time the fly crosses an infrared light beam (Fig. 4). The DAM system can accommodate 32 tubes simultaneously, and the system is housed in a fly incubator at a controlled temperature and light cycle. With an available food source in the tubes, flies can be monitored for several days, if needed. A 5-min absence of activity is defined as sleep (Hendricks et al., 2000; Shaw et al., 2000). Activity data can be analyzed using the ShinyR-DAM program (Cichewicz & Hirsh, 2018), which provides information about total activity, day and night activity, sleep bouts, and total day and night sleep. All measurements are performed on toxicant-exposed flies and controls, sexes separately.
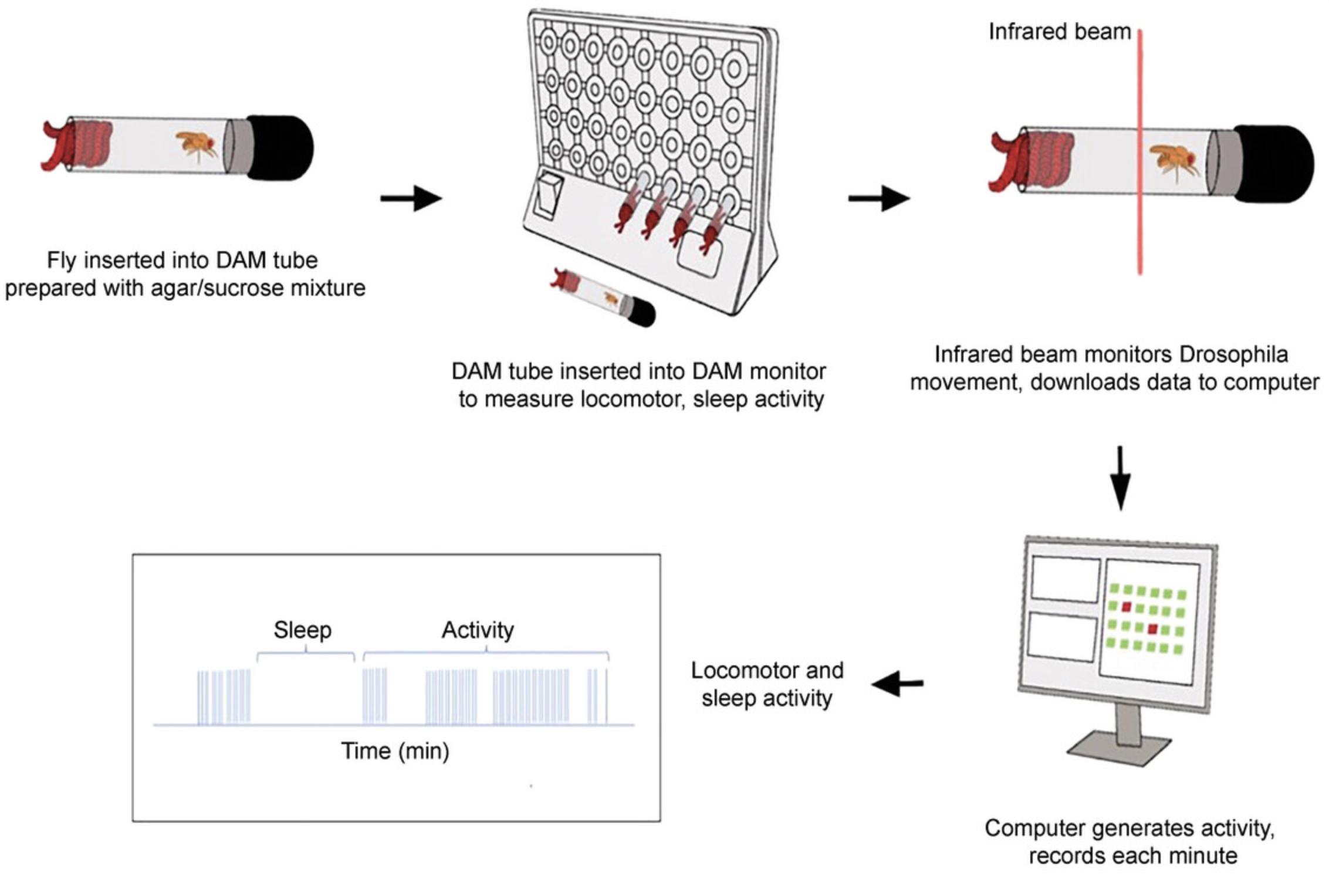
In addition to locomotor activities, the effects of toxicants on sensorimotor integration can be assessed using phototaxis, geotaxis, and startle behavior assays. Phototaxis is measured with the counter-current apparatus designed by Benzer, where flies move toward a light source by moving from a vial into a connected vial during eight successive time-limited opportunities (Benzer, 1967). This gives rise to a distribution of flies across the eight time points as quantification of their phototaxis sensitivity. When the apparatus is placed vertically instead of horizontally and without a directional light source, the same system can be used to assess climbing behavior as an expression of the fly's characteristic negative geotaxis response.
Startle behavior can be measured by measuring the time flies move immediately after being exposed to a mechanical stimulus (Yamamoto et al., 2008). To standardize the mechanical startle stimulus, a vial housing a single fly is placed in a chute, and the removal of a supporting dowel allows the vial to drop from a height of 42 cm, after which it comes to rest horizontally (Huggett et al., 2021). The short duration of the assay enables large numbers of flies to be tested at high throughput. As with all behavioral assays, males and females are analyzed separately. Aberrant startle-induced behaviors (e.g., seizures, compulsive grooming behavior) can be documented and have been observed after exposure to cocaine (Huggett et al., 2021).
A microtiter plate–based high-throughput assay for alcohol sensitivity has been developed where alcohol knockdown time is measured when flies are exposed to ethanol vapors (Sass et al., 2020). This assay can, in principle, be applied to any toxic vapor. Similarly, a multiplate feeder assay has been developed in which the consumption of liquids can be measured accurately and at high throughput (Walters et al., 2021). This system can be used for no-choice and two-choice consumption assays.
Genome-Wide Association Analyses for Susceptibility to Agricultural Toxicants
The DGRP has emerged as a valuable resource for studies on genetic factors that determine susceptibility to once widely used toxic herbicides that cause oxidative stress and mitochondrial dysfunction, including paraquat (1,1′-dimethyl-4,4′-bipyridine; Lovejoy et al., 2021; Weber et al., 2012) and to pesticides such as malathion and permethrin (Battlay et al., 2018).
Paraquat, a commonly used herbicide, is known to induce mitochondrial oxidative stress (Chen et al., 2021). Due to its high toxicity, the use of paraquat has been banned in many countries. The neurotoxic effects of paraquat may lead to the development of Parkinson's disease (Chung See et al., 2023), although a definitive causative link between paraquat exposure and development of Parkinson's disease is still debated (Weed, 2021). A genome-wide association analysis for resistance to paraquat and menadione sodium bisulfite with 167 DGRP lines uncovered significant genetic variation for susceptibility to both toxins (Weber et al., 2012). The SNPs that were identified with variation in susceptibility to paraquat or the oxidative stressor menadione sodium bisulfite were often sex- and toxicant-specific, but a small subset of associated SNPs was common for both sexes or treatments. There was an inverse relationship between effect size and allele frequency. Linear models with up to 12 SNPs could explain 67% to 79% and 56% to 66% of the phenotypic variance for resistance to paraquat and to menadione sodium bisulfite, respectively. Bioinformatics analyses revealed genetic interaction networks associated with DNA metabolism and neuronal development. Many genes that harbored SNPs associated with variation in susceptibility to these compounds were novel with no known role in oxidative stress resistance (Weber et al., 2012).
A subsequent study measured climbing activity after exposure to a low dose of paraquat in 100 DGRP lines and identified 17 genes in females and 35 genes in males that were associated with variation in climbing activity (Lovejoy et al., 2021). Only two candidate genes overlapped between the sexes despite a significant positive correlation between male and female susceptibilities, again highlighting sexual dimorphism in susceptibility to toxicants. Associations of variants within these candidate genes and phenotypic variation was assessed with RNAi knockdowns, gene expression analyses, and the quantification of dopamine. The genes identified in this study were distinct from those identified by Weber et al. (2012). This is not surprising since the genetic architecture that underlies susceptibility to toxic exposure may shift with the concentration of exposure, and different genetic factors can contribute to variation in different phenotypes affected by exposure to the same toxicant. This is exemplified by a study that showed that different gene-gene interactions were associated with aggression and different morphological parameters of the mushroom bodies, structures of the Drosophila brain that mediate experience-dependent modulation of behaviors and that are essential for enabling aggression (Zwarts et al., 2011).
Drosophila as a Model for Heavy Metal Toxicity
Heavy metals are ubiquitous in the environment. Some heavy metals (e.g., zinc, copper, and iron) play essential roles as cofactors for enzymatic reactions but are toxic in excess. Other heavy metals, including methylmercury, lead, and cadmium, do not contribute to biological functions. They may compete for endogenous enzymatic cofactors, affect ion channels, or cause oxidative damage. They can affect multiple organ systems, including the central nervous system, since they can cross the blood-brain barrier.
Drosophila can serve as an advantageous model for toxicogenomic studies of heavy metal toxicity, including methylmercury (Magnusson & Ramel, 1986; Montgomery et al., 2014), lead (Hirsch et al., 2009; Zhou et al., 2017; Zhou et al., 2016), and cadmium (Akins et al., 1992; Mu et al., 2021; Zhou et al., 2017).
A genome-wide association study in the DGRP for susceptibility to methylmercury found enrichment for candidate genes involved in muscle and neuromuscular development with disruption in flight muscle morphogenesis in pupae (Montgomery et al., 2014). The identification of muscle development as a new target for methylmercury toxicity was further supported by the demonstration that susceptibility to methylmercury is modulated by a central myogenic gene, kirre , and by muscle-specific overexpression of glutamate-cysteine ligase, which offers protection to methylmercury toxicity (Montgomery et al., 2014).
Extreme QTL mapping using a DGRP-derived advanced intercross population (Anholt & Mackay, 2018) identified SNPs associated with variation in resistance to lead acetate and cadmium chloride (Zhou et al., 2017). These SNPs were largely sex specific. Mutational analyses and targeted RNA interference were used to validate candidate genes, which enabled the construction of genetic networks for sexes separately that were either unique for lead acetate exposure or cadmium chloride exposure as well as a comprehensive network that combined all candidate genes associated with variation in susceptibility to both heavy metals. These networks were enriched in genes associated with neurodevelopment and connectivity, including members of the dpr gene family that act as neuronal surface markers that mediate the specificity of synaptic connections. Human orthologs could be superimposed on the D. melanogaster networks. The corresponding human network was larger than the fly network since multiple human paralogs could correspond to a single Drosophila ortholog (Zhou et al., 2017).
