Towards the Development, Maintenance, and Standardized Phenotypic Characterization of Single-Seed-Descent Genetic Resources for Common Bean
Saleh Alseekh, Saleh Alseekh, Alisdair R. Fernie, Alisdair R. Fernie, Elena Bitocchi, Elena Bitocchi, Markus Oppermann, Markus Oppermann, Kerstin Neumann, Kerstin Neumann, Elisa Bellucci, Elisa Bellucci, Andreas Graner, Andreas Graner, Roberto Papa, Roberto Papa, Gaia Cortinovis, Gaia Cortinovis, Tania Gioia, Tania Gioia, Marco Marsella, Marco Marsella
common bean
genetic diversity
genetic resources
intelligent collections
single-seed-descent line
standardized phenotyping protocols
Abstract
The optimal use of legume genetic resources represents a key prerequisite for coping with current agriculture-related societal challenges, including conservation of agrobiodiversity, agricultural sustainability, food security, and human health. Among legumes, the common bean (Phaseolus vulgaris) is the most economically important for human consumption, and its evolutionary trajectories as a species have been crucial to determining the structure and level of its present and available genetic diversity. Genomic advances are considerably enhancing the characterization and assessment of important genetic variants. For this purpose, the development and availability of, and access to, well-described and efficiently managed genetic resource collections that comprise pure lines derived by single-seed-descent cycles will be paramount for the use of the reservoir of common bean variability and for the advanced breeding of legume crops. This is one of the main aims of the new and challenging European project INCREASE, which is the implementation of Intelligent Collections with appropriate standardized protocols that must be characterized, maintained, and made available, along with the related data, to users such as breeders and researchers. © 2021 The Authors. Current Protocols published by Wiley Periodicals LLC.
Basic Protocol 1 : Characterizing common bean seeds for seed trait descriptors
Basic Protocol 2 : Bean seed imaging
Basic Protocol 3 : Characterizing bean lines for plant trait descriptors specific for common bean Primary Seed Increase
INTRODUCTION
Origin, Domestication and Diffusion, and Evolution Out of the Centers of Origin
The Leguminosae family consists of about 770 genera and over 19,500 species (Azani et al., 2017; Lewis et al., 2013). It currently represents the second most agriculturally important crop family globally, after the cereals (Graham & Vance, 2003). Due to their nutritional quality, biological nitrogen fixation capacity, and broad adaptation to various agro-ecological conditions, legumes have a crucial role in helping to overcome key agriculture-related societal challenges, such as the mitigation of and adaptation to climate change, agrobiodiversity conservation, agricultural sustainability, food security, and human health. Among the legumes, beans (Phaseolus ssp.) are the most important for direct human consumption throughout the world, particularly the common bean (Phaseolus vulgaris ; Broughton et al., 2003). Here, we provide a brief overview of the evolutionary history of P. vulgaris and the ongoing efforts for innovative and sustainable conservation and management of its broad genetic diversity.
P. vulgaris originated in Mexico (Bitocchi et al., 2012) and later, through different migration events, became widespread across the highlands of Latin America and into northwestern Argentina (Toro et al., 1990). The common bean is characterized by three eco-geographic gene pools: Mesoamerica and the Andes, the two major gene pools, which include both wild and domesticated forms; and northern Peru–Ecuador, with a relatively narrow distribution (i.e., western slopes of the Andes) that includes only wild forms. The Mesoamerican origin of the common bean was confirmed relatively recently (Ariani, Mier y Teran, & Gepts, 2017; Desiderio et al., 2013; Rendón-Anaya et al., 2017; Schmutz et al., 2014). However, debate continues on the timing of its dispersal to South America and the evolutionary consequences (see Cortinovis, Frascarelli, et al., 2020, for a review).
The wide geographic extent where wild forms of P. vulgaris grow implies that they are characterized by adaptation to different environments, as distinct from those of the Mesoamerican population. In this regard, Rodriguez et al. (2016), and more recently Ariani and Gepts (2019), carried out landscape genomics analyses based on wide samples of wild P. vulgaris genotypes and high-throughput genomic data to identify several genomic regions that show signatures of selection for adaptation.
Common bean also underwent two parallel and independent domestication events, one in Mesoamerica and the other in the Andes, which gave rise to the current two major domesticated gene pools. At the genomic level, domestication caused a reduction in the genetic diversity of the domesticated germplasm (for review, see Bitocchi, Rau, Bellucci et al., 2017, and Cortinovis, Frascarelli, et al., 2020) due to demographic factors that affected the entire genome, and to natural and artificial selection at target loci. Domestication had a major impact not only by reducing the diversity of domesticated forms compared to the wild population at the nucleotide level but also reducing the diversity of gene expression, as was seen through a scan of transcriptome diversity performed by Bellucci, Bitocchi, Ferrarini, et al. (2014).
In addition, some observations have identified an increase in functional diversity at target loci that harbor genes involved in environmental adaptation, including adaptation to both biotic and abiotic factors (Bellucci, Bitocchi, Ferrarini et al., 2014; Bitocchi, Rau, Benazzo, et al., 2017).
The next step in the evolution of the common bean was its spread out of the Americas (Cortinovis, Di Vittori, et al., 2020). This was a very complex process involving several introductions from the American continent, accompanied by several exchanges between different continents and countries, due to intensive commercial interactions (for reviews, see Bellucci, Bitocchi, Rau, Rodriguez, et al., 2014; Bitocchi, Rau, Bellucci, et al., 2017; Cortinovis, Di Vittori, et al., 2020). Particularly interesting, in terms of genetic variability and adaptation, is the breakdown of the spatial geographical barriers between the Mesoamerican and Andean genotypes that characterized the evolution of the common bean out of the New World. In particular, in Europe, this process favored hybridization and introgression between gene pools, increasing the possibility that novel genotypes and phenotypes would arise (Angioi et al., 2010; Gioia et al., 2013).
