Tutorial: a statistical genetics guide to identifying HLA alleles driving complex disease
Saori Sakaue, Saisriram Gurajala, Michelle Curtis, Yang Luo, Wanson Choi, Kazuyoshi Ishigaki, Joyce B. Kang, Laurie Rumker, Aaron J. Deutsch, Sebastian Schönherr, Lukas Forer, Jonathon LeFaive, Christian Fuchsberger, Buhm Han, Tobias L. Lenz, Paul I. W. de Bakker, Yukinori Okada, Albert V. Smith, Soumya Raychaudhuri
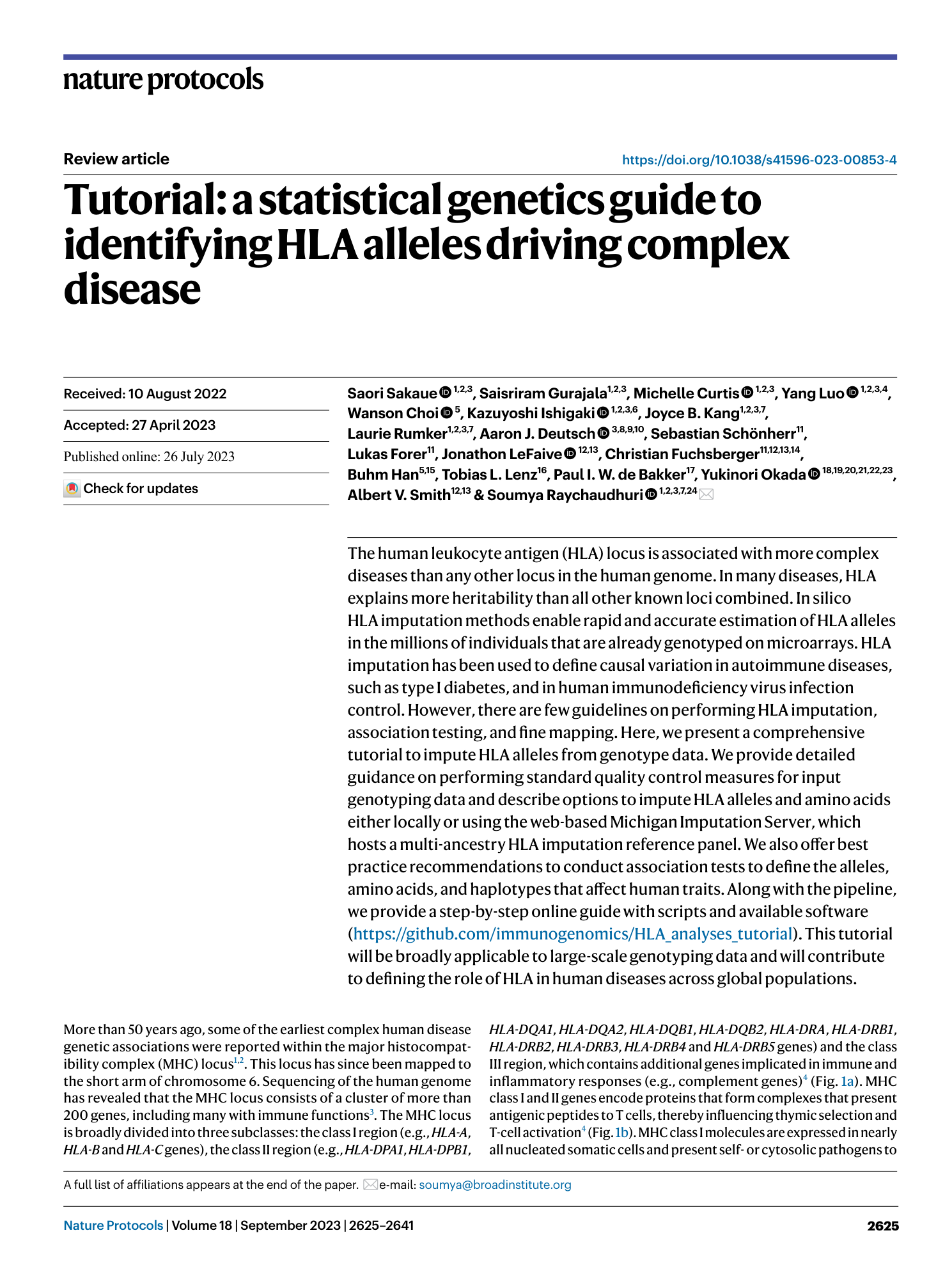
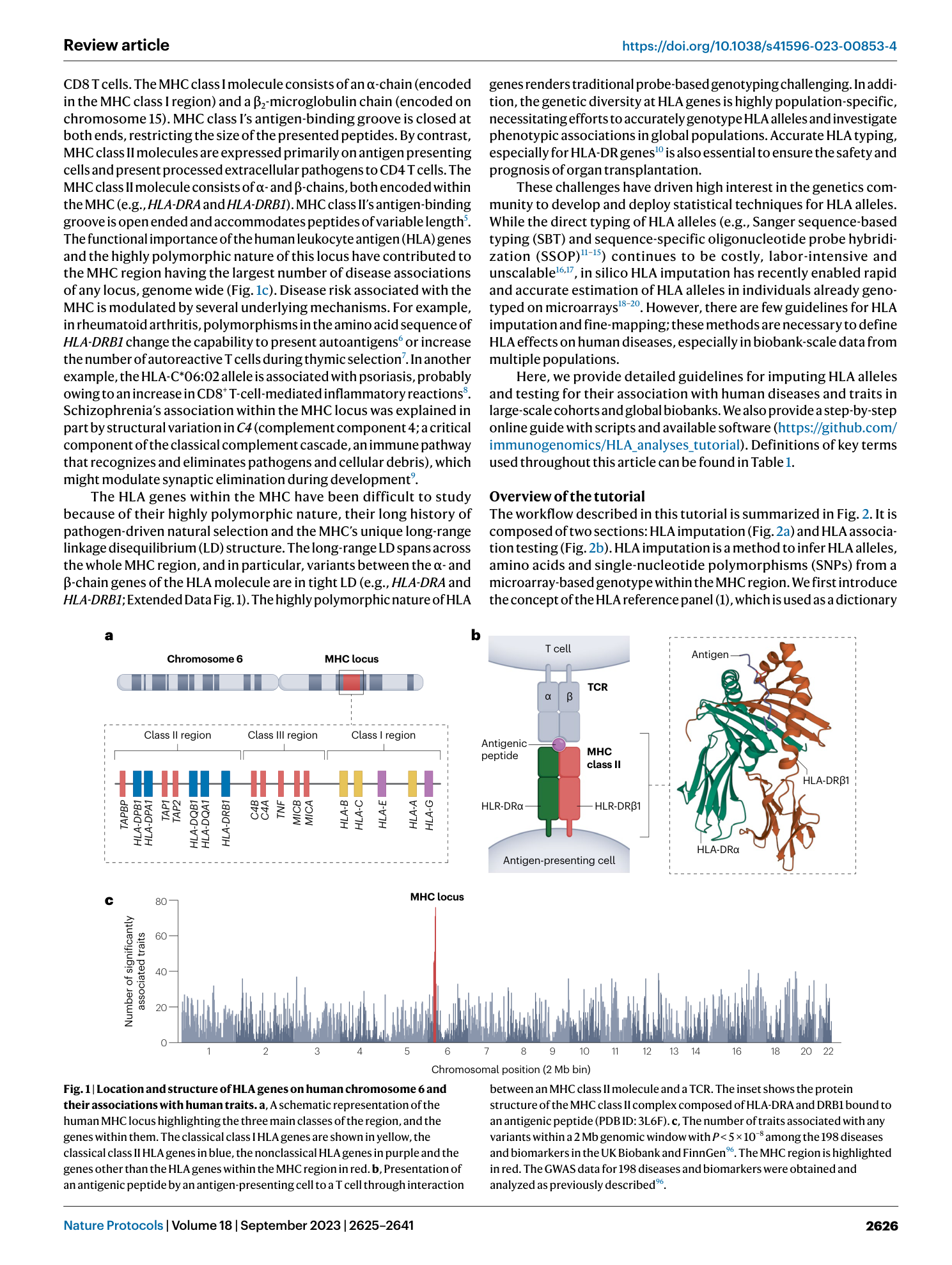
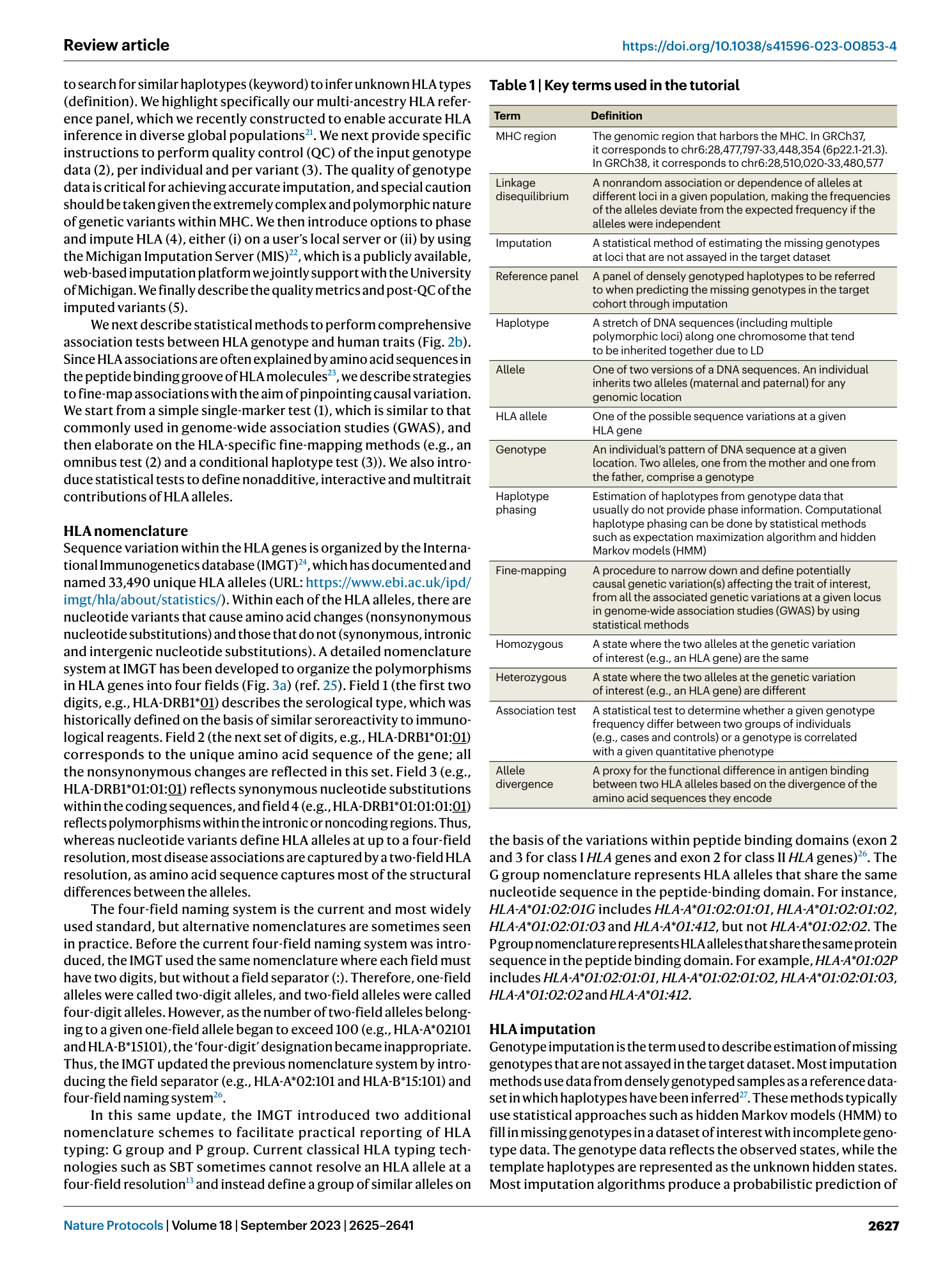
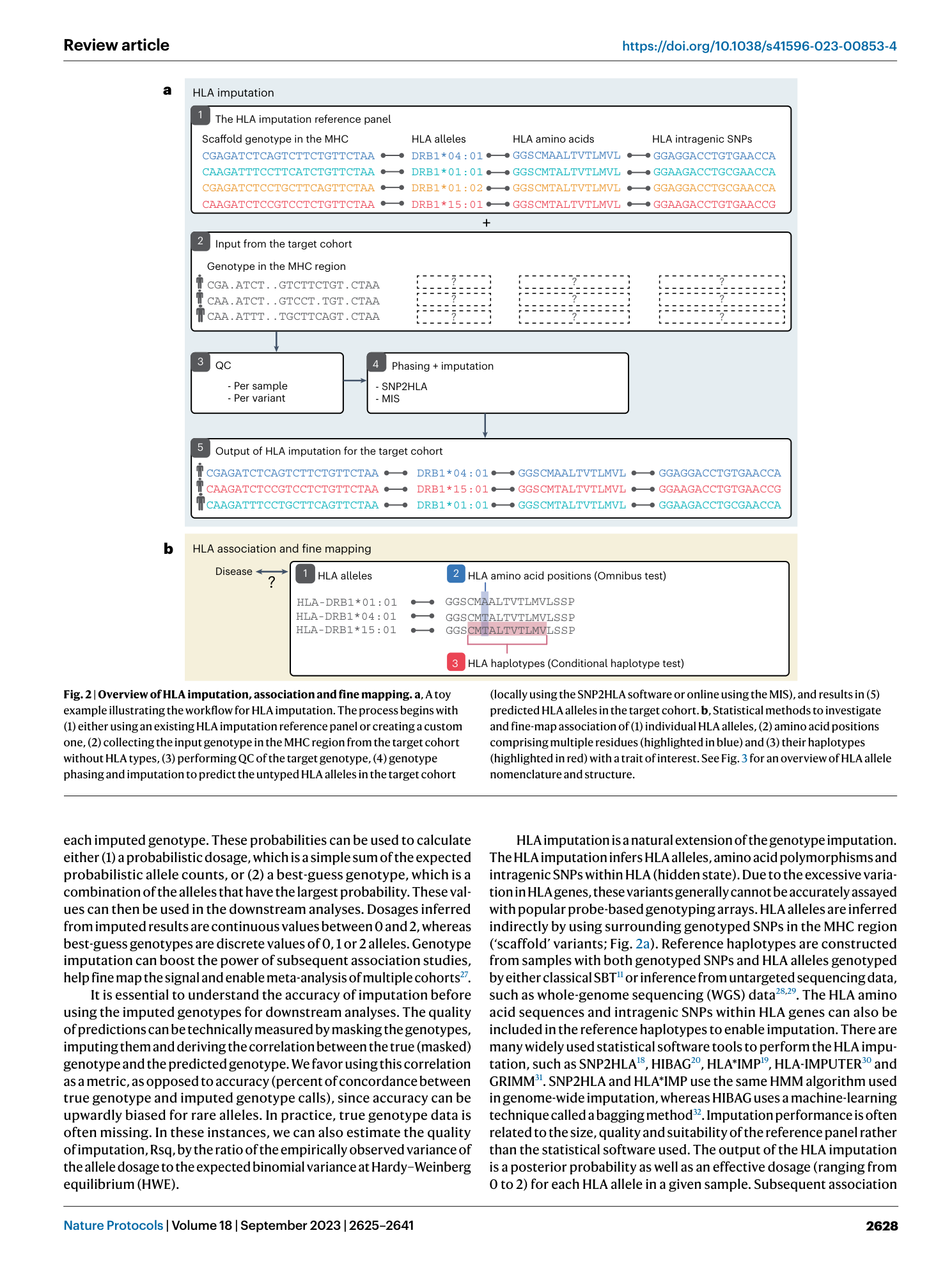
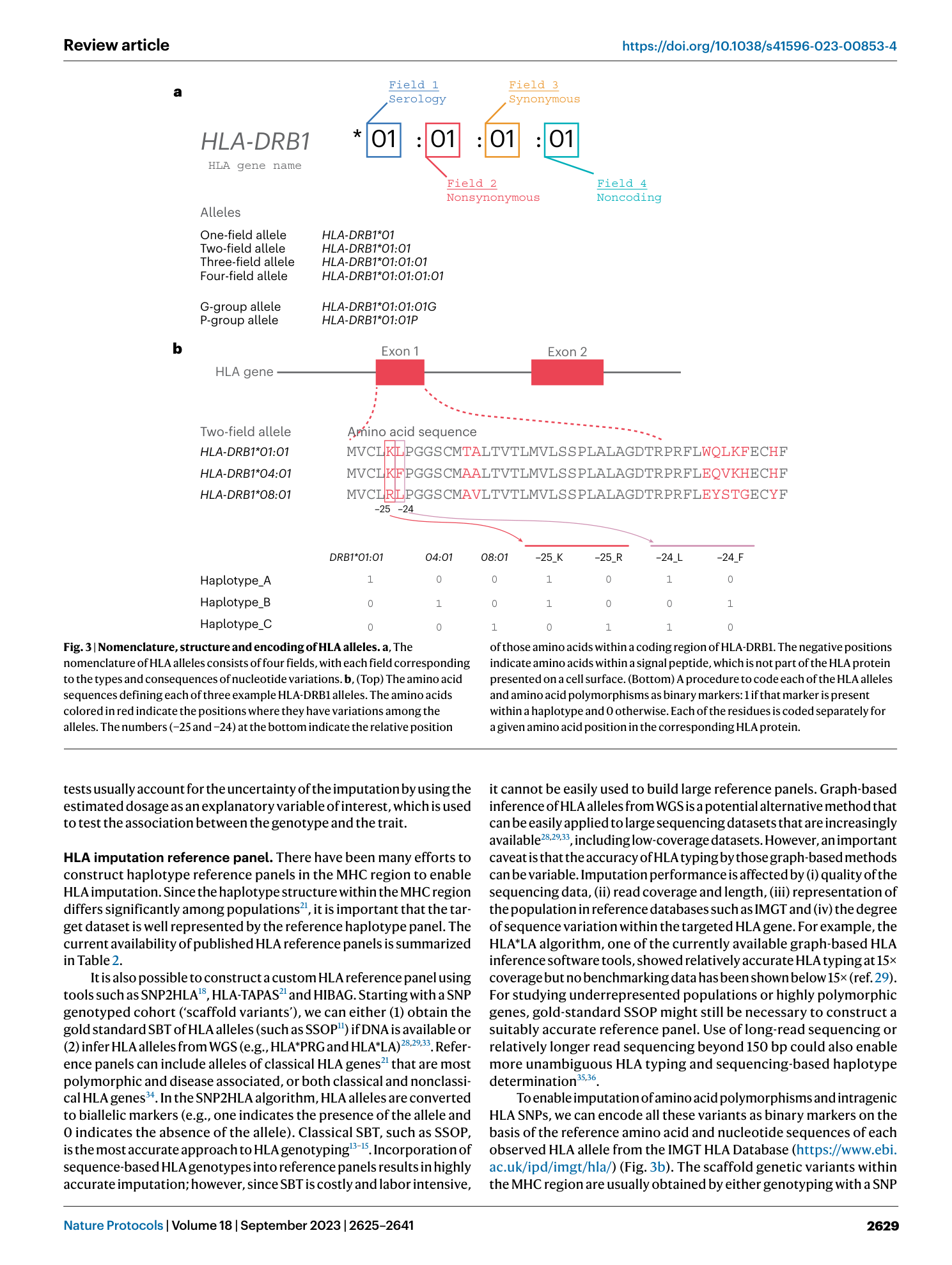
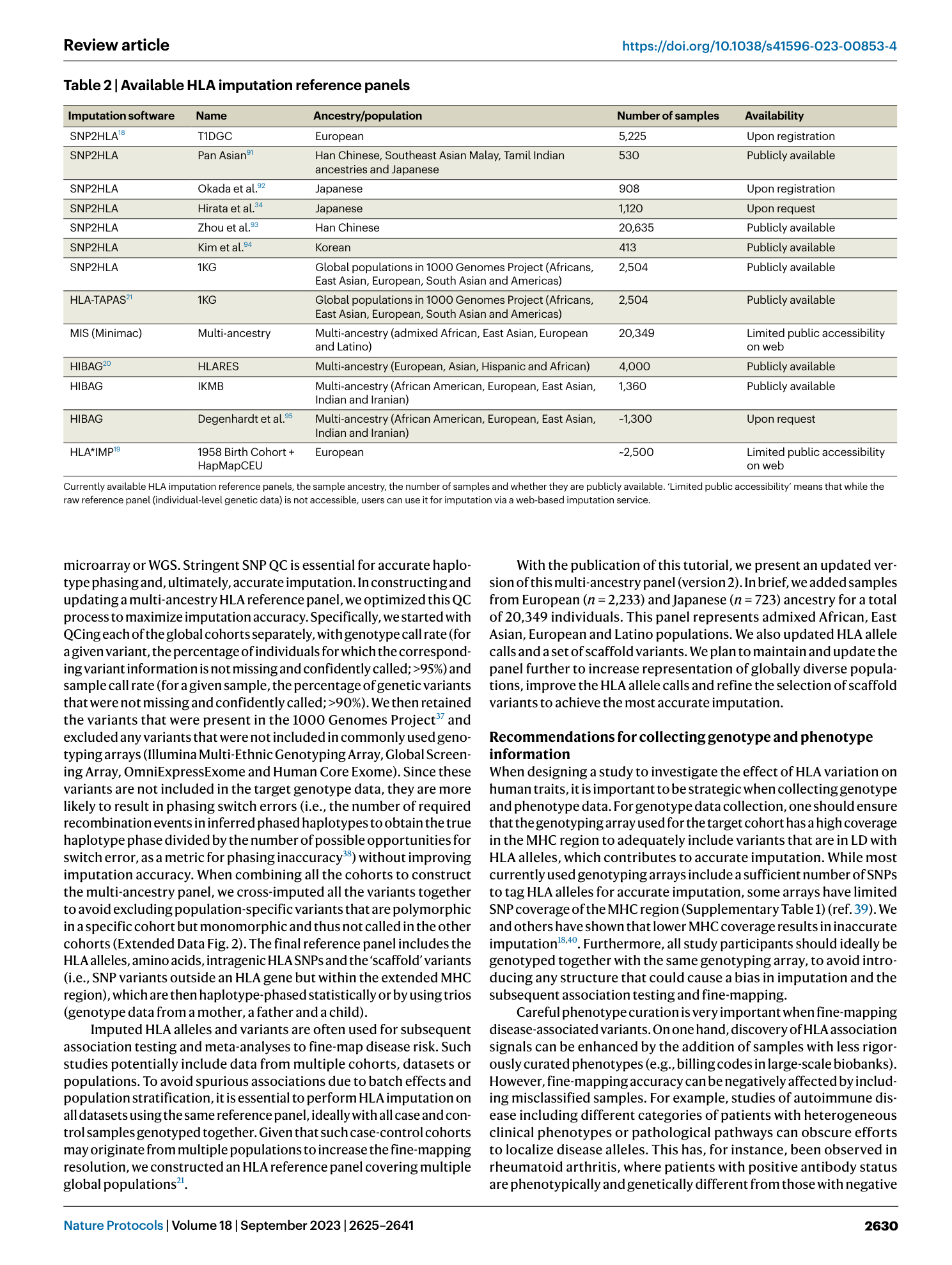
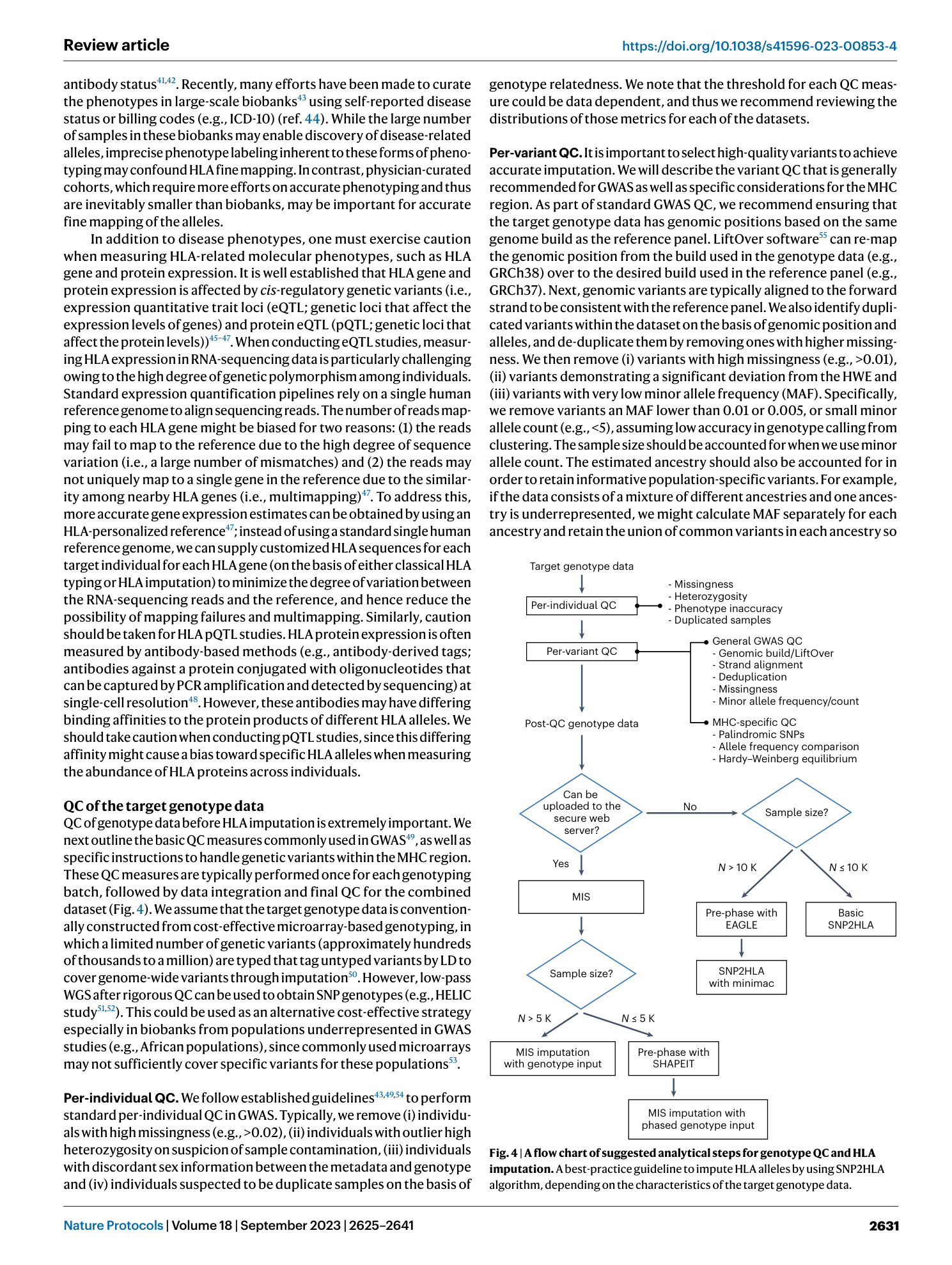
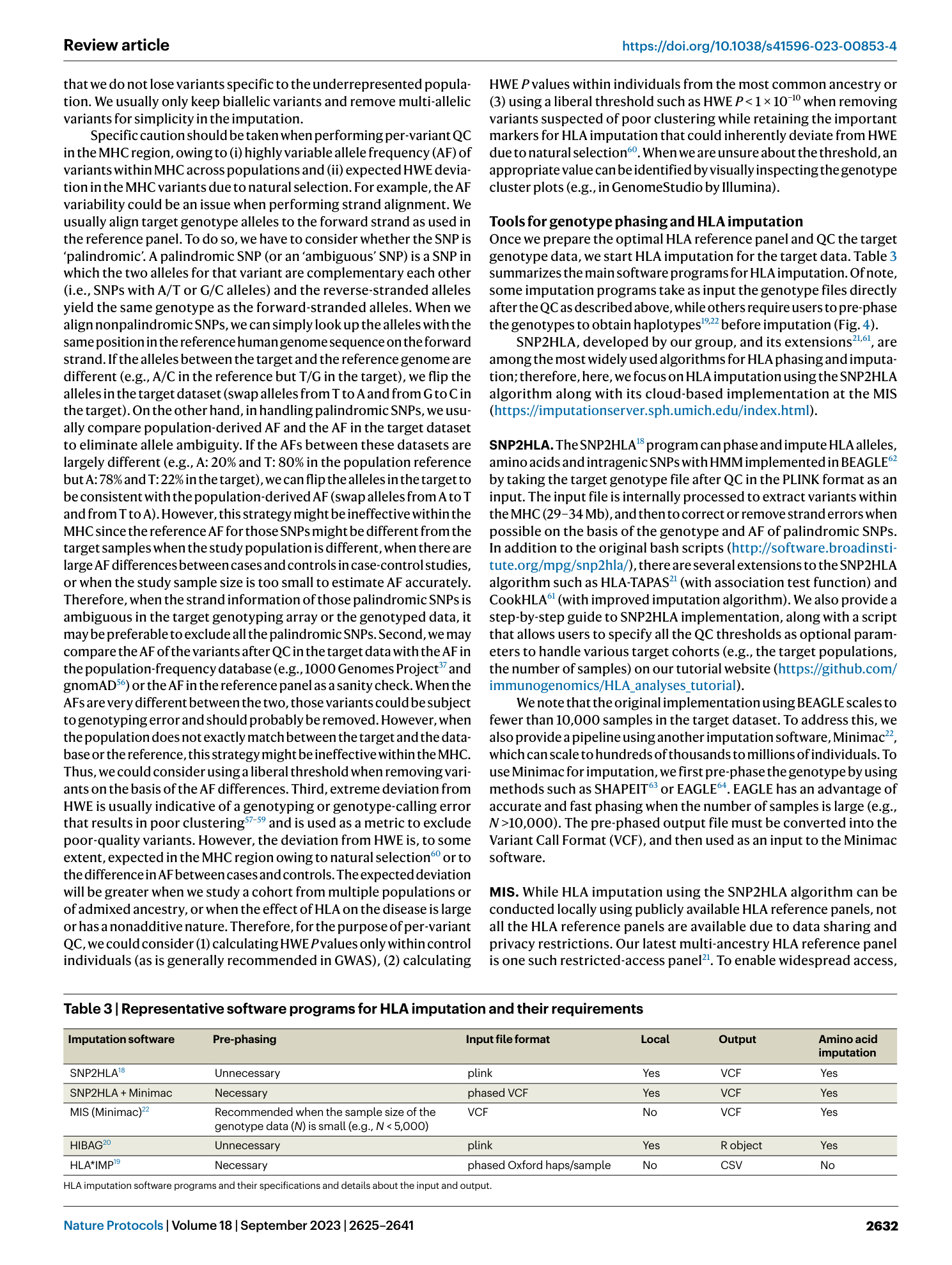
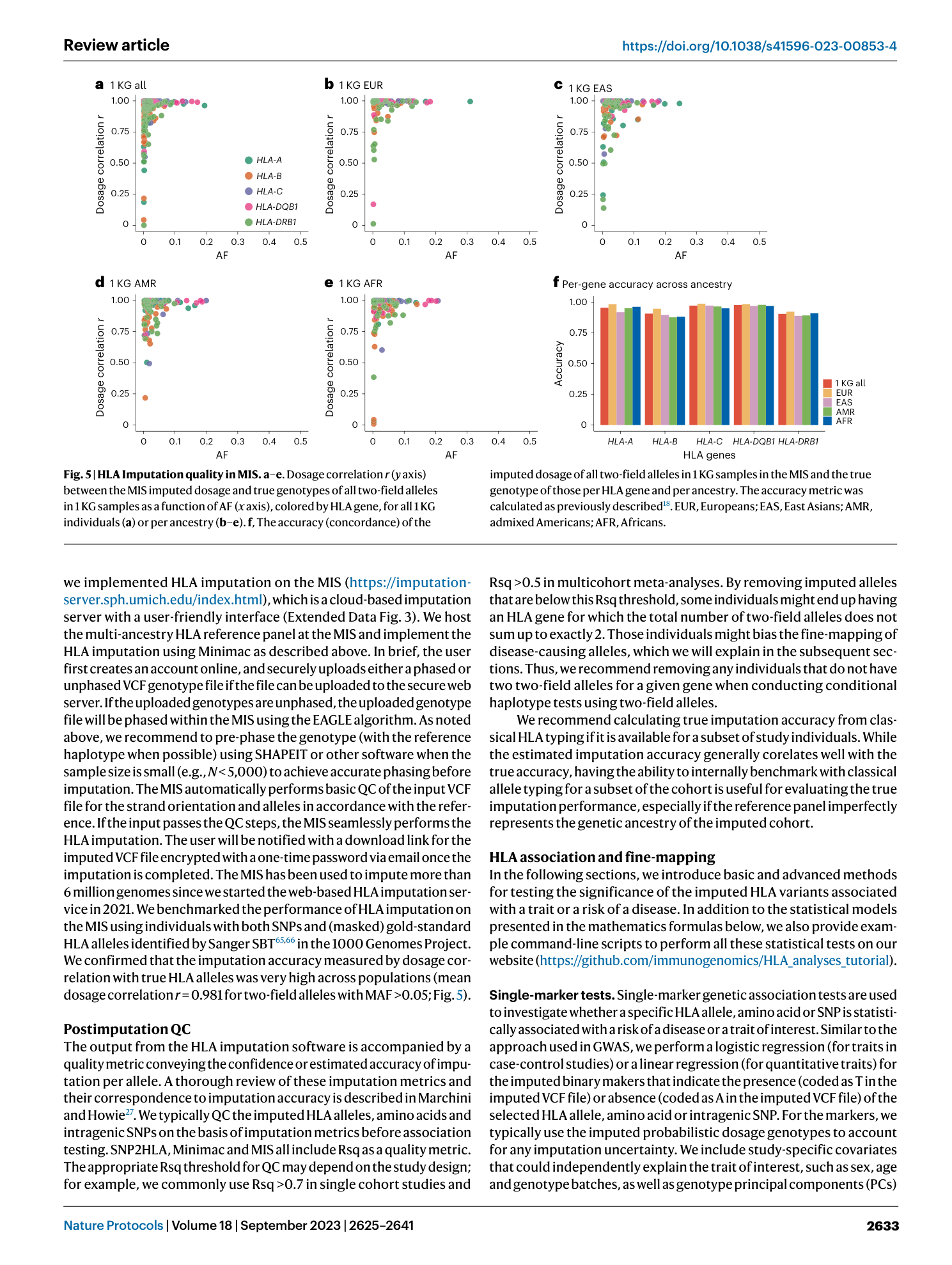
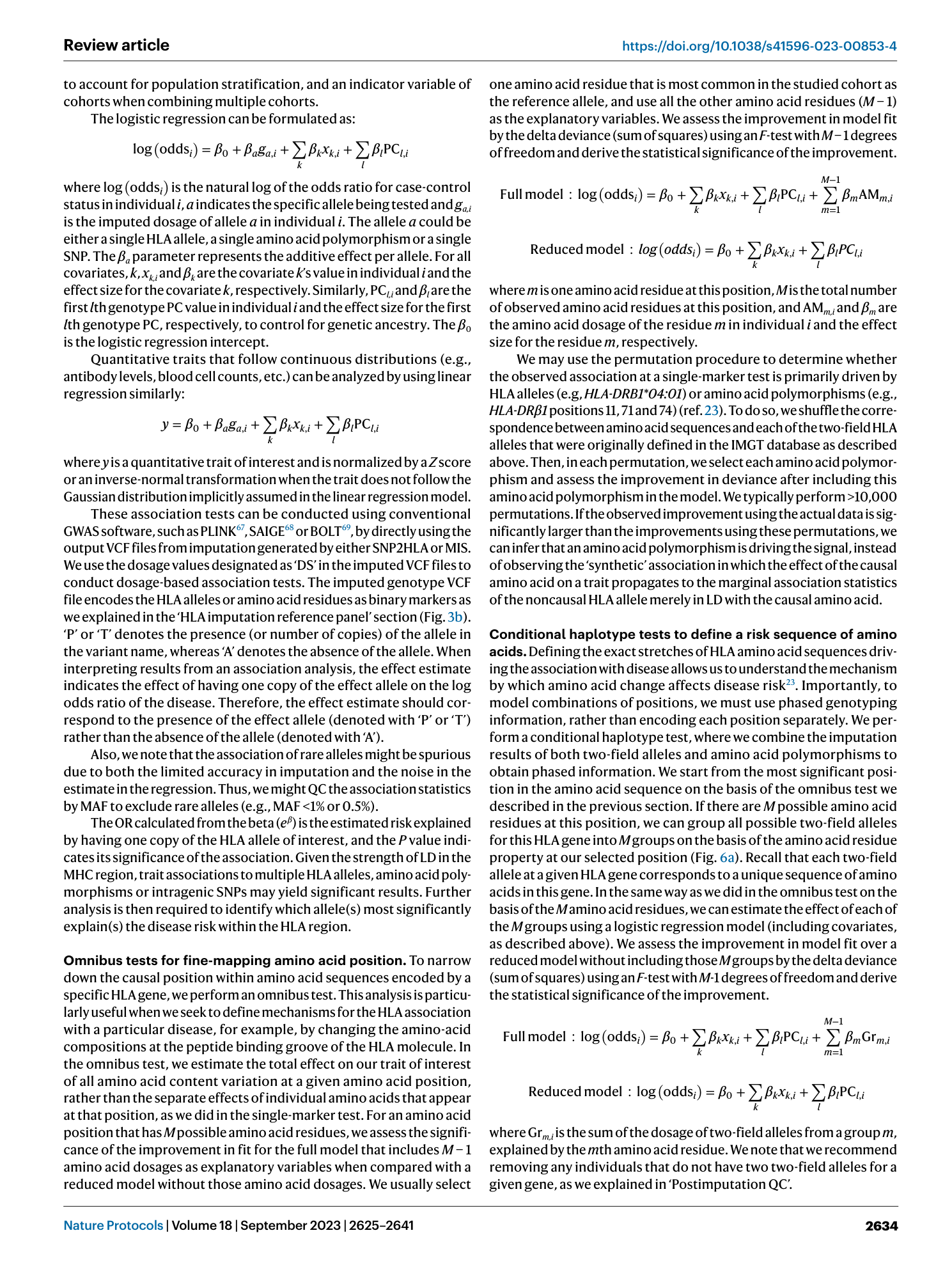
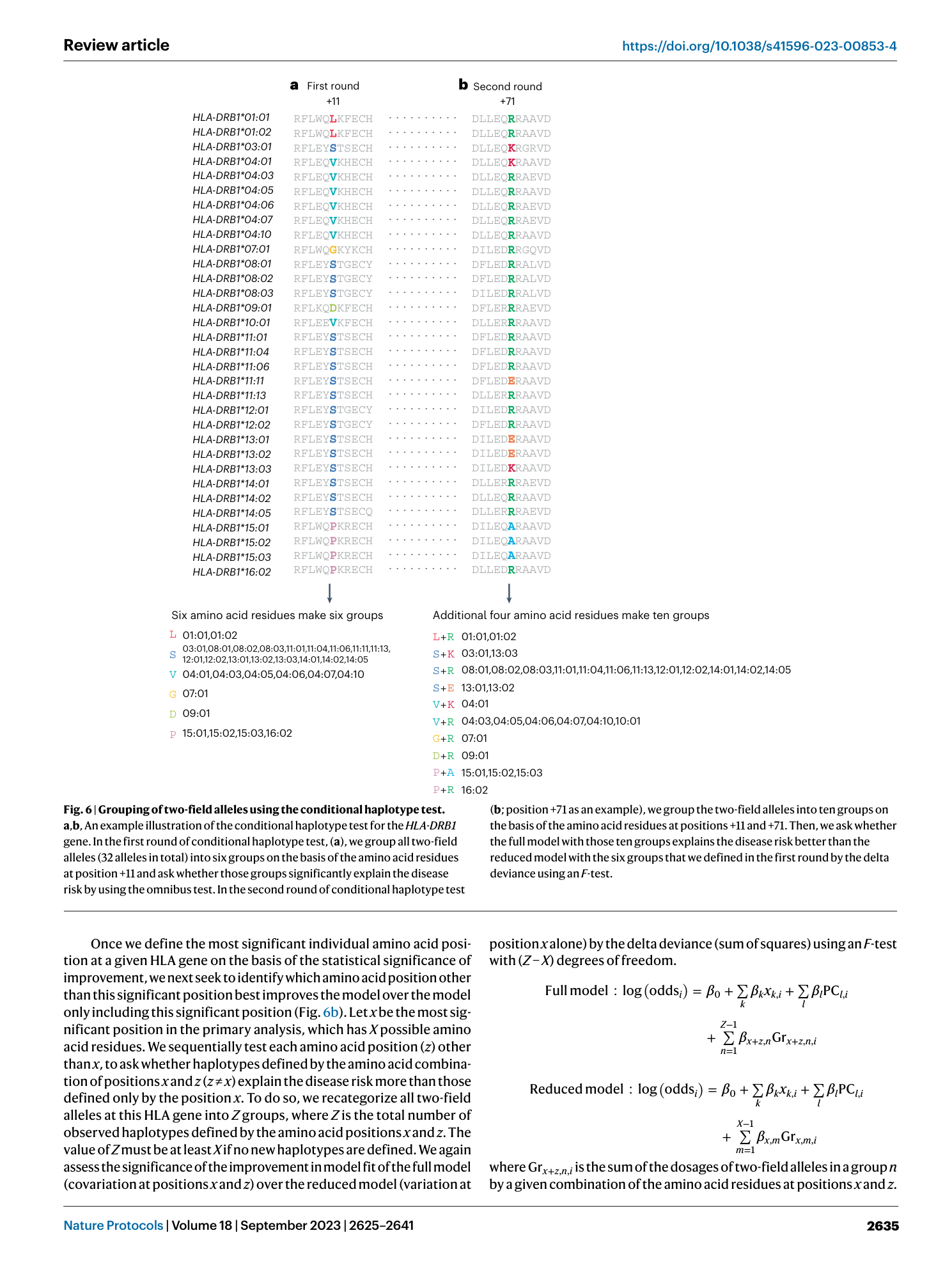
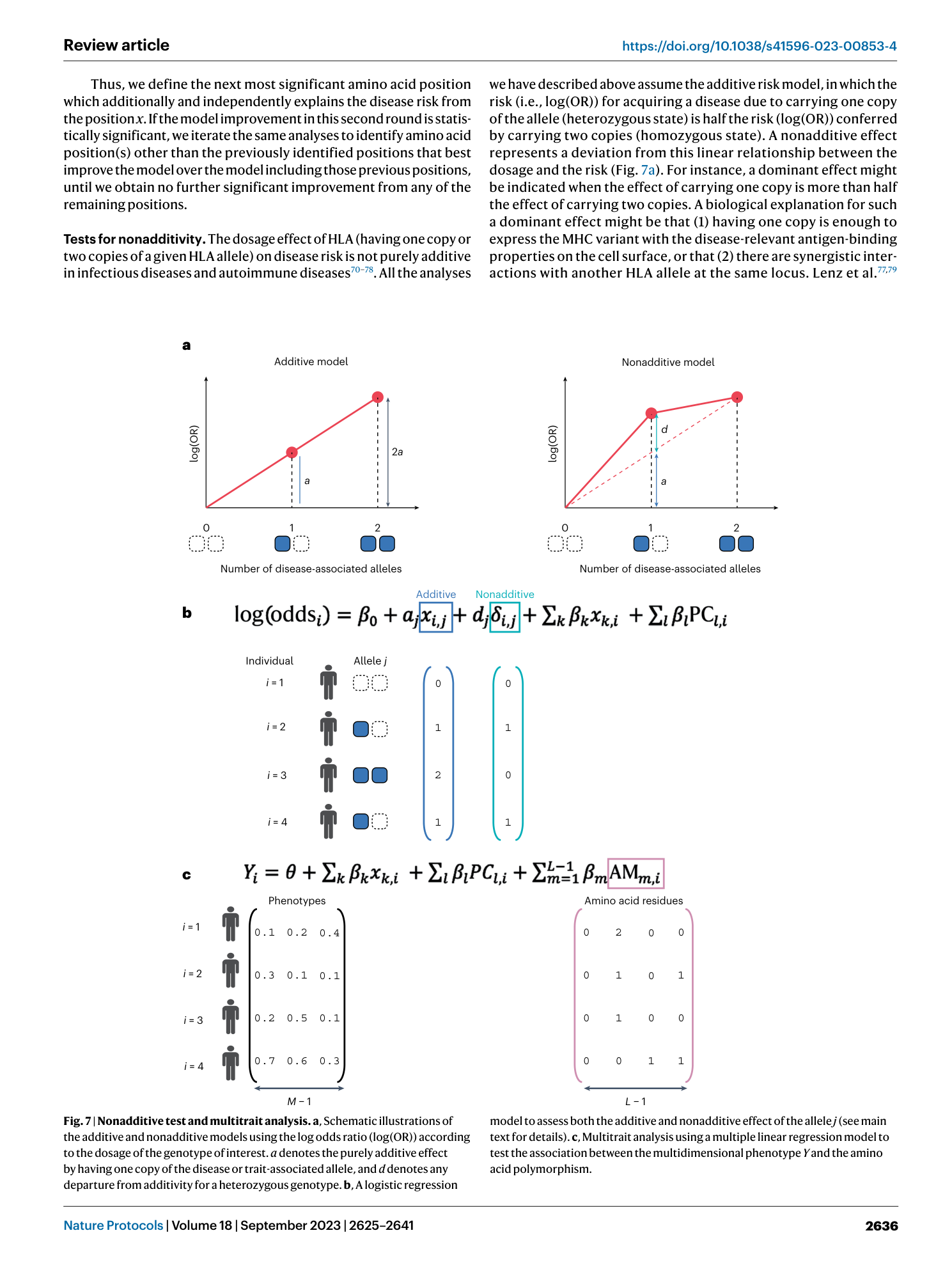
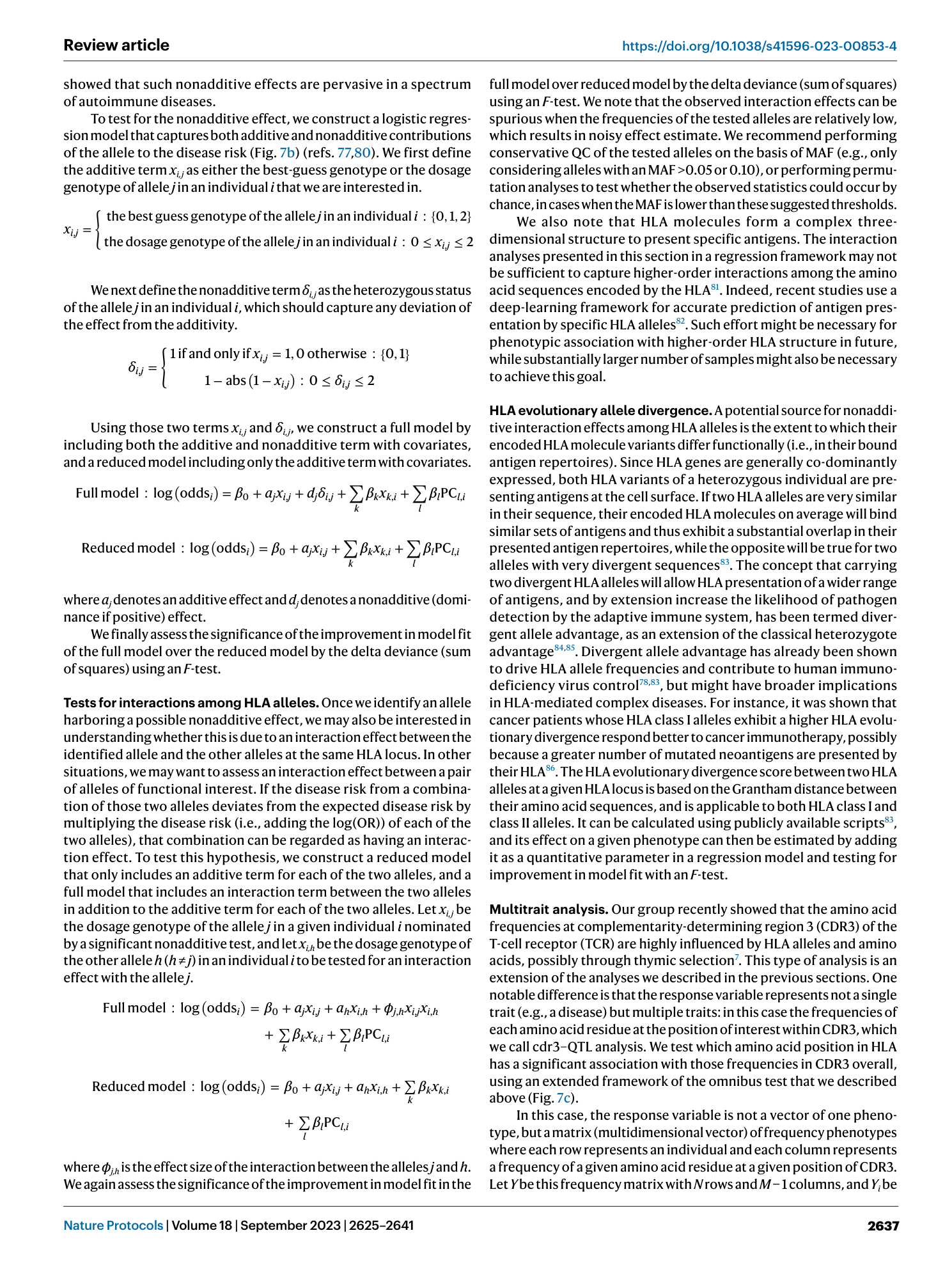
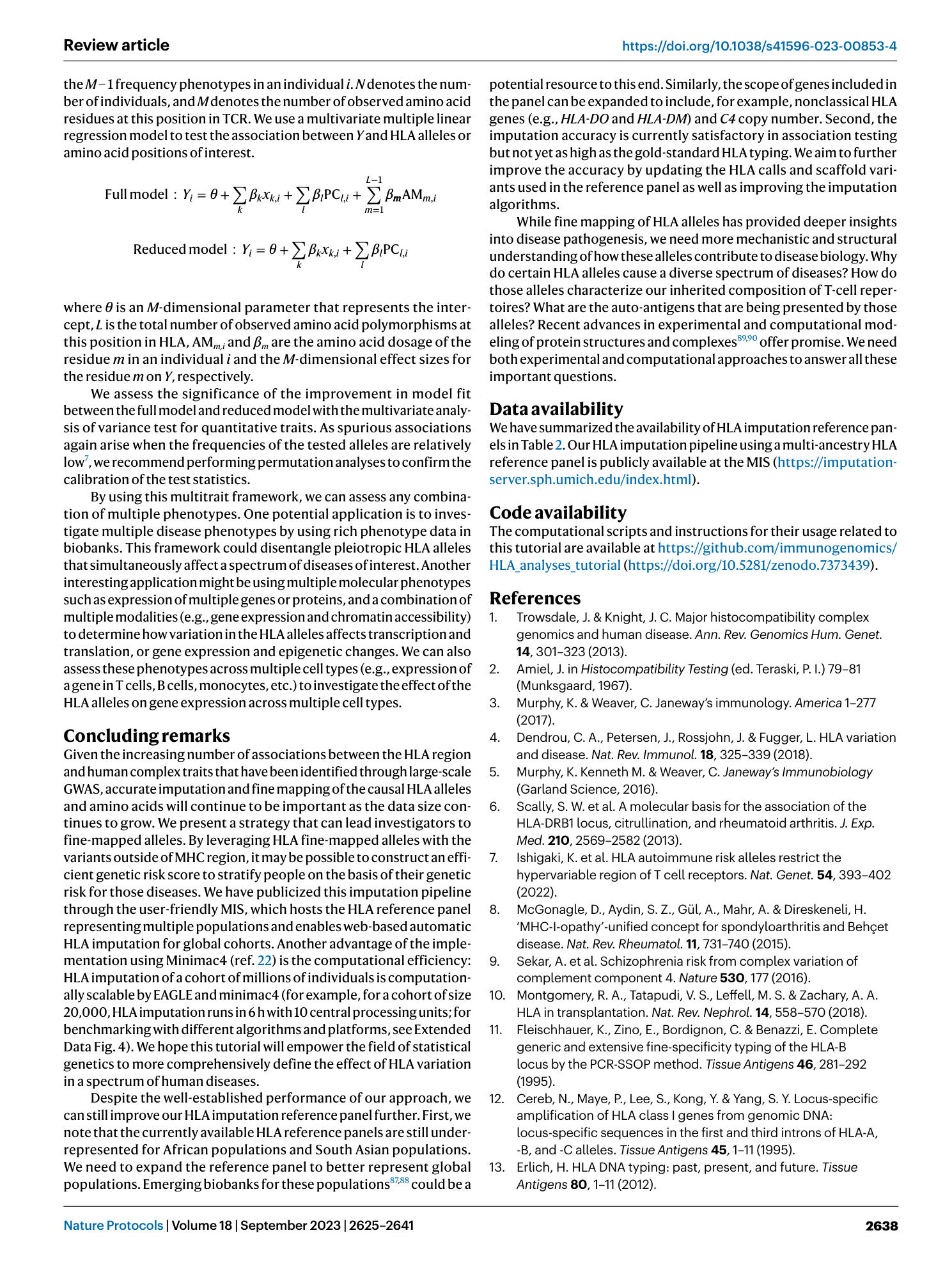
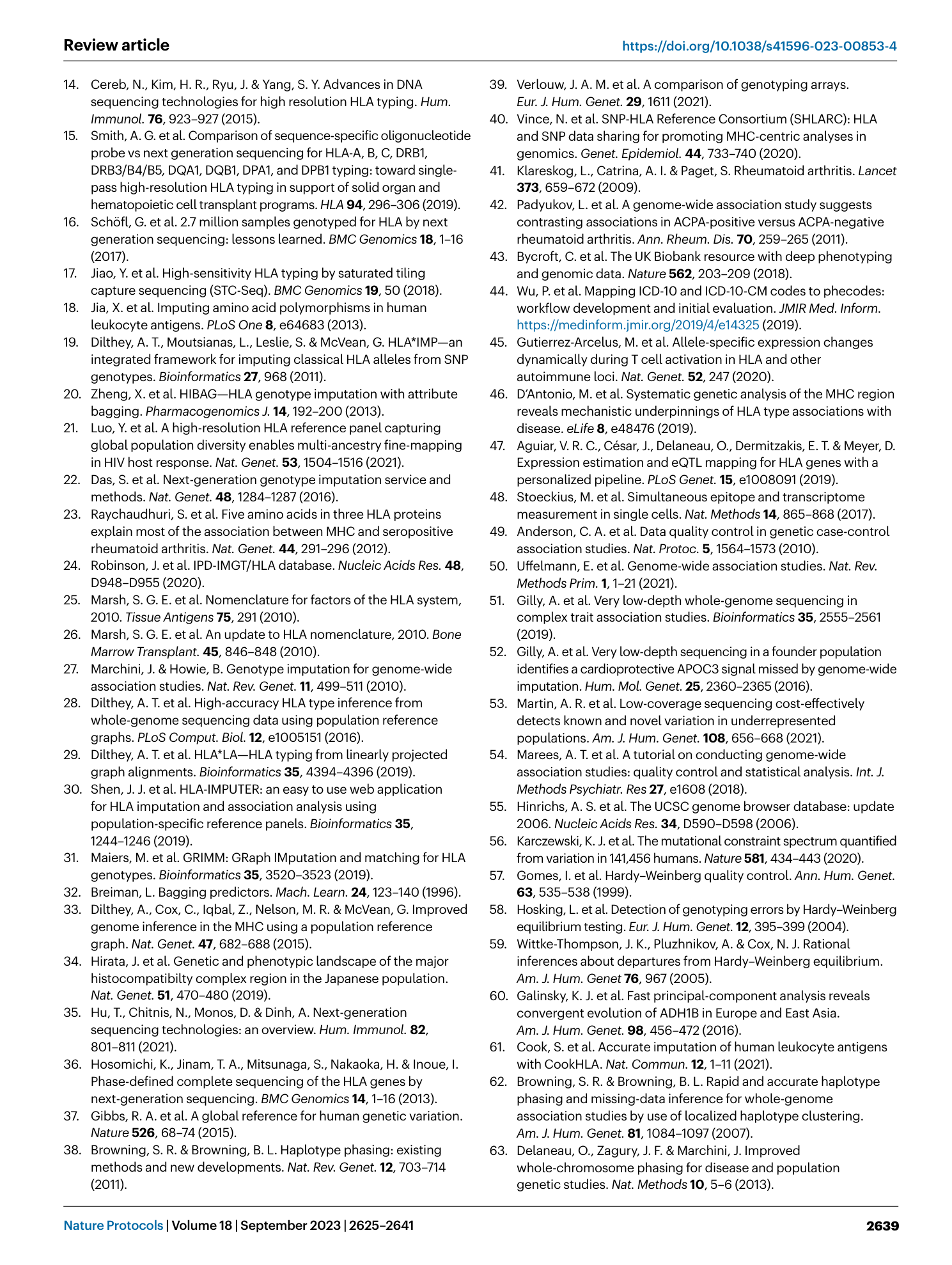
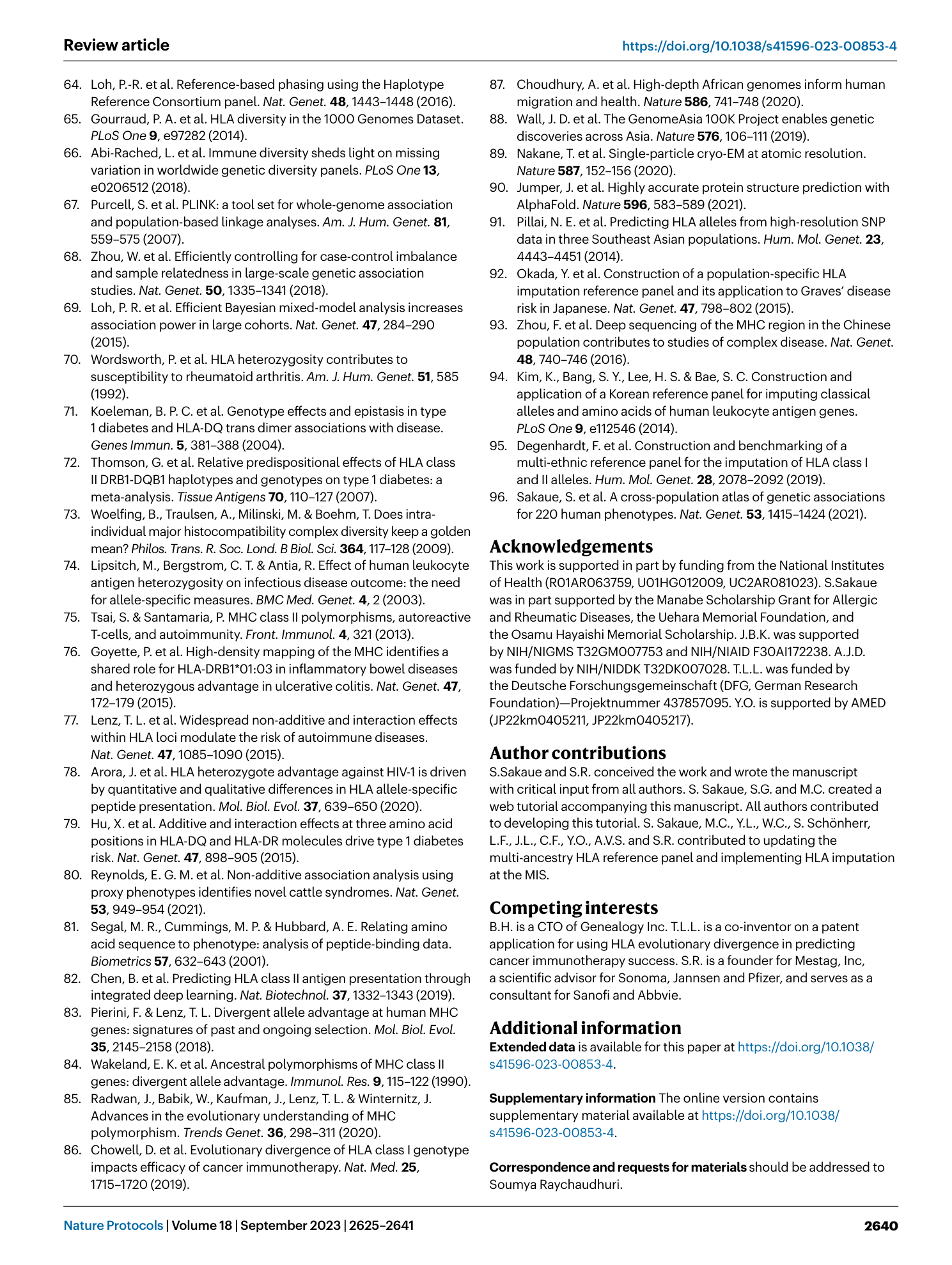
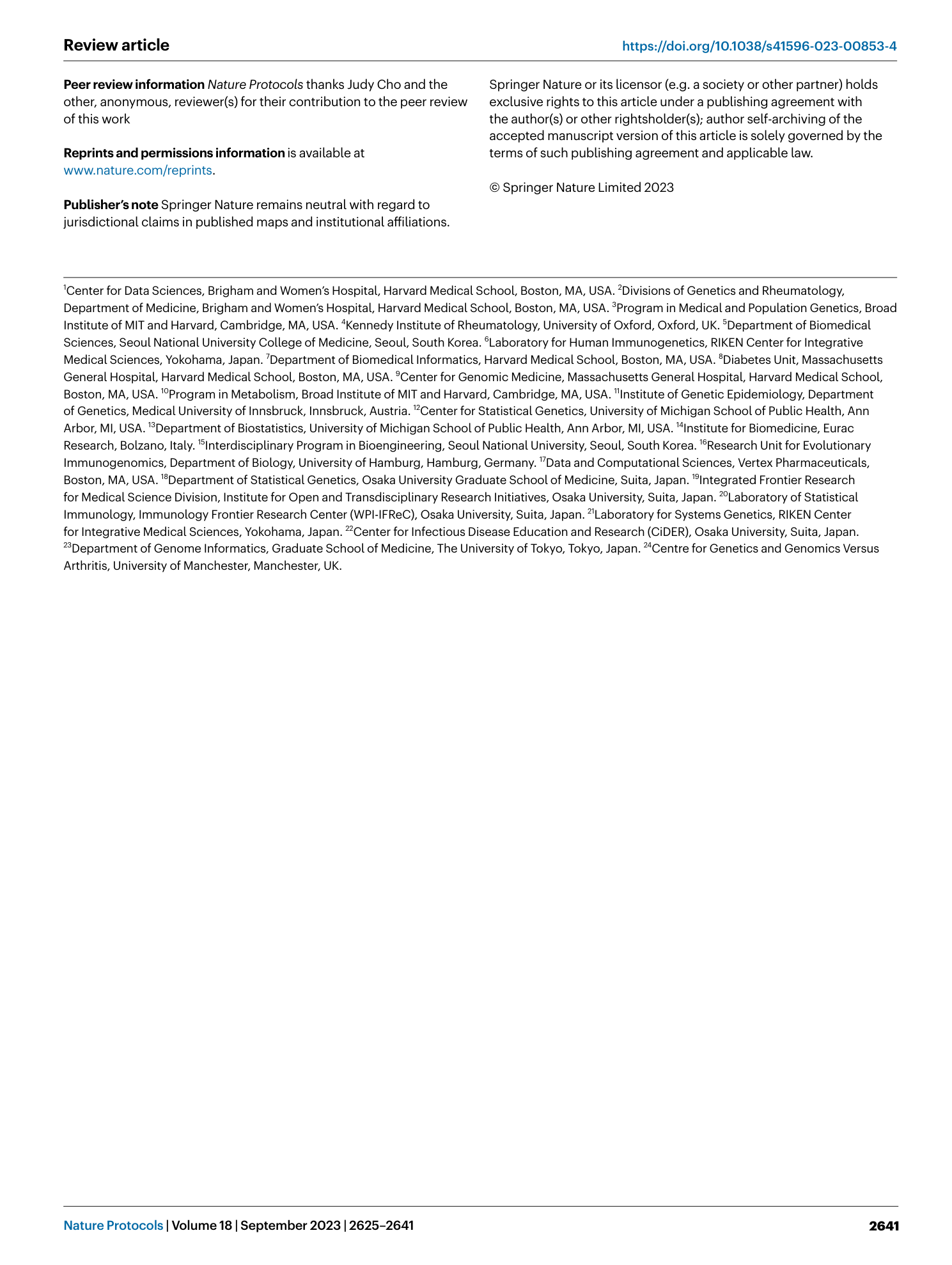
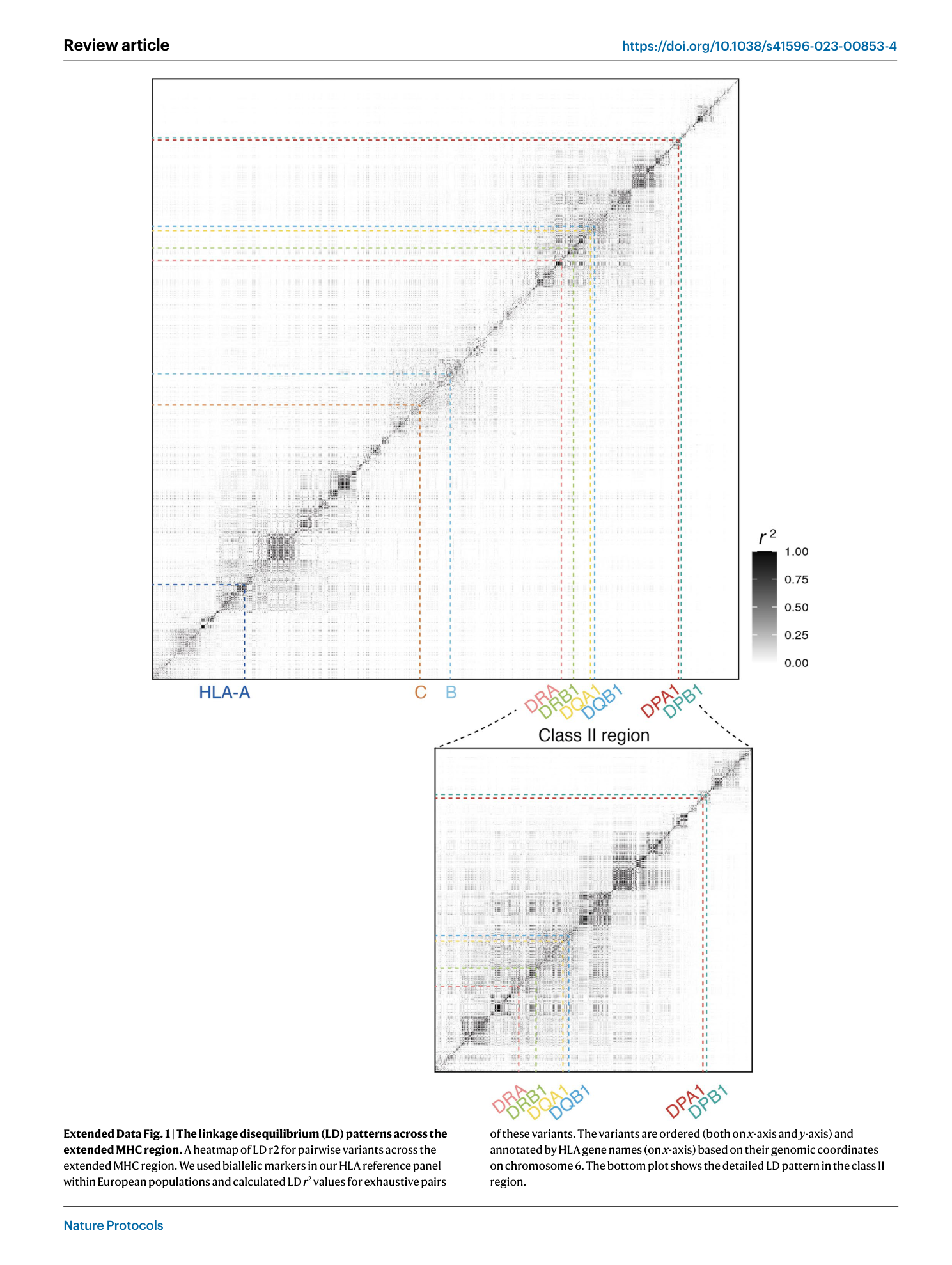
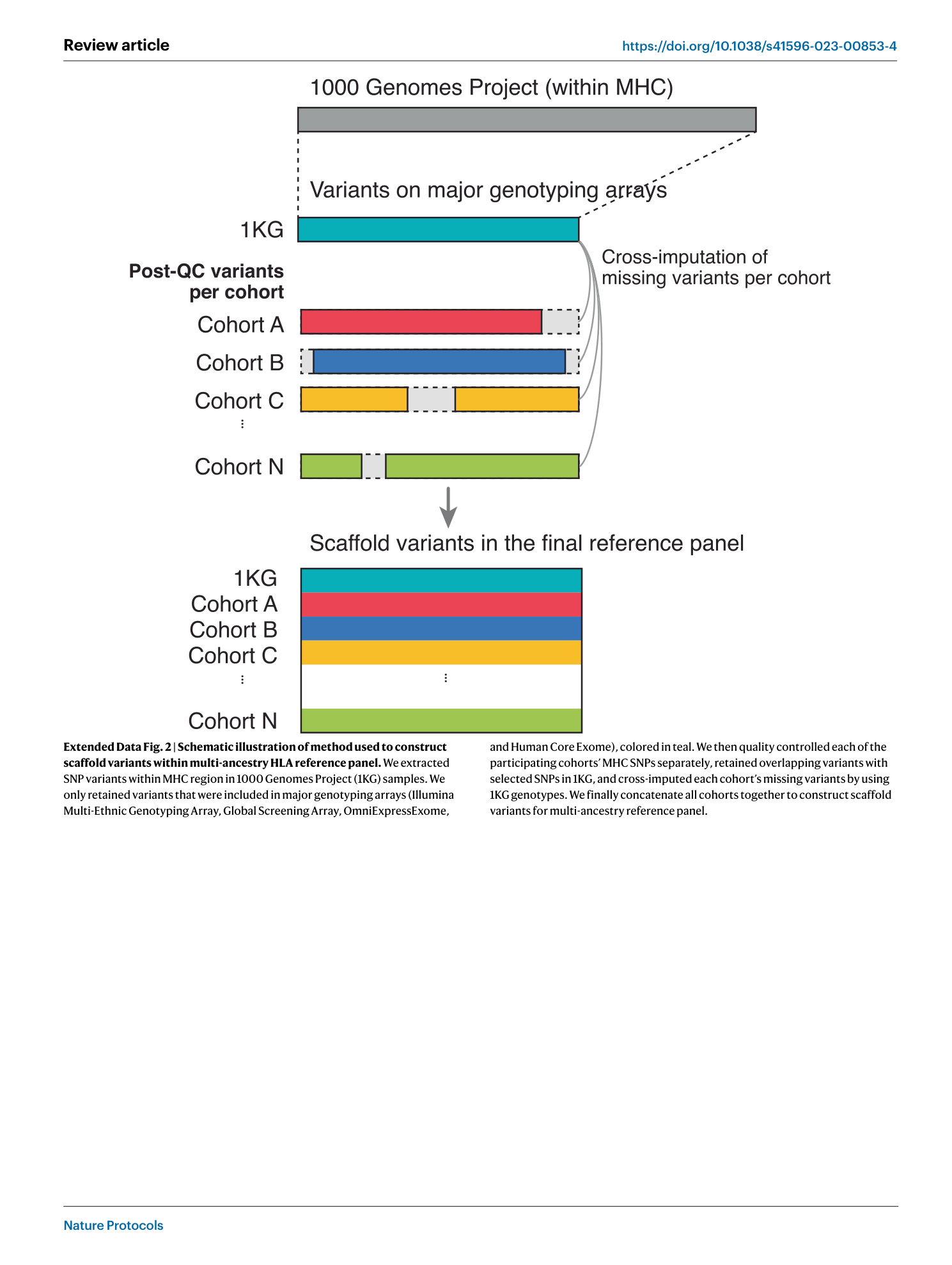
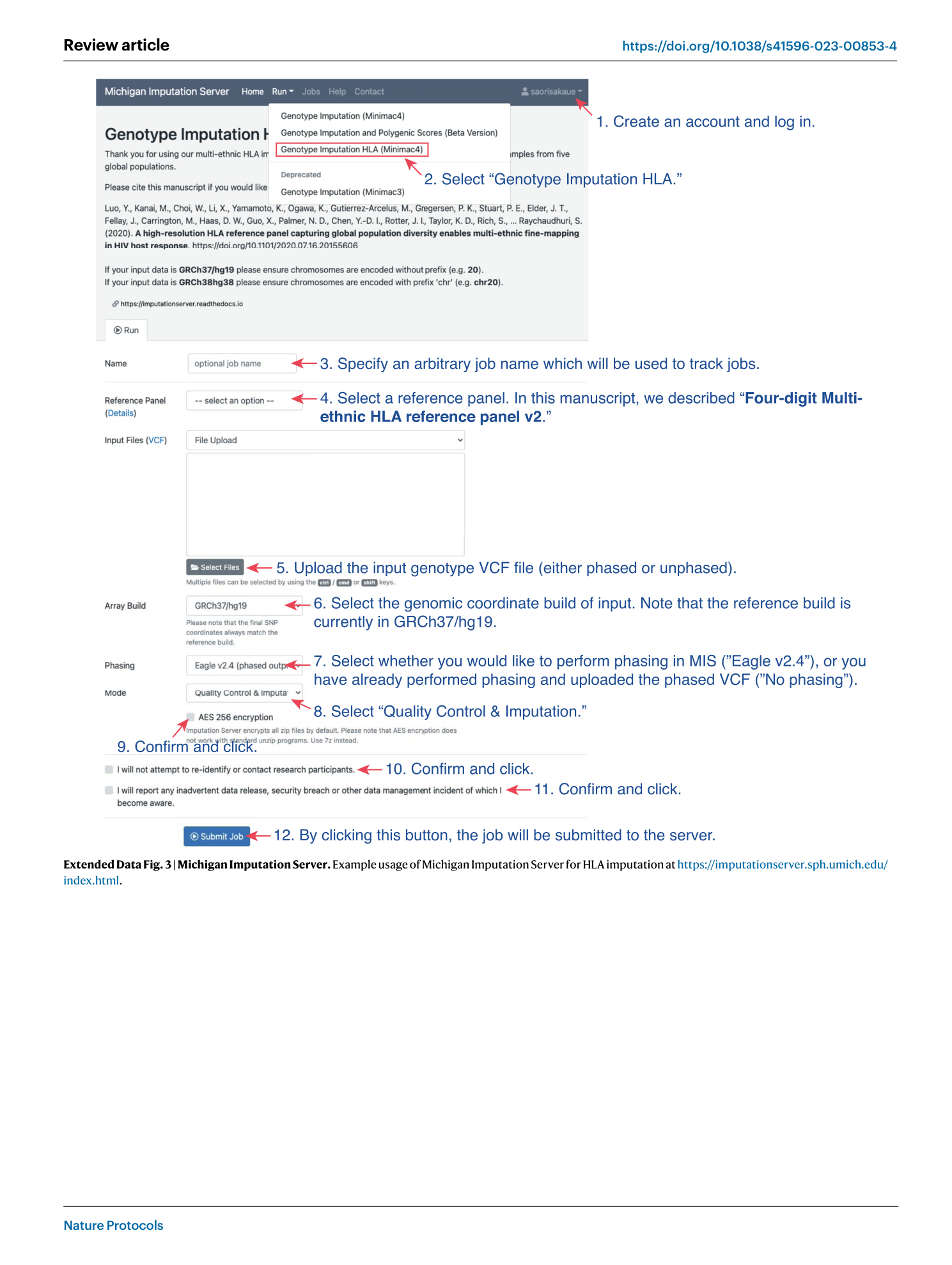
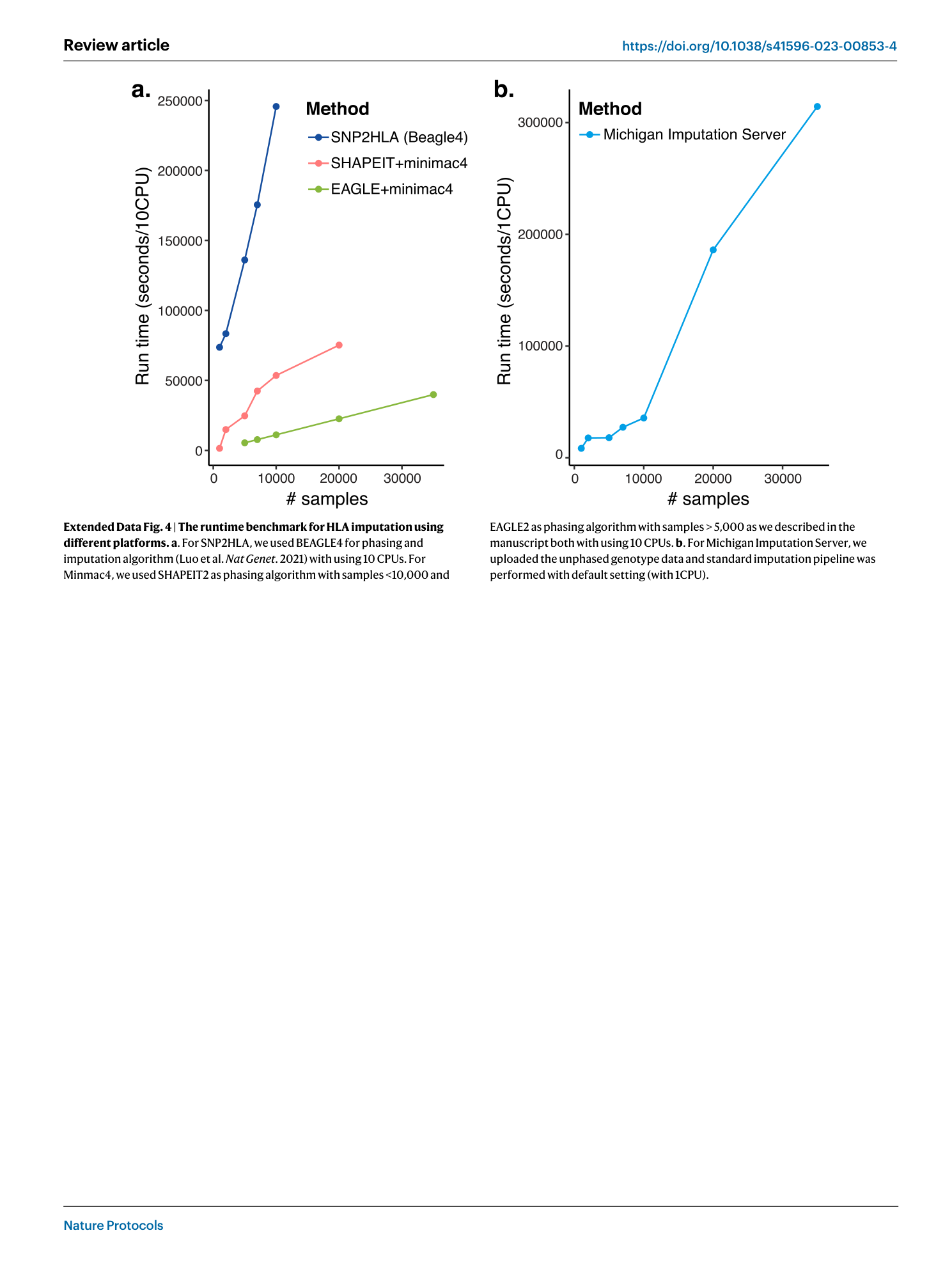
Extended
Extended Data Fig. 1 The linkage disequilibrium (LD) patterns across the extended MHC region.
A heatmap of LD r2 for pairwise variants across the extended MHC region. We used biallelic markers in our HLA reference panel within European populations and calculated LD r 2 values for exhaustive pairs of these variants. The variants are ordered (both on x -axis and y -axis) and annotated by HLA gene names (on x -axis) based on their genomic coordinates on chromosome 6. The bottom plot shows the detailed LD pattern in the class II region.
Extended Data Fig. 2 Schematic illustration of method used to construct scaffold variants within multi-ancestry HLA reference panel.
We extracted SNP variants within MHC region in 1000 Genomes Project (1KG) samples. We only retained variants that were included in major genotyping arrays (Illumina Multi-Ethnic Genotyping Array, Global Screening Array, OmniExpressExome, and Human Core Exome), colored in teal. We then quality controlled each of the participating cohorts’ MHC SNPs separately, retained overlapping variants with selected SNPs in 1KG, and cross-imputed each cohort’s missing variants by using 1KG genotypes. We finally concatenate all cohorts together to construct scaffold variants for multi-ancestry reference panel.
Extended Data Fig. 3 Michigan Imputation Server.
Example usage of Michigan Imputation Server for HLA imputation at https://imputationserver.sph.umich.edu/index.html .
Extended Data Fig. 4 The runtime benchmark for HLA imputation using different platforms.
a . For SNP2HLA, we used BEAGLE4 for phasing and imputation algorithm (Luo et al. Nat Genet . 2021) with using 10 CPUs. For Minmac4, we used SHAPEIT2 as phasing algorithm with samples <10,000 and EAGLE2 as phasing algorithm with samples > 5,000 as we described in the manuscript both with using 10 CPUs. b . For Michigan Imputation Server, we uploaded the unphased genotype data and standard imputation pipeline was performed with default setting (with 1CPU).
Supplementary information
Supplementary Information
Supplementary Table 1.