Negative-Stain Transmission Electron Microscopy of Molecular Complexes for Image Analysis by 2D Class Averaging
John R. Gallagher, John R. Gallagher, Alexander J. Kim, Alexander J. Kim, Neetu M. Gulati, Neetu M. Gulati, Audray K. Harris, Audray K. Harris
Abstract
Negative-stain transmission electron microscopy (EM) is a technique that has provided nanometer resolution images of macromolecules for about 60 years. Developments in cryo-EM image processing have maximized the information gained from averaging large numbers of particles. These developments can now be applied back to negative-stain image analysis to ascertain domain level molecular structure (10 to 20 Å) more quickly and efficiently than possible by atomic resolution cryo-EM. Using uranyl acetate stained molecular complexes of influenza hemagglutinin bound to Fab 441D6, we describe a simple and efficient means to collect several hundred micrographs with SerialEM. Using RELION, we illustrate how tens of thousands of complexes can be auto-picked and classified to accurately describe the domain level topology of this unconventional hemagglutinin head-domain epitope. By comparing to the cryo-EM density map of the same complex, we show that questions about epitope mapping and conformational heterogeneity can readily be answered by this negative-stain method. © 2019 The Authors.
INTRODUCTION
Technical advances in cryo-electron microscopy (cryo-EM) have drawn attention for atomic resolution structure determination of macromolecular complexes at a revolutionary pace (Kuhlbrandt, 2014). Yet, even as infrastructure supporting atomic resolution cryo-EM has expanded rapidly, obtaining time for data collection on high-resolution (field emission gun operated at 300 kV with a direct electron detector) transmission electron microscopes (TEM) can be a bottleneck to progress for a research project. Meanwhile, low-voltage (80-120 kV) TEMs have already been widely procured. Low-voltage TEM designs, such as the Philips CM10 and Tecnai 12, have been workhorses since the 1980s. Continued improvements in microscope components and hardware updates have improved vacuum robustness, stage stability, and filament brightness. Upgraded software has made microscope operation and alignment more accessible to non-experts. Improvements in image acquisition have also been enormous, summarized by how digital cameras replaced film. Automatic drift correction, recently available in complementary metal-oxide-semiconductor (CMOS) cameras, can now compensate dramatically for stage instability. Automatic drift correction allows a staggering amount of data to be acquired quickly since it permits the stage stabilization delay between image acquisitions to be inconsequentially small. Negative-stain TEM is perfectly suited to a low-voltage TEM microscope, where the absolute resolution is limited by the grain size of the negative stain. Grain sizes range from 4 to 5 Å for uranium-based stains, which typically results in structural information on a biological complex to about 10 to 20 Å resolution (Scarff, Fuller, Thompson, & Iadaza, 2018).
In general, lower voltage microscopes (e.g., 100 kV) used for negative stain are easier to use and maintain than their higher voltage cryo-EM equivalents (e.g., 300 kV). As a result, low-voltage microscopes are often underutilized and undervalued compared to their high-voltage counterparts. Additionally, negative-stain images are inherently higher contrast than cryo-EM due to the heavy metal stain, and thus require fewer particles for data analysis. Even conformationally heterogeneous samples can be well suited to negative-stain analysis, as the image contrast for individual particles is sufficient to guide proper image classification (Bianchi et al., 2018; Ohi, Li, Cheng, & Walz, 2004). By using fewer particles, negative-stain data analysis can commonly be performed on a workstation computer, instead of a computer cluster commonly utilized for cryo-EM. Together, these attributes permit a streamlined rendition of the cryo-EM data collection and processing workflow to be applied to negative-stain data collection and analysis from low-voltage TEM microscopes already in service. The result of this revised negative-stain workflow is improved resolution of the biological complex, and a better understanding of the diversity of structures among the population of imaged complexes.
Information from 2D-image analysis of macromolecules from negative-stain EM images are important for a number of reasons. First, information about the molecular morphology, organization, and heterogeneity of samples can be obtained. Second, negative-stain structural data can be obtained for a larger number of samples in a given amount of time than possible with cryo-EM, allowing many samples to be screened before pursuing atomic resolution cryo-EM. Lastly, structural information from negative-stain imaging can be coupled with other information such as antibody competition data or functional data, to gain insights into the structure of macromolecules.
In this article, we discuss the steps following sample purification through generation of representative 2D class averages that visualize the most common orientations of the particle. This article aims to depict the workflow moving from particles in buffer to a large number of electron micrographs, and then analyzing these micrographs by 2D class averaging. 2D class averages enable robust conclusions founded upon the full dataset, rather than relying on individual particles in single micrographs to be representative. Furthermore, the increased signal-to-noise of negatively stained sample facilitates dramatically faster data acquisition and data analysis times than cryo-EM, enabling categorically different experimental questions to be addressed, such as epitope mapping of Fabs derived from polyclonal sera (Bianchi et al., 2018). This article targets existing users of low-voltage TEM microscopes who wish to add 2D classification to an existing workflow, as well as individuals who may have access to an underutilized low-voltage TEM microscope, yet do not have an established workflow. A primary motivation to implement a workflow such as described in this article is to draw overarching conclusions about the entire population of particles captured by EM imaging.
As an example for this article, we use the molecular complex of the antibody fragment antigen-binding (Fab) 441D6 in complex with influenza hemagglutinin (HA) A/New York/653/1996. The epitope of the 441D6 antibody targets the immunodominant head region of influenza hemagglutinin, yet 441D6 is unique in that it has broad activity against viruses spanning 90 years of evolution by the H1 subtype of influenza. The structure of this complex was previously published as a cryo-EM map at 8 Å resolution (Kanekiyo et al., 2019), and this map is available in the EM data bank as entry EMD-7021. The HA ectodomain in this complex is just 10 nm tall, but the 3 Fabs bound to HA add substantial information to the images that aid in particle alignment during 2D classification. Basic Protocol 1 addresses the collection of images in a semi-automated fashion to arrive at sufficiently high particle counts for analysis. Support Protocol describes preparation of an EM grid by negative stain before images can be collected. Basic Protocol 2 enumerates the computational steps for analyzing a set of images, resulting in a set of representative 2D images summarizing all particles observed in the sample. Using the protocols presented here, structural information on different macromolecules could be obtained and coupled to other data from techniques such as microbiology, biochemistry, virology, and immunology. This would facilitate understanding of molecular structure and function for a broad-range of projects of interest to scientists across disciplines.
Basic Protocol 1: DATA ACQUISITION
With an EM grid prepared (see the Support Protocol), the first milestone is to collect a large number of micrographs, containing approximately 50,000 particles in total. Towards this goal, the first step is to evaluate if the grid is good, or, at the least, better than previously acquired data. If the grid is not good, a new grid should be remade before continuing. A good grid has good contrast for individual particles, and good particle dispersion (see Critical Parameters and Troubleshooting). When a grid of suitable quality is made, the objective becomes to collect a much data as possible in a short amount of time. The data should be collected close to focus to minimize the importance of the contrast transfer function (CTF) correction, yet with enough defocus to properly fit the defocus value. Using a camera that supports motion correction can greatly reduce the dwell time for stage settling between camera shots, such as implemented in the OneView camera (Gatan, Warrendale, PA).
Classically, a “micrograph” referred to the picture taken on film from the electron microscope. Now with digital cameras, the term “image” is commonly used, and often “image” and “micrograph” can be used interchangeably. “Micrograph” may be more appropriate when describing the immutable raw data for analysis, as compared to the processed images during analysis. Meanwhile “image” more commonly refers to everything coming from the electron microscope camera, especially data which is used only transiently while configuring the microscope.
Generally, collecting more micrographs is better for data analysis, but available compute resources will impose a limit on the dataset size that can be efficiently processed. Looking forward to data processing, 2D classification in RELION may behave differently with when 2D classifying large numbers of particles, compared to the same data processed in smaller batches. Yet working with multiple batches of 2D classifications is primarily recommended for curating giant datasets for 3D reconstruction by cryo-EM, not necessarily negative stain. If more than 50,000 particles are used in a single 2D classification, it can become difficult to parameterize the classification to create the desired separation between classes. For the high contrast of negative stain, 20,000 particles can be very effective, and as few as 2,000 to 5000 particles in a dataset can sometimes reveal important insights beyond what is visible in single micrographs. While sample-specific factors will influence the count of micrographs desired, if one assumes 150 particles per micrograph and a target of 20,000 total particles, then roughly 130 micrographs are needed.
This article assumes the EM microscope alignments and the software package SerialEM (Mastronarde, 2005) have been properly parameterized previously. Many resources for SerialEM are available at varying levels of engagement (see Internet Resources). We note that in a context of EM software that typically fluctuate in stability as they are developed, SerialEM software and support has categorically exhibited sustained excellence.
Materials
-
Liquid nitrogen
-
Negatively stained sample prepared on an EM grid (see Support Protocol)
-
FEI Tecnai 12 electron microscope (operated at 80, 100, or 120 kV), or equivalent TEM microscope
-
Digital camera containing:
- Preferably with a 4k sensor size, or larger, to maximize particles per micrograph
- Preferably with drift compensation, to avoid significant stage settling delays
-
SerialEM installation (requires FEI scripting interface software add-on)
Prepare the microscope
1.Chill the microscope cold finger with liquid nitrogen.
2.Turn on high voltage to the filament and ramp up the current to the filament to bring it to operating temperature.
3.Wait 30 min to 1 hr for the microscope cold finger to chill.
4.Open the column valves and confirm the electron beam is visible on the phosphor screen.
5.Close the column valves in preparation to load an EM grid.
6.Load EM grid in the microscope sample holder and insert the sample into microscope.
7.After the microscope vacuum recovers and stabilizes, open the column valves to reveal the electron beam.
8.Find the electron beam.
Parameterize the sample
9.Bring the sample to a Z-height previously known to be near eucentric for this microscope sample holder.
10.Find a visible feature on the carbon surface of the grid at an intermediate magnification (e.g., 9.7 kX).
11.Set the proper eucentric height for this sample (e.g., use the alpha-wobbler on a FEI Tecnai 12).
12.Find focus on the sample, using the visible feature on the carbon surface.
13.Increase the magnification to that desired for acquisition [e.g., 59 kX at the phosphor screen, which becomes 67 kX (1.8 Å/pixel) at the camera for a bottom-mount Gatan OneView].
14.Adjust the beam to a brightness appropriate to the camera.
15.Find focus on the molecules of interest using the camera.