Worldwide Germplasm Collections
Nikolai Ivanovich Vavilov was one of the first pioneers to recognize the exceptional importance and potential value of collecting and conserving the wide genetic diversity of a crop and its wild relatives (Vavilov, 1920, 1922), which still remain largely unexploited. He highlighted the crucial role of wild relatives of crop plants as sources of genes for the exploitation of natural and artificial introgression. This specific point was formalized by Harlan and de Wet (1971) through the introduction of the “gene-pool concept,” which was very useful for investigating how genes can be transferred between species. Each crop is characterized by a pool of genetic diversity that can potentially be available for use in breeding. This pool can be classified on the basis of the degree of crossing ability between the crop itself and its wild relatives. The primary gene pool (GP-1) corresponds to the biological species, which includes the crop and individuals that have no barriers to reproduction. The secondary gene pool (GP-2) includes less closely related species, for which hybridization with the crop is possible, but difficult. Within the tertiary gene pool (GP-3), crosses are feasible through advanced techniques, such as protoplast fusion, embryo rescue, or genetic engineering, whereas the quaternary gene pool (GP-4) is characterized by a lack of success in obtaining fertile hybrids by any means. Based on this concept, Figure 1 illustrates the species related to P. vulgaris and their degrees of relationship with P. vulgaris in terms of crossing ability.
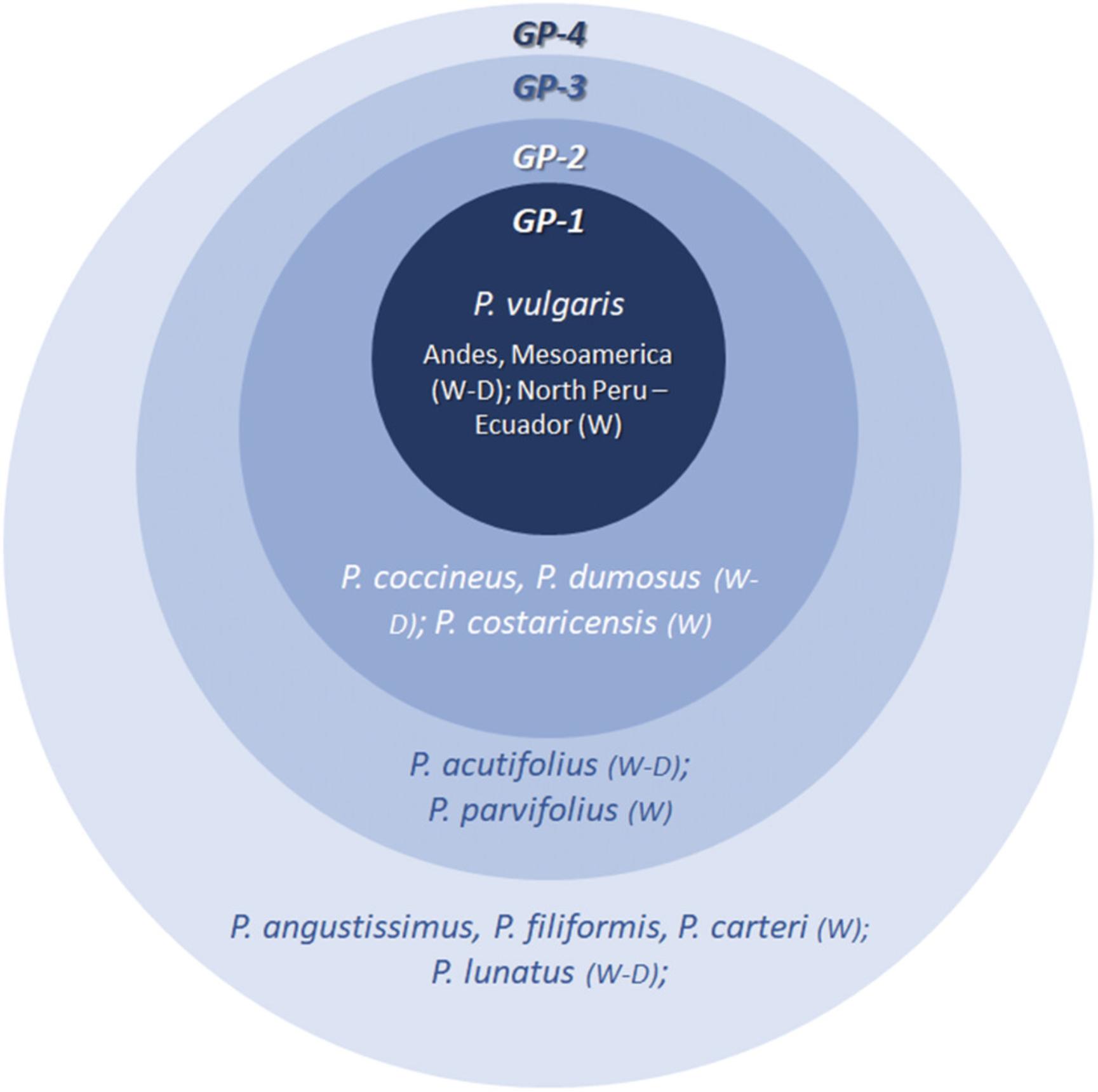
Considering the current challenges posed by climate, agriculture, and food production, the conservation of plant genetic resources (PGRs) is now becoming imperative, along with their characterization and use (McCouch et al., 2020; Mousavi-Derazmahalleh et al., 2019). Generally, the major proportion of the genetic diversity of a crop is contributed by its wild relatives, as they have not experienced domestication and the resulting reduction of diversity (Diamond, 2002; Gepts, 2010; Glémin & Bataillon, 2009). Landraces are also important repositories of the genetic diversity of a crop, as these represent local varieties that have evolved through natural and artificial selection over millennia adapting to specific and diversified agro-environmental conditions, without undergoing genetic bottlenecks due to modern breeding techniques (Zeven, 1998) or rapid adaptation to specific and diversified agro-environmental conditions (Bellucci et al., 2013; Bitocchi et al., 2009, 2015; Dwivedi et al., 2016; Mir, Sharma, & Mahajan, 2020; Zhu et al., 2000). Thus, landraces and wild relatives harbor functional and adaptive genetic variation that needs to be more easily managed and used.