Several human orthologs that were identified in this study have previously been implicated in lead or cadmium toxicity (Zhou et al., 2017). Polymorphisms in Drosophila MtnB and MtnC , which encode metallothioneins, are associated with variation in resistance to both cadmium and lead. Human metallothionein binds cadmium and offers protection against cadmium toxicity. Cadmium-metallothionein complexes have been implicated in renal toxicity (Klaassen et al., 2009). The Drosophila ortholog of the human VDR gene, Hr3 (also known as Hr46), was identified as a network hub gene associated with lead resistance in females as well as cadmium resistance in both sexes. VDR encodes the vitamin D3 receptor, which is essential for the incorporation of calcium in bone, and polymorphisms in VDR have been implicated in sensitivity to lead toxicity (Onalaja & Claudio, 2000; Schwartz et al., 2000). The identification of human orthologs known to be associated with heavy metal toxicity provides a proof-of-principle for translational inferences from Drosophila toxicogenomic studies and their integration into orthologous networks places them in a genetic context that would not otherwise be evident.
Drosophila as a Model for Adverse Effects of Substance Use and Alcohol Disorders
Illicit drugs, like cocaine and methamphetamine, and alcohol represent a special class of toxicants that cause a large socioeconomic burden in the US and worldwide. Studies to identify genetic variants predisposing to substance use disorders in human populations have been underpowered due to the criminalization of drug use (which makes it difficult to recruit subjects), uncontrolled genetic backgrounds, heterogeneity in environmental histories, and confounding neuropsychiatric disorders and multidrug and alcohol use. The Drosophila system is amenable to comprehensive systems genetic analyses of cocaine consumption (Kaun et al., 2012). Cocaine acts by blocking presynaptic reuptake of dopamine via a dopamine transporter thereby prolonging dopaminergic neurotransmission. Drosophila have a dopamine transporter for which the crystal structure has been obtained, with a binding site that can accommodate cocaine (Wang et al., 2015). When flies are exposed to cocaine they show motor responses that resemble those observed in rodents exposed to cocaine, and they develop sensitization to repeated intermittent exposure to cocaine (Filosevic et al., 2018; McClung & Hirsh, 1998). Dopamine (Bainton et al., 2000), the dopamine transporter (Wu & Gu, 2003), and the serotonin transporter (Borue et al., 2009; Borue et al., 2010; Corey et al., 1994; Demchyshyn et al., 1994) have been implicated in mediating cocaine-induced behaviors in flies (Li et al., 2000; Simon et al., 2009), showing that fundamental neural mechanisms activated by psychostimulants are conserved.
A study on the DGRP identified candidate genes expressed in dopaminergic neurons and mushroom bodies that are associated with variation in consumption and development of preference for cocaine and methamphetamine (Highfill et al., 2019). One advantage of Drosophila is the ability to survey gene expression in the entire brain in a single analysis with single-cell RNA sequencing. In addition, pooling multiple brains from the same genotypes in each replicate enhances statistical power by averaging out environmental variation. Initial studies on single cell RNA sequencing in the Drosophila brain relied on methods to dissociate fly brains under conditions in which live cells could be obtained, which could then be separated in a 10X Genomics microfluidics device where they are lysed and their RNA reverse transcribed for subsequent cDNA sequencing (Baker, Mokashi, et al., 2021; Davie et al., 2018; Mokashi et al., 2021). Subsequent technologic improvements now enable the separation of intact single nuclei from frozen tissue for single-cell transcriptome analysis. Cells can be grouped in cell clusters based on similarities of their transcriptional signatures, and clusters can be annotated based on the expression of known biomarkers. The data can be subjected to dimensionality reduction to provide a two-dimensional visualization of color-coded cell clusters. A study in which flies were allowed to consume a fixed amount of cocaine in a sucrose solution versus a control of sucrose only during a defined circadian period revealed 86,224 cells that could be grouped in 36 distinct clusters (Baker, Mokashi, et al., 2021). These cells represented the total of duplicate samples for both sexes separately under both treatment conditions. In each case, about 5% of the ∼200,000 cells of the Drosophila brain were recovered. This restricted sample, however, represented all major cell types (neuronal and glial) as well as neurotransmitter types from most brain regions. Differential gene expression was widespread across both neuronal and glial cell populations and showed profound sexual dimorphism with greater responses to cocaine exposure in males than females. Kenyon cells of the mushroom bodies and glia showed especially large changes in gene expression upon cocaine exposure.
Studies on alcohol-related phenotypes are also problematic in human populations because of the difficulty in controlling environmental conditions and the reliance on self-reported questionnaires to quantify alcohol-related phenotypes, often confounded with other neuropsychiatric conditions. To assess the effects of alcohol exposure during development on gene expression in the adult brain, the same single-cell RNA sequencing approach was used (Mokashi et al., 2021). Gene expression differences following developmental alcohol exposure showed extensive sexual dimorphism with little overlap between males and females. Differentially expressed genes were associated with glutathione metabolism, lipid transport, glutamate and GABA metabolism, and vision in sexually dimorphic global multicluster interaction networks. Overlap between the results from single-cell RNA sequencing studies on cocaine and alcohol indicate that common neural substrates may be involved in the transcriptional response to both chemicals (Mokashi et al., 2021). The single-cell RNA sequencing approach used for studies on cocaine and alcohol can be employed to look at the effects of other neurotoxins on the Drosophila brain.
THE EMERGING IMPORTANCE OF METABOLOMICS-BASED TOXICOLOGIC STUDIES IN DROSOPHILA
Metabolomics has emerged as a powerful tool for studying toxicology. Since metabolomic approaches can reveal changes in cellular energetics and biosynthesis that would go undetected using techniques that quantify gene expression and protein abundance, this emerging field significantly expands our ability to explore the molecular and cellular events that compose adverse outcome pathways (Viant et al., 2019). Moreover, metabolomics approaches not only complement the genomic, transcriptomic, and proteomic techniques described here and elsewhere, but the resulting data provide a unique perspective of how individual chemicals interact with some of the most highly conserved processes in all of biology. After all, small molecules represent the simplest means of comparing the effects of toxicants on distantly related species, as the metabolites produced by central carbon metabolism and peripheral enzymatic pathways are identical across vast evolutionary distances (Colbourne et al., 2022). Metabolites also represent the ultimate phenotypic readout as they provide a direct snapshot of the energetic and biosynthetic state of a cell. Thus, any modern chemical safety study should use metabolomics as part of a multiomics approach.
Drosophila has proven to be highly amenable to metabolomic approaches (Cox et al., 2017). For example, metabolomic studies in the fly identified novel molecular mechanisms linking growth and nutrition (Jouandin et al., 2022), uncovered noncanonical functions for highly conserved enzymes (Li et al., 2017), and examined the metabolic changes that occur during development (An et al., 2014; Nishimura, 2020; Petrova et al., 2018; Tennessen et al., 2014), aging (Jin et al., 2020; Maslov et al., 2021; Parkhitko et al., 2020; Zhao et al., 2022), and the progression of chronic metabolic diseases (Bertrand et al., 2020; Cormier et al., 2021; Hofbauer et al., 2021; Tuthill et al., 2020). However, despite the growing use of metabolomics among Drosophila researchers, very few Drosophila toxicology studies examine the metabolome (for examples see Brinzer et al., 2015; Li et al., 2020). Considering that the conserved nature of Drosophila metabolism holds the potential to discover novel metabolic mechanisms by which toxicants disrupt disease-causing metabolic pathways (Colbourne et al., 2022; Holsopple et al., 2023), metabolomics must become common practice in fly toxicology research.
A variety of technical approaches, including nuclear magnetic resonance spectroscopy, gas chromatography-mass spectroscopy, and liquid chromatography-mass spectroscopy, can be used for Drosophila metabolomic studies (Tennessen et al., 2014). Together, these techniques allow for the measurement of metabolites ranging from small polar metabolites—such as sugars, citric acid cycle intermediates, and amino acids—to lipids and more complex molecules. In theory, any molecule capable of being analyzed in a biological tissue can be measured in flies, and as a result, the technique used to conduct Drosophila metabolomic studies is largely dictated by the question of interest. In the context of toxicology, metabolomics and mass spectrometry–based approaches can address key questions that are important in any study, including measuring the amount of toxicant that is present in the fly, determining the metabolic derivatives produced from the toxicant, and examining how the toxicant influences a broad-range of metabolic pathways.
Since there is no single assay capable of measuring all metabolites within biological systems, the metabolomic approach used to study exposed flies must be thoughtfully selected to examine metabolites that will provide the most insight as to how a toxicant alters metabolism and physiology. For instance, metabolomics analysis of flies exposed to a heavy metal such as arsenic would use targeted methods to quantify metal ions, small polar metabolites, and lipids (for examples see Beamish et al., 2021; Carvalho et al., 2012; Li & Tennessen, 2018). Individual studies must also choose whether to employ a targeted approach designed to quantify a predetermined set of metabolites, an untargeted approach, which aims to measure as many metabolites as possible, or a hybrid method. Both targeted and untargeted approaches have been used in Drosophila, and the decision as to which method to use depends on whether an analysis is focused on discovery or hypothesis testing. Regardless of the method, metabolomic studies in the fly hold the potential to quickly identify the molecular mechanisms that link chemical exposure to phenotypic outcomes. Here we briefly describe basic principles of study design and data interpretation.
Considerations for Generating Reproducible Metabolomics Data
An emerging concept in toxicology is that the exposome, broadly defined as the cumulative environmental and physiologic factors encountered during the lifecycle, interacts with an organism's genetic makeup to influence phenotypic outcomes. Factors ranging from air pollution and subtle changes in dietary composition to other, often unnoticed, environmental stressors such as acute stress caused by handling can have long-term effects on phenotypes. Thus, the generation of meaningful toxicologic data requires carefully controlled experiments, and even then, laboratory-specific environmental conditions can introduce substantial variability from experiment to experiment. The influence of the exposome is perhaps most obvious when studying metabolism, where large pools of metabolites turn over on the order of minutes or even seconds, and emerging evidence suggests that environmental stress can influence the metabolism of subsequent generations. As a result, minor variations in both long-term culture conditions and short-term exposure protocols can dramatically affect the results of a metabolomics study. In this regard, there are three major variables to consider in a fly laboratory: culture conditions, sample collection, and genetic background. Here we briefly describe how these major variables should be controlled.
The most obvious factors that influence the metabolome are laboratory culture conditions (e.g., temperature, humidity, light cycle, food composition). Care must be taken to ensure that these variables remain constant over the course of a study. Changes in any of these variables can lead to significant variability in metabolite levels, as evidenced by the many studies examining the effect of temperature on the metabolome. In this regard, long-term studies should consider bulk purchasing any seasonally harvested dietary ingredient (e.g., cornmeal, molasses, malt, soy flour) to ensure consistency across experiments, as natural variations within these dietary components can affect the metabolome. We would recommend using a defined or semidefined diet for metabolomic studies, such as previously described recipes for semidefined diets (Reis, 2016), holidic diets (Piper et al., 2014), or a recently described liquid diet designed for adult toxicology exposures (Holsopple et al., 2023).