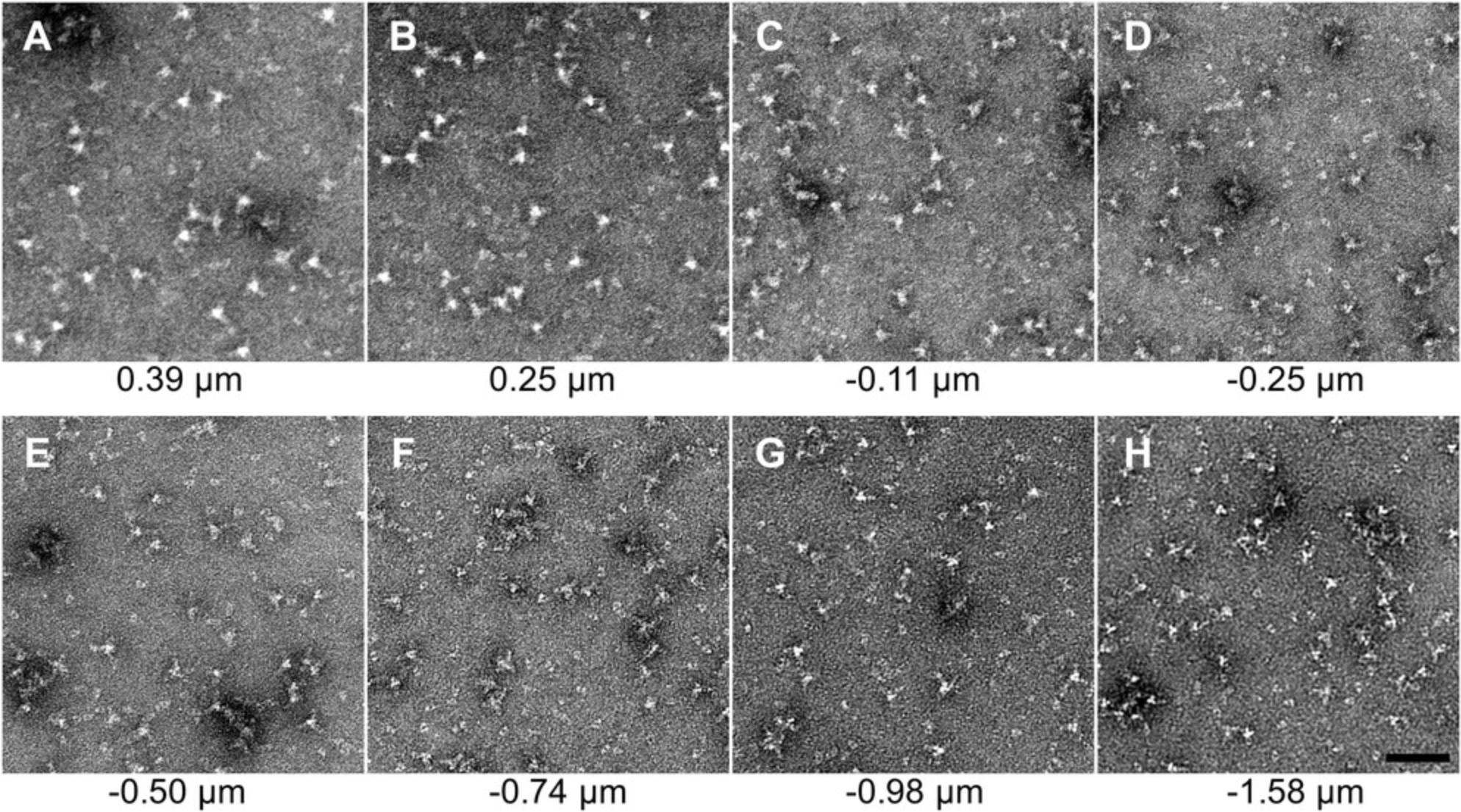
16.Confirm distinct molecules of the samples are visualized.
EM imaging setup
17.Confirm “tune” alignments of EM microscope.
18.Open the software application SerialEM, if not already open.
19.Acquire a “Record” image (“Camera and Script Controls” Panel → “Record”) with SerialEM to confirm camera and SerialEM operability (Fig. 2A).
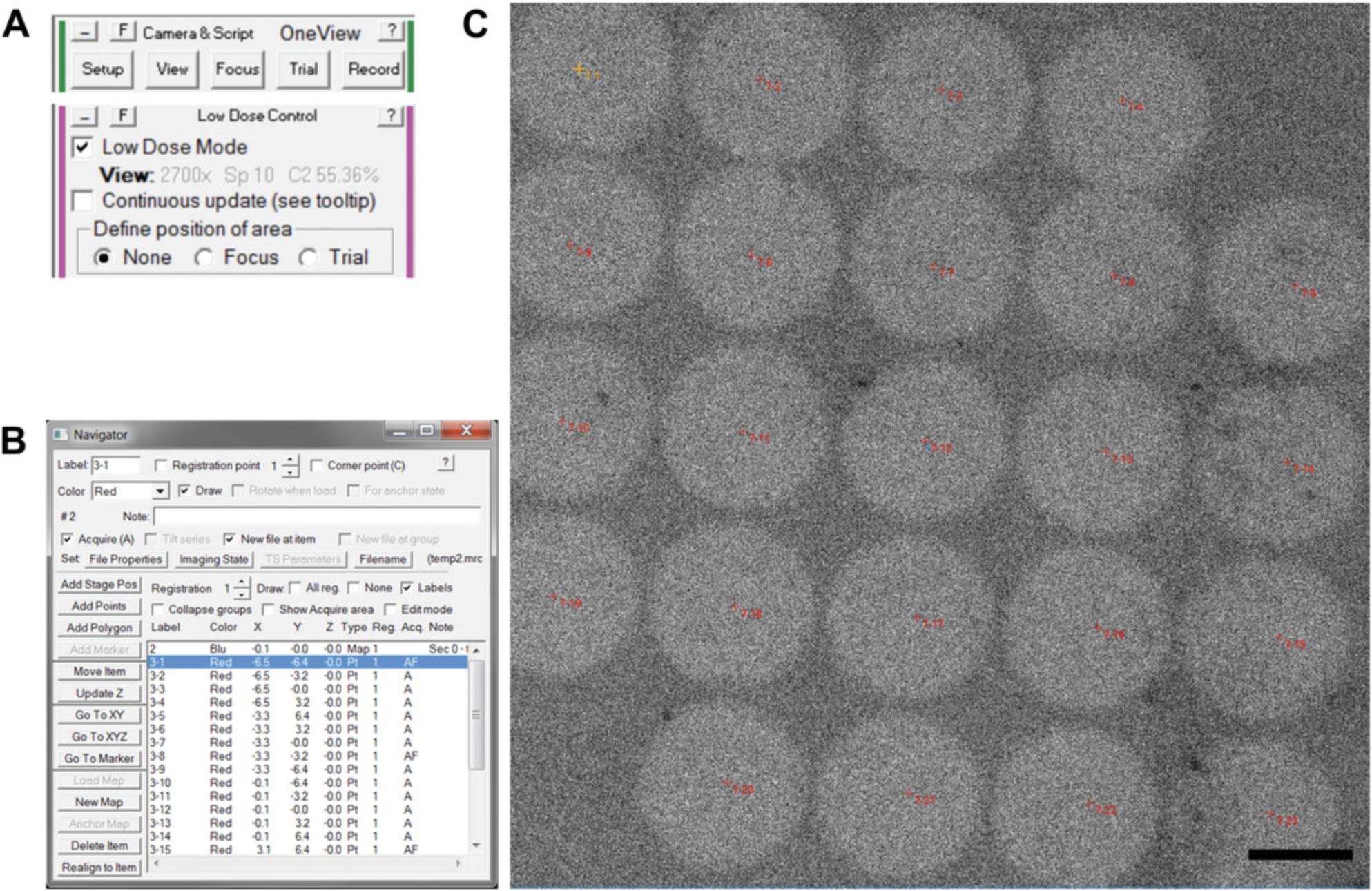
20.Enable SerialEM low-dose mode (Fig. 2A).
21.Acquire a “Record” image with SerialEM in low-dose mode, performed as in step 19.
22.Confirm the beam is centered on the camera, and beam intensity is correct by observing the beam on the phosphor screen at the “Record” settings.
23.Acquire a “View” image (“Camera and Script Controls” Panel → “View”) with SerialEM to acquire a lower magnification micrograph (Fig. 2A).
Acquire the data
24.Initiate a new montage in SerialEM by selecting “File” menu →”New Montage…”.
25.Acquire the montage: “Montage Controls” Panel → “Start” button.
26.Open the navigator window: “Navigator” menu → “Open”.
27.Load the montage into the navigator: select “New Map” in the navigator window (Fig. 2B).
28.Add locations for acquisition of “Record” images: “Navigator” menu → “Montaging & Grids” → “Add Grid of Points.”
29.When prompted by the “Add Grid of Points” menu, enter the point spacing in micrometer, which is appropriate for your magnification and beam settings. This can be determined empirically, as shown in Fig. 2C.
30.When prompted by the “Add Grid of Points” menu, enable acquisition for all points.
31.Set acquisition file names. In the navigator window, select the first point to be acquired, then check the box: “New file at item.”
32.Start the acquisition: “Navigator” Menu → Acquire at points” → "Just acquire and save image."
Repeat data acquisition on a fresh area of the grid
33.Pan the stage to a fresh location on the grid by acquiring a view image, then right-clicking and dragging the image in SerialEM to pan the stage.
34.Collect more data repeating from step 25.
35.Collect as much data as possible during a fixed time interval, such as 30 min or 1 hr.
Finish the EM session
36.Close the EM column valves, retract the camera, remove the grid holder and store the EM grid, then replace the grid holder in the microscope. If the microscope is done for the day, remove the cold finger liquid nitrogen Dewar and cryo-cycle the microscope.
37.Transfer images from the data acquisition computer to a location where data will be processed.
Support Protocol: NEGATIVE-STAIN SAMPLE PREPARATION
Preparation of negatively stained sample is both an art and a science, and it has been described well elsewhere (Booth, Avila-Sakar, & Cheng, 2011; Burghardt & Droleskey, 2006). A carbon-coating machine can be used to apply a carbon coat to a thin layer of plastic on the copper grids or pre-made carbon coated grids can be purchased as done in this protocol. Many approaches exist to apply sample and negative stain to an EM grid (Kastner et al., 2008; Scarff et al., 2018), but here we highlight a single method that has proved effective the majority of the time for the authors. The quality of the negatively stained EM grid determines the difficulty of the data analysis. Often, increased effort and computational resources during data analysis cannot compensate for a poorly stained EM grid. Thus, it is often desirable to screen several negatively stained grids before acquisition of a dataset, and when revisiting a sample, to collect data only on grids that offer an improvement to previous data collections.
Sample purity can be paramount to the success of a project. Some generalities about producing a pure sample and avoiding aggregation are described in Critical Parameters.
Materials
-
Distilled water (dH2O)
-
0.5% EM-grade UA, diluted in dH2O from a 2% stock (see recipe)
-
15-30 µg/ml sample, 5 µl per EM grid, “pure,” monodisperse
-
Formvar/carbon (F/C) 300 mesh grids (e.g., Ted Pella, cat. no. 01753-F)
-
Glass microscope slide
-
Fine-tipped self-closing tweezers (e.g., Electron Microscopy Sciences, cat. no. 0304-N1-PO)
-
EM grid glow discharger (e.g., PELCO easiGlow model 91000, Ted Pella)
-
Parafilm
-
Improvised cover for the EM grid and solutions: e.g., petri dish lid or plastic container for 96-well plate
-
Blot paper (e.g., Whatman grade 1 qualitative filter paper, standard grade, 90 mm circle)
-
EM grid storage box (e.g., Electron Microscopy Sciences, cat. no. 71150 or use empty boxes from purchased grids)
Glow discharge the EM grid
1.Select one formvar/carbon EM grid and place on the glass slide with the carbon side up (dark side of the grid up) using the fine tweezers.
2.Place the glass slide (containing the EM grid) in the PELCO glow discharger.
3.Place the glass bell over the sample pedestal.
4.Run the PELCO easiGlow glow discharge program.