Thus, as the first step, PGRs and their wide diversity need to be maintained. Germplasm banks can guarantee the conservation ex situ of such biodiversity. Genesys PGR is a free online global portal that allows the exploration of plant species diversity through a single website (accessible at www.genesys-pgr.org). The data published on Genesys follow the standards for Multi-Crop Passport Descriptors (as defined by the United Nations Food and Agriculture Organization [FAO] and Bioversity). For P. vulgaris , Genesys contains over 135,500 accessions (as of October 19, 2020), which are stored in several holding institutions worldwide. Most of these accessions are landraces (∼71,000), followed by improved cultivars (∼20,000), and wild (∼2000) and breeding/research materials (∼2600). Figure 2 shows the geographic distribution of the wild and landrace accessions of common bean for which information on geographic coordinates is available in Genesys. The International Centre for Tropical Agriculture (CIAT) in Colombia holds the largest P. vulgaris collection, with ∼32,000 accessions, followed by the United States Department of Agriculture Agricultural Research Service (USDA-ARS), with nearly 14,000 accessions, and the Leibniz Institute of Plant Genetics and Crop Plant Research (IPK) in Germany, with ∼8400 accessions. Numerous common bean accessions (∼27,700 overall) are also held by a Brazilian gene bank (the Empresa Brasileira de Pesquisa Agropecuária, or EMBRAPA). Recursos Genéticos e Biotecnologia CENARGEN, Brasília, DF, and Arroz e Feijão, CNPAF, Goiânia, GO). Furthermore, the Russian collection of common beans at the N.I. Vavilov Institute of Plant Genetic Resources (VIR) is one of the oldest in the world. The VIR collection now numbers ∼6400 accessions (according to information in Genesys) and is the result of the collective efforts of several generations of scientists and explorers, beginning in the early twentieth century.
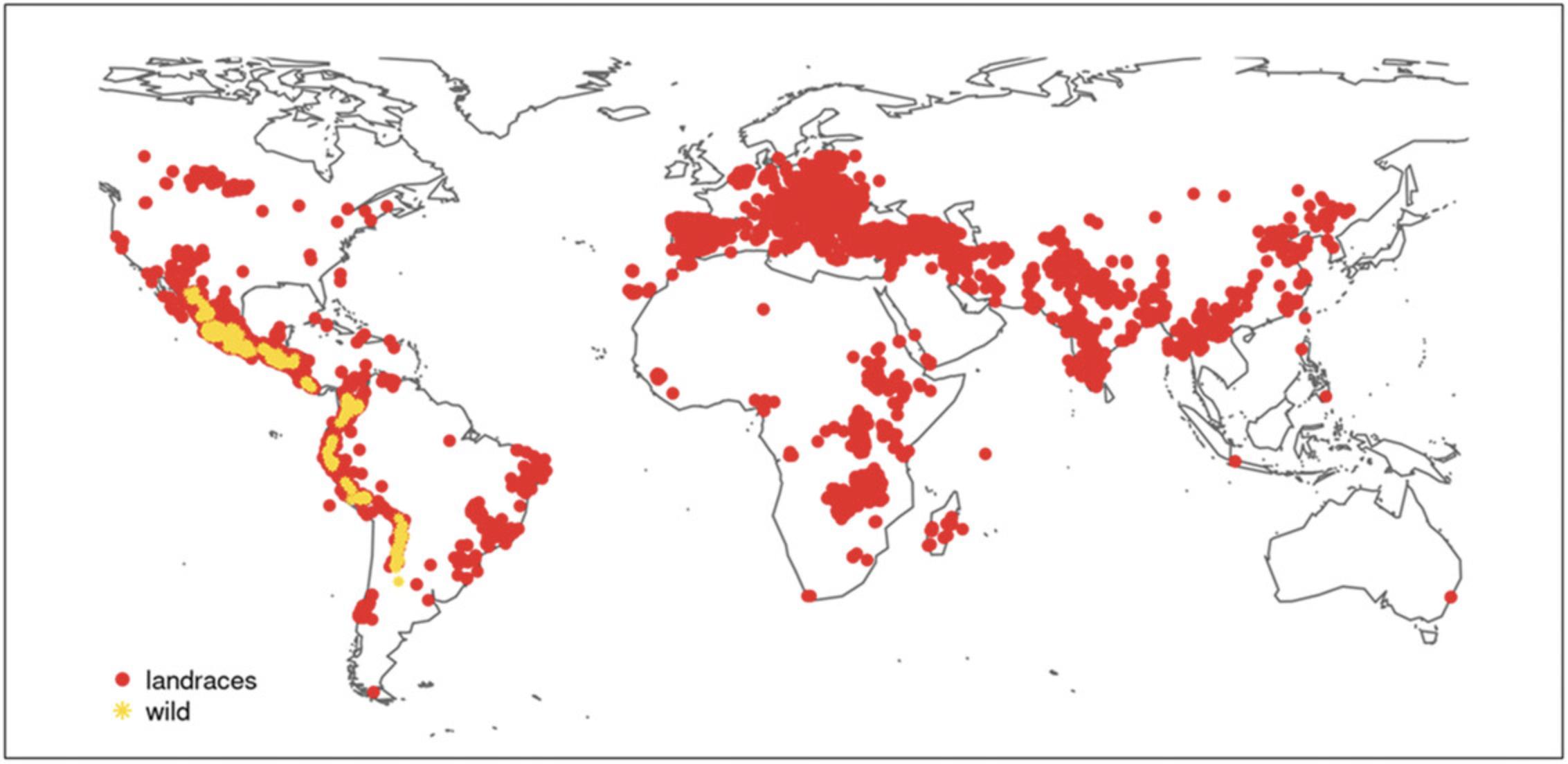
P. vulgaris germplasm collections stored in gene banks all around the world are essential for national and international attempts to ensure the safety (i.e., conservation and maintenance) and use of such PGRs.