In addition to the standard culture conditions, toxicology studies are further complicated by the possibility that the compound of interest may alter feeding behavior, digestion, and physical activity (Arguello et al., 2013; Anholt, 2020). Any changes in behavior or nutrient intake will significantly alter metabolism; thus, the interpretater of metabolomics data must be aware of the possibility that changes in metabolite abundance could reflect changes in behavior instead of a metabolic response to the compound of interest. Although a simple blue-dye feeding assay can serve as an initial screen for detecting changes in food intake, more thorough analyses involving quantitative measurement of feeding behavior and nutrient consumption should be considered before generating and interpreting metabolomics datasets (Jaime et al., 2023; Ro et al., 2014; Walters et al., 2021).
Many of the same factors that influence the metabolome during an exposure experiment must also be controlled for during sample collection. Metabolites in central carbon metabolism turn over on the order of seconds and minutes, as evidenced by a recent study that found that a 30-s delay in the freezing of harvested mouse liver resulted in significant remodeling of levels of 31 metabolites (Rauckhorst et al., 2022). Although similar studies have not yet been conducted in Drosophila, anyone studying the fly metabolome should assume that Drosophila metabolic turnover progresses at a similar rate. The key to avoiding such issues is to rapidly collect the sample, immediately freeze the collection tube in dry ice or liquid nitrogen, store at −80°C, and never allow the samples to warm during homogenization and metabolite extraction (for protocols see Li & Tennessen, 2018). Any delays before quenching of endogenous metabolic pathways present within the sample will inevitably produce unreliable data. In this regard, tissue-specific metabolomics using fly tissues are possible, but quite difficult (see Chintapalli et al., 2013), and require exceptional dissection skills and immediate freezing of tissues.
Finally, metabolomic studies are highly sensitive to genetic background. Different genetic background effects can disrupt metabolomic studies. As reviewed elsewhere, many commonly used genetic backgrounds will produce artifacts in metabolomic studies, with mutations in the gene white proving particularly problematic. white mutations, which are often present in Drosophila strains, have dramatic effects on the metabolome (Sasaki et al., 2021), and all studies using transgenes containing mini-white must account for the possibility of differential white expression. But beyond the standard array of background mutations used for Drosophila genetics, there is tremendous variability among metabolite levels in individual strains, as evidenced by metabolomic studies of large collections of inbred strains (Rohde et al., 2021; Zhou et al., 2020). Notably, of 483 metabolites examined in Zhou et al. (2020), the steady-state levels of 381 metabolites significantly varied across 40 DGRP lines. Thus, metabolomic studies must carefully account for genetic background effects.
Using Metabolomics to Link Chemical Exposure With Phenotypic Outcomes
Metabolomics provides a unique approach to identify the metabolic mechanisms disrupted by toxicant exposure. Unlike RNA sequencing and most proteomic data, changes in the metabolome can occur independent of transcription, translation, and protein turnover; thus, metabolomics enables discoveries that would be missed by other technologies. An example of such a discovery can be found in Drosophila larvae lacking lactate dehydrogenase (Ldh), where elevated glycerol-3-phosphate (G3P) production partially compensates for the loss of Ldh activity (Li et al., 2019). Metabolomics was key in discovering this mechanism; G3P dehydrogenase, the primary enzyme responsible for synthesizing G3P, is expressed at normal levels in Ldh mutants. This finding is of direct relevance to toxicology studies where a compound of interest could directly inhibit a specific enzyme or metabolic pathway. If the inhibition of a specific enzyme can be compensated for by simply redirecting metabolic flux into a parallel pathway with excess enzymatic capacity, the underlying mechanism would be missed by gene expression analysis and only be detectable using metabolomics.
A number of user-friendly tools are available for analyzing metabolomics data. In this regard, we would highlight the online tool Metaboanalyst (Pang et al., 2021), which is easily accessible to the novice user, provides online tutorials and training data, and enables studies ranging from simple statistical studies to pathway-enrichment analysis and biomarker discovery (see Current Protocols article: Chong et al., 2019). This tool is well suited for analyzing fly data and has been used in several Drosophila metabolomics studies (for examples see Li et al., 2019). Just as gene enrichment analysis can implicate individual transcription factors or signal transduction pathways in a cellular response, so too can tools such as Metaboanalyst highlight trends within a metabolomics dataset that can be used to identify the pathways disrupted by a chemical exposure.
There is no single approach for inferring the metabolic targets of a toxicant based on metabolomics data. Ideally, such data are interpreted in the context of complementary 'omics data and, when possible, known effects of the studied compound on Drosophila and other organisms. In this regard, most metabolomic studies of exposed Drosophila will likely include metabolite changes that reflect the activation of general xenobiotic and stress-response pathways, which drive detoxification and removal of the toxicant. For example, induction of expression and activation of GSTs (described above) would, in theory, drive changes in the levels of reduced and oxidized glutathione, metabolites used to synthesize glutathione, and NADPH production. Although there are currently insufficient data from Drosophila toxicologic studies to infer exactly which metabolites are involved in such a generalized response, the production of future toxicologic datasets should help define such metabolomic changes.
Beyond the metabolite changes associated with a generalized stress response, metabolomics datasets hold the potential to pinpoint disruption of a single enzyme or metabolic pathway. For example, any molecule that directly inhibits a metabolic enzyme or small molecule transporter can potentially induce a rapid buildup of upstream metabolites and a corresponding decrease of downstream molecules. Moreover, cells often react to such inhibition by activating alternative metabolic pathways that compensate for the loss of the target enzyme or transporter. Although there are few metabolomic studies that serve as examples of how toxicants can inhibit Drosophila metabolic pathways in this manner, studies of the Drosophila gene lysine ketoglutarate reductase/saccharopine dehydrogenase (LKRSDH) provide an ideal case study (St Clair et al., 2017). Mutations in the LKRSDH gene induce buildup of the immediately upstream metabolites lysine and saccharopine, depletion of the downstream metabolite 2-aminoadipate, and increase of metabolites levels in two parallel pathways (i.e., pipecolate and 5-aminopentanoate). When such trends emerge from a dataset, the candidate enzyme can be studied using standard loss-of-function and gain-of-function approaches described elsewhere in this review.
KEY FUTURE DIRECTIONS AT THE INTERSECTION OF METABOLOMICS AND DROSOPHILA TOXICOLOGY
Although relatively few documented Drosophila toxicology studies have used metabolomic approaches, there is significant potential to redefine chemical safety studies in the fly using this technology. In this regard, the true power of conducting studies using Drosophila is that the large collection of genetic tools enables rapid mechanistic studies. As described throughout this review, both the DGRP collection (Mackay et al., 2012) and Drosophila Synthetic Population Resource collection (King et al., 2012) will be essential for studying toxicant responses in the fly. In an ideal experiment, dozens or hundreds of these strains would be exposed to a specific toxicant and subsequently analyzed using metabolomics. These data, when interpreted in light of genomic sequencing data and RNA sequencing, would be used to identify candidate target genes, which could then be manipulated using the vast UAS, RNAi, and CRISPR lines and characterized using metabolomics. Unfortunately, a combination of cost- and labor-intensive methodology has historically limited high-throughput metabolomics methods. However, the recent development of high-throughput metabolomic techniques, such as those used to analyze cultured cells and human blood (Malinowska et al., 2022; Nemkov et al., 2022), hold the potential to redefine metabolomics studies in the fly.
CONCLUDING REMARKS
Drosophila presents an attractive alternative to mammalian systems for toxicologic studies. Anatomic, physiologic, and behavioral effects of toxicants can be studied on the same genotypes at different developmental stages under controlled environmental conditions, and this can be done rapidly, economically, and without regulatory restrictions. Multiomics approaches that combine genome-wide association studies, transcriptomics, single-cell RNA sequencing, and metabolomics can identify cellular processes and metabolic pathways that are perturbed by toxic exposure and can yield mechanistic insights into responses to toxicants. Evolutionary conservation of fundamental biological processes renders findings from environmental toxicity in Drosophila relevant to human populations.
AUTHOR CONTRIBUTIONS
Matthew Rand : conceptualization, funding acquisition, supervision, writing–review and editing; Jason Tennessen : conceptualization, funding acquisition, writing–review and editing; Trudy Mackay : funding acquisition, writing–review and editing; Robert Anholt : conceptualization, funding acquisition, writing–review and editing.
CONFLICT OF INTEREST
The authors declare no conflicts of interests.
Open Research
DATA AVAILABILITY STATEMENT
The data that support the findings of this study are available from the corresponding author upon reasonable request.
LITERATURE CITED
- Abolaji, A. O., Kamden, J. P., Farombi, E. O., & Rocha, J. B. T. (2013). Drosophila melanogaster as a promising model organism in toxicological studies. Archives of Basic and Applied Medicine , 1, 33–38.