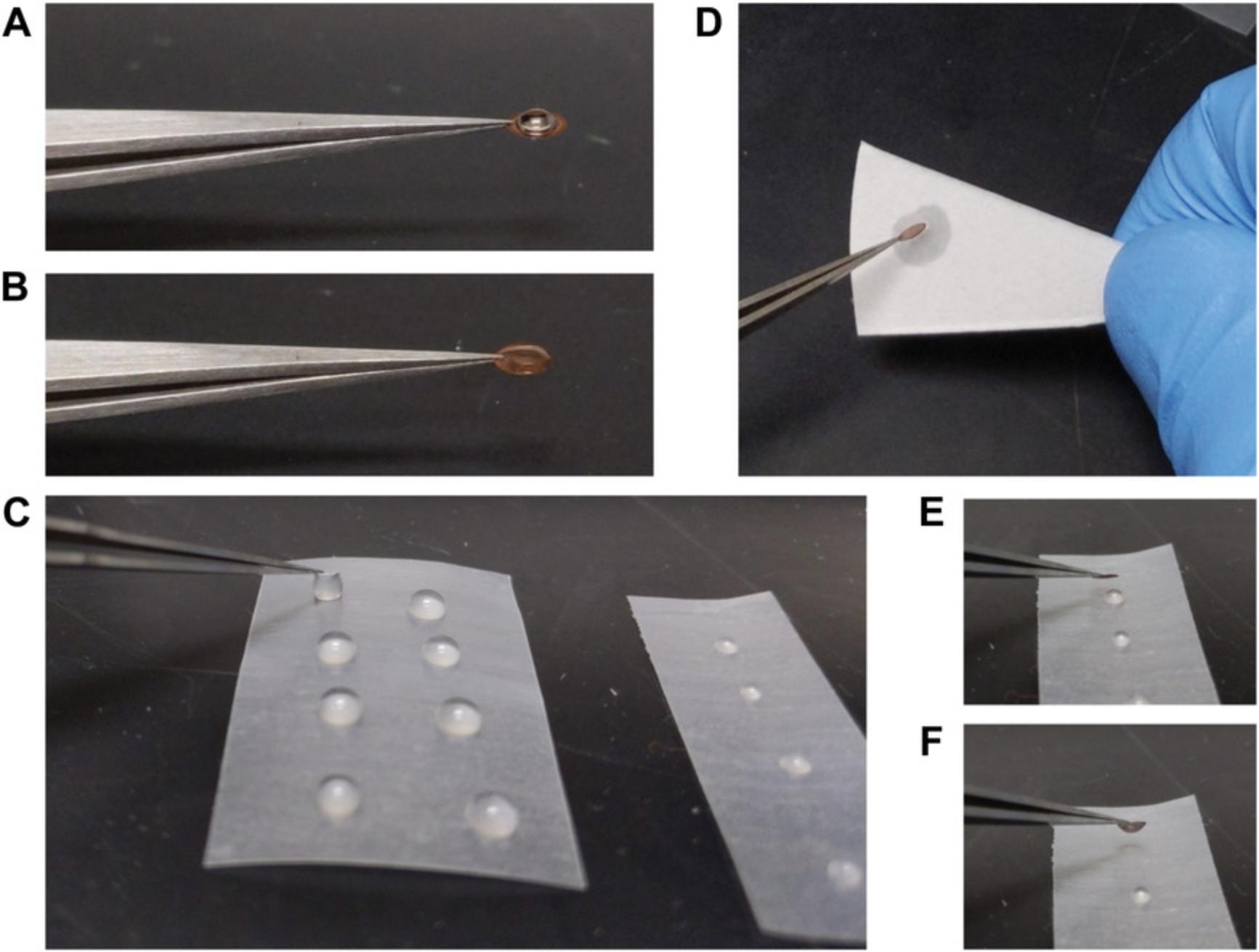
5.Pick up the EM grid with the self-closing tweezers and place the tweezers with the grid on the laboratory bench.
Prepare EM grid rinsing and staining solutions
6.Cut Parafilm into a square or rectangle and place on a working surface, such as the laboratory bench (Fig. 3C).
7.For each EM grid: place two droplets of dH2O, approximately 30 µl each, and place one droplet of 0.5% UA negative stain, approximately 8 µl each.
8.Apply 5 µl sample to the carbon side (dark side) of the grid (Fig. 3B), and then cover the grid.
9.Allow sample to settle on the EM grid for 1 min.
Stain the EM grid
10.Pick up the tweezers with the EM grid in one hand and blot paper in the other hand.
11.Blot the sample from the grid by touching the grid to the blot paper briefly, approximately 0.5 s.
12.Immediately touch the sample side of the grid to the first dH2O droplet to initiate the first rinse (Fig. 3C).
13.Blot the first rinse from the grid by touching it to a fresh location on the blot paper (Fig. 3D).
14.Immediately touch the sample side of the grid to the second dH2O droplet for the second rinse.
15.Blot the second rinse from the grid by touching it to a fresh location on the blot paper.
16.Immediately touch the sample side of the grid to the UA droplet (Fig. 3E and F).
17.Wait 1 min: allow staining of the sample by UA.
18.Blot the excess negative stain from the grid by gently touching/sliding the side of the grid along fresh blotting paper for 0.5-1 s.
19.Initiate drying of the grid by gently waving the grid in the air, using the tweezers, for up to 5 s to promote initial drying.
20.Set down the tweezers with the EM grid on the working surface and wait 10 or more minutes for the EM grid to completely dry.
Basic Protocol 2: DATA ANALYSIS
While negative-stain EM of molecular complexes is a classic technique, substantial improvements in data analysis, recently driven by single-particle cryo-EM, can be leveraged to arrive at conclusions about molecular structure from large datasets that are far superior to conclusions drawn from single micrographs. By employing a contrast transfer function (CTF) correction to correct for varying micrograph defocuses, destructive interference during particle averaging can be minimized. By averaging across thousands of particles, less-common views and conformations can still be well defined. If multiple conformations are present, the relative proportions of these conformations can be estimated by the population of particles assigned to each of the different class averages. A foundational description of the interpretation of negative-stain EM micrographs and the impact of the CTF has been well described previously (Belnap, 2015). This protocol focuses on RELION3 with assistance from other software such as EMAN2, yet we note that EMAN2 is itself a full package that itself could be used to complete all the steps described here, and as noted in the background section of this article, many other packages exist as well. Many software packages are freely available and provide example data sets and online tutorials.
Much or all of the analysis can be done with the graphical user interface (GUI) of these software packages, but the command line interface at the Linux (or macOS) command terminal must be utilized to explore the full set of runtime parameters. In addition to the GUI buttons, we describe the text-based terminal commands, some of which are provided in the appendix, with the goal of familiarizing a user with commands they may later elaborate on. A general familiarity with the Linux command line (see Internet Resources) facilitates organization of data between analysis steps, as well as troubleshooting of data analysis programs.
One such Linux command line tool is “xargs.” While usages can be complicated, we use it in a single methodology, which is more straightforward. Given a command that creates a list of words, such as “ls”, which creates a listing of the files in the current directory, then this list of files can be “piped” into xargs with the Linux syntax of a vertical bar, “|“. The command xargs then runs a given command, provided as an xargs argument, on all the text piped into xargs. For example, “ls * | xargs -I{} cp {} {}.back” will first create a list of files with the list command, “ls”. The argument to “ls” is the wildcard, “*”, which matches all file names. The resulting filename list from “ls” is passed to “xargs” with the pipe command (vertical bar). Then “xargs” will use those filenames to substitute into the “{}” characters in the copy command, “cp”, and execute it. The result is each of those files being copied to a new file of the same name, but with “.back” appended to the destination filename. In this protocol, we use xargs to unstack all the MRC image stacks in a directory or make JPG thumbnails of all MRC files in a directory. It is essential to be capable of managing data as a batch rather than manually on a per-file basis when working with larger datasets, but batch operations open the possibility of errors going unnoticed. An important follow-up to each command issued in a batch format is to always spot-check a few results. This is to ensure the desired action indeed occurred.
Terminal commands are listed as bold annotations under their respective steps. Commands spanning more than one line will be broken across lines as could be done at the Linux command line using the “\“ character.
Materials
- Digital micrographs of negatively stained complexes (see Basic Protocol 1)
- Linux system—(e.g., CentOS or Ubuntu): either remote or local access to a Linux operating system (macOS may also be used, but may require additional effort to compile software)
- Data storage of 50-200 GB
- RELION3 software (e.g., RELION 3.0)
- CTFFIND4 software (e.g., CTFFIND 4.1.10)
- EMAN2 software (e.g., EMAN 2.12, although newer versions are likely a great choice)
- FIJI software (e.g., based on ImageJ 1.52i, but continually updated)
- 3-button mouse, or capability to emulate a 3-button mouse
Review raw micrographs
1.Within a new (empty) project directory, make a “relion” directory, and a “data” directory. Copy the set of micrographs into the “data” directory.
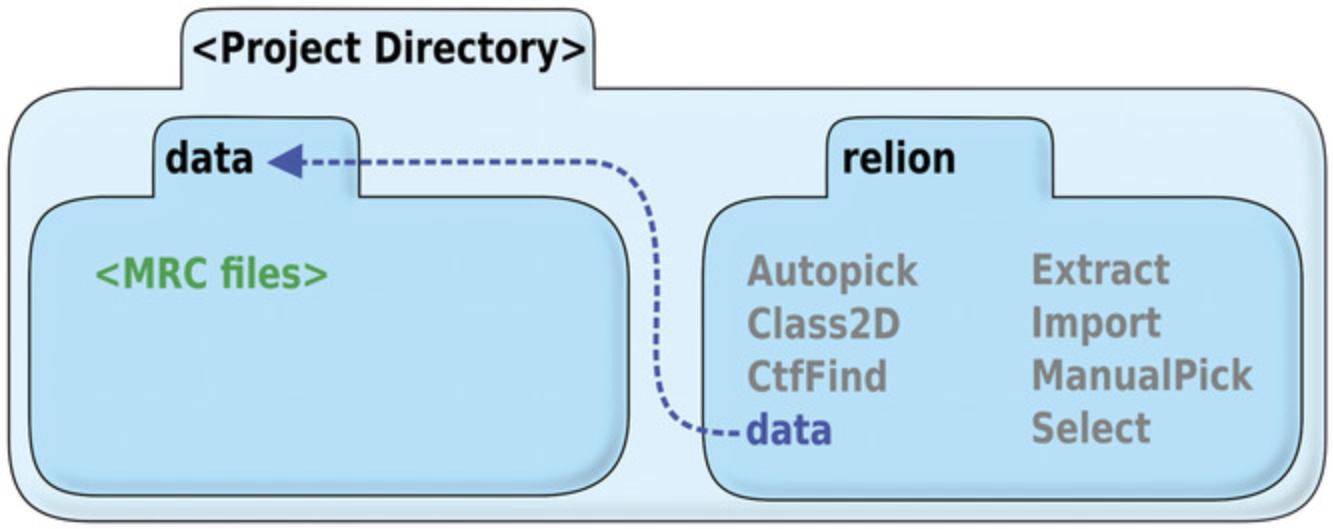
2.Create a symbolic link from the “data” directory above the “relion” directory to inside the “relion” directory (Fig. 4).
- cd relion
- ln -s ../data ./data
3.If the micrographs exist as an MRC stack containing multiple micrographs per file, convert them to a single micrograph per file using EMAN2 as follows:
- *ls .mrc | xargs -I{} e2proc2d.py --threed2twod {} {}-split.mrc –unstacking
4.Create a full set of JPG thumbnails using EMAN2, since it is commonly more practical to review a large set of JPGs than a large set of raw micrographs in MRC format as follows:
- **find . -maxdepth 1 -name ".mrc" | xargs -I{} e2proc2d.py --process normalize *
- --scale 0.25 --clip 1024,1024 {} {}_THUMB.jpg
- *mkdir jpg; mv .jpg ./jpg/
5.Review the JPG files in their respective subdirectory, and discard or set aside corresponding raw micrographs that are excessively out of focus or exhibit negative staining artifacts.
RELION micrograph processing
6.Use the Linux command terminal to navigate into the “relion” directory, and start the RELION GUI:
- relion
- relion --idle 86400
7.Import data into RELION. Select “Import” in the GUI, and the input files can be specified as “data/.mrc”. Choose “Node type: 2D micrographs (.mrc)”. (See Appendix for corresponding RELION terminal commands.)