Genomics of Genetic Resources
The genomics era has led to a rapid increase in available sequence data, which has thus provided a more detailed picture of the genetic diversity and structure of crop germplasm, as well as enabling the identification of genetic variants that are the bases of important heritable target traits (Luikart et al., 2018). Genome-wide fingerprinting of single-seed descent (SSD) gene-bank accessions allowed the characterization of a complete barley collection (Milner et al., 2019) and resulted in further identification of potential duplicated material within (Milner et al., 2019) and across (Singh et al., 2019) collections. Moreover, the accurate description of the genetic topology of a gene pool, as revealed by principal-component analysis or other population genomics approaches, allows the identification of hitherto under-represented parts of the overall genetic diversity, and can suggest strategies for completing a collection. Furthermore, once a collection is fingerprinted, existing historic phenotype data obtained during seed multiplication can be used. In the case of barley, the statistical analysis of historic data of key agronomic traits resulted in high heritability estimates for the collection (González et al., 2018), so that users can make informed selections of lines of interest.
For the common bean, the current availability of high-throughput sequencing platforms has allowed the release of high-quality reference genomes of the Andean G19833 (Schmutz et al., 2014) and Mesoamerican BAT93 (Vlasova et al., 2016) genotypes. A further high-quality common bean reference genome of the race Durango pinto UI111 genotype was also released recently (P. vulgaris UI111 v1.1, DOE-JGI and USDA-NIFA, http://phytozome.jgi.doe.gov/). Genetic characterization through high-throughput sequencing of common bean collections and segregant populations is increasing in the literature. One major study that aims to characterize a very wide sample of common bean genetic resources is the BEAN_ADAPT project, which is funded through the second European Research Area Network for Coordinating Action in Plant Sciences (ERA-CAPS) joint funding call. As PGRs are often heterogeneous, working with them has required the development of SSD seeds to obtain purified and genetically homogeneous material that is suitable for genetic studies. Therefore, BEAN_ADAPT is based on the characterization of nested core collections, developed by SSD, that have been genotyped at different levels on the basis of their size. The Pv_ALL collection, a large collection of ∼10,000 accessions (mostly European and from the American centers of origin), and a subsample of 500 lines (Pv_core1) have both been characterized through genotyping-by-sequencing methods, while a further subsample of 220 accessions have been genotyped by whole-genome sequencing. The genetic data are now being used in combination with the different phenotypic evaluations to identify the genetic basis of phenotypic variants related to adaptation to new environments, through the study of the introduction and wide spread of the common bean from the Americas into Europe. Moreover, the overall design of the project and the data obtained can be also used to predict the phenotypes of the whole collection based on the genotype data, resulting in a very useful tool for exploiting the huge number of PGRs that are currently maintained in gene banks and that remain widely underexploited.
Several genomic studies have been performed based on two particular panels: the Middle American Diversity Panel, which was developed as part of the USDA-funded Common Bean Coordinated Agricultural Project (BeanCAP) and consists of 280 modern bean genotypes that are mostly from the race Mesoamerica, but also from Durango and Jalisco (Moghaddam et al., 2016); and the Andean Diversity Panel, which consists of 396 accessions, the majority of which belong to the Andean gene pool (349), while 21 are Mesoamerican, and 26 are from admixtures between the Mesoamerican and Andean gene pools (Chichy, Porch, et al., 2015). Moghaddam et al. (2016) genotyped the Middle American Diversity Panel with two Illumina iSelect 6K gene chip sets (BARCBEAN6K_1 and BARCBEAN6K_2; Song et al., 2015) and two low-pass sequencing protocols (Elshire et al., 2011; Schröder et al., 2016). Overall, the panel was genotyped at 217,486 mapped single-nucleotide polymorphisms (SNPs). These data were combined with phenotype evaluations for classical agronomic traits (carried out in four different American locations), which were used to perform genome-wide association studies (GWAS). This identified new and known genomic regions that affect various agronomic traits, such as days to flowering, days to maturity, growth habit, lodging, canopy height, and seed weight (Moghaddam et al., 2016).