- Akins, J. M., Schroeder, J. A., Brower, D. L., & Aposhian, H. V. (1992). Evaluation of Drosophila melanogaster as an alternative animal for studying the neurotoxicity of heavy metals. Biometals , 5(2), 111–120. https://doi.org/10.1007/BF01062222
- Aleman-Meza, B., Jung, S. K., & Zhong, W. (2015). An automated system for quantitative analysis of Drosophila larval locomotion. BMC Developmental Biology , 15, 11. https://doi.org/10.1186/s12861-015-0062-0
- An, P. N., Yamaguchi, M., Bamba, T., & Fukusaki, E. (2014). Metabolome analysis of Drosophila melanogaster during embryogenesis. PLoS ONE , 9(8), e99519. https://doi.org/10.1371/journal.pone.0099519
- Anholt, R. R. H. (2020). Chemosensation and evolution of Drosophila host plant selection. iScience , 23(1), 100799. https://doi.org/10.1016/j.isci.2019.100799
- Anholt, R. R. H., & Mackay, T. F. C. (2018). The road less traveled: From genotype to phenotype in flies and humans. Mammalian Genome , 29(1–2), 5–23. https://doi.org/10.1007/s00335-017-9722-7
- Arguello, J. R., Sellanes, C., Lou, Y. R., & Raguso, R. A. (2013). Can yeast (S. cerevisiae) metabolic volatiles provide polymorphic signaling? PLoS ONE , 8(8), e70219. https://doi.org/10.1371/journal.pone.0070219
- Arking, R., Buck, S., Berrios, A., Dwyer, S., & Baker, G. T. 3rd (1991). Elevated paraquat resistance can be used as a bioassay for longevity in a genetically based long-lived strain of Drosophila. Developmental Genetics , 12(5), 362–370. https://doi.org/10.1002/dvg.1020120505
- Arya, G. H., Magwire, M. M., Huang, W., Serrano-Negron, Y. L., Mackay, T. F. C, & Anholt, R. R. H. (2015). The genetic basis for variation in olfactory behavior in Drosophila melanogaster. Chemical Senses , 40(4), 233–243. https://doi.org/10.1093/chemse/bjv001
- Ayroles, J. F., Carbone, M. A., Stone, E. A., Jordan, K. W., Lyman, R. F., Magwire, M. M., Rollmann, S. M., Duncan, L. H., Lawrence, F., Anholt, R. R. H, & Mackay, T. F. C. (2009). Systems genetics of complex traits in Drosophila melanogaster. Nature Genetics , 41(3), 299–307. https://doi.org/10.1038/ng.332
- Bainton, R. J., Tsai, L. T., Singh, C. M., Moore, M. S., Neckameyer, W. S., & Heberlein, U. (2000). Dopamine modulates acute responses to cocaine, nicotine and ethanol in Drosophila. Current Biology , 10(4), 187–194. https://doi.org/10.1016/s0960-9822(00)00336-5
- Baker, B. M., Carbone, M. A., Huang, W., Anholt, R. R. H., & Mackay, T. F. C. (2021). Genetic basis of variation in cocaine and methamphetamine consumption in outbred populations of Drosophila melanogaster. Proceedings of the National Academy of Sciences of the United States of America , 118(23), e2104131118. https://doi.org/10.1073/pnas.2104131118
- Baker, B. M., Mokashi, S. S., Shankar, V., Hatfield, J. S., Hannah, R. C., Mackay, T. F. C., & Anholt, R. R. H. (2021). The Drosophila brain on cocaine at single-cell resolution. Genome Research , 31(10), 1927–1937. https://doi.org/10.1101/gr.268037.120
- Banerjee, S., Venkatesan, A., & Bhat, M. A. (2017). Neurexin, neuroligin and wishful thinking coordinate synaptic cytoarchitecture and growth at neuromuscular junctions. Molecular and Cellular Neuroscience , 78, 9–24. https://doi.org/10.1016/j.mcn.2016.11.004
- Barthmaier, P., & Fyrberg, E. A. (1995). Monitoring development and pathology of Drosophila indirect flight muscles using green fluorescent protein. Developmental Biology , 169, 770–774. https://doi.org/10.1006/dbio.1995.1186
- Battlay, P., Leblanc, P. B., Green, L., Garud, N. R., Schmidt, J. M., Fournier-Level, A., & Robin, C. (2018). Structural variants and selective sweep foci contribute to insecticide resistance in the Drosophila genetic reference panel. G3 , 8(11), 3489–3497. https://doi.org/10.1534/g3.118.200619
- Beamish, C. R., Love, T. M., & Rand, M. D. (2021). Developmental toxicology of metal mixtures in Drosophila: Unique properties of potency and interactions of mercury isoforms. International Journal of Molecular Sciences , 22(22), 12131. https://doi.org/10.3390/ijms222212131
- Benzer, S. (1967). Behavioral mutants of Drosophila isolated by countercurrent distribution. Proceedings of the National Academy of Sciences of the United States of America , 58(3), 1112–1119. https://doi.org/10.1073/pnas.58.3.1112
- Benzer, S. (1973). Genetic dissection of behavior. Scientific American , 229(6), 24–37. https://doi.org/10.1038/scientificamerican1273-24
- Bertrand, M., Decoville, M., Meudal, H., Birman, S., & Landon, C. (2020). Metabolomic nuclear magnetic resonance studies at presymptomatic and symptomatic stages of Huntington's disease on a Drosophila model. Journal of Proteome Research , 19(10), 4034–4045. https://doi.org/10.1021/acs.jproteome.0c00335
- Borue, X., Condron, B., & Venton, B. J. (2010). Both synthesis and reuptake are critical for replenishing the releasable serotonin pool in Drosophila. Journal of Neurochemistry , 113(1), 188–199. https://doi.org/10.1111/j.1471-4159.2010.06588.x
- Borue, X., Cooper, S., Hirsh, J., Condron, B., & Venton, B. J. (2009). Quantitative evaluation of serotonin release and clearance in Drosophila. Journal of Neuroscience Methods , 179(2), 300–308. https://doi.org/10.1016/j.jneumeth.2009.02.013
- Boyd, E. S., Lange, R. K., Mitchell, A. C., Havig, J. R., Hamilton, T. L., Lafreniere, M. J., Shock, E. L., Peters, J. W., & Skidmore, M. (2011). Diversity, abundance, and potential activity of nitrifying and nitrate-reducing microbial assemblages in a subglacial ecosystem. Applied and Environmental Microbiology , 77(14), 4778–4787. https://doi.org/10.1128/AEM.00376-11
- Brand, A. H., & Perrimon, N. (1993). Targeted gene expression as a means of altering cell fates and generating dominant phenotypes. Development , 118(2), 401–415. https://doi.org/10.1242/dev.118.2.401
- Brinzer, R. A., Henderson, L., Marchiondo, A. A., Woods, D. J., Davies, S. A., & Dow, J. A. T. (2015). Metabolomic profiling of permethrin-treated Drosophila melanogaster identifies a role for tryptophan catabolism in insecticide survival. Insect Biochemistry and Molecular Biology , 67, 74–86. https://doi.org/10.1016/j.ibmb.2015.09.009
- Carbone, M. A., Yamamoto, A., Huang, W., Lyman, R. A., Meadors, T. B., Yamamoto, R., Anholt, R. R. H., & Mackay, T. F. C. (2016). Genetic architecture of natural variation in visual senescence in Drosophila. Proceedings of the National Academy of Sciences of the United States of America , 113(43), E6620–E6629. https://doi.org/10.1073/pnas.1613833113
- Carvalho, M., Sampaio, J. L., Palm, W., Brankatschk, M., Eaton, S., & Shevchenko, A. (2012). Effects of diet and development on the Drosophila lipidome. Molecular Systems Biology , 8, 600. https://doi.org/10.1038/msb.2012.29
- Chen, J., Su, Y., Lin, F., Iqbal, M., Mehmood, K., Zhang, H., & Shi, D. (2021). Effect of paraquat on cytotoxicity involved in oxidative stress and inflammatory reaction: A review of mechanisms and ecological implications. Ecotoxicology and Environmental Safety , 224, 112711. https://doi.org/10.1016/j.ecoenv.2021.112711
- Chi, W., Liu, W., Fu, W., Xia, S., Heckscher, E. S., & Zhuang, X. (2021). RNA-binding protein syncrip regulates starvation-induced hyperactivity in adult Drosophila. PLoS Genetics , 17(2), e1009396. https://doi.org/10.1371/journal.pgen.1009396
- Chifiriuc, M. C., Ratiu, A. C., Popa, M., & Ecovoiu, A. A. (2016). Drosophotoxicology: An emerging research area for assessing nanoparticles interaction with living organisms. International Journal of Molecular Sciences , 17(2), 36. https://doi.org/10.3390/ijms17020036
- Chintapalli, V. R., Al Bratty, M., Korzekwa, D., Watson, D. G., & Dow, J. A. (2013). Mapping an atlas of tissue-specific Drosophila melanogaster metabolomes by high resolution mass spectrometry. PLoS ONE , 8(10), e78066. https://doi.org/10.1371/journal.pone.0078066
- Chong, J., Wishart, D. S., & Xia, J. (2019). Using MetaboAnalyst 4.0 for comprehensive and integrative metabolomics data analysis. Current Protocols in Bioinformatics , 68, e86. https://doi.org/10.1002/cpbi.86
- Chung See, W. Z., Naidu, R., & Tang, K. S. (2023). Paraquat and Parkinson's disease: The molecular crosstalk of upstream signal transduction pathways leading to apoptosis. Current Neuropharmacology , [online ahead of print]. https://doi.org/10.2174/1570159x21666230126161524
- Cichewicz, K., & Hirsh, J. (2018). ShinyR-DAM: A program analyzing Drosophila activity, sleep and circadian rhythms. Communications Biology , 1, 25. https://doi.org/10.1038/s42003-018-0031-9
- Colbourne, J. K., Shaw, J. R., Sostare, E., Rivetti, C., Derelle, R., Barnett, R., Campos, B., LaLone, C., Viant, M. R., & Hodges, G. (2022). Toxicity by descent: A comparative approach for chemical hazard assessment. Environmental Advances , 9, 100287. https://doi.org/10.1016/j.envadv.2022.100287
- Corey, J. L., Quick, M. W., Davidson, N., Lester, H. A., & Guastella, J. (1994). A cocaine-sensitive Drosophila serotonin transporter: Cloning, expression, and electrophysiological characterization. Proceedings of the National Academy of Sciences of the United States of America , 91(3), 1188–1192. https://doi.org/10.1073/pnas.91.3.1188
- Cormier, R. J., Strang, R., Menail, H., Touaibia, M., & Pichaud, N. (2021). Systemic and mitochondrial effects of metabolic inflexibility induced by high fat diet in Drosophila melanogaster. Insect Biochemistry and Molecular Biology , 133, 103556. https://doi.org/10.1016/j.ibmb.2021.103556
- Cox, J. E., Thummel, C. S., & Tennessen, J. M. (2017). Metabolomic studies in drosophila. Genetics , 206(3), 1169–1185. https://doi.org/10.1534/genetics.117.200014
- Daborn, P. J., Lumb, C., Boey, A., Wong, W., Ffrench-Constant, R. H., & Batterham, P. (2007). Evaluating the insecticide resistance potential of eight Drosophila melanogaster cytochrome P450 genes by transgenic over-expression. Insect Biochemistry and Molecular Biology , 37(5), 512–519. https://doi.org/10.1016/j.ibmb.2007.02.008
- Daly, M. J., Rioux, J. D., Schaffner, S. F., Hudson, T. J., & Lander, E. S. (2001). High-resolution haplotype structure in the human genome. Nature Genetics , 29(2), 229–232. https://doi.org/10.1038/ng1001-229
- Davie, K., Janssens, J., Koldere, D., de Waegeneer, M., Pech, U., Kreft, L., Aibar, S., Makhzami, S., Christiaens, V., Bravo Gonzalez-Blas, C., Poovathingal, S., Hulselmans, G., Spanier, K. I., Moerman, T., Vanspauwen, B., Geurs, S., Voet, T., Lammertyn, J., Thienpont, B., … Aerts, S. (2018). A single-cell transcriptome atlas of the aging Drosophila brain. Cell , 174(4), 982–998.e20. https://doi.org/10.1016/j.cell.2018.05.057
- Dembeck, L. M., Boroczky, K., Huang, W., Schal, C., Anholt, R. R., & Mackay, T. F. (2015). Genetic architecture of natural variation in cuticular hydrocarbon composition in Drosophila melanogaster. eLife , 4, e09861. https://doi.org/10.7554/eLife.09861
- Demchyshyn, L. L., Pristupa, Z. B., Sugamori, K. S., Barker, E. L., Blakely, R. D., Wolfgang, W. J., Forte, M. A., & Niznik, H. B. (1994). Cloning, expression, and localization of a chloride-facilitated, cocaine-sensitive serotonin transporter from Drosophila melanogaster. Proceedings of the National Academy of Sciences of the United States of America , 91(11), 5158–5162. https://doi.org/10.1073/pnas.91.11.5158
