Quantification of Genetic Heterogeneity Using Long-Read Targeted Individual DNA Molecule Sequencing
Mo Li, Mo Li, Yingzi Zhang, Yingzi Zhang, Arun Pandian Chandrasekaran, Arun Pandian Chandrasekaran, Chongwei Bi, Chongwei Bi
Abstract
Understanding genetic heterogeneity is of paramount importance in unraveling the intricate functioning of biological systems, as it contributes to the diversity of phenotypes of gene-environment interactions. We have developed a method termed targeted Individual DNA Molecule Sequencing (IDMseq) to accurately quantify genetic heterogeneity within cell populations, even those with rare variants present at low frequencies. IDMseq ensures that each original DNA molecule is distinctively represented by one unique molecule identifier (UMI) group, preventing false UMI groups and enabling precise quantification of allele frequency within the original population. IDMseq is a versatile sequencing technique that combines error correction and long-read sequencing, enabling sensitive detection of various genetic variants, including single nucleotide variants and large structural variants in both basic and clinical research settings. This protocol provides a comprehensive, step-by-step guide to preparing samples and performing IDMseq to determine genetic variations. © 2023 The Authors. Current Protocols published by Wiley Periodicals LLC.
Basic Protocol : UMI labeling and amplification of DNA
Support Protocol 1 : AMPure XP beads cleanup
Support Protocol 2 : Suggested data analysis pipeline
INTRODUCTION
Molecular consensus sequencing (MCS) is a technique that utilizes unique molecular identifiers (UMIs) to enhance the accuracy of short-read next-generation sequencing (NGS) (Hiatt et al., 2010; Kinde et al., 2011; Shendure & Ji, 2008). UMIs, short sequences appended to each DNA molecule before sequencing, enable the distinct identification of individual molecules. By integrating UMIs with computational tools, MCS facilitates the correction of random errors introduced during amplification, sequencing, or detection processes. While MCS effectively detects single nucleotide variants (SNVs), its success rate in identifying rare and complex variants has been limited (Lincoln et al., 2021; Petersen et al., 2017).
The advancements in single-molecule sequencing (SMS) have introduced novel avenues for characterizing complex genetic variants with greater precision compared to conventional methods (Alfaro et al., 2021; Macchia et al., 2020; Treffer & Deckert, 2010; Xu et al., 2009). SMS technologies offer the ability to generate long reads, which provide comprehensive insights into genome organization and structure (Bickhart et al., 2017; Thompson & Milos, 2011). However, it is important to note that SMS methods generally exhibit lower raw read accuracy when compared to other sequencing technologies (Salk et al., 2018). To address the limitations associated with both molecular consensus sequencing (MCS) and SMS techniques, we have devised a novel strategy termed targeted Individual DNA Molecule sequencing (IDMseq) (Bi et al., 2020). IDMseq combines the strengths of MCS and SMS while integrating unique molecular identifiers (UMIs) to ensure the distinct representation of each DNA molecule in the sequencing data. This integration allows for error correction through molecular consensus while providing the essential long reads necessary for resolving complex genetic variants with enhanced accuracy and sensitivity. Additionally, a bioinformatics toolkit called Variant Analysis with UMI for Long-read Technology (VAULT) has been developed for data analysis for IDMseq (Bi et al., 2020). Bi et al. (2020) demonstrated the robustness and efficacy of IDMseq and VAULT in characterizing the frequency and molecular feature of the DNA repair outcomes of Cas9 editing. The study showed that Cas9 cleavage induced structural variants (SVs) in ∼4% of edited human embryonic stem cells while a significant proportion of SVs (up to 87%) were recurring deletions and insertions. The study, facilitated by the application of IDMseq and VAULT, provides the first quantitative evidence of nonrandom repair outcomes of Cas9 cutting and hotspots for Cas9-induced large indels. Additionally, Yuan et al. (2022) harnessed IDMseq to identify critical factors that regulate Cas9-induced large deletions and homology-directed repair.
IDMseq represents a robust and innovative tool specifically designed for detecting and quantifying genetic variants. Using this technique, we were able to detect the rare variants down to 10−5 with high fidelity. This methodology demonstrates high efficacy in analyzing rare and complex variants, which often pose challenges for detection using alternative approaches. The current protocol of IDMseq addresses a significant gap in the field of genomics by offering a sensitive and quantitative means of comprehensively capturing the full spectrum of genetic variants, encompassing SNVs, indels, and large SVs.
NOTE : All protocols involving animals must be reviewed and approved by the appropriate Animal Care and Use Committee and must follow regulations for the care and use of laboratory animals. Appropriate informed consent is necessary for obtaining and use of human study material.
Basic Protocol: UMI LABELING AND AMPLIFICATION OF DNA
This basic protocol describes the step-by-step procedures involved in UMI labeling and two rounds of PCR amplification. This protocol assumes users have already prepared their DNA samples of interest. We recommend isolating the DNA using DNeasy Blood & Tissue Kit if working with human samples or an equivalent kit appropriate for the sample.