The Andean Diversity Panel was developed and genotyped at 5398 SNPs using the Illumina BARCBean6K_3 SNP BeadChip (Cichy, Porch, et al., 2015). The SNP data were used to genetically characterize the panel by investigating the level and structure of its genetic diversity, and they were also coupled with phenotypic data (i.e., plant determinacy) to identify marker-trait associations. These panels (or subsamples of them, or as part of larger collections) have been used in further GWAS studies (Cichy, Wiesinger, & Mendoza, 2015; Kamfwa, Cichy, & Kelly, 2015a, 2015b; McClean et al., 2017, 2018; Moghaddam et al., 2017; Oladzad, Zitnick-Anderson, et al., 2019; Parker et al., 2020; Soltani et al., 2017; Tock et al., 2017; Zuiderveen, Padder, Kamfwa, Song, & Kelly, 2016), demonstrating the potential of such materials for pre-breeding and breeding studies. Recently, Almeida et al. (2020) also efficiently used SNPs to characterize the diversity of an association panel of Carioca strains from Brazil.
Recently, Oladzad, Porch, et al. (2019) reported the development of the moderately sized Bean Abiotic Stress Evaluation panels for the U.S. Agency for International Development (USAID) Climate Resilience Bean (CRIB) project, which aims to investigate the genetics and physiological mechanisms of the responses of dry beans cultivated under abiotic stress. These panels are of modest size (∼120 lines) and are managed by research groups with limited resources; however, at the same time, they are large enough to define the genetic variance at the basis of phenotypic variability. Oladzad, Porch, et al. (2019) carried out genotyping by sequencing for the Bean Abiotic Stress Evaluation panels, and they analyzed all of the data to identify markers associated with production traits under both heat- and drought-stress environments.
Collections and/or panels have also been developed with a focus on snap bean genetic resources. Within the BeanCAP project, a snap bean diversity panel was developed that consisted of 149 cultivars from North America and Europe (Kleintop, Myers, Echeverria, Thompson, & Brick, 2016). Wallace, Arkwazee, Vining, and Myers (2018) genotyped the BeanCAP Snap Bean Panel along with a further 55 Chinese and 19 Spanish snap bean genotypes and 24 heirloom beans at 5398 SNPs. This study provided a deep investigation of the genetic diversity and structure of this collection, which represents a very useful tool for further GWAS. Myers et al. (2019) characterized this panel for total phenolic contents and genotyped them at 10,073 SNPs, and they used GWAS for identification of 11 quantitative trait nucleotides associated with this trait.
Another interesting example of the use of whole-genome scan analysis to characterize P. vulgaris genetic resources was conducted by Wu et al. (2020) and focused on the variability of Chinese common bean germplasm compared to worldwide accessions, and on the relevant contributions of landrace material. They applied whole-genome resequencing to a collection of 683 accessions, which comprised 529 landraces and 154 breeding lines that were representative of both the Mesoamerican and Andean gene pools. The plant material was grown over 3 years and in four locations in China that are characterized by different agro-ecological conditions, with the aim of investigating trait associations related to P. vulgaris yield across the north-south geographic clines. Wu et al. (2020) detected a total of 4,811,097 SNPs and several genetic differences in terms of structural variations affecting DNA segments of >1 kbp, such as insertions, deletions, copy number variations, and presence/absence variations. By using GWAS, these researchers defined several marker-trait associations that were dispersed across the entire genome for all of the traits considered, even though they were found in different proportions depending on the inheritance level and the complexity of the trait itself.
Segregant populations also represent valuable PGRs. As an example of the use of wild individuals to introgress new variability into elite germplasm, for instance for the common bean, Murgia et al. (2017) phenotypically characterized a very interesting introgression line (IL) population. This population was developed from an initial cross between the domesticated Andean variety Midas and the G12873 line, a wild Mesoamerican accession (Koinage et al., 1996). Several cycles of selfing and subsequent backcrosses with the recurrent parent Midas allowed the development of a set of 70 ILs from BC3/F4:F5 families and 217 ILs from BC3/F6:F7 families (Murgia et al., 2017; Rau et al., 2019). Phenotypic characterization for pod shattering (Murgia et al., 2017), combined with genetic characterization through genotyping by sequencing at 14,196 SNPs, allowed Rau et al. (2019) to investigate the genetic architecture of the shattering trait in common bean. They identified a locus in the distal part of chromosome Pv05 as the primary locus responsible for the pod indehiscence phenotype, along with numerous other secondary quantitative trait loci that contributed to the modulation of this phenotype. Recently, Di Vittori et al. (2020) continued the development of this IL population: from the Murgia et al. (2017) IL population, they selected six highly shattering ILs and used these as donor parents for high pod shattering for further backcrosses (BC4) with Midas, to provide an IL population of 1197 BC4/F4 individuals that was then genetically (19,420 SNPs) and phenotypically evaluated for pod shattering. Along with gene expression and parallel histological analysis of dehiscent and indehiscent pods, a GWAS by Di Vittori et al. (2020) identified an ortholog of AtMYB26 from Arabidopsis thaliana as the best candidate for loss of pod shattering, in a genomic region ∼11 kb downstream of the most highly associated peak.
Development and Maintenance of Common Bean Increase Intelligent Collections
Several drawbacks characterize conventional conservation management of PGRs. Among these, there is the problem that seed collections are assembled and maintained on an accession basis, with each accession usually constituting a mixture of genotypes that represents a population. As a consequence, the information collected at the phenotype level cannot be directly linked to a specific genotype. Furthermore, hundreds of accessions are conserved and maintained in gene banks with very little information available (i.e., there is a lack of comprehensive information regarding passport data and descriptors useful for users, combined with accession heterogeneity and unharmonized data), which makes their selection and use for specific purposes by researchers and breeders often difficult. Moreover, the available information is not easily accessible, being in databases that are centralized and were not designed to integrate data obtained by external users.