- Demir, E. (2020). Drosophila as a model for assessing nanopesticide toxicity. Nanotoxicology , 14(9), 1271–1279. https://doi.org/10.1080/17435390.2020.1815886
- Dermauw, W., & van Leeuwen, T. (2014). The ABC gene family in arthropods: Comparative genomics and role in insecticide transport and resistance. Insect Biochemistry and Molecular Biology , 45, 89–110. https://doi.org/10.1016/j.ibmb.2013.11.001
- Diegelmann, S., Jansen, A., Jois, S., Kastenholz, K., Velo Escarcena, L., Strudthoff, N., & Scholz, H. (2017). The CApillary FEeder assay measures food intake in Drosophila melanogaster. Journal of Visualized Experiments , 121, e55024. https://doi.org/10.3791/55024
- Dubnau, J., & Tully, T. (1998). Gene discovery in Drosophila: New insights for learning and memory. Annual Review of Neuroscience , 21, 407–444. https://doi.org/10.1146/annurev.neuro.21.1.407
- El Kholy, S., & Al Naggar, Y. (2023). Exposure to polystyrene microplastic beads causes sex-specific toxic effects in the model insect Drosophila melanogaster. Scientific Reports , 13(1), 204. https://doi.org/10.1038/s41598-022-27284-7
- Engel, G. L., & Rand, M. D. (2014). The Notch target E(spl)mdelta is a muscle-specific gene involved in methylmercury toxicity in motor neuron development. Neurotoxicology and Teratology , 43, 11–18. https://doi.org/10.1016/j.ntt.2014.03.001
- Everett, L. J., Huang, W., Zhou, S., Carbone, M. A., Lyman, R. F., Arya, G. H., Geisz, M. S., Ma, J., Morgante, F., St Armour, G., Turlapati, L., Anholt, R. R. H., & Mackay, T. F. C. (2020). Gene expression networks in the Drosophila genetic reference panel. Genome Research , 30(3), 485–496. https://doi.org/10.1101/gr.257592.119
- Ferveur, J. F. (2010). Drosophila female courtship and mating behaviors: Sensory signals, genes, neural structures and evolution. Current Opinion in Neurobiology , 20(6), 764–769. https://doi.org/10.1016/j.conb.2010.09.007
- Filosevic, A., Al-Samarai, S., & Andretic Waldowski, R. (2018). High throughput measurement of locomotor sensitization to volatilized cocaine in Drosophila melanogaster. Frontiers in Molecular Neuroscience , 11, 25. https://doi.org/10.3389/fnmol.2018.00025
- Garlapow, M. E., Everett, L. J., Zhou, S., Gearhart, A. W., Fay, K. A., Huang, W., Morozova, T. V., Arya, G. H., Turlapati, L., St Armour, G., Hussain, Y. N., McAdams, S. E., Fochler, S., & Mackay, T. F. C. (2017). Genetic and genomic response to selection for food consumption in Drosophila melanogaster. Behavior Genetics , 47(2), 227–243. https://doi.org/10.1007/s10519-016-9819-x
- Gerber, B., & Stocker, R. F. (2007). The Drosophila larva as a model for studying chemosensation and chemosensory learning: A review. Chemical Senses , 32(1), 65–89. https://doi.org/10.1093/chemse/bjl030
- Gerber, B., Stocker, R. F., Tanimura, T., & Thum, A. S. (2009). Smelling, tasting, learning: Drosophila as a study case. Results and Problems in Cell Differentiation , 47, 139–185. https://doi.org/10.1007/400_2008_9
- Ghaemi, R., Arefi, P., Stosic, A., Acker, M., Raza, Q., Roger Jacobs, J., & Selvaganapathy, P. R. (2017). A microfluidic microinjector for toxicological and developmental studies in Drosophila embryos. Lab on A Chip , 17(22), 3898–3908. https://doi.org/10.1039/c7lc00537g
- Guarnieri, D. J., & Heberlein, U. (2003). Drosophila melanogaster , a genetic model system for alcohol research. International Review of Neurobiology , 54, 199–228. https://doi.org/10.1016/s0074-7742(03)54006-5
- Gunderson, J. T., Peppriell, A. E., Krout, I. N., Vorojeikina, D., & Rand, M. D. (2021). Neuroligin-1 is a mediator of methylmercury neuromuscular toxicity. Toxicological Sciences , 184(2), 236–251. https://doi.org/10.1093/toxsci/kfab114
- Gunderson, J. T., Peppriell, A. E., Vorojeikina, D., & Rand, M. D. (2020). Tissue-specific Nrf2 signaling protects against methylmercury toxicity in Drosophila neuromuscular development. Archives of Toxicology , 94(12), 4007–4022. https://doi.org/10.1007/s00204-020-02879-z
- Guryev, V., Smits, B. M., van de Belt, J., Verheul, M., Hubner, N., & Cuppen, E. (2006). Haplotype block structure is conserved across mammals. PLoS Genetics , 2(7), e121. https://doi.org/10.1371/journal.pgen.0020121
- Harbison, S. T., Kumar, S., Huang, W., McCoy, L. J., Smith, K. R., & Mackay, T. F. C. (2019). Genome-wide association study of circadian behavior in Drosophila melanogaster. Behavior Genetics , 49(1), 60–82. https://doi.org/10.1007/s10519-018-9932-0
- Harbison, S. T., McCoy, L. J., & Mackay, T. F. C. (2013). Genome-wide association study of sleep in Drosophila melanogaster. BMC Genomics , 14, 281. https://doi.org/10.1186/1471-2164-14-281
- Hendricks, J. C., Finn, S. M., Panckeri, K. A., Chavkin, J., Williams, J. A., Sehgal, A., & Pack, A. I. (2000). Rest in Drosophila is a sleep-like state. Neuron , 25(1), 129–138. https://doi.org/10.1016/s0896-6273(00)80877-6
- Highfill, C. A., Baker, B. M., Stevens, S. D., Anholt, R. R. H., & Mackay, T. F. C. (2019). Genetics of cocaine and methamphetamine consumption and preference in Drosophila melanogaster. PLoS Genetics , 15(5), e1007834. https://doi.org/10.1371/journal.pgen.1007834
- Hirsch, H. V., Possidente, D., Averill, S., Despain, T. P., Buytkins, J., Thomas, V., Goebel, W. P., Shipp-Hilts, A., Wilson, D., Hollocher, K., Possidente, B., Lnenicka, G., & Ruden, D. M. (2009). Variations at a quantitative trait locus (QTL) affect development of behavior in lead-exposed Drosophila melanogaster. Neurotoxicology , 30(2), 305–311. https://doi.org/10.1016/j.neuro.2009.01.004
- Hofbauer, H. F., Heier, C., Sen Saji, A. K., & Kühnlein, R. P. (2021). Lipidome remodeling in aging normal and genetically obese Drosophila males. Insect Biochemistry and Molecular Biology , 133, 103498. https://doi.org/10.1016/j.ibmb.2020.103498
- Hoffmann, J. A. (2003). The immune response of Drosophila. Nature , 426(6962), 33–38. https://doi.org/10.1038/nature02021
- Holsopple, J. M., Smoot, S. R., Popodi, E. M., Colbourne, J. K., Shaw, J. R., Oliver, B., Kaufman, T. C., & Tennessen, J. M. (2023). Assessment of chemical toxicity in adult Drosophila melanogaster. Journal of Visualized Experiments , 193, e65029. https://doi.org/10.3791/65029
- Huang, W., Campbell, T., Carbone, M. A., Jones, W. E., Unselt, D., Anholt, R. R. H., & Mackay, T. F. C. (2020). Context-dependent genetic architecture of Drosophila life span. PLoS Biology , 18(3), e3000645. https://doi.org/10.1371/journal.pbio.3000645
- Huang, W., Carbone, M. A., Magwire, M. M., Peiffer, J. A., Lyman, R. F., Stone, E. A., Anholt, R. R H., & Mackay, T. F. C. (2015). Genetic basis of transcriptome diversity in Drosophila melanogaster. Proceedings of the National Academy of Sciences of the United States of America , 112(44), E6010–6019. https://doi.org/10.1073/pnas.1519159112
- Huang, W., Massouras, A., Inoue, Y., Peiffer, J., Ramia, M., Tarone, A. M., Turlapati, L., Zichner, T., Zhu, D., Lyman, R. F., Magwire, M. M., Blankenburg, K., Carbone, M. A., Chang, K., Ellis, L. L., Fernandez, S., Han, Y., Highnam, G., Hjelmen, C. E., … Mackay, T. F. C. (2014). Natural variation in genome architecture among 205 Drosophila melanogaster genetic reference panel lines. Genome Research , 24(7), 1193–1208. https://doi.org/10.1101/gr.171546.113
- Huang, W., Richards, S., Carbone, M. A., Zhu, D., Anholt, R. R. H., Ayroles, J. F., Duncan, L., Jordan, K. W., Lawrence, F., Magwire, M. M., Warner, C. B., Blankenburg, K., Han, Y., Javaid, M., Jayaseelan, J., Jhangiani, S. N., Muzny, D., Ongeri, F., Perales, L., … Mackay, T. F. C. (2012). Epistasis dominates the genetic architecture of Drosophila quantitative traits. Proceedings of the National Academy of Sciences of the United States of America , 109(39), 15553–15559. https://doi.org/10.1073/pnas.1213423109
- Huggett, S. B., Hatfield, J. S., Walters, J. D., McGeary, J. E., Welsh, J. W., Mackay, T. F. C., Anholt, R. R. H., & Palmer, R. H. C. (2021). Ibrutinib as a potential therapeutic for cocaine use disorder. Transl Psychiatry , 11(1), 623. https://doi.org/10.1038/s41398-021-01737-5
- Inamdar, A. A., Zaman, T., Morath, S. U., Pu, D. C., & Bennett, J. W. (2014). Drosophila melanogaster as a model to characterize fungal volatile organic compounds. Environmental Toxicology , 29(7), 829–836. https://doi.org/10.1002/tox.21825
- Ja, W. W., Carvalho, G. B., Mak, E. M., de la Rosa, N. N., Fang, A. Y., Liong, J. C., Brummel, T., & Benzer, S. (2007). Prandiology of Drosophila and the CAFE assay. Proceedings of the National Academy of Sciences of the United States of America , 104(20), 8253–8256. https://doi.org/10.1073/pnas.0702726104
- Jaime, M., Salem, G. H., Martinez, D. J., Karott, S., Flores, A., Palmer, C. D., Mahadevaraju, S., Krynitsky, J., Garmendia-Cedillos, M., Anderson, S., Harbison, S., Pohida, T. J., Ludington, W. B., & Oliver, B. (2023). Whole Animal Feeding FLat (WAFFL): A complete and comprehensive validation of a novel, high-throughput fly experimentation system. G3 , 13(3), jkad012. https://doi.org/10.1093/g3journal/jkad012
- Jin, K., Wilson, K. A., Beck, J. N., Nelson, C. S., Brownridge, G. W., 3rd, Harrison, B. R., Djukovic, D., Raftery, D., Brem, R. B., Yu, S., Drton, M., Shojaie, A., Kapahi, P., & Promislow, D. (2020). Genetic and metabolomic architecture of variation in diet restriction-mediated lifespan extension in Drosophila. PLoS Genetics , 16(7), e1008835. https://doi.org/10.1371/journal.pgen.1008835
- Johnstone, O., & Lasko, P. (2001). Translational regulation and RNA localization in Drosophila oocytes and embryos. Annual Review of Genetics , 35, 365–406. https://doi.org/10.1146/annurev.genet.35.102401.090756
- Jordan, K. W., Craver, K. L., Magwire, M. M., Cubilla, C. E., Mackay, T. F. C., & Anholt, R. R. H. (2012). Genome-wide association for sensitivity to chronic oxidative stress in Drosophila melanogaster. PLoS ONE , 7(6), e38722. https://doi.org/10.1371/journal.pone.0038722
- Jouandin, P., Marelja, Z., Shih, Y.-H., Parkhitko, A. A., Dambowsky, M., Asara, J. M., Nemazanyy, I., Dibble, C. C., Simons, M., & Perrimon, N. (2022). Lysosomal cystine mobilization shapes the response of TORC1 and tissue growth to fasting. Science , 375(6582), eabc4203. https://doi.org/10.1126/science.abc4203
- Kaun, K. R., Devineni, A. V., & Heberlein, U. (2012). Drosophila melanogaster as a model to study drug addiction. Human Genetics , 131(6), 959–975. https://doi.org/10.1007/s00439-012-1146-6
- King, E. G., Merkes, C. M., McNeil, C. L., Hoofer, S. R., Sen, S., Broman, K. W., Long, A. D., & Macdonald, S. J. (2012). Genetic dissection of a model complex trait using the Drosophila Synthetic Population Resource. Genome Research , 22(8), 1558–1566. https://doi.org/10.1101/gr.134031.111
- C. D. Klaassen (Ed.), (2013). Casarett and Doull's toxicology: The basic science of poisons ( 8th ed.). McGraw Hill.