8.Run CTFFIND4 to fit defocus values. Select “CTF estimation” through the RELION GUI. Set the spherical aberration, voltage, and micrograph pixel size. Select “Yes” for “Use CTFFIND-4.1.” Default values are otherwise likely good enough to begin. (see Appendix)
- cd Import/job001/
- cp -i micrographs.star micrographs-test.star
- gedit micrographs-test.star
9.Review the CTF fitting results by checking for correspondence of actual and predicted Thon rings (Fig. 5A). Either (1) start “Manual picking” through the GUI using the same “micrographs.star” file, then select the “CTF” button for each micrograph to be examined, or (2) convert the CTFFIND output files to JPGs and review them directly:
- cd CtfFind/job002/data
- *ls .ctf | xargs -I{} e2proc2d.py {} {}.jpg
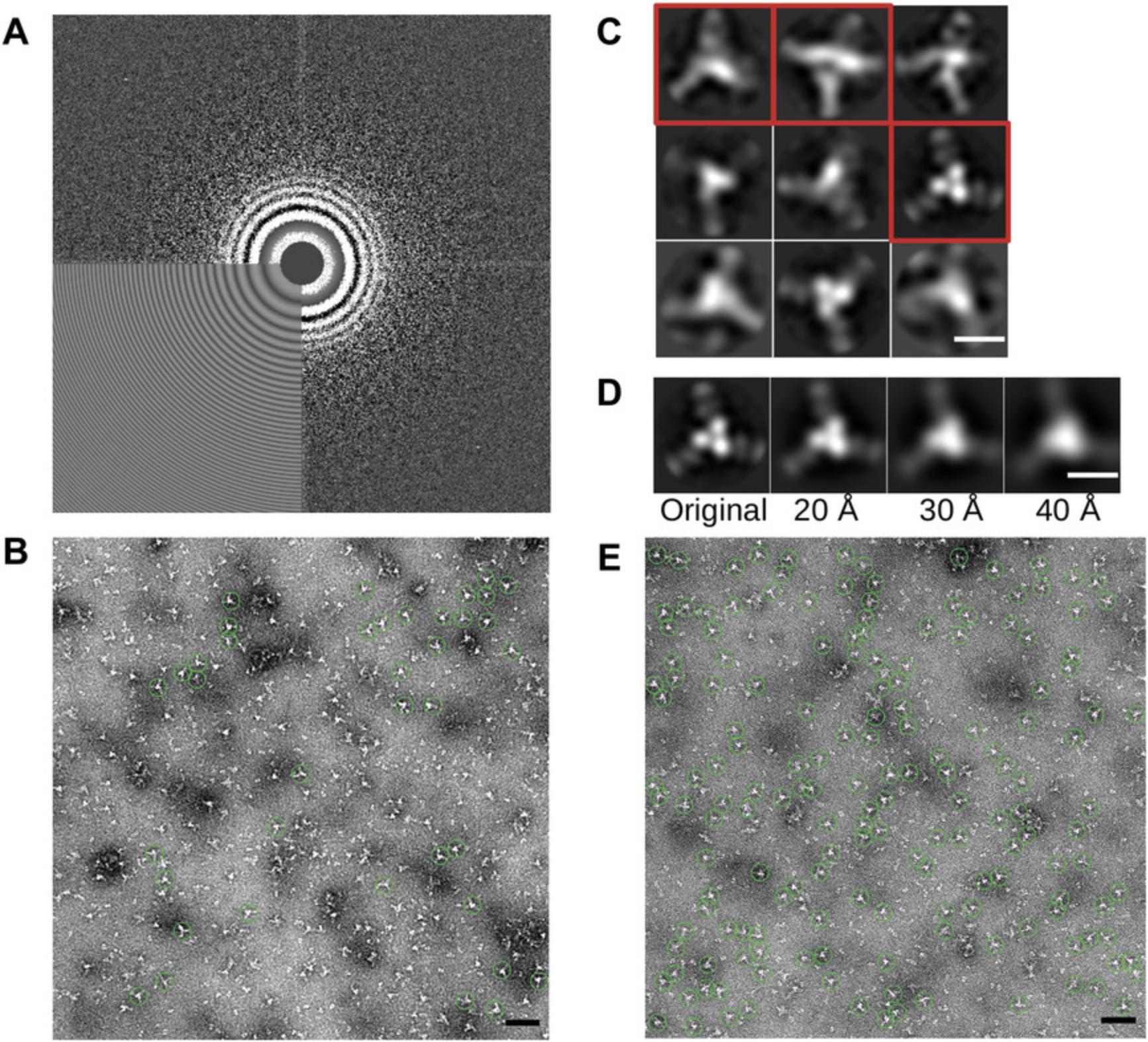
RELION particle processing
10.Manually pick 500 to 1000 particles (Fig. 5B). Select “Manual picking” in the GUI. Choose the input file as the CTFFIND result, micrographs_ctf.star. Input the particle diameter. Default values are otherwise a reasonable start. (see Appendix)
11.Extract particles. Select “Particle extraction” in the GUI. Choose the “micrographs_ctf.star” from the CTFFIND result, and the particle coordinates picked from manual picking. Choose a box size (in pixels) that is 1.5-2× the diameter of the particle. Choose “No” for “Invert Contrast.” Otherwise, default values are reasonable to start. (see Appendix)
12.Run 2D classification to generate auto-picking templates (Fig. 5C). Select “2D Classification” in the GUI. Choose the “particles.star” produced from particle extraction. Set “Number of Classes” to between 4 and 10.Select a mask diameter in angstroms that is about half-way between the actual particle diameter, and the particle box size. Increase the number of threads to a reasonable number for the CPUs at your disposal. Default values are otherwise a reasonable start. (see Appendix)
13.If only a subset of classes from 2D classification will be used for auto-picking, such as when excluding a “junk” class, the subset needs to be generated explicitly. Select “Subset selection” and select a model.star file from the final iteration of the 2D classification. Use the RELION GUI to select the classes to keep (Fig. 5C). To save the particle selection, right click on the images and select “Save selected classes.” A “class_averages.star” file will be written that can be used as a reference for auto-picking.
14.Auto-pick particles using templates from 2D classification. Select “Auto-picking” from the GUI. Choose the micrographs_ctf.star from CTFFIND. Select the 2D references as the mrcs file from the conclusion of 2D classification, or the “class_averages.star” from subset selection. The parameters “Picking threshold,” “Minimum inter-particle distance,” and “Maximum stddev noise” will need to be manually optimized. Otherwise, default values are reasonable to start. (see Appendix)
15.Confirm auto-picked particles are accurate by clicking on the auto-pick output via the RELION3 GUI. With the auto-picking job selected, select the output field indicated by “Display: out: coords_suffix_autopick.star.” The particle picking GUI will spawn, and the picked particles will be those resulting from auto-picking (Fig. 5E).
16.Extract auto-picked particles. Proceed as in step 11 in this protocol, except select the particle coordinates from auto-picking.
17.Run 2D classification. Proceed as in step 12 in this protocol, except select the “particles.star” from the particle extraction from auto-picking. Set “Number of Classes” to be between 8 and 50 (Fig. 6A).
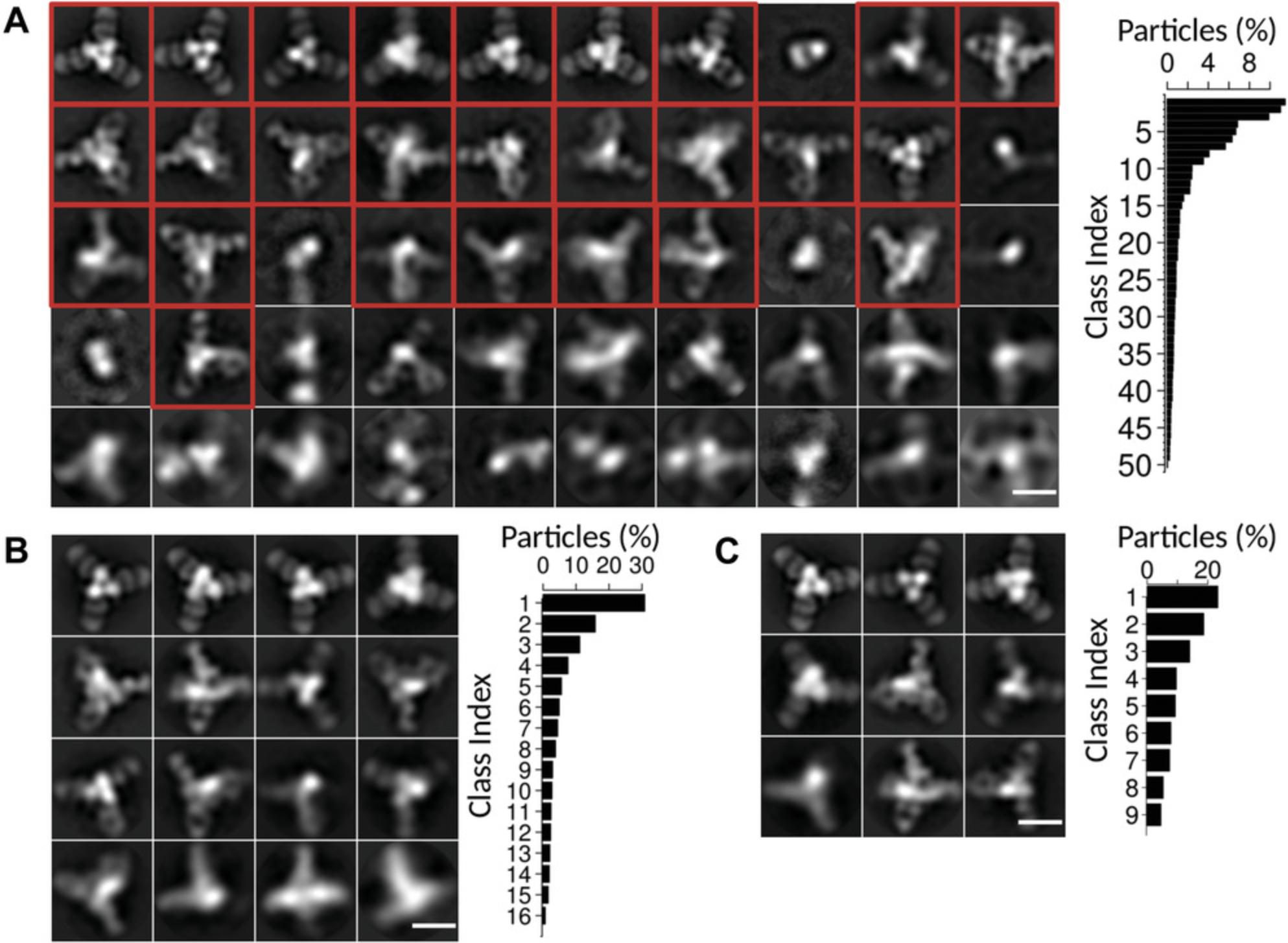
18.Cull the particles in poorly resolved classes from the particle set. Similar to step 13, select “Subset selection” and select a model.star file from the final iteration of the 2D classification, select the classes to keep, and then save the particle selection. Differing from step 13, the output that will be used for future 2D classification is the “particles.star” file.
19.Multiple rounds of 2D classification and subset selection can be repeated until the particle set consists of well resolved classes, and the classes are representative of the particles in the sample as best as possible.
20.Perform a final 2D classification. The output becomes the final result for this sample (Fig. 6B and C).
REAGENTS AND SOLUTIONS
Phosphotungstic acid (PTA)
- Prepare 3% PTA stock can be made by dissolving PTA (e.g., Electron Microscopy Sciences, cat. no. 19500) in water
- Titrate the pH to 7 using sodium hydroxide
- Store up to 1 year at room temperature
Uranyl acetate (UA)
- Prepare 2% UA stock by dissolving UA (e.g., Electron Microscopy Sciences, cat. no. 22400) in water
- Filter the solution with a 0.22-µm syringe filter (or centrifuge and retain the supernatant)
- Store in an aluminum foil wrapped container up to 1 year at 4°C (UA is light sensitive)
COMMENTARY
Background Information
Negative-stain electron microscopy (EM) has been widely used in identification and evaluation of virus particles (Gelderblom, Renz, & Ozel, 1991; Horne & Wildy, 1979). To improve resolution of biological complexes that were even smaller than viruses, computational averaging of images was first performed on negatively stained glutamine synthetase (Frank, Goldfarb, Eisenberg, & Baker, 1978). 2D classification of particles into distinct sets was first performed on negative-stain micrographs of hemocyanin (van Heel & Frank, 1981), and facilitated statistical analysis of particle conformations. Automation of EM data collection initiated with tomography, where multiple micrographs of a tilt series needed to be acquired precisely to enable reconstruction of the 3D volume (Dierksen, Typke, Hegerl, & Baumeister, 1993; Dierksen, Typke, Hegerl, Koster, & Baumeister, 1992; Mastronarde, 2005). Development for acquisition of single particle data as described in this article, but with full automation, required integrated effort managing multiple magnifications, stage control, focus, and machine vision algorithms (Suloway et al., 2005).