Recently, European Union Horizon 2020 funded Project INCREASE—Intelligent Collections of Food Legumes Genetic Resources for European Agrofood Systems—with the aim of overcoming such limitations and implementing a new approach for conservation, management, and characterization of PGRs (Bellucci et al., submitted).
Along with chickpea, lentil, and lupin (Kroc et al., in preparation; Guerra-García, Gioia, von Wettberg, Logozzo, & Bett, in preparation; Kumar et al., in preparation), common bean is one of the legume species that form the basis of the INCREASE project. With the purpose of conserving, managing, and making the best use of food-legume genetic resources, the INCREASE project is developing innovative conservation procedures through the integration of two complementary strategies (i.e., ex situ and in situ approaches) and the development of SSD-purified accessions based on single homozygous genotypes. SSD collections provide the possibility of associating phenotypes with reliable genotype information, which can then be used to advance and improve legume research, breeding, and cultivation.
To achieve this goal, the project plans to develop INCREASE Intelligent Collections ("intelligent" in terms of being able to memorize, learn, improve, and evolve) as a set of three nested core collections that will be characterized genetically and/or phenotypically at different levels, according to their size. These comprise:
- The Reference Core (R-CORE), as the largest collection. For common bean, this will rely on already available SSD lines from previous projects (e.g., BEAN_ADAPT, BRESOV) complemented with heterogeneous accessions conserved in situ and ex situ, from which SSD lines will be developed to provide a worldwide and highly diverse panel of wild accessions, landraces, and cultivars. This collection will include more than 10,000 SSD lines that will be genotyped using at least a low-coverage approach (e.g., genotyping by sequencing and exome capture), and will be maintained in gene banks for long-term conservation.
- The Training Core (T-CORE), representing a subsample of the R-CORE and comprising ∼450 lines. A deeper sequencing approach is planned for T-CORE (e.g., Illumina whole-genome sequencing), along with broad phenotypic characterization (e.g., classical, molecular, high-throughput phenotyping) under both controlled and field conditions.
- The Hyper-CORE (H-CORE), which will consist of 40 to 80 accessions that are carefully chosen on an evolutionary transect, with the primary aim being to sample the largest possible genotypic diversity of P. vulgaris , including closely related species. These lines will be deeply phenotyped and genotyped, and a subsample of the H-CORE will be also sequenced to develop a pangenome.
The large amount of data produced will be analyzed to (i) investigate the level and structure of the genetic diversity of legume genetic resources; (ii) identify functional variants that might have major roles in determining the phenotypic variation for a large number of traits; and (iii) predict the phenotypes of PGRs in gene banks only on the basis of their genotypic characterization. This will provide an incredible tool in the hands of geneticists and breeders for the improvement of food legumes.