- Klaassen, C. D., Liu, J., & Diwan, B. A. (2009). Metallothionein protection of cadmium toxicity. Toxicology and Applied Pharmacology , 238(3), 215–220. https://doi.org/10.1016/j.taap.2009.03.026
- Konopka, R. J., & Benzer, S. (1971). Clock mutants of Drosophila melanogaster. Proceedings of the National Academy of Sciences of the United States of America , 68(9), 2112–2116. https://doi.org/10.1073/pnas.68.9.2112
- Kurata, S., Go, M. J., Artavanis-Tsakonas, S., & Gehring, W. J. (2000). Notch signaling and the determination of appendage identity. Proceedings of the National Academy of Sciences of the United States of America , 97(5), 2117–22. https://doi.org/10.1073/pnas.040556497
- le Goff, G., Hilliou, F., Siegfried, B. D., Boundy, S., Wajnberg, E., Sofer, L., Audant, P., Ffrench-Constant, R. H., & Feyereisen, R. (2006). Xenobiotic response in Drosophila melanogaster : Sex dependence of P450 and GST gene induction. Insect Biochemistry and Molecular Biology , 36(8), 674–682. https://doi.org/10.1016/j.ibmb.2006.05.009
- Li, H., Chaney, S., Roberts, I. J., Forte, M., & Hirsh, J. (2000). Ectopic G-protein expression in dopamine and serotonin neurons blocks cocaine sensitization in Drosophila melanogaster. Current Biology , 10(4), 211–214. https://doi.org/10.1016/s0960-9822(00)00340-7
- Li, H., Chawla, G., Hurlburt, A. J., Sterrett, M. C., Zaslaver, O., Cox, J., Karty, J. A., Rosebrock, A. P., Caudy, A. A., & Tennessen, J. M. (2017). Drosophila larvae synthesize the putative oncometabolite L-2-hydroxyglutarate during normal developmental growth. Proceedings of the National Academy of Sciences of the United States of America , 114(6), 1353–1358. https://doi.org/10.1073/pnas.1614102114
- Li, H., Rai, M., Buddika, K., Sterrett, M. C., Luhur, A., Mahmoudzadeh, N. H., Julick, C. R., Pletcher, R. C., Chawla, G., Gosney, C. J., Burton, A. K., Karty, J. A., Montooth, K. L., Sokol, N. S., & Tennessen, J. M. (2019). Lactate dehydrogenase and glycerol-3-phosphate dehydrogenase cooperatively regulate growth and carbohydrate metabolism during Drosophila melanogaster larval development. Development , 146(17), dev175315. https://doi.org/10.1242/dev.175315
- Li, H., & Tennessen, J. M. (2018). Preparation of Drosophila larval samples for gas chromatography-mass spectrometry (GC-MS)-based metabolomics. Journal of Visualized Experiments , 136, e57847. https://doi.org/10.3791/57847
- Li, J. Q., Fang, J. S., Qin, X. M., & Gao, L. (2020). Metabolomics profiling reveals the mechanism of caffeic acid in extending lifespan in Drosophila melanogaster. Food & Function, 11(9), 8202–8213. https://doi.org/10.1039/d0fo01332c
- Li, X., Li, Z., Shen, H., Zhao, H., Qin, G., & Xue, J. (2022). Effects of long-term and low-concentration exposures of benzene and formaldehyde on mortality of Drosophila melanogaster. Environmental Pollution , 300, 118924. https://doi.org/10.1016/j.envpol.2022.118924
- Lovejoy, P. C., Foley, K. E., Conti, M. M., Meadows, S. M., Bishop, C., & Fiumera, A. C. (2021). Genetic basis of susceptibility to low-dose paraquat and variation between the sexes in Drosophila melanogaster. Molecular Ecology , 30(9), 2040–2053. https://doi.org/10.1111/mec.15878
- Mackay, T. F. C. (2002). The nature of quantitative genetic variation for Drosophila longevity. Mechanisms of Ageing and Development , 123(2–3), 95–104. https://doi.org/10.1016/s0047-6374(01)00330-x
- Mackay, T. F. C., Richards, S., Stone, E. A., Barbadilla, A., Ayroles, J. F., Zhu, D., Casillas, S., Han, Y., Magwire, M. M., Cridland, J. M., Richardson, M. F., Anholt, R. R. H., Barron, M., Bess, C., Blankenburg, K. P., Carbone, M. A., Castellano, D., Chaboub, L., Duncan, L., … Gibbs, R. A. (2012). The Drosophila melanogaster genetic reference panel. Nature , 482(7384), 173–178. https://doi.org/10.1038/nature10811
- Mackenzie, P. I., Owens, I. S., Burchell, B., Bock, K. W., Bairoch, A., Belanger, A., Fournel-Gigleux, S., Green, M., Hum, D. W., Iyanagi, T., Lancet, D., Louisot, P., Magdalou, J., Chowdhury, J. R., Ritter, J. K., Schachter, H., Tephly, T. R., Tipton, K. F., & Nebert, D. W. (1997). The UDP glycosyltransferase gene superfamily: Recommended nomenclature update based on evolutionary divergence. Pharmacogenetics , 7(4), 255–269. https://doi.org/10.1097/00008571-199708000-00001
- Magnusson, J., & Ramel, C. (1986). Genetic variation in the susceptibility to mercury and other metal compounds in Drosophila melanogaster. Teratogenesis, Carcinogenesis, and Mutagenesis , 6(4), 289–305. https://doi.org/10.1002/tcm.1770060405
- Mahapatra, C. T., Bond, J., Rand, D. M., & Rand, M. D. (2010). Identification of methylmercury tolerance gene candidates in Drosophila. Toxicological Sciences , 116(1), 225–238. https://doi.org/10.1093/toxsci/kfq097
- Malinowska, J. M., Palosaari, T., Sund, J., Carpi, D., Bouhifd, M., Weber, R. J. M., Whelan, M., & Viant, M. R. (2022). Integrating in vitro metabolomics with a 96-well high-throughput screening platform. Metabolomics , 18(1), 11. https://doi.org/10.1007/s11306-021-01867-3
- Manoli, D. S., Foss, M., Villella, A., Taylor, B. J., Hall, J. C., & Baker, B. S. (2005). Male-specific fruitless specifies the neural substrates of Drosophila courtship behaviour. Nature , 436(7049), 395–400. https://doi.org/10.1038/nature03859
- Martin, C. A., & Krantz, D. E. (2014). Drosophila melanogaster as a genetic model system to study neurotransmitter transporters. Neurochemistry International , 73, 71–88. https://doi.org/10.1016/j.neuint.2014.03.015
- Maslov, D. L., Zemskaya, N. V., Trifonova, O. P., Lichtenberg, S., Balashova, E. E., Lisitsa, A. V., Moskalev, A. A., & Lokhov, P. G. (2021). Comparative metabolomic study of Drosophila species with different lifespans. International Journal of Molecular Sciences , 22(23), 12873. https://doi.org/10.3390/ijms222312873
- McClung, C., & Hirsh, J. (1998). Stereotypic behavioral responses to free-base cocaine and the development of behavioral sensitization in Drosophila. Current Biology , 8(2), 109–112. https://doi.org/10.1016/s0960-9822(98)70041-7
- Menon, K. P., Carrillo, R. A., & Zinn, K. (2013). Development and plasticity of the Drosophila larval neuromuscular junction. Developmental Biology , 2(5), 647–670. https://doi.org/10.1002/wdev.108
- Misra, J. R., Horner, M. A., Lam, G., & Thummel, C. S. (2011). Transcriptional regulation of xenobiotic detoxification in Drosophila. Genes & Development, 25(17), 1796–1806. https://doi.org/10.1101/gad.17280911
- Mokashi, S. S., Shankar, V., MacPherson, R. A., Hannah, R. C., Mackay, T. F. C., & Anholt, R. R. H. (2021). Developmental alcohol exposure in Drosophila: Effects on adult phenotypes and gene expression in the brain. Frontiers in Psychiatry , 12, 699033. https://doi.org/10.3389/fpsyt.2021.699033
- Montgomery, S. L., Vorojeikina, D., Huang, W., Mackay, T. F. C., Anholt, R. R. H., & Rand, M. D. (2014). Genome-wide association analysis of tolerance to methylmercury toxicity in Drosophila implicates myogenic and neuromuscular developmental pathways. PLoS ONE , 9(10), e110375. https://doi.org/10.1371/journal.pone.0110375
- Morozova, T. V., Huang, W., Pray, V. A., Whitham, T., Anholt, R. R. H., & Mackay, T. F. C. (2015). Polymorphisms in early neurodevelopmental genes affect natural variation in alcohol sensitivity in adult Drosophila. BMC Genomics , 16, 865. https://doi.org/10.1186/s12864-015-2064-5
- Moulin, T. C., Covill, L. E., Itskov, P. M., Williams, M. J., & Schioth, H. B. (2021). Rodent and fly models in behavioral neuroscience: An evaluation of methodological advances, comparative research, and future perspectives. Neuroscience and Biobehavioral Reviews , 120, 1–12. https://doi.org/10.1016/j.neubiorev.2020.11.014
- Mu, Y., Hu, X., Yang, P., Sun, L., Gu, W., & Zhang, M. (2021). The effects of cadmium on the development of Drosophila and its transgenerational inheritance effects. Toxicology , 462, 152931. https://doi.org/10.1016/j.tox.2021.152931
- Nassel, D. R. (2018). Substrates for neuronal cotransmission with neuropeptides and small molecule neurotransmitters in Drosophila. Frontiers in Cellular Neuroscience , 12, 83. https://doi.org/10.3389/fncel.2018.00083
- Nelson, D. R., Koymans, L., Kamataki, T., Stegeman, J. J., Feyereisen, R., Waxman, D. J., Waterman, M. R., Gotoh, O., Coon, M. J., Estabrook, R. W., Gunsalus, I. C., & Nebert, D. W. (1996). P450 superfamily: Update on new sequences, gene mapping, accession numbers and nomenclature. Pharmacogenetics , 6(1), 1–42. https://doi.org/10.1097/00008571-199602000-00002
- Nemkov, T., Yoshida, T., Nikulina, M., & D'Alessandro, A. (2022). High-throughput metabolomics platform for the rapid data-driven development of novel additive solutions for blood storage. Frontiers in Physiology , 13, 833242. https://doi.org/10.3389/fphys.2022.833242
- Nishimura, T. (2020). Feedforward regulation of glucose metabolism by steroid hormones drives a developmental transition in Drosophila. Current Biology , 30(18), 3624–3632.e3625. https://doi.org/10.1016/j.cub.2020.06.043