Image processing advances have been driven by the massive amounts of cryo-EM data generated by automated image acquisition by tools such as EPU (FEI, Eindhoven, The Netherlands), or Leginon (Suloway et al., 2005). A variety of software are commonly employed by each lab working in cryo-EM image analysis, but major packages include RELION (Kimanius, Forsberg, Scheres, & Lindahl, 2016), EMAN2 (Tang et al., 2007), BSOFT (Heymann, 2018), and FREALIGN (Lyumkis, Brilot, Theobald, & Grigorieff, 2013). New software suites are coming into use as well, such as cryoSPARC (Punjani, Rubinstein, Fleet, & Brubaker, 2017). These major packages are commonly supplemented by smaller software with very specific purposes, such as CTFFIND4 (Rohou & Grigorieff, 2015) for CTF fitting, and GAUTOMATCH (developed by Kai Zhang, MRC Laboratory of Molecular Biology, Cambridge, UK) for GPU accelerated particle picking. A data-processing pipeline for cryo-EM may utilize substantial computational resources and require hundreds of computer cores. Both the data acquisition and data processing steps of cryo-EM analysis are resource intensive for personnel, and for infrastructure. Because of the increased signal-to-noise of negative-stain micrographs, the requirements for data collection and data analysis are much less stringent, all the while offering a substantial fraction of the information available from an atomic resolution cryo-EM reconstruction.
Critical Parameters
Negative-stain EM grid preparation
Pure sample greatly facilitates 2D image classification. Obtaining pure and monodisperse sample for negative-stain sample preparation can be difficult and may require developing a customized protocol. Nonetheless, the following observations may guide the process. Protein samples can be synthesized in many different expression systems, but generally all will require purification of the target protein away from other components (Wingfield, 2015). Contaminating proteins not only confound analysis by outnumbering the protein of interest, contaminants can imperil the target protein's integrity via the presence of trace proteases. At least two purification steps are typically required to attain high purity. Inclusion of a third purification step may lead to ideal purity, but also may be the point of diminishing returns on time manipulating the sample. Categorically, those steps are an initial affinity purification step, followed by an ion exchange purification step, and a final gel filtration step. This combination of selections by an expression tag, the neutral point of the protein, and the molecular size often leads to either pure protein or a clear understanding when aggregation prevents further purification. Nucleic acids and other samples also benefit directly from purification methods such as gel purification.
Pure samples may still suffer from aggregation. A monodisperse sample is important for image analysis since particle overlap confounds the shape recognition that is essential for particle alignment and averaging. EM is tolerant to detergent, and addition of detergents such as n -dodecyl-β-D-maltoside (DDM) and lauryldimethylamine oxide at concentrations below the critical micelle concentration may increase sample dispersion on the EM grid. These detergents may also be employed in cryo-EM, so knowledge gained from negative-stain analysis may be applied directly to ensuing atomic-resolution cryo-EM investigations.
When studying a complex of multiple components, such as our example of influenza hemagglutinin in complex with Fab 441D6, it is preferred that the complex can be purified away from its un-complexed constituents. This is not a strict requirement; however, since the unbound constituents may often be computationally identified and deleted from the analysis. Therefore, it may be most practical to first image the mixture of bound and unbound components of the complex, while also creating a plan to purify the bound complex. The purification plan for the complex may be invoked either when analysis of the mixture indicates it is necessary or as soon as sufficient reagents have been generated to pursue the purified complex in parallel.
Both the stain depth and molecular complex concentration are critical to producing useful images for generating 2D class averages. Stain depth plays a critical role in image contrast to define the features of the molecular complex. Concentration of the complex on the grid has a dramatic effect on the rate particles are collected, and a sparse sample could require an untenable number of micrographs to reach a practical particle count. Meanwhile, an overly dense sample will suffer from neighboring particles confusing the particle alignment during averaging.
Data analysis
Particle box size and radial mask can have large and sometimes surprising effects on generating 2D class averages. Ideally, the box of a picked particle in a micrograph should have no other particles in the same boxed out region. Using either the box size or the radial mask to disregard information at larger radial distances can avoid the particle alignment from being distracted by instances of neighboring particles, which could dominate the alignment. Less intuitively, increasing the box size or radial mask size can sometimes permit the 2D classification to discern more subtle details of the particle, particularly at the outer edges. In cases where a particle has a core which is especially easy to align, such as the round core of a virus capsid or an encapsulating complex such as ferritin, the round core can dominate the alignment at early stages while further structural details at the surface of the particle remain unresolved.
Runtimes can be dramatically affected by the angular sampling of the search space, the number of classes chosen for 2D classification, or the number of templates chosen for auto-picking. RELION output will provide runtime estimates. Monitor these runtimes, and strongly consider killing the job (see Troubleshooting) and restarting it with increasingly coarse search parameters if the runtime will be prohibitively long. Empirically determining the coarseness of sampling required to create class separation for particular dataset will likely recoup any time invested if multiple rounds of subclass selection are performed (see Basic Protocol 2, step 19).
Troubleshooting
Negative-stain contrast is low
When negative-stain depth is too thin, both particles and background appear light, and a subtle dark staining of the particle outline is seen. When negative-stain depth is too thick, the background is uniformly dark, while particles are very light, but the particle edges are poorly resolved. At even thicker stain depths, particles begin to darken and are hard to discern from the dark background, and the entire beam intensity will be reduced. Remaking a grid is the solution to incorrect stain depth, and it may require slight modifications to the standard protocol to consistently obtain stain at a desired stain depth (Booth et al., 2011; Scarff et al., 2018).
Some negative stains may provide better contrast for a particular sample, for example if the stain is bound by a complex with unusual affinity compared to average protein or nucleotide complexes. While UA reliably provides good contrast with most samples, phosphotungstic acid (PTA) is another stain that is widely successful at generating sample contrast. PTA is stable and is used at neutral pH, whereas UA requires acidic pH. Uranyl formate, not discussed here, can offer excellent contrast at neutral pH, but is unstable and must be made fresh each day. To use PTA stain, start from a 3% stock (see Reagents and Solutions), prepare a 1.5% working solution diluted in water. 3% PTA is commonly used to stain samples, but the authors have had excellent results with 1.5% PTA, and thus recommend it. Staining with PTA can be performed by substituting the PTA solution for the UA solution in the Support Protocol, step 16, and by inserting an additional two steps of dH2O rinse following the negative staining at step 18, performed as described by steps 12 to 15.
If no features attributed to the molecular complex of interest are visible on the EM grid, it is useful to have a control sample to confirm functionality of the negative-stain reagents and of the microscope. Protein complexes such as keyhole limpet hemocyanin (KLH) are an excellent positive control. KLH forms a 390 kDa complex that is a hollow cylindrical structure which provides good contrast with negative stain (Fig. 7A). KLH is commercially available (e.g., Sigma-Aldrich, cat. no. 9013-72-3) making it straightforward to obtain, though any large molecular complex less than 100 nm in diameter could be used as an alternative, under the constraints that they are readily available in pure form, and that negative stain of the complex produces good contrast.
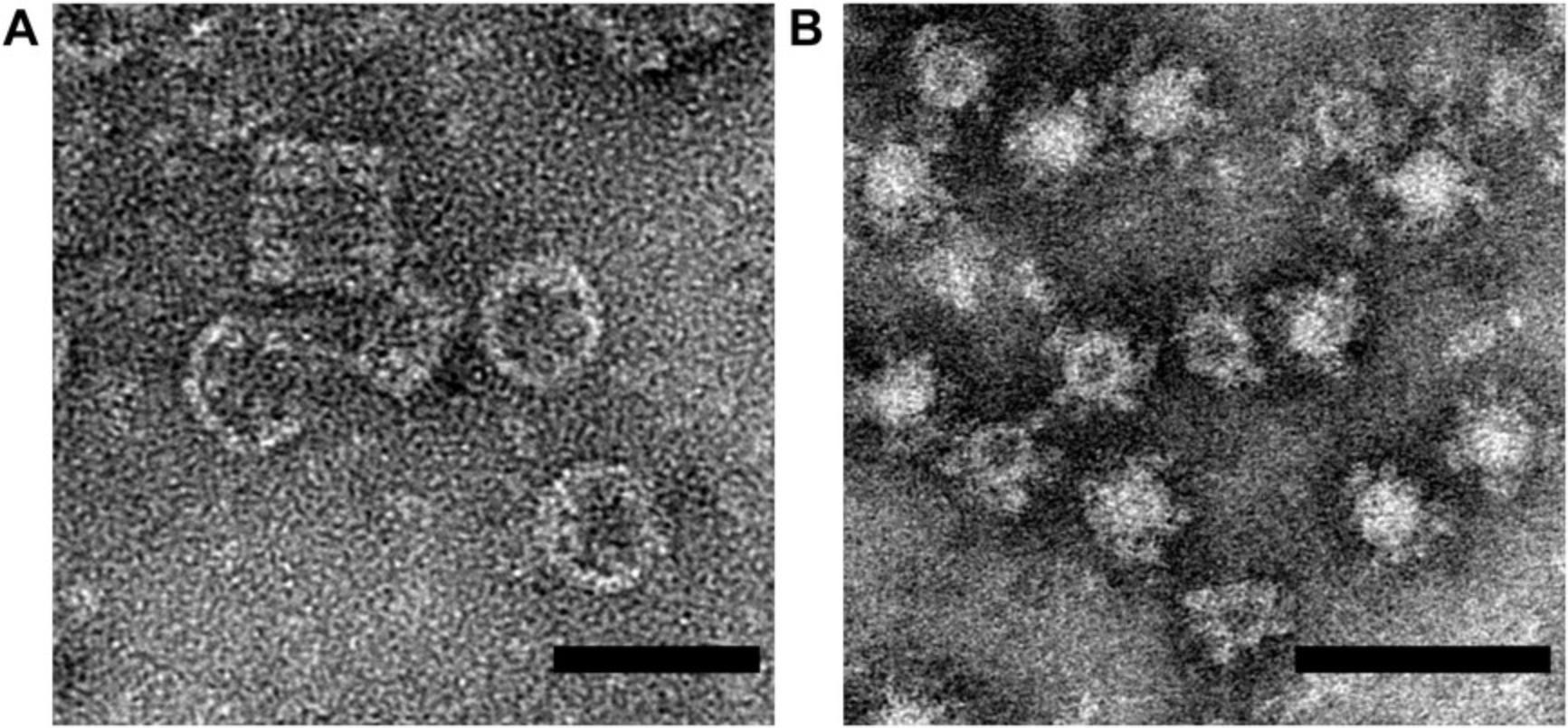
If the sample concentration is wrong by being either too dilute, or too concentrated, then it is possible that no complexes may be discerned in the micrographs. Confirming the sample concentration is important when no complexes are observed by negative-stain EM. Also, confirm sample is being applied to the carbon side of the grid rather than the plastic support side (formvar or collodion). Complexes may not adhere to the plastic, giving the appearance that the sample was excessively dilute.
Salt crystals and other semi-crystalline artifacts are found in the sample
Some buffers can react with the negative stain. An example of one such common pitfall of UA is that it forms precipitate with phosphate. Rinsing of the sample with dH2O while adhered to the EM grid, but before negative staining, commonly avoids the interaction of stain and buffer. Air drying of the grid at any intermediate steps of staining can create strange artifacts. Remaking the EM grid is a good first step in understanding the source of inexplicable sample features. Some lipoparticles are known to bind to PTA and aggregate, presenting artifactual morphology (Zhang et al., 2011). Thus, another negative stain should be used when artifacts such as these are observed.
Acquired images are blurred
Check if stage drift is causing image blurring by acquiring consecutive images of the same location of the grid, as indicated by transposition of the particles in one direction between consecutive images. Drift in one direction also manifests in the Fourier transform of the image (typically available in the camera's image acquisition software, or in SerialEM's “Process” menu). Drift will cause the Thon rings to disappear along one axis of the Thon ring ellipse, indicating loss of resolution on that axis. If stage drift is manifest during automated image acquisition in SerialEM, increase the stage settling delay before image acquisition by setting the SerialEM property “StageMoveDelay” in the properties file (see SerialEM in Internet Resources).
Vibrations from the room or microscope can also cause blurring of the micrograph. Listening or feeling for vibration at the microscope can identify problems. For nanometer resolution, all discernable vibrations of the microscope are bad.
RELION programs are not running properly
RELION is available on some high-performance computer clusters. However, it can be more complicated to troubleshoot a RELION job submitted to a cluster than running RELION interactively, or locally. Consider building a separate RELION directory tree on a local computer solely for RELION testing, i.e., using just two micrographs. Use this testing RELION directory to generate skeletal input files, such as by picking only 10 particles. This directory can be used to confirm that RELION runs successfully in the skeletal setup, and that the command syntax is correct. Differences between the production run of particles and the testing RELION directory tree can be investigated until the expected behavior occurs on the production run. The most useful information in debugging a RELION run is often available in the STDOUT and STDERR terminal output. When run through the GUI, STDOUT and STDERR are saved as “run.out” and “run.err” files in the job directory. Direct inspection of the text form of the RELION input star files and the output star files can also help resolve problems such as a star file missing an entire field (column) of data.
Information on RELION program arguments can be found either at the terminal by running the program with no arguments (i.e., “relion_refine”) and observing the help output, or by clicking on the “?” in the GUI for the box that corresponds to that argument. Information from these two places can be complementary, so both should be checked for relevant information.
Currently running RELION jobs are not shown in the GUI
The RELION GUI can become disconnected from, or unaware of the number of actual RELION processes running. Use Linux system commands to interrogate which RELION processes are running, and then kill any undesired RELION processes using its system process id (PID):
ps -u $USER kill -9
RELION 2D classification is not generating distinct classes
There are some circumstances where RELION converges all of the particles into a few, or even just a single 2D class, while other classes are black and contain no particles. Instead of working from an unconverged iteration earlier in the 2D classification, use the following additional arguments to 2D classification that affect the probabilities of particles being considered as the same class.
--sigma_psi 0.001 --fix_sigma_noise --fix_sigma_offset
Some particle sets are not well separated during 2D classification, but they can be separated by using search templates. At the manual picking and auto-picking step, particle classes can be separately picked to create distinct “particles.star” files that can be independently 2D classified. Instances where negative stain penetrates some particles, but not others, can create large differences in particle appearance that may not be of biological relevance, as depicted by ferritin-based nanoparticles in Fig. 7B. Particles with dark centers (penetrated by negative stain) and those with light centers (not penetrated by negative stain) can be processed independently in these cases (Kanekiyo et al., 2019). The RELION “Particle Sorting” job type also offers the ability to sort out subsets of particles subsequent to particle auto-picking.
Understanding Results
2D class averages represent the average of the constituent particles. Perhaps counterintuitively, instead of representing the most commonly observed structure within a class, the class average represents all structures that comprise it. Therefore, the final image for a class average may actually be a physically impossible conformation. A single class average can be composed of different conformations which may be vastly different, and they have been grouped by virtue of being more similar to each other than to the other particles included in the refinement. Therefore, multiple rounds of 2D classification are desirable. Each round culls false-positive particle picks, overlapping particles, or other artifacts in the region of the picked particle that do not reflect the particle's structure, and can influence the alignment of its image. As the differences between particles across the dataset decreases (i.e., staining artifacts and partially resolved particles are removed), the refinement can increasingly focus on details within the complex of interest. A series of rounds of 2D classifications will show that a highly populated single class of particles can give rise to multiple distinct, well-resolved classes. After curation of picked particles derived from a well-prepared grid, final results from negative-stain 2D analysis can be in excellent agreement with a final map derived cryo-EM (Fig. 8).
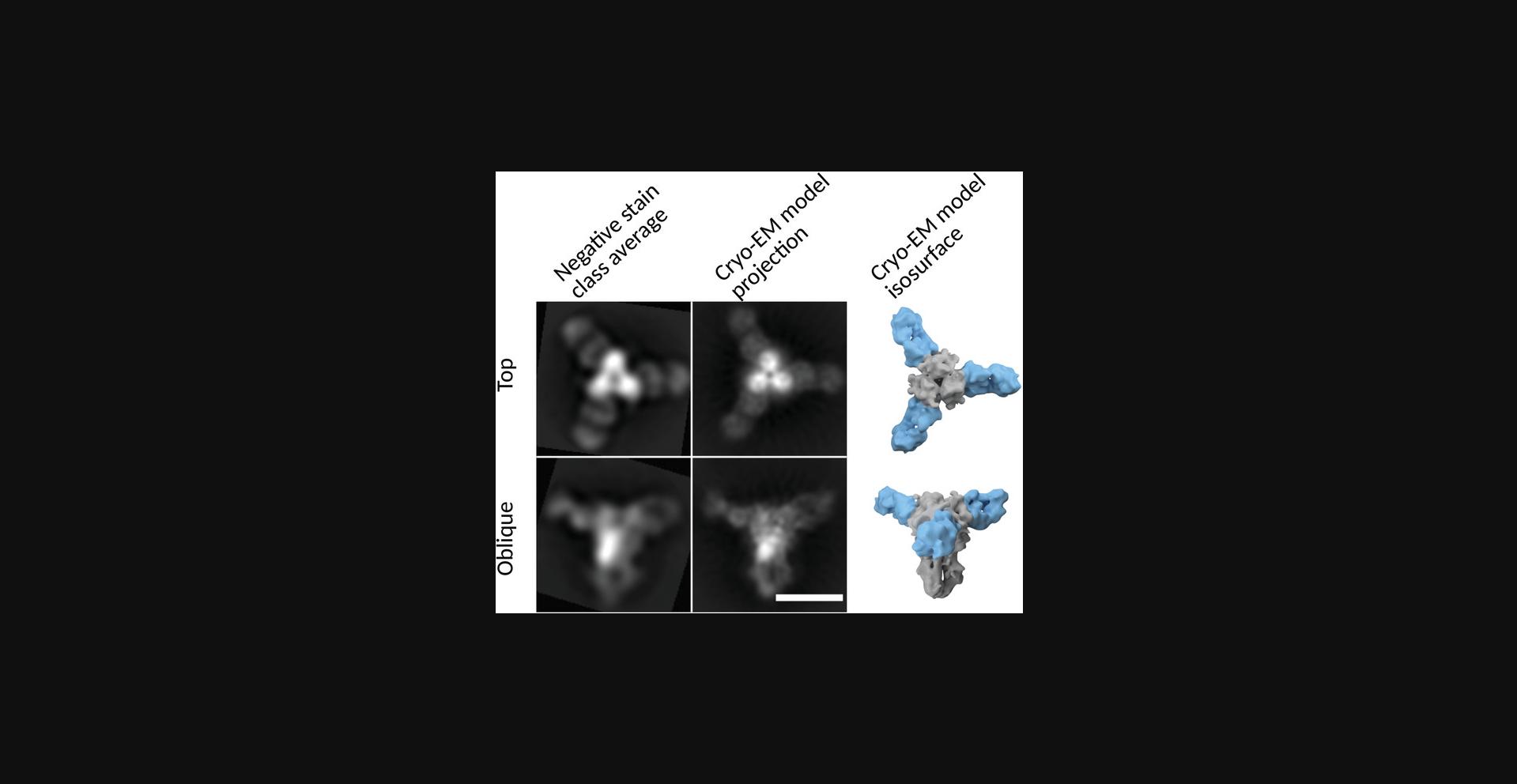
A full 3D reconstruction is possible from negative stain, but there are important considerations before proceeding to 3D, which we merely introduce here. The stain depth may not cover the entire complex, causing the portion of the complex farthest from the carbon surface to be invisible. The carbon surface may impart preferred orientations to the complex, causing some views of the complex to be ill-defined, and resulting in poor resolution on the axes of these views of the complex. The particle may also be compressed towards the carbon surface during drying of the negative-stain sample, distorting a 3D reconstruction.
To illustrate that 3D reconstruction from negative stain can be successful, we used the culled particle set depicted in Figure 6A as input to RELION “3D Classification” with one class, followed by RELION “3D auto-refine” to arrive at a plausible 3D model of the HA-441D6 complex (Fig. 9A and B). Atomic coordinates were available for the Fab and HA molecules, and these could be readily docked into the negative-stain 3D reconstruction of the complex to depict the Fab epitope. To enumerate how to begin to assess a 3D reconstruction, two simple criteria are helpful. (1) Direct of inspection of the map in molecular visualization software such as Chimera (Pettersen et al., 2004) should reveal the map does not appear stretched or compressed along any particular axis or radially from the center, and that the density map is not discontinuous or disjoint when using an appropriate threshold level. (2) The Fourier shell correlation (FSC) plot resulting from a “gold-standard” refinement (Scheres, 2012; Scheres & Chen, 2012), which depicts the agreement of two independently refined sets of data according to resolution shell (i.e., Fourier space), should begin near 1.0 at low resolution, and then smoothly decline to 0 at some higher resolution (Fig. 9C). The exact resolution of the map is indicated by the FSC curve crossing the threshold 0.143 (Rosenthal & Henderson, 2003). Maps meeting these two criteria are on a good start to indicating a robust result.
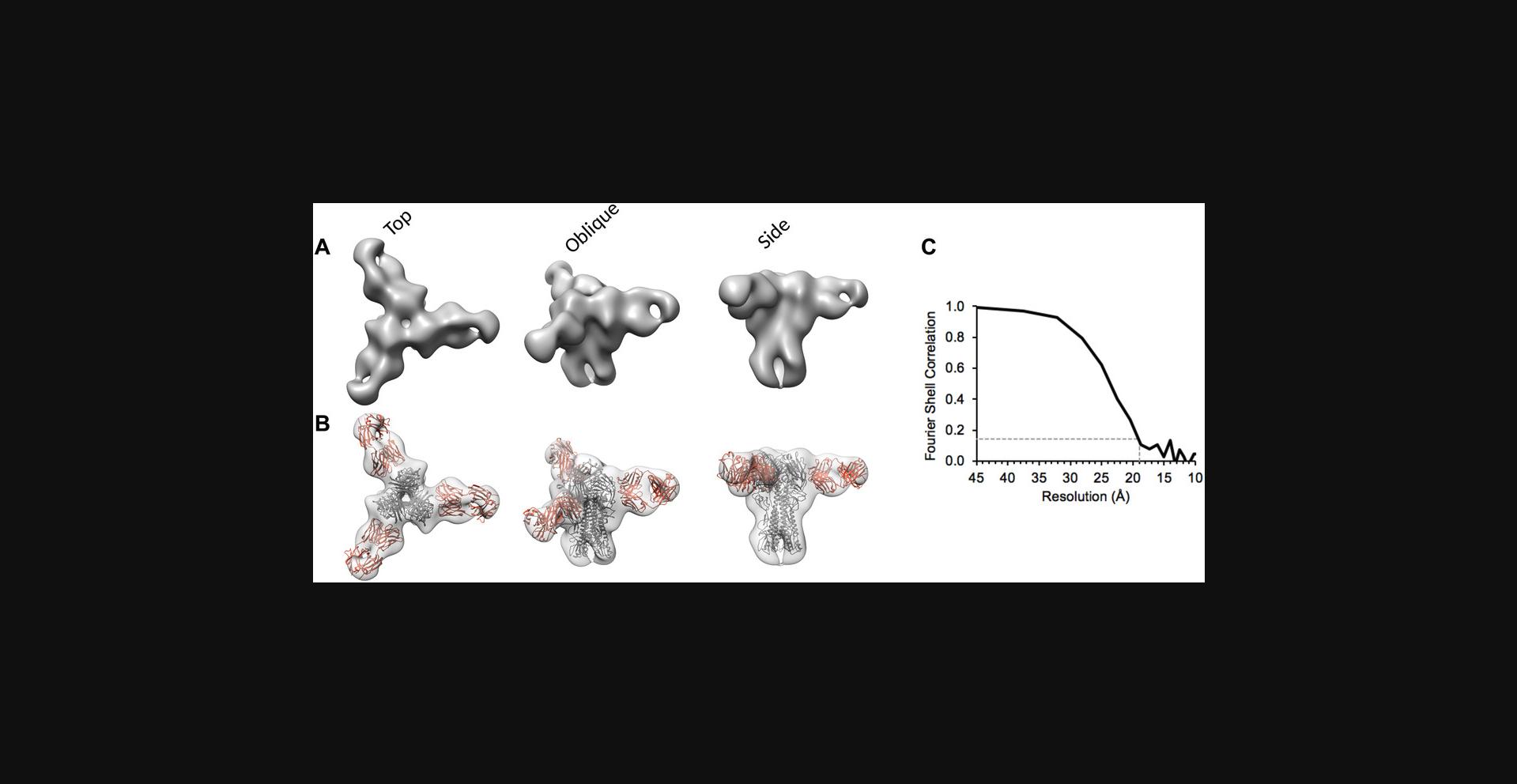
Time Considerations
Data collection
Preparation of negatively stained EM grids can take approximately 30 min, although multiple grids can be made concurrently, as long as one takes care not to mix up the unlabeled grids. Often, multiple iterations of grids will need to be prepared before an appropriate “good” grid is found, but once made, that grid can be stored and imaged as needed.
Operating the EM microscope may encounter unpredictable delays, but here we provide some optimistic times. Chilling the microscope and validating its functionality may take approximately 1 hr. Inserting a sample and setting up operating parameters can take 10 min, then browsing the grid before deciding if a particular EM grid deserves to have a dataset collected may take 10-30 min. Once collecting data, we have averaged just over six micrographs per minute, collecting about 180 micrographs in 30 min. With good particle density on the EM grid and a 4k resolution detector, 30 min of collection is likely to already be enough data (e.g., 180 micrographs with 200 particles per micrographs yields 36,000 particles). Even while it is tempting to simply embark on the additional 30 min of data collection after an hour has already been invested in the grid, the additional data may not be of any utility if the grid quality is not better than previously imaged. Concluding the EM session may take just 10 min, although copying the acquired data to a data analysis system may take significant time. Setting up acquisition parameters for the first dataset in this semi-automated fashion with SerialEM may be a slow process, taking between a day and a week. Once a protocol is established that is optimized for the available equipment, it is not unreasonable to collect good data on four different samples in a single afternoon.
Data analysis
Manual particle picking may be as short as 20 to 60 min if one is confident the chosen particles are representative, and then auto-picking is relied upon to bootstrap picking of a full dataset. CTFFIND runtimes may be fast or slow depending on the chosen parameters and available computational power but may take several hours. Auto-picking via template matching in RELION3 can have runtimes of several hours. Thus, it is advisable to develop and test parameters on just one, or only a few micrographs, and ensure the results are as desired before queuing the processing of the entire dataset. Alternative auto-picking programs such as Gautomatch (GPU based) are available, and these can process the whole dataset in minutes to an hour once the correct picking parameters are discovered. It is worthwhile to spend extra time tuning the auto-picking parameters to avoid problems induced by overabundant false-positive particles during 2D classification. RELION3 2D class averaging will run quickly on the manually picked dataset, but it can be slow for a full dataset of auto-picked particles. For production 2D classification runs on a full particle set, the availability of a computer cluster will greatly improve refinement runtimes. RELION3 scales well and can commonly be run on several hundred CPU cores via MPI. 2D classification can still be performed on a desktop workstation computer, but the search parameters will require optimization, and the dataset may need to be processed in subsets. If extending the analysis to 3D, runtimes on a desktop workstation may be excessively long, thus access to a compute cluster becomes more desirable. The full gamut of negative-stain data analysis, up to definitive 2D classification, may be completed in a week. The manual effort of setting up processing parameters, organizing files and directories, and curating the data is not negligible, which becomes more apparent when compute cluster resources are available and compute job runtimes are shorter.
Acknowledgment
This work was supported by the Intramural Research Program of the National Institute of Allergy and Infectious Diseases, National Institutes of Health, and the Biomedical Advanced Research and Development Authority.
Literature Cited
- Belnap, D. M. (2015). Electron microscopy and image processing: Essential tools for structural analysis of macromolecules. Current Protocols in Protein Science , 82, 17.2.1−17.2.61. doi: 10.1002/0471140864.ps1702s82.
- Bianchi, M., Turner, H. L., Nogal, B., Cottrell, C. A., Oyen, D., Pauthner, M., … Hangartner, L. (2018). Electron-microscopy-based epitope mapping defines specificities of polyclonal antibodies elicited during HIV-1 BG505 envelope trimer immunization. Immunity , 49(2), 288–300.e8. doi: 10.1016/j.immuni.2018.07.009.
- Booth, D. S., Avila-Sakar, A., & Cheng, Y. (2011). Visualizing proteins and macromolecular complexes by negative stain EM: From grid preparation to image acquisition. Journal of Visualized Experiments: JoVE , (58), pii: 3227. doi: 10.3791/3227.
- Burghardt, R. C., & Droleskey, R. (2006). Transmission electron microscopy. Current Protocols in Microbiology , 3, 2B.1.1−2B.1.39. doi: 10.1002/9780471729259.mc02b01s03.
- Dierksen, K., Typke, D., Hegerl, R., & Baumeister, W. (1993). Towards automatic electron tomography II. Implementation of autofocus and low-dose procedures. Ultramicroscopy , 49(1), 109–120. doi: 10.1016/0304-3991(93)90217-L.
- Dierksen, K., Typke, D., Hegerl, R., Koster, A. J., & Baumeister, W. (1992). Towards automatic electron tomography. Ultramicroscopy , 40(1), 71–87. doi: 10.1016/0304-3991(92)90235-C.
- Frank, J., Goldfarb, W., Eisenberg, D., & Baker, T. S. (1978). Reconstruction of glutamine synthetase using computer averaging. Ultramicroscopy , 3(3), 283–290. doi: 10.1016/S0304-3991(78)80038-2.
- Gallagher, J. R., & Harris, A. K. (2018). Observed structural heterogeneity of human hepatitis B virus surface antigen particles by cryo-electron microscopy. Microscopy and Microanalysis , 24(Suppl 1), 1246–1247. doi: 10.1017/S1431927618006712.
- Gallagher, J. R., Torian, U., McCraw, D. M., & Harris, A. K. (2017). Structural studies of influenza virus RNPs by electron microscopy indicate molecular contortions within NP supra-structures. Journal of Structural Biology , 197(3), 294–307. doi: 10.1016/j.jsb.2016.12.007.
- Gelderblom, H. R., Renz, H., & Ozel, M. (1991). Negative staining in diagnostic virology. Micron and Microscopica Acta , 22(4), 435–447. doi: 10.1016/0739-6260(91)90061-4.
- Heymann, J. B. (2018). Guidelines for using Bsoft for high resolution reconstruction and validation of biomolecular structures from electron micrographs. Protein Science , 27(1), 159–171. doi: 10.1002/pro.3293.
- Horne, R. W., & Wildy, P. (1979). An historical account of the development and applications of the negative staining technique to the electron microscopy of viruses. Journal of Microscopy , 117(1), 103–122. doi: 10.1111/j.1365-2818.1979.tb00234.x.
- Kanekiyo, M., Joyce, M. G., Gillespie, R. A., Gallagher, J. R., Andrews, S. F., Yassine, H. M., … Graham, B. S. (2019). Mosaic nanoparticle display of diverse influenza virus hemagglutinins elicits broad B cell responses. Nature Immunology , 20(3), 362–372. doi: 10.1038/s41590-018-0305-x.
- Kastner, B., Fischer, N., Golas, M. M., Sander, B., Dube, P., Boehringer, D., … Stark, H. (2008). GraFix: Sample preparation for single-particle electron cryomicroscopy. Nature Methods , 5(1), 53–55. doi: 10.1038/nmeth1139.
- Kimanius, D., Forsberg, B. O., Scheres, S. H., & Lindahl, E. (2016). Accelerated cryo-EM structure determination with parallelisation using GPUs in RELION-2. Elife , 5, e18722. doi: 10.7554/eLife.18722.
- Kremer, J. R., Mastronarde, D. N., & McIntosh, J. R. (1996). Computer visualization of three-dimensional image data using IMOD. Journal of Structural Biology , 116(1), 71–76. doi: 10.1006/jsbi.1996.0013.
- Kuhlbrandt, W. (2014). Biochemistry. The resolution revolution. Science , 343(6178), 1443–1444. doi: 10.1126/science.1251652.
- Lyumkis, D., Brilot, A. F., Theobald, D. L., & Grigorieff, N. (2013). Likelihood-based classification of cryo-EM images using FREALIGN. Journal of Structural Biology , 183(3), 377–388. doi: 10.1016/j.jsb.2013.07.005.
- Mastronarde, D. N. (2005). Automated electron microscope tomography using robust prediction of specimen movements. Journal of Structural Biology , 152(1), 36–51. doi: 10.1016/j.jsb.2005.07.007.
- Ohi, M., Li, Y., Cheng, Y., & Walz, T. (2004). Negative staining and image classification—Powerful tools in modern electron microscopy. Biological Procedures Online , 6, 23–34. doi: 10.1251/bpo70.
- Pettersen, E. F., Goddard, T. D., Huang, C. C., Couch, G. S., Greenblatt, D. M., Meng, E. C., & Ferrin, T. E. (2004). UCSF Chimera—A visualization system for exploratory research and analysis. Journal of Computational Chemistry , 25(13), 1605–1612. doi: 10.1002/jcc.20084.
- Punjani, A., Rubinstein, J. L., Fleet, D. J., & Brubaker, M. A. (2017). cryoSPARC: Algorithms for rapid unsupervised cryo-EM structure determination. Nature Methods , 14(3), 290–296. doi: 10.1038/nmeth.4169.
- Rohou, A., & Grigorieff, N. (2015). CTFFIND4: Fast and accurate defocus estimation from electron micrographs. Journal of Structural Biology , 192(2), 216–221. doi: 10.1016/j.jsb.2015.08.008.
- Rosenthal, P. B., & Henderson, R. (2003). Optimal determination of particle orientation, absolute hand, and contrast loss in single-particle electron cryomicroscopy. Journal of Molecular Biology , 333(4), 721–745. doi: 10.1016/j.jmb.2003.07.013.
- Scarff, C. A., Fuller, M. J. G., Thompson, R. F., & Iadaza, M. G. (2018). Variations on negative stain electron microscopy methods: Tools for tackling challenging systems. Journal of Visualized Experiments: JoVE(132). doi: 10.3791/57199.
- Scheres, S. H. (2012). RELION: Implementation of a Bayesian approach to cryo-EM structure determination. Journal of Structural Biology , 180(3), 519–530. doi: 10.1016/j.jsb.2012.09.006.
- Scheres, S. H., & Chen, S. (2012). Prevention of overfitting in cryo-EM structure determination. Nature Methods , 9(9), 853–854. doi: 10.1038/nmeth.2115.
- Schindelin, J., Arganda-Carreras, I., Frise, E., Kaynig, V., Longair, M., Pietzsch, T., … Cardona, A. (2012). Fiji: An open-source platform for biological-image analysis. Nature Methods , 9(7), 676–682. doi: 10.1038/nmeth.2019.
- Suloway, C., Pulokas, J., Fellmann, D., Cheng, A., Guerra, F., Quispe, J., … Carragher, B. (2005). Automated molecular microscopy: The new Leginon system. Journal of Structural Biology , 151(1), 41–60. doi: 10.1016/j.jsb.2005.03.010.
- Tang, G., Peng, L., Baldwin, P. R., Mann, D. S., Jiang, W., Rees, I., & Ludtke, S. J. (2007). EMAN2: An extensible image processing suite for electron microscopy. Journal of Structural Biology , 157(1), 38–46. doi: 10.1016/j.jsb.2006.05.009.
- van Heel, M., & Frank, J. (1981). Use of multivariate statistics in analysing the images of biological macromolecules. Ultramicroscopy , 6(2), 187–194. doi: 10.1016/0304-3991(81)90059-0.
- Wingfield, P. T. (2015). Overview of the purification of recombinant proteins. Current Protocols in Protein Science , 80, 6 1 1–35. doi: 10.1002/0471140864.ps0601s80.
- Zhang, K. (2016). Gctf: Real-time CTF determination and correction. Journal of Structural Biology , 193(1), 1–12. doi: 10.1016/j.jsb.2015.11.003.
- Zhang, L., Song, J., Cavigiolio, G., Ishida, B. Y., Zhang, S., Kane, J. P., … Ren, G. (2011). Morphology and structure of lipoproteins revealed by an optimized negative-staining protocol of electron microscopy. Journal of Lipid Research , 52(1), 175–184. doi: 10.1194/jlr.D010959.
Internet Resources
CCPEM listserve is for discussion related to the RELION software package.
RELION Web site includes descriptions of software installation and best practices, as well as discussion of errors and software bugs.
RELION FAQ is an important resource for strategies on CPU and memory usage by RELION.
General Linux command line tutorials, which can be used to solve many problems that arise in batch processing large sets of data files.
The VI command-line text editor is powerful, especially for the “search,” and “search and replace” functionality.
“xargs” often requires working examples to learn from, such as offered here.
SerialEM Web site includes usage and installation guides.
Citing Literature
Number of times cited according to CrossRef: 26
- Chloe Kan, Ata Ullah, Shangyu Dang, Hong Xue, Modular Structure and Polymerization Status of GABAA Receptors Illustrated with EM Analysis and AlphaFold2 Prediction, International Journal of Molecular Sciences, 10.3390/ijms251810142, 25 , 18, (10142), (2024).
- Kayla Neyra, Heather R. Everson, Divita Mathur, Dominant Analytical Techniques in DNA Nanotechnology for Various Applications, Analytical Chemistry, 10.1021/acs.analchem.3c04176, 96 , 9, (3687-3697), (2024).
- Chantal M. Trepanier, Jonathan Rubianto, Jonah Burke-Kleinman, Ryan Appings, Michelle P. Bendeck, J. Paul Santerre, Synthesis, characterization, and surface modification of degradable polar hydrophobic ionic polyurethane nanoparticles for the delivery of therapeutics to vascular tissue, Acta Biomaterialia, 10.1016/j.actbio.2024.09.024, 188 , (184-196), (2024).
- Nora Mallouk, Sisareuth Tan, Claire Scepaniack, Arnauld Garcin, Camille Pradat, Claude Lambert, Guorong Li, A specific technique of immunolabelling of urinary small extracellular vesicle biomarkers for the diagnostic of renal cancer, Microscopy, 10.1093/jmicro/dfad007, 72 , 5, (450-454), (2023).
- Eugene M. Obeng, David L. Steer, Alex J. Fulcher, Kylie M. Wagstaff, Sortase A transpeptidation produces seamless, unbranched biotinylated nanobodies for multivalent and multifunctional applications, Nanoscale Advances, 10.1039/D3NA00014A, 5 , 8, (2251-2260), (2023).
- Mallory L. Myers, John R. Gallagher, Alexander J. Kim, Walker H. Payne, Samantha Maldonado-Puga, Haralabos Assimakopoulos, Kevin W. Bock, Udana Torian, Ian N. Moore, Audray K. Harris, Commercial influenza vaccines vary in HA-complex structure and in induction of cross-reactive HA antibodies, Nature Communications, 10.1038/s41467-023-37162-z, 14 , 1, (2023).
- Fabio Lisi, Jun Sawayama, Shreedhar Gautam, Sergey Rubanov, Xiaofei Duan, Nicholas Kirkwood, Re-Examination of the Polymer Encapsulation of Quantum Dots for Biological Applications, ACS Applied Nano Materials, 10.1021/acsanm.3c00529, 6 , 5, (4046-4055), (2023).
- Antonio José Guillot, Miquel Martínez-Navarrete, Teresa M. Garrigues, Ana Melero, Skin drug delivery using lipid vesicles: A starting guideline for their development, Journal of Controlled Release, 10.1016/j.jconrel.2023.02.006, 355 , (624-654), (2023).
- Francisco Mendoza-Hoffmann, Canyong Guo, Yanzhuo Song, Dandan Feng, Lingyun Yang, Kurt Wüthrich, 19F-NMR studies of the impact of different detergents and nanodiscs on the A2A adenosine receptor, Journal of Biomolecular NMR, 10.1007/s10858-023-00430-7, 78 , 1, (31-37), (2023).
- Stephan Niebling, Katharina Veith, Benjamin Vollmer, Javier Lizarrondo, Osvaldo Burastero, Janina Schiller, Angelica Struve García, Philipp Lewe, Carolin Seuring, Susanne Witt, María García-Alai, Biophysical Screening Pipeline for Cryo-EM Grid Preparation of Membrane Proteins, Frontiers in Molecular Biosciences, 10.3389/fmolb.2022.882288, 9 , (2022).
- Xiangwen Wang, Yonggang Lu, Xianghong Lin, Heterogeneous cryo-EM projection image classification using a two-stage spectral clustering based on novel distance measures, Briefings in Bioinformatics, 10.1093/bib/bbac032, 23 , 3, (2022).
- Sachin Dev, Man Singh, Lanthanide sulfide decorated graphene oxide nanorods as an effective photocatalysts for reducing dyes, free radicals, and water split to produce H2 in sunlight, Solid State Sciences, 10.1016/j.solidstatesciences.2022.107045, 134 , (107045), (2022).
- Ryan Boyd, Liqiang Zhang, Petra Fromme, Native Deglycosylation and Size Exclusion Chromatography of Viral Chemokine Binding Proteins for Structural Discovery, Chemokine-Glycosaminoglycan Interactions, 10.1007/978-1-0716-2835-5_18, (251-259), (2022).
- Huping Wang, Manajit Hayer-Hartl, Phase Separation of Rubisco by the Folded SSUL Domains of CcmM in Beta-Carboxysome Biogenesis, Phase-Separated Biomolecular Condensates, 10.1007/978-1-0716-2663-4_14, (269-296), (2022).
- Ilona Nudelman, Javier Fernandez-Martinez, Michael P. Rout, Affinity Isolation of Endogenous Saccharomyces Cerevisiae Nuclear Pore Complexes, The Nuclear Pore Complex, 10.1007/978-1-0716-2337-4_1, (3-34), (2022).
- Ishtiaque Rashid, Miaw-Sheue Tsai, Aleksandr Sverzhinsky, Aye Su Hlaing, Brian Shih, Aye C. Thwin, Judy G. Lin, Su S. Maw, John M. Pascal, Alan E. Tomkinson, Purification and Characterization of Human DNA Ligase IIIα Complexes After Expression in Insect Cells, DNA Damage Responses, 10.1007/978-1-0716-2063-2_15, (243-269), (2022).
- Ahmed Ali Abd El-Maksoud, Amal I. A. Makhlouf, Ammar B. Altemimi, Ismail H. Abd El-Ghany, Amr Nassrallah, Francesco Cacciola, Tarek Gamal Abedelmaksoud, Nano Milk Protein-Mucilage Complexes: Characterization and Anticancer Effect, Molecules, 10.3390/molecules26216372, 26 , 21, (6372), (2021).
- Xiangwen Wang, Yonggang Lu, Jiaxuan Liu, A Fast Image Alignment Approach for 2D Classification of Cryo-EM Images Using Spectral Clustering, Current Issues in Molecular Biology, 10.3390/cimb43030117, 43 , 3, (1652-1668), (2021).
- Ryan G. Gaudet, Shiwei Zhu, Anushka Halder, Bae-Hoon Kim, Clinton J. Bradfield, Shuai Huang, Dijin Xu, Agnieszka Mamiñska, Thanh Ngoc Nguyen, Michael Lazarou, Erdem Karatekin, Kallol Gupta, John D. MacMicking, A human apolipoprotein L with detergent-like activity kills intracellular pathogens, Science, 10.1126/science.abf8113, 373 , 6552, (2021).
- Kelly Parker, Justin Modica, Charlene Wilke, Roberto dos Reis, Milan Mrksich, Vinayak Dravid, To Cryo or Not to Cryo? A Consideration of Length Scales During Macromolecule Sample Preparation, Microscopy and Microanalysis, 10.1017/S1431927621005213, 27 , S1, (1404-1407), (2021).
- Janaína Artem Ataide, Danilo Costa Geraldes, Eloah Favero Gérios, Fernanda Mazon Bissaco, Letícia Caramori Cefali, Laura Oliveira-Nascimento, Priscila Gava Mazzola, Freeze-dried chitosan nanoparticles to stabilize and deliver bromelain, Journal of Drug Delivery Science and Technology, 10.1016/j.jddst.2020.102225, 61 , (102225), (2021).
- Keisuke Kawakami, Ryo Nagao, Yuhei O. Tahara, Tasuku Hamaguchi, Takehiro Suzuki, Naoshi Dohmae, Daisuke Kosumi, Jian-Ren Shen, Makoto Miyata, Koji Yonekura, Nobuo Kamiya, Structural implications for a phycobilisome complex from the thermophilic cyanobacterium Thermosynechococcus vulcanus, Biochimica et Biophysica Acta (BBA) - Bioenergetics, 10.1016/j.bbabio.2021.148458, 1862 , 9, (148458), (2021).
- George R. Heath, Yi-Chih Lin, Tina R. Matin, Simon Scheuring, Structural dynamics of channels and transporters by high-speed atomic force microscopy, Ion Channels: Channel Biochemistry, Reconstitution, and Function, 10.1016/bs.mie.2021.03.011, (127-159), (2021).
- Molly S. C. Gravett, Ryan C. Cocking, Alistair P. Curd, Oliver Harlen, Joanna Leng, Stephen P. Muench, Michelle Peckham, Daniel J. Read, Jarvellis F. Rogers, Robert C. Welch, Sarah A. Harris, Moving in the mesoscale: Understanding the mechanics of cytoskeletal molecular motors by combining mesoscale simulations with imaging, WIREs Computational Molecular Science, 10.1002/wcms.1570, 12 , 3, (2021).
- Susan Krueger, Joseph E. Curtis, Daniel R. Scott, Alexander Grishaev, Greg Glenn, Gale Smith, Larry Ellingsworth, Oleg Borisov, Ernest L. Maynard, Structural Characterization and Modeling of a Respiratory Syncytial Virus Fusion Glycoprotein Nanoparticle Vaccine in Solution, Molecular Pharmaceutics, 10.1021/acs.molpharmaceut.0c00986, 18 , 1, (359-376), (2020).
- Kevin J. Metcalf, Blaise R. Kimmel, Daniel J. Sykora, Justin A. Modica, Kelly A. Parker, Eric Berens, Raymond Dai, Vinayak P. Dravid, Zena Werb, Milan Mrksich, Synthetic Tuning of Domain Stoichiometry in Nanobody–Enzyme Megamolecules, Bioconjugate Chemistry, 10.1021/acs.bioconjchem.0c00578, 32 , 1, (143-152), (2020).