Materials
- UMI primer, PAGE purified
- 50 to 100 ng input genomic DNA
- Nuclease-free H2O
- 2× Platinum SuperFi PCR Master Mix (Invitrogen, cat. no. 12358010)
- AMPure XP beads (Beckman Coulter, cat. no. A63882)
- Universal primer
- Gene-specific primer
- PrimeSTAR GXL DNA Polymerase (Takara, cat. no. R050)
-
- Qubit 4 fluorometer (Thermo Fisher, cat. no. Q33238)
- NanoDrop 8000 spectrophotometer (Thermo Fisher, cat. no. ND-8000-GL)
- DNeasy Blood & Tissue Kit (Qiagen, cat. no. 69504)
- 0.2-ml thin-walled PCR tubes (VWR, cat. no. 732-0545)
- Thermal cycler
- Ligation Sequencing Kit V14 (SQK-LSK114)
- Flow cell (R10.4.1) (Oxford Nanopore Technologies, FLO-MIN114)
- MinION sequencing device (Oxford Nanopore Technologies)
- Computational hardware
- Sequencing on a MinION Mk1B necessitates a high-spec computer/server capable of effectively handling the data acquisition rate. Sequencing on a MinION Mk1C requires no additional computer/server and screen accessories for nanopore data generation. Refer to the MinION IT requirement document for further details.
UMI primer design
1.Design the UMI primer using software such as Primer3, NCBI primer blast, or other equivalent tools, and order the UMI primer mix with PAGE purification from a reputable oligo synthesis service provider.
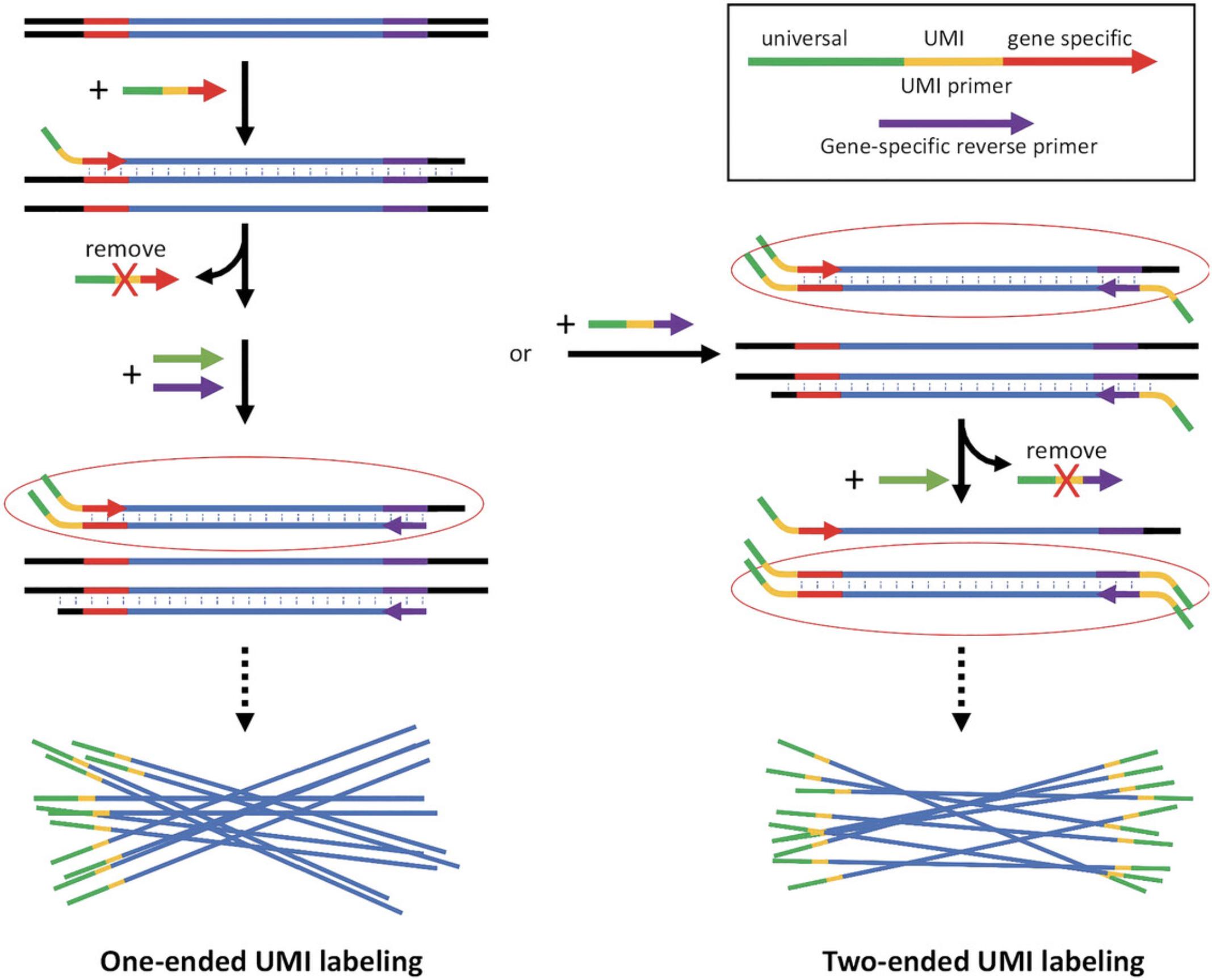
UMI labeling and DNA amplification
2.Quantify the input DNA concentration using a Qubit 4 fluorometer or its equivalent and estimate the purity using a Nanodrop 8000 spectrophotometer or its equivalent.