- Olivares, G. H., Nunez-Villegas, F., Candia, N., Orostica, K., Gonzalez-Ramirez, M. C., Vega-Macaya, F., Zuniga, N., Molina, C., Oliva, C., Mackay, T. F. C., Verdugo, R. A., & Olguin, P. (2023). Early-life nutrition interacts with developmental genes to shape the brain and sleep behavior in Drosophila melanogaster. Sleep , 46(4), zsad016. https://doi.org/10.1093/sleep/zsad016
- Onalaja, A. O., & Claudio, L. (2000). Genetic susceptibility to lead poisoning. Environmental Health Perspectives , 108(Suppl 1), 23–28. https://doi.org/10.1289/ehp.00108s123
- Özsoy, E. D., Yilmaz, M., Patlar, B., Emecen, G., Durmaz, E., Magwire, M. M., Zhou, S., Huang, W., Anholt, R. R. H., & Mackay, T. F. C. (2021). Epistasis for head morphology in Drosophila melanogaster. G3 , 11(10), jkab285. https://doi.org/10.1093/g3journal/jkab285
- Pang, Z., Chong, J., Zhou, G., de Lima Morais, D. A., Chang, L., Barrette, M., Gauthier, C., Jacques, P., Li, S., & Xia, J. (2021). MetaboAnalyst 5.0: Narrowing the gap between raw spectra and functional insights. Nucleic Acids Research , 49(W1), W388–W396. https://doi.org/10.1093/nar/gkab382
- Parkhitko, A. A., Ramesh, D., Wang, L., Leshchiner, D., Filine, E., Binari, R., Olsen, A. L., Asara, J. M., Cracan, V., Rabinowitz, J. D., Brockmann, A., & Perrimon, N. (2020). Downregulation of the tyrosine degradation pathway extends Drosophila lifespan. eLife , 9, e58053. https://doi.org/10.7554/eLife.58053
- Peppriell, A. E., Gunderson, J. T., Krout, I. N., Vorojeikina, D., & Rand, M. D. (2021). Latent effects of early-life methylmercury exposure on motor function in Drosophila. Neurotoxicology and Teratology , 88, 107037. https://doi.org/10.1016/j.ntt.2021.107037
- Petrova, B., Liu, K., Tian, C., Kitaoka, M., Freinkman, E., Yang, J., & Orr-Weaver, T. L. (2018). Dynamic redox balance directs the oocyte-to-embryo transition via developmentally controlled reactive cysteine changes. Proceedings of the National Academy of Sciences of the United States of America , 115(34), E7978–E7986. https://doi.org/10.1073/pnas.1807918115
- Pfeiffer, B. D., Jenett, A., Hammonds, A. S., Ngo, T. T., Misra, S., Murphy, C., Scully, A., Carlson, J. W., Wan, K. H., Laverty, T. R., Mungall, C., Svirskas, R., Kadonaga, J. T., Doe, C. Q., Eisen, M. B., Celniker, S. E., & Rubin, G. M. (2008). Tools for neuroanatomy and neurogenetics in Drosophila. Proceedings of the National Academy of Sciences of the United States of America , 105(28), 9715–9720. https://doi.org/10.1073/pnas.0803697105
- Piper, M. D., Blanc, E., Leitão-Gonçalves, R., Yang, M., He, X., Linford, N. J., Hoddinott, M. P., Hopfen, C., Soultoukis, G. A., Niemeyer, C., Kerr, F., Pletcher, S. D., Ribeiro, C., & Partridge, L. (2014). A holidic medium for Drosophila melanogaster. Nature Methods , 11(1), 100–105. https://doi.org/10.1038/nmeth.2731
- Piper, M. D. W., & Partridge, L. (2018). Drosophila as a model for ageing. Biochimica et Biophysica Acta. Molecular Basis of Disease , 1864(9 Pt A), 2707–2717. https://doi.org/10.1016/j.bbadis.2017.09.016
- Prince, L. M., & Rand, M. D. (2017). Notch target gene E(spl)mdelta is a mediator of methylmercury-induced myotoxicity in Drosophila. Frontiers in Genetics , 8, 233. https://doi.org/10.3389/fgene.2017.00233
- Rand, M. D. (2010). Drosophotoxicology: The growing potential for Drosophila in neurotoxicology. Neurotoxicology and Teratology , 32(1), 74–83. https://doi.org/10.1016/j.ntt.2009.06.004
- Rand, M. D. (2014). A method of permeabilization of Drosophila embryos for assays of small molecule activity. Journal of Visualized Experiments , 89, e51634. https://doi.org/10.3791/51634
- Rand, M. D., Bland, C. E., & Bond, J. (2008). Methylmercury activates enhancer-of-split and bearded complex genes independent of the notch receptor. Toxicological Sciences , 104(1), 163–176. https://doi.org/10.1093/toxsci/kfn060
- Rand, M. D., Dao, J. C., & Clason, T. A. (2009). Methylmercury disruption of embryonic neural development in Drosophila. Neurotoxicology , 30(5), 794–802. https://doi.org/10.1016/j.neuro.2009.04.006
- Rand, M. D., Kearney, A. L., Dao, J., & Clason, T. (2010). Permeabilization of Drosophila embryos for introduction of small molecules. Insect Biochemistry and Molecular Biology , 40(11), 792–804. https://doi.org/10.1016/j.ibmb.2010.07.007
- Rand, M. D., Vorojeikina, D., Peppriell, A., Gunderson, J., & Prince, L. M. (2019). Drosophotoxicology: Elucidating kinetic and dynamic pathways of methylmercury toxicity in a Drosophila model. Frontiers in Genetics , 10, 666. https://doi.org/10.3389/fgene.2019.00666
- Rauckhorst, A. J., Borcherding, N., Pape, D. J., Kraus, A. S., Scerbo, D. A., & Taylor, E. B. (2022). Mouse tissue harvest-induced hypoxia rapidly alters the in vivo metabolome, between-genotype metabolite level differences, and 13C-tracing enrichments. Nature Metabolism , 66, 101596. https://doi.org/10.1016/j.molmet.2022.101596
- Reich, D. E., Cargill, M., Bolk, S., Ireland, J., Sabeti, P. C., Richter, D. J., Lavery, T., Kouyoumjian, R., Farhadian, S. F., Ward, R., & Lander, E. S. (2001). Linkage disequilibrium in the human genome. Nature , 411(6834), 1990–204. https://doi.org/10.1038/35075590
- Reis, T. (2016). Effects of synthetic diets enriched in specific nutrients on Drosophila development, body fat, and lifespan. PLoS ONE , 11(1), e0146758. https://doi.org/10.1371/journal.pone.0146758
- Ro, J., Harvanek, Z. M., & Pletcher, S. D. (2014). FLIC: High-throughput, continuous analysis of feeding behaviors in Drosophila. PLoS ONE , 9(6), e101107. https://doi.org/10.1371/journal.pone.0101107
- Rohde, P. D., Kristensen, T. N., Sarup, P., Muñoz, J., & Malmendal, A. (2021). Prediction of complex phenotypes using the Drosophila melanogaster metabolome. Heredity , 126(5), 717–732. https://doi.org/10.1038/s41437-021-00404-1
- Salminen, T. S., & Vale, P. F. (2020). Drosophila as a model system to investigate the effects of mitochondrial variation on innate immunity. Frontiers in Immunology , 11, 521. https://doi.org/10.3389/fimmu.2020.00521
- Sasaki, A., Nishimura, T., Takano, T., Naito, S., & Yoo, S. K. (2021). white regulates proliferative homeostasis of intestinal stem cells during ageing in Drosophila. Nature Metabolism , 3(4), 546–557. https://doi.org/10.1038/s42255-021-00375-x
- Sass, T. N., MacPherson, R. A., Mackay, T. F. C., & Anholt, R. R. H. (2020). High-throughput method for measuring alcohol sedation time of individual Drosophila melanogaster. Journal of Visualized Experiments , 158, e61108. https://doi.org/10.3791/61108
- Schwartz, B. S., Stewart, W. F., Kelsey, K. T., Simon, D., Park, S., Links, J. M., & Todd, A. C. (2000). Associations of tibial lead levels with BsmI polymorphisms in the vitamin D receptor in former organolead manufacturing workers. Environmental Health Perspectives , 108(3), 199–203. https://doi.org/10.1289/ehp.00108199
- Shaw, P. J., Cirelli, C., Greenspan, R. J., & Tononi, G. (2000). Correlates of sleep and waking in Drosophila melanogaster. Science , 287(5459), 1834–1837. https://doi.org/10.1126/science.287.5459.1834
- Shell, B. C., Luo, Y., Pletcher, S., & Grotewiel, M. (2021). Expansion and application of dye tracers for measuring solid food intake and food preference in Drosophila. Scientific Reports , 11(1), 20044. https://doi.org/10.1038/s41598-021-99483-7
- Shin, M., Copeland, J. M., & Venton, B. J. (2018). Drosophila as a model system for neurotransmitter measurements. ACS Chemical Neuroscience , 9(8), 1872–1883. https://doi.org/10.1021/acschemneuro.7b00456
- Shorter, J., Couch, C., Huang, W., Carbone, M. A., Peiffer, J., Anholt, R. R. H., & Mackay, T. F. C. (2015). Genetic architecture of natural variation in Drosophila melanogaster aggressive behavior. Proceedings of the National Academy of Sciences of the United States of America , 112(27), E3555–3563. https://doi.org/10.1073/pnas.1510104112
- Siddique, H. R., Gupta, S. C., Mitra, K., Bajpai, V. K., Mathur, N., Murthy, R. C., Saxena, D. K., & Chowdhuri, D. K. (2008). Adverse effect of tannery waste leachates in transgenic Drosophila melanogaster : Role of ROS in modulation of Hsp70, oxidative stress and apoptosis. Journal of Applied Toxicology , 28(6), 734–748. https://doi.org/10.1002/jat.1332
- Siddique, H. R., Gupta, S. C., Mitra, K., Murthy, R. C., Saxena, D. K., & Chowdhuri, D. K. (2007). Induction of biochemical stress markers and apoptosis in transgenic Drosophila melanogaster against complex chemical mixtures: Role of reactive oxygen species. Chemico-Biological Interactions , 169(3), 171–188. https://doi.org/10.1016/j.cbi.2007.06.035
- Simon, A. F., Daniels, R., Romero-Calderon, R., Grygoruk, A., Chang, H. Y., Najibi, R., Shamouelian, D., Salazar, E., Solomon, M., Ackerson, L. C., Maidment, N. T., Diantonio, A., & Krantz, D. E. (2009). Drosophila vesicular monoamine transporter mutants can adapt to reduced or eliminated vesicular stores of dopamine and serotonin. Genetics , 181(2), 525–541. https://doi.org/10.1534/genetics.108.094110