Here, we describe the procedures that will be applied in INCREASE to develop the Intelligent Collections, with a specific focus on the protocols that INCREASE has implemented and proposed for adoption by gene banks and research institutions to obtain SSD lines, to conserve and maintain their seeds, to make the seeds available for users, and to characterize them and integrate the data obtained into a centralized system, which will ultimately be accessible to anyone interested in these PGRs.
Figure 3 shows the workflow established by INCREASE in terms of the SSD development, conservation, maintenance, and characterization procedures. The protocols developed within INCREASE refer to activities related to Work Package 3 of the project, entitled “Sampling core collections, SSD development, DNA extraction and seed distribution.” In particular, the three different phenotyping protocols described below were established to characterize the genetic resources of the project during SSD development and the subsequent seed increase cycles that will be performed under controlled conditions. These protocols were developed starting from IPGRI P. vulgaris descriptors (International Board for Plant Genetic Resources [IBPGR], 1982) and Crop Ontology (Bioversity International, 2011), and they have been modified specifically for purposes related to these SSD development activities.
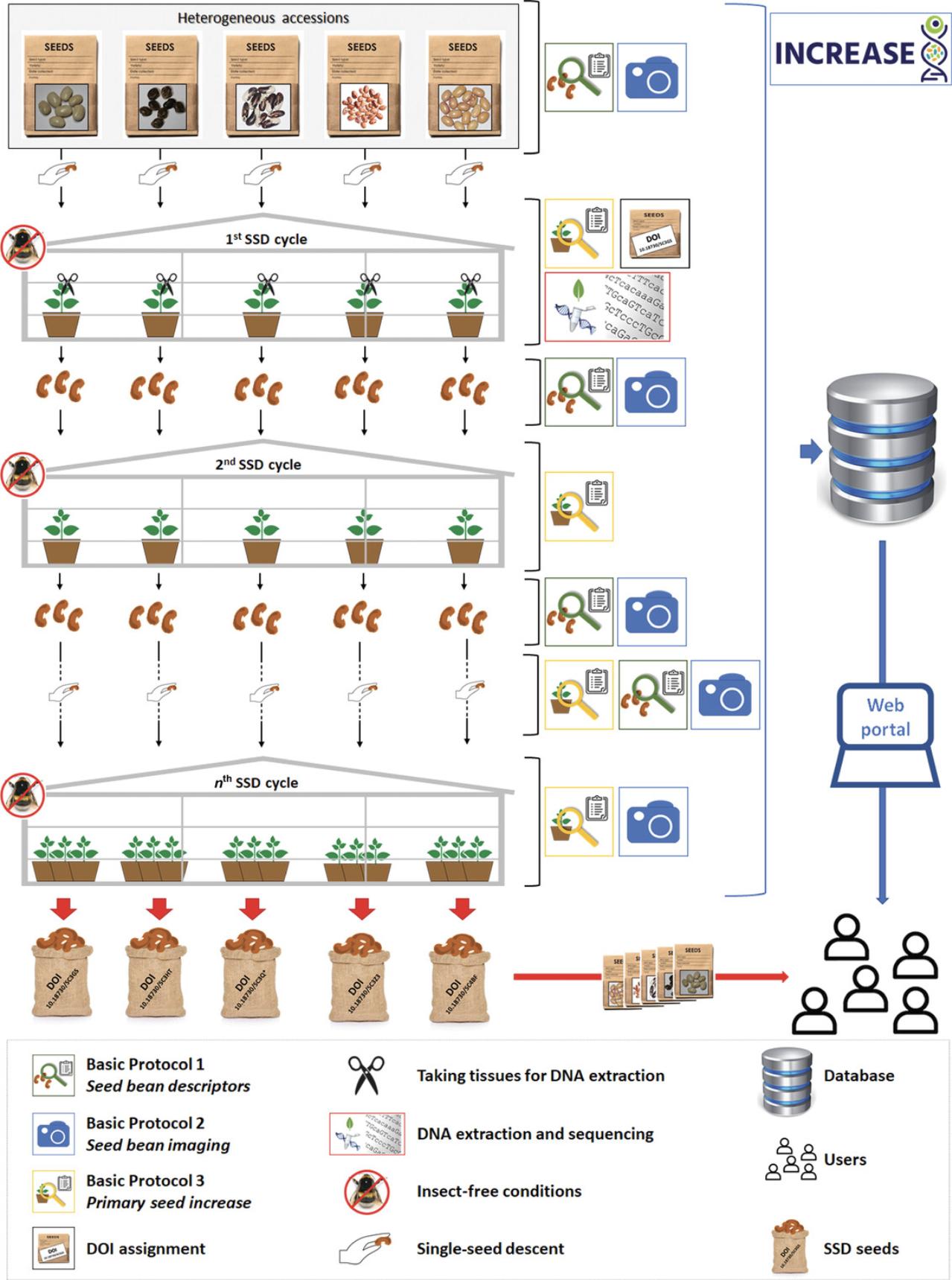
Basic Protocol 1: CHARACTERIZING COMMON BEAN SEEDS FOR SEED TRAIT DESCRIPTORS
This protocol was developed to characterize seeds before the start of any cycle of seed increase. It allows for the characterization of the original phenotypes of seeds from heterogeneous materials, if the cycle to be carried out is the first (development of SSD lines from heterogeneous accessions), and for obtaining data on the seed morphology of each SSD line for each subsequent cycle of seed multiplication. Such data are important not only to obtain a characterization of seeds from different genetic resources but also to detect human and/or technical errors that can eventually occur during the chain of the selfing cycles.
NOTE : Record the seed traits as detailed below at the beginning of each primary seed increase cycle. The phenotypic characterization must be performed before using the seeds in any (and all) selfing cycles.
Materials
- Seeds
- Ruler
- Analytical balance
- Spreadsheet software
1.Take at least five seeds from each accession (heterogeneous materials) or line (previously developed SSD lines), and through visual observation, evaluate and classify the Seed Coat Pattern according to the following categories (Fig. 4):
1.0 = absent 2.1 = constant mottled, spotted 3.2 = striped 4.3 = mottled 5.4 = constant mottled, marmorated 6.5 = spot near the hilum (swallow, geometric type) 7.6 = spot near the hilum (soldier, irregular spot) 8.7 = spot near the hilum (large, diffuse) 9.8 = bipartite/tripartite 10.9 = covered 11.10 = coated 12. 11 = other (specify in the Notes).
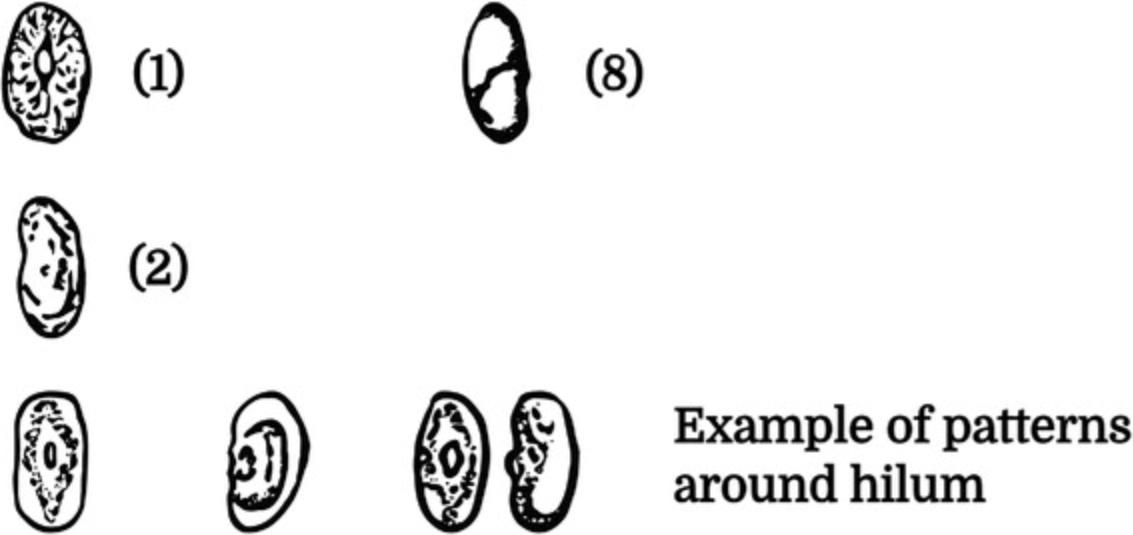
2.Take at least five seeds from each accession (heterogeneous materials) or line (previously developed SSD lines), and through visual observation, evaluate and classify the Seed Coat Coloring according to the following three categories:
- 1 = single colored
- 2 = two colored
- 3 = three (or more than three) colored.