3.Transfer 50 to 100 ng of input gDNA (up to 10 μl) into a 0.2-ml thin-walled PCR tube. Use nuclease-free H2O to make up the volume.
4.Set up the UMI labeling reaction as follows:
- 50 to 100 ng gDNA
- 1 μM UMI labeling primer mix (a pool of oligos containing random sequences in the UMI segment and shared fixed sequences, see Fig. 1 for design strategy)
- 12.5 μl 2× Platinum SuperFi PCR Master Mix
- H2O to make up the final volume of 25 μl
5.Execute the UMI labeling on a thermocycler with a ramp rate of 1°C/s using the following:
- 98°C 1 min
- 70°C 5 s
- 69°C 5 s
- 68°C 5 s
- 67°C 5 s
- 66°C 5 s
- 65°C 5 s
- 72°C (1 min/kb of DNA of interest)
- 4°C hold
6.Perform AMPure beads cleanup of DNA (see Support Protocol 1).
7.Perform PCR amplification using the universal primer and the gene-specific reverse primer. The PrimeSTAR GXL DNA polymerase is recommended in this step. Follow the manufacturer's guidelines.
8.Perform AMPure beads cleanup of DNA (see Support Protocol 1).
9.To estimate the concentration and quality of the obtained DNA, use a Qubit 4 fluorometer or equivalent for the concentration and a Nanodrop 8000 or equivalent for the quality.
Nanopore sequencing
10.Construct the Nanopore sequencing library with the Ligation Sequencing Kit by following the manufacturer's instructions.
11.Perform Nanopore long-read sequencing with an appropriate flow cell (e.g., FLO-MIN114 for R10.4.1 flow cell on a MinION sequence device) by following the manufacturer's instructions. Ensure supportive computational hardware. Ensure appropriate selection of kits, output file format, and output location. Opt for live basecalling using the guppy basecaller provided by Oxford Nanopore Technologies to convert the raw signals into DNA sequences in fastq format.
Data processing and analysis
12.Follow the desired workflow for alignment and data analysis (see Support Protocol 2 for a suggested pipeline).
Support Protocol 1: AMPURE XP BEADS CLEANUP
This protocol offers guidance on using AMPure XP beads to purify and concentrate DNA samples in Basic Protocol. Oxford Nanopore Technologies recommends the use of AMPure XP beads for this purpose, as it has demonstrated successful recovery of samples with minimal loss.
Materials
- AMPure XP beads (Beckman Coulter, cat. no. A63882)
- Sample tubes to be purified (from Basic Protocol 1, step 6)
- Ethanol (Merck, cat. no. 100983)
- Nuclease-free H2O
-
- Vortex mixer
- Mini centrifuge
- Magnetic rack
- 1.5-ml DNA LoBind microcentrifuge tubes (Eppendorf, cat. no. 022431021)
1.Allow the AMPure XP beads to equilibrate at room temperature for 30 min.
2.Resuspend the beads immediately by vortexing before use.
3.Add the above AMPure beads to the sample tubes in a 1:1 ratio (e.g., 25 μl sample and 25 μl beads).
4.Incubate at room temperature for 5 min and perform step 5 during the 5 min incubation period.
5.Make fresh 70% ethanol using nuclease-free H2O.
6.Using a mini centrifuge, briefly centrifuge the tubes containing the samples at maximum speed.
7.Keep the tube on a magnetic rack and pellet beads until the supernatant is clear.
8.Slowly discard the supernatant.
9.Wash the beads with 200 μl of 70% fresh ethanol. Prepare a fresh 70% ethanol stock solution before experimenting (step 5). While the sample is still on the magnetic stand, add 200 μl of 70% ethanol without disturbing the bead pellet.
10.Repeat steps 8 and 9 two more times.
11.Remove the tube from the magnetic rack and microcentrifuge briefly at maximum speed.
12.Return the tube to the magnetic rack for 2 min.
13.Discard any residual ethanol.
14.Allow the pellet to air dry for 1 min.