- Spierer, A. N., Mossman, J. A., Smith, S. P., Crawford, L., Ramachandran, S., & Rand, D. M. (2021). Natural variation in the regulation of neurodevelopmental genes modifies flight performance in Drosophila. PLoS Genetics , 17(3), e1008887. https://doi.org/10.1371/journal.pgen.1008887
- St Clair, S. L., Li, H., Ashraf, U., Karty, J. A., & Tennessen, J. M. (2017). Metabolomic analysis reveals that the Drosophila melanogaster gene lysine influences diverse aspects of metabolism. Genetics , 207(4), 1255–1261. https://doi.org/10.1534/genetics.117.300201
- Swarup, S., Huang, W., Mackay, T. F. C., & Anholt, R. R. H. (2013). Analysis of natural variation reveals neurogenetic networks for Drosophila olfactory behavior. Proceedings of the National Academy of Sciences of the United States of America , 110(3), 1017–1022. https://doi.org/10.1073/pnas.1220168110
- Sykiotis, G. P., & Bohmann, D. (2008). Keap1/Nrf2 signaling regulates oxidative stress tolerance and lifespan in Drosophila. Developmental Cell , 14(1), 76–85. https://doi.org/10.1016/j.devcel.2007.12.002
- Tassetto, M., Kunitomi, M., & Andino, R. (2017). Circulating immune cells mediate a systemic rnai-based adaptive antiviral response in Drosophila. Cell , 169(2), 314–325.e313. https://doi.org/10.1016/j.cell.2017.03.033
- Tennessen, J. M., Bertagnolli, N. M., Evans, J., Sieber, M. H., Cox, J., & Thummel, C. S. (2014). Coordinated metabolic transitions during Drosophila embryogenesis and the onset of aerobic glycolysis. G3 , 4(5), 839–850. https://doi.org/10.1534/g3.114.010652
- Tiwari, A. K., Pragya, P., Ravi Ram, K., & Chowdhuri, D. K. (2011). Environmental chemical mediated male reproductive toxicity: Drosophila melanogaster as an alternate animal model. Theriogenology , 76(2), 197–216. https://doi.org/10.1016/j.theriogenology.2010.12.027
- Tu, C. P., & Akgul, B. (2005). Drosophila glutathione S-transferases. Methods in Enzymology , 401, 204–226. https://doi.org/10.1016/S0076-6879(05)01013-X
- Tuthill, B. F., 2nd, Searcy, L. A., Yost, R. A., & Musselman, L. P. (2020). Tissue-specific analysis of lipid species in Drosophila during overnutrition by UHPLC-MS/MS and MALDI-MSI. Journal of Lipid Research , 61(3), 275–290. https://doi.org/10.1194/jlr.RA119000198
- Viant, M. R., Ebbels, T. M. D., Beger, R. D., Ekman, D. R., Epps, D. J. T., Kamp, H., Leonards, P. E. G., Loizou, G. D., MacRae, J. I., van Ravenzwaay, B., Rocca-Serra, P., Salek, R. M., Walk, T., & Weber, R. J. M. (2019). Use cases, best practice and reporting standards for metabolomics in regulatory toxicology. Nature Communications , 10(1), 3041. https://doi.org/10.1038/s41467-019-10900-y
- Vorojeikina, D., Broberg, K., Love, T. M., Davidson, P. W., van Wijngaarden, E., & Rand, M. D. (2017). Editor's highlight: Glutathione S-transferase activity moderates methylmercury toxicity during development in Drosophila. Toxicological Sciences , 157(1), 211–221. https://doi.org/10.1093/toxsci/kfx033
- Walters, J. D., Hatfield, J. S., Baker, B. B., Mackay, T. F. C., & Anholt, R. R. H. (2021). A high throughput microplate feeder assay for quantification of consumption in Drosophila. Journal of Visualized Experiments , 172, e62771. https://doi.org/10.3791/62771
- Wang, K. H., Penmatsa, A., & Gouaux, E. (2015). Neurotransmitter and psychostimulant recognition by the dopamine transporter. Nature , 521(7552), 322–327. https://doi.org/10.1038/nature14431
- Weber, A. L., Khan, G. F., Magwire, M. M., Tabor, C. L., Mackay, T. F. C., & Anholt, R. R. H. (2012). Genome-wide association analysis of oxidative stress resistance in Drosophila melanogaster. PLoS ONE , 7(4), e34745. https://doi.org/10.1371/journal.pone.0034745
- Weed, D. L. (2021). Does paraquat cause Parkinson's disease? A review of reviews. Neurotoxicology , 86, 180–184. https://doi.org/10.1016/j.neuro.2021.08.006
- Williams, D. W., & Truman, J. W. (2004). Mechanisms of dendritic elaboration of sensory neurons in Drosophila: Insights from in vivo time lapse. Journal of Neuroscience , 24(7), 1541–1550. https://doi.org/10.1523/JNEUROSCI.4521-03.2004
- Willingham, A. T., Dike, S., Cheng, J., Manak, J. R., Bell, I., Cheung, E., Drenkow, J., Dumais, E., Duttagupta, R., Ganesh, M., Ghosh, S., Helt, G., Nix, D., Piccolboni, A., Sementchenko, V., Tammana, H., Kapranov, P., Genes, E., Transcripts, G., & Gingeras, T. R. (2006). Transcriptional landscape of the human and fly genomes: Nonlinear and multifunctional modular model of transcriptomes. Cold Spring Harbor Symposia on Quantitative Biology , 71, 101–110. https://doi.org/10.1101/sqb.2006.71.068
- Willoughby, L., Chung, H., Lumb, C., Robin, C., Batterham, P., & Daborn, P. J. (2006). A comparison of Drosophila melanogaster detoxification gene induction responses for six insecticides, caffeine and phenobarbital. Insect Biochemistry and Molecular Biology , 36(12), 934–942. https://doi.org/10.1016/j.ibmb.2006.09.004
- Wu, X., & Gu, H. H. (2003). Cocaine affinity decreased by mutations of aromatic residue phenylalanine 105 in the transmembrane domain 2 of dopamine transporter. Molecular Pharmacology , 63(3), 653–658. https://doi.org/10.1124/mol.63.3.653
- Xiao, S., Baik, L. S., Shang, X., & Carlson, J. R. (2022). Meeting a threat of the Anthropocene: Taste avoidance of metal ions by Drosophila. Proceedings of the National Academy of Sciences of the United States of America , 119(25), e2204238119. https://doi.org/10.1073/pnas.2204238119
- Yamamoto, A., Zwarts, L., Callaerts, P., Norga, K., Mackay, T. F. C., & Anholt, R. R. H. (2008). Neurogenetic networks for startle-induced locomotion in Drosophila melanogaster. Proceedings of the National Academy of Sciences of the United States of America , 105(34), 12393–12398. https://doi.org/10.1073/pnas.0804889105
- Yildirim, K., Petri, J., Kottmeier, R., & Klambt, C. (2019). Drosophila glia: Few cell types and many conserved functions. Glia , 67(1), 5–26. https://doi.org/10.1002/glia.23459
- Zamberlan, D. C., Halmenschelager, P. T., Silva, L. F. O., & da Rocha, J. B. T. (2020). Copper decreases associative learning and memory in Drosophila melanogaster. Science of the Total Environment , 710, 135306. https://doi.org/10.1016/j.scitotenv.2019.135306
- Zhao, X., Golic, F. T., Harrison, B. R., Manoj, M., Hoffman, E. V., Simon, N., Johnson, R., MacCoss, M. J., McIntyre, L. M., & Promislow, D. E. L. (2022). The metabolome as a biomarker of aging in Drosophila melanogaster. Aging Cell , 21(2), e13548. https://doi.org/10.1111/acel.13548
- Zhou, S., Campbell, T. G., Stone, E. A., Mackay, T. F., & Anholt, R. R. (2012). Phenotypic plasticity of the Drosophila transcriptome. PLoS Genetics , 8(3), e1002593. https://doi.org/10.1371/journal.pgen.1002593
- Zhou, S., Luoma, S. E., St Armour, G. E., Thakkar, E., Mackay, T. F. C., & Anholt, R. R. H. (2017). A Drosophila model for toxicogenomics: Genetic variation in susceptibility to heavy metal exposure. PLoS Genetics , 13(7), e1006907. https://doi.org/10.1371/journal.pgen.1006907
- Zhou, S., Morgante, F., Geisz, M. S., Ma, J., Anholt, R. R. H., & Mackay, T. F. C. (2020). Systems genetics of the Drosophila metabolome. Genome Research , 30(3), 392–405. https://doi.org/10.1101/gr.243030.118
- Zhou, S., Morozova, T. V., Hussain, Y. N., Luoma, S. E., McCoy, L., Yamamoto, A., Mackay, T. FC., & Anholt, R. R. H. (2016). The genetic basis for variation in sensitivity to lead toxicity in Drosophila melanogaster. Environmental Health Perspectives , 124(7), 1062–1070. https://doi.org/10.1289/ehp.1510513
- Zwarts, L., Magwire, M. M., Carbone, M. A., Versteven, M., Herteleer, L., Anholt, R. R. H., Callaerts, P., & Mackay, T. F. C. (2011). Complex genetic architecture of Drosophila aggressive behavior. Proceedings of the National Academy of Sciences of the United States of America , 108(41), 17070–17075. https://doi.org/10.1073/pnas.1113877108
- Zwarts, L., Vanden Broeck, L., Cappuyns, E., Ayroles, J. F., Magwire, M. M., Vulsteke, V., Clements, J., Mackay, T. F. C., & Callaerts, P. (2015). The genetic basis of natural variation in mushroom body size in Drosophila melanogaster. Nature Communications , 6, 10115. https://doi.org/10.1038/ncomms10115
- INTERNET RESOURCE.
- http://flybase.org/
- Launch page for FlyBase, which provides access to the resources described in this article.
Citing Literature
Number of times cited according to CrossRef: 2
- Elisabetta Catalani, Kashi Brunetti, Simona Del Quondam, Silvia Bongiorni, Simona Picchietti, Anna Maria Fausto, Gabriele Lupidi, Enrico Marcantoni, Cristiana Perrotta, Gabriele Achille, Federico Buonanno, Claudio Ortenzi, Davide Cervia, Exposure to the Natural Compound Climacostol Induces Cell Damage and Oxidative Stress in the Fruit Fly Drosophila melanogaster, Toxics, 10.3390/toxics12020102, 12 , 2, (102), (2024).
- Michele Colombo, Laura Grauso, Virginia Lanzotti, Guido Incerti, Adele Adamo, Aurora Storlazzi, Silvia Gigliotti, Stefano Mazzoleni, Self-DNA Inhibition in Drosophila melanogaster Development: Metabolomic Evidence of the Molecular Determinants, Biology, 10.3390/biology12111378, 12 , 11, (1378), (2023).