3.Take at least five seeds from each accession (heterogeneous materials) or line (previously developed SSD lines), and through visual observation, evaluate and classify the Seed Coat Ground Color (i.e., primary color) according to the following 24 categories:
- 1 = white
- 2 = greenish white
- 3 = yellow
- 4 = light cream
- 5 = ochre
- 6 = green
- 7 = olive green
- 8 = gray
- 9 = light brown
- 10 = dark brown
- 11 = light purple
- 12 = red purple
- 13 = purple
- 14 = blue-purple
- 15 = black
- 16 = red
- 17 = mustard yellow
- 18 = gray-yellow
- 19 = red-brown
- 20 = pink
- 21 = black-purple
- 22 = gray-black
- 23 = blue
- 24 = other (specify in the Notes).
4.Take at least five seeds from each accession (heterogeneous materials) or line (previously developed SSD lines), and through visual observation, evaluate and classify the Seed Coat Secondary Color (if any) according to the following 24 categories:
- 1 = white
- 2 = greenish white
- 3 = yellow
- 4 = light cream
- 5 = ochre
- 6 = green
- 7 = olive green
- 8 = gray
- 9 = light brown
- 10 = dark brown
- 11 = light purple
- 12 = red-purple
- 13 = purple
- 14 = blue-purple
- 15 = black
- 16 = red
- 17 = mustard yellow
- 18 = gray-yellow
- 19 = red-brown
- 20 = pink
- 21 = black-purple
- 22 = gray-black
- 23 = blue
- 24 = other (specify in the Notes).
5.Take at least five seeds from each accession (heterogeneous materials) or line (previously developed SSD lines), and through visual observation, evaluate the presence/absence of Seed Color Veining.
- 0 = absent
- 1 = present.
6.Take at least five seeds from each accession (heterogeneous materials) or line (previously developed SSD lines), and through visual observation, evaluate and classify the Seed Brilliance (i.e., seed shininess or opaqueness at harvest) according to one of the following three categories:
- 1 = matte
- 2 = medium
- 3 = shiny.
7.Take five seeds from each accession (heterogeneous materials) or line (previously developed SSD lines), and using a ruler, measure the Seed Coat Length (mm) by performing a lateral measurement, parallel to the hilum (Fig. 5). Use the mean of the values obtained from five seeds as the final measure.
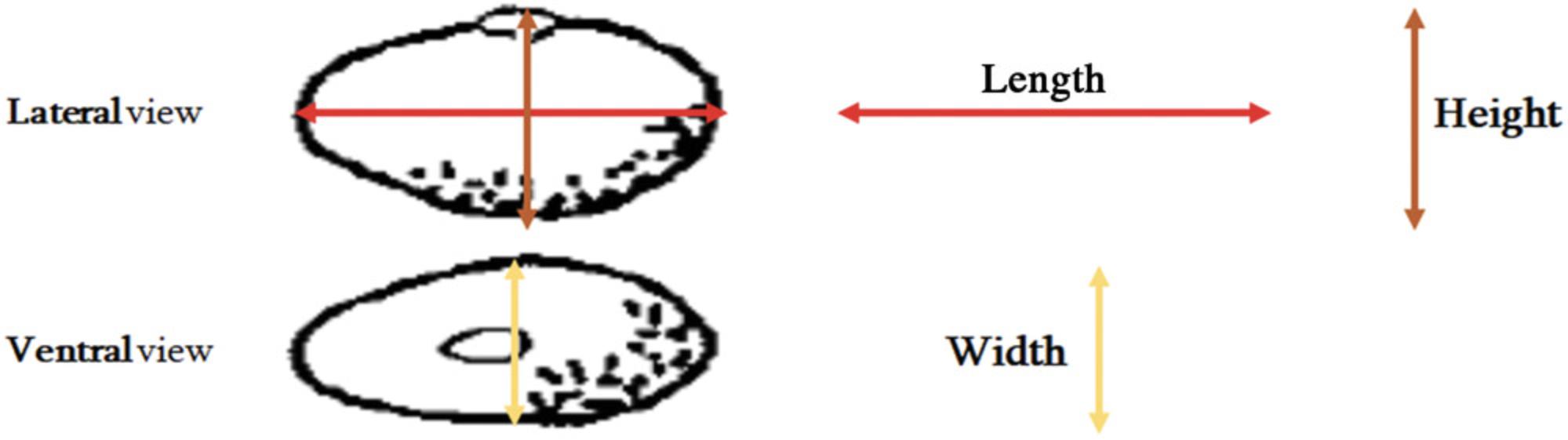
8.Take five seeds from each accession (heterogeneous materials) or line (already developed SSD lines), and using a ruler, measure the Seed Coat Height (mm) by performing a lateral measurement, measured from the hilum to the opposite side (Fig. 5). Use the mean of the values obtained from five seeds as the final measure.
9.Take five seeds from each accession (heterogeneous materials) or line (already developed SSD lines), and using a ruler, measure the Seed Coat Width (mm) by performing a ventral measurement (Fig. 5). Use the mean of the values obtained from five seeds as the final measure.
10.Take at least five seeds from each accession (heterogeneous materials) or line (already developed SSD lines), and through visual observation, evaluate and classify the Seed Shape according to one of the following five categories (Fig. 6):
- 1 = round
- 2 = oval
- 3 = cuboid
- 4 = kidney shaped