15.To elute the DNA off the beads, add 10.5 μl nuclease-free H2O and mix well by pipetting. Incubate the sample at room temperature for 5 min, then place the samples on the magnetic stand for 5 min or until the solution is clear and colorless.
16.Transfer 10 μl of the eluate to a clean 1.5-ml DNA LoBind microcentrifuge tube.
Support Protocol 2: SUGGESTED DATA ANALYSIS PIPELINE
This protocol offers guidance for the analysis of UMI-labeled reads data generated in the Basic Protocol. The bioinformatics toolkit VAULT, along with its required modules are recommended to be installed and implemented in the protocol. VAULT utilizes established algorithms for UMI extraction, alignment, and variant calling (Fig. 2) (Bi, Wang et al., 2023a; Bi, Wang et al., 2023b; Bi et al., 2020).
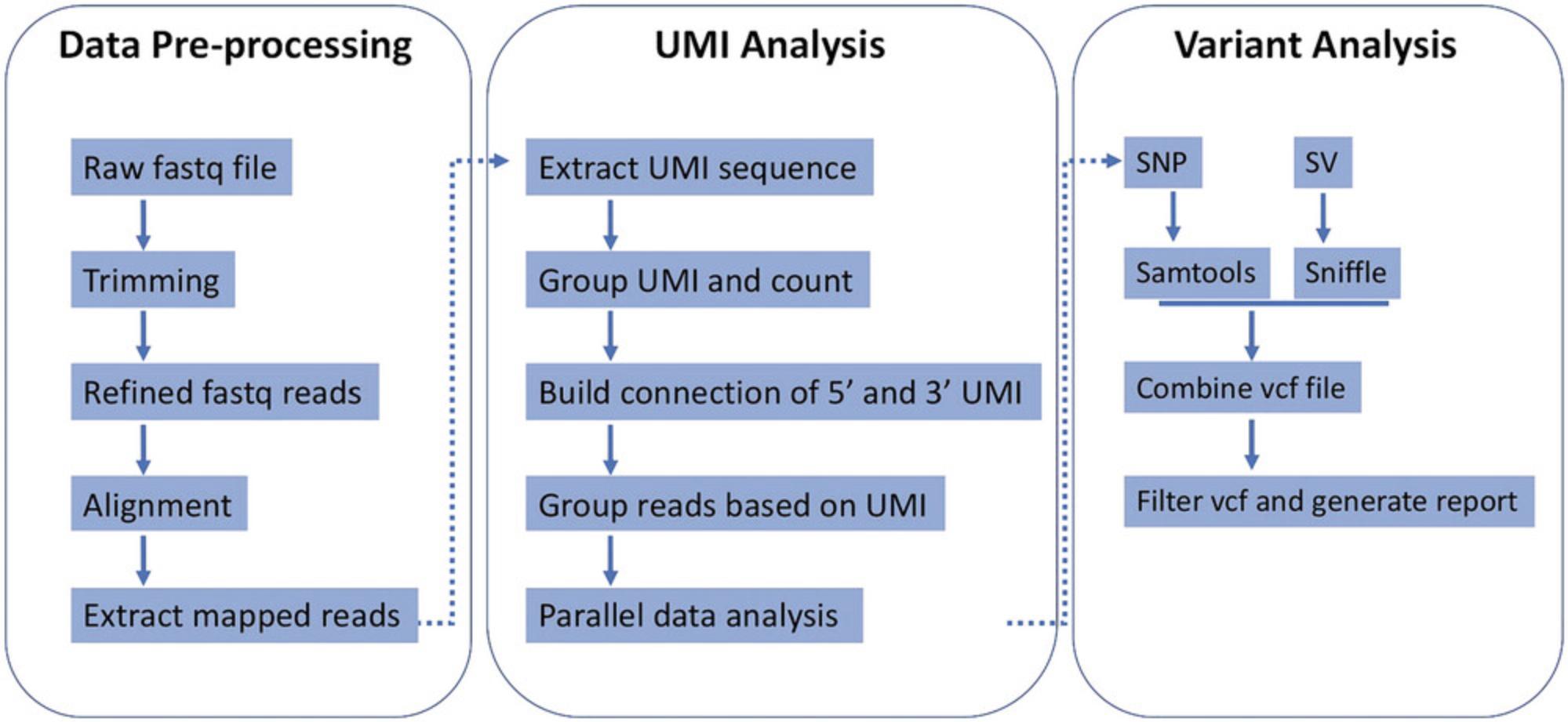
The whole analysis can be performed with one command. The ensuing steps outline the data analysis process (Fig. 2) (Bi et al., 2020). For materials and software dependencies, please refer to the Necessary Resources and Installing VAULT and required modules sections. For usage examples, please refer to the VAULT Usage Example section.
Preprocessing
- Trim Nanopore reads to remove adapter sequences.
- Align the trimmed reads to the reference gene to extract mappable reads.
- Utilize cutadapt to extract the UMI sequences.
UMI grouping and counting
- Count the occurrence of each UMI, which reflects the number of reads in each UMI group.
- Verify the UMI structure if a structured UMI (e.g., NNNNTGNNNN) is used in the experiment.
UMI group filtering
- Define a user-defined threshold for the minimum number of reads per UMI group.
- Bin reads into eligible UMI bins based on the user-defined threshold.
Alignment
- Align the grouped reads using minimap2.
Variant calling
- Perform SNP calling using samtools.
- Perform SV calling using sniffles.
Data cleanup
- Combine individual variant call files (VCF).
- Apply filters to the VCF based on variant quality, depth, and VAF.
- Write the number of reads in UMI groups and the corresponding UMI sequences in the ID field of the VCF.
- Save individual folders named after the UMI sequences. Alignment summaries and BAM files for each UMI group are contained inside the folder.
SNV annotation
- Perform SNV annotation of combined VCF using SnpEff.
Additional features
VAULT supports both long-read data and single-end/paired-end short-read data. VAULT utilizes parallel computing for each UMI group to expedite analysis and avoid crosstalk during data analysis.
COMMENTARY
Background Information
IDMseq ensures that each original DNA molecule is uniquely represented by a single UMI group, comprising reads that share the same UMI, thereby preventing false UMI groups and enabling accurate quantification of allele frequency within the original population. This approach is adaptable to various sequencing platforms. It combines error correction by molecular consensus with long-read sequencing, enabling sensitive detection of all types of genetic variants, including SNVs, indels, large deletions, and complex rearrangements.
IDMseq is accurate in profiling rare somatic mutations, which can be expanded to applications including quantitative assessments of genomic variations, and on-target mutagenesis induced by CRISPR-Cas9. IDMseq holds immense potential in advancing the understanding of the genetic underpinnings of various diseases, while also facilitating the development of novel diagnostic and therapeutic interventions.
Critical Parameters
Primer design
The primer design plays a crucial role in ensuring the specificity of IDMseq. Select a primer sequence with an annealing temperature higher than 65°C to enhance the specificity of the primer to the target gene. Choose a short UMI sequence (10 to 12 nucleotides) to reduce the chance of sequencing errors within the UMI and enhance the accuracy of downstream analysis. Adopt a unique sequence structure in the UMI (e.g., NNNNTGNNNN) to avoid homopolymer regions that may introduce errors due to polymerase slippage or lower accuracy of Nanopore sequencing in such sequences. Maintaining a balanced CpG content in the primers for optimal performance.
Available disk space
Ensuring sufficient free space is crucial to guarantee a successful sequencing run. When the drive approaches its maximum capacity during the run, the MinKNOW software program will automatically terminate the run. Rebooting is recommended before the sequencing run to avoid any unexpected disruptions during the sequencing process.
Flow cell quality and circuit integrity
Assessing the quality of the nanopore flow cell before initiating the sequencing process is critical for achieving successful sequencing outcomes and efficient data accumulation. A Flow cell check should be conducted using the MinKNOW software program. The reported number of available pores serves as a reliable indicator of the flow cell quality.
Throughout the flow cell loading process, utmost care must be taken to prevent air bubbles from the application-specific integrated circuit (ASIC). The sequencing outcome would substantially be decreased due to air bubbles.
Compatibility of materials and software
The Oxford Nanopore Technologies library preparation kits, flow cells, and software employed in this protocol undergo continuous development and refinement. These advancements aim to enhance the quality of the obtained read data. However, ensuring compatibility between the specific library preparation kit, the flow cell version, and the software components is crucial to guarantee a smooth and successful sequencing and data analysis.
Troubleshooting
See Table 1 for a list of problems, possible causes, and solutions.
Problem | Possible cause | Solution |
---|---|---|
Loss of DNA during library preparation | Loss of DNA during the bead cleanup step due to insufficient pelleting of the beads or inadequate pellet drying; insufficient pelleting can result in the beads being pipetted and discarded along with the supernatant; inadequate drying leaves residual ethanol in the tube inhibiting the downstream steps of library preparation | Ensure that the beads are tightly pelleted, and the pellet is dried for the appropriate amount of time; the pellet may dry partially if the drying time is short, or the beads may dry out if the drying time is too long; using 80% ethanol instead of 70% ethanol can help accelerate the drying process; ensure that the pellet dries properly by watching it closely and allowing it to dry until it is dull but not cracked |
Low numbers of UMI-containing reads in data analysis | Inefficient PCR amplification of UMI-labeled individual DNA molecules | Users should try different DNA template amounts during PCR amplification; increasing the number of PCR amplification cycles or re-designing primers may help optimize the amplification efficiency and improve yield of UMI-labeled DNA molecules |
Insufficient amounts of input DNA within the sequencing library | Users should increase the DNA input amount during the library preparation process or perform multiple sequencing runs to increase the sequencing coverage |
Understanding Results
Through the IDMseq approach, individual DNA molecules are labeled with unique UMIs and amplified for sequencing on appropriate platforms (e.g., Illumina, PacBio, and Nanopore). During data analysis, reads are binned by UMIs to correct errors introduced during amplification and sequencing processes. Every UMI group represents an original allele in the genome of the initial population. The analysis pipeline encompasses both SNV and SV calling, providing a comprehensive evaluation of genetic variations.
Users can refer to the Data Availability Statement section for an example dataset of Nanopore sequencing data.
Below is a result example using VAULT:
./result/ ├── 20210622_08.46.11_vault.log # VAULT log ├── nanopore_reads.300-20000_alignment_summary.log # raw reads alignment summary ├── nanopore_reads.300-20000.bam ├── nanopore_reads.300-20000.bam.bai ├── nanopore_reads.300-20000.fastq ├── nanopore_reads.300-20000.mapped.fastq # length filtered and alignment mapped reads used in the VAULT analysis ├── nanopore_reads.300-20000.mapped.lst ├── nanopore_reads.300-20000.sam ├── grouped_reads # fastq reads for every UMI groups │ └── perfect_umi ├── snp # folder for variant analysis │ ├── all_snp_from_perfect_umi.vcf # raw variant calling result for small variants │ ├── all_snp_from_perfect_umi.pcent.vcf # add variant allele frequency (supported reads percentage) in the info field of vcf file │ ├── all_snp_from_perfect_umi.pcent.rem.vcf # remove wrong UMI group │ ├── all_snp_from_perfect_umi.pcent.rem.flt.vcf # filter by depth, quality, allele frequency │ ├── all_sv_from_perfect_umi.vcf # raw variant calling result for large variants (>= 30bp) │ ├── all_sv_from_perfect_umi.filtered.0.67.vcf # FINAL SV result (remove wrong UMI group and filter SVs by allele frequency [0.5]) │ ├── all_sv_from_perfect_umi.filtered.0.67.sorted.vcf # sort by position │ ├── coverage.3plus.txt # The region with coverage >= 3 in each UMI groups, can be used to filter out UMI groups │ ├── pass.group.lst # UMI groups that pass group_filter │ ├── pass_snp_from_perfect_umi.flt.vcf # FINAL SNV and InDel result (remove wrong UMI group and filter by depth, quality, allele frequency) │ ├── umi_group.flt.summary.txt # intermediate file in [–group_filter] │ ├── wrong.group.lst # UMI groups that fail in [–group_filter] │ ├── wrong.group.summary.txt # intermediate file in [–group_filter] for wrong UMI groups │ └── perfect_umi # individual UMI analysis result for 5' and 3' end of reads └── umi_analysis ├── 3end_UMIs └── 5end_UMIs
In the "snp" folder, the files with "all" prefix mean that they are from all UMI groups in ./snp/perfect_umi folder. The files with "pass" prefix mean they are from "–group_filter" passed UMI groups, which is shown in ./snp/pass.group.lst.
Below is an example of an individual UMI group folder:
./result/snp/perfect_umi/ ├── 14_ATCGATGATTTT_AAAATCATCGAT # 14 reads in this group, 5' UMI is ATCGATGATTTT, 3' is AAAATCATCGAT ├── 33_GACATTGTCTGG_CCAGACAATGTC ├── 35_5end_AACAGTGCTGCT # 35 reads in this group, all reads from 5' UMI ├── 5_3end_AAAAACATGGCA # 5 reads in this group, all reads from 3' UMI ├── 7_5end_ATTCTTGGTGTC ├── 7_CTATGTGAAGAA_TTCTTCACATAG ├── 8_3end_ACAAGCAAAAAA ├── 8_AGTTGTGCCATA_TATGGCACAACT ├── 8_CCGCGTGAGATG_CATCTCACGCGG ├── 8_CGTTGTGTTACT_AGTAACACAACG ├── 8_CTATTTGTCACT_AGTGACAAATAG ├── 8_GGGTTTGGTTTG_CAAACCAAACCC ├── 8_GTGGGTGACGGG_CCCGTCACCCAC ├── 8_GTGTTTGTTAGA_TCTAACAAACAC ├── 8_TCAATTGCAGAA_TTCTGCAATTGA ├── 8_TTACTTGATTTT_AAAATCAAGTAA ├── 8_TTGGATGGAAGT_ACTTCCATCCAA ├── 9_AAAGATGCGCGT_ACGCGCATCTTT ├── 9_AGAAATGATAGC_GCTATCATTTCT ├── 9_ATCGATGGTGCG_CGCACCATCGAT ├── 9_ATGTTTGCCAAT_ATTGGCAAACAT ├── 9_CTAACTGCTTAT_ATAAGCAGTTAG ├── 9_GAGAATGAGTAC_GTACTCATTCTC └── 9_GTTTATGTACAT_ATGTACATAAAC
VAULT generates a comprehensive summary of individual sequencing runs, providing essential metrics that enable a general understanding of the sequencing results, including the number of UMI-containing reads and identified UMI groups, read number per UMI group, variant constitution, and variant allele frequency (calculated by mutant molecule percentage).
Below is an example of VAULT summary:
raw_read_number is: 252 used_read_number is: 246 reads_with_umi is: 246 detected_molecule_number is: 24 # detected UMI groups(molecule) detected_passed_molecule_number is: 21 # filtered-passed UMI groups(molecule) refer_seq_length is: 7077 covered_region_of_molecule(avg,median,min,max) is: 5784.67,6858,1582,7077 # length of regions with >=3 depth in each UMI group p95_coverage_molecule is: 12 # UMI groups(molecule) with more than 95% of regions covered by >=3 depth molecule_with_snv is: 16 total_snv_number is: 63 unique_snv_number is: 14 normalized_snv_number_per_SNVContainingMolecule(avg,median,min,max) is: 4.00683,4.04804,1.00493,9.00194 total_somatic_snv_number is: 0 # somatic SNV is defined as SNVs with VAF < $threshold (defined by -T) unique_somatic_snv_number is: 0 somatic_snv_load_per_Mbp is: 0 molecule_with_sv is: 16/76.19% total_sv_number is: 32 unique_sv_number is: 13 molecule_with_deletion is: 15/71.43% # Below shows only deletion, insertion, inversion, and duplication. For more information of SVs, please check ./example/result/snp/summary/all_sv.1count.2pos.3type.4length.txt total_deletion is: 30 molecule_with_insertion is: 1/4.76% total_insertion is: 2 molecule_with_inversion is: 0/0.00% total_inversion is: 0 molecule_with_duplication is: 0/0.00% total_duplication is: 0
A more detailed interpretation of the detected genetic variations can be achieved by manually analyzing VCF files.
Time Considerations
UMI labeling and amplification process require 16 to 24 hr to complete. Nanopore sequencing library preparation requires 3 hr to complete. The sequencing run can range from 24 to 48 hr, depending on the sequencing performance and data amount requirement of the experiment. For the subsequent data analysis, a typical VAULT analysis of 2.5 million long reads is estimated to take around 4 hr on a 32-core workstation. The actual time required for the VAULT analysis may vary based on the specific hardware configurations and dataset characteristics.
Acknowledgments
The work was supported by KAUST Office of Sponsored Research (OSR), under the award number BAS/1/1080-01.
Author Contributions
Yingzi Zhang : Conceptualization, Methodology, Project administration, Supervision, Validation, Writing original draft, Writing review and editing; Arun Pandian Chandrasekaran : Writing original draft, Writing review and editing; Chongwei Bi : Conceptualization, Data curation, Formal analysis, Investigation, Methodology, Project administration, Resources, Software, Supervision, Validation, Writing review and editing; Mo Li : Conceptualization, Funding acquisition, Methodology, Project administration, Resources, Supervision, Validation, Writing review and editing
Conflict of Interest
The authors declare no conflict of interest.
Open Research
Data Availability Statement
The example dataset of Nanopore sequencing data is available in the SRA database (accession ID PRJNA606194), which is accessible with the following link: https://www.ncbi.nlm.nih.gov/bioproject/PRJNA606194. The example of sample data using VAULT is accessible at GitHub under the GPL-3.0 open-source license.
Literature Cited
- Alfaro, J. A., Bohländer, P., Dai, M., Filius, M., Howard, C. J., van Kooten, X. F., Ohayon, S., Pomorski, A., Schmid, S., Aksimentiev, A., Anslyn, E. V., Bedran, G., Cao, C., Chinappi, M., Coyaud, E., Dekker, C., Dittmar, G., Drachman, N., Eelkema, R., … Joo, C. (2021). The emerging landscape of single-molecule protein sequencing technologies. Nature Methods , 18, 604–617. https://doi.org/10.1038/s41592-021-01143-1
- Bi, C., Wang, L., Fan, Y., Yuan, B., Alsolami, S., Zhang, Y., Zhang, P. Y., Huang, Y., Yu, Y., Izpisua Belmonte, J. C., & Li, M. (2023a). Quantitative haplotype-resolved analysis of mitochondrial DNA heteroplasmy in human single oocytes, blastoids, and pluripotent stem cells. Nucleic Acids Research , 51, 3793–3805. https://doi.org/10.1093/nar/gkad209
- Bi, C., Wang, L., Fan, Y., Yuan, B., Ramos-Mandujano, G., Zhang, Y., Alsolami, S., Zhou, X., Wang, J., Shao, Y., Reddy, P., Zhang, P. Y., Huang, Y., Yu, Y., Izpisua Belmonte, J. C., & Li, M. (2023b). Single-cell individual full-length mtDNA sequencing by iMiGseq uncovers unexpected heteroplasmy shifts in mtDNA editing. Nucleic Acids Research , 51, e48–e48. https://doi.org/10.1093/nar/gkad208
- Bi, C., Wang, L., Yuan, B., Zhou, X., Li, Y., Wang, S., Pang, Y., Gao, X., Huang, Y., & Li, M. (2020). Long-read individual-molecule sequencing reveals CRISPR-induced genetic heterogeneity in human ESCs. Genome Biology , 21, 213. https://doi.org/10.1186/s13059-020-02143-8
- Bickhart, D. M., Rosen, B. D., Koren, S., Sayre, B. L., Hastie, A. R., Chan, S., Lee, J., Lam, E. T., Liachko, I., Sullivan, S. T., Burton, J. N., Huson, H. J., Nystrom, J. C., Kelley, C. M., Hutchison, J. L., Zhou, Y., Sun, J., Crisà, A., Ponce de León, F. A., … Smith, T. P. (2017). Single-molecule sequencing and chromatin conformation capture enable de novo reference assembly of the domestic goat genome. Nature Genetics , 49, 643–650. https://doi.org/10.1038/ng.3802
- Hiatt, J. B., Patwardhan, R. P., Turner, E. H., Lee, C., & Shendure, J. (2010). Parallel, tag-directed assembly of locally derived short sequence reads. Nature Methods , 7, 119–122. https://doi.org/10.1038/nmeth.1416
- Kinde, I., Wu, J., Papadopoulos, N., Kinzler, K. W., & Vogelstein, B. (2011). Detection and quantification of rare mutations with massively parallel sequencing. Proceedings of the National Academy of Sciences , 108, 9530–9535. https://doi.org/10.1073/pnas.1105422108
- Lincoln, S. E., Hambuch, T., Zook, J. M., Bristow, S. L., Hatchell, K., Truty, R., Kennemer, M., Shirts, B. H., Fellowes, A., Chowdhury, S., Klee, E. W., Mahamdallie, S., Cleveland, M. H., Vallone, P. M., Ding, Y., Seal, S., DeSilva, W., Tomson, F. L., Huang, C., … Nussbaum, R. L. (2021). One in seven pathogenic variants can be challenging to detect by NGS: An analysis of 450,000 patients with implications for clinical sensitivity and genetic test implementation. Genetics in Medicine , 23, 1673–1680. https://doi.org/10.1038/s41436-021-01187-w
- Macchia, E., Manoli, K., Di Franco, C., Scamarcio, G., & Torsi, L. (2020). New trends in single-molecule bioanalytical detection. Analytical and Bioanalytical Chemistry , 412, 5005–5014. https://doi.org/10.1007/s00216-020-02540-9
- Petersen, B.-S., Fredrich, B., Hoeppner, M. P., Ellinghaus, D., & Franke, A. (2017). Opportunities and challenges of whole-genome and -exome sequencing. BMC Genetics , 18, 14. https://doi.org/10.1186/s12863-017-0479-5
- Salk, J. J., Schmitt, M. W., & Loeb, L. A. (2018). Enhancing the accuracy of next-generation sequencing for detecting rare and subclonal mutations. Nature Reviews Genetics , 19, 269–285. https://doi.org/10.1038/nrg.2017.117
- Shendure, J., & Ji, H. (2008). Next-generation DNA sequencing. Nature Biotechnology , 26, 1135–1145. https://doi.org/10.1038/nbt1486
- Thompson, J. F., & Milos, P. M. (2011). The properties and applications of single-molecule DNA sequencing. Genome Biology , 12, 217. https://doi.org/10.1186/gb-2011-12-2-217
- Treffer, R., & Deckert, V. (2010). Recent advances in single-molecule sequencing. Current Opinion in Biotechnology , 21, 4–11. https://doi.org/10.1016/j.copbio.2010.02.009
- Xu, M., Fujita, D., & Hanagata, N. (2009). Perspectives and challenges of emerging single-molecule DNA sequencing technologies. Small , 5, 2638–2649. https://doi.org/10.1002/smll.200900976
- Yuan, B., Bi, C., Wang, J., Jin, Y., Alsayegh, K., Tehseen, M., Yi, G., Hamdan, S., Huang, Y., & Li, M. (2022). Modulation of the microhomology-mediated end joining pathway suppresses large deletions and enhances homology-directed repair following CRISPR-Cas9-induced DNA breaks. BioRxiv , 2022.2011.2016.516713.
Internet Resources
Python3.7
Anaconda
Miniconda
Cutadapt: extracting UMI sequence and counting the occurrence of each UMI.
Guppy: base-calling from Nanopore raw signals; registration is required to download.
Minimap2: Read alignment.
Samtools (v1.9): SNV calling and filtering.
Sniffles (v1.0.11): SV calling.
SnpEff (v4.3): SNV annotation.
VAULT: UMI extraction, alignment, and variant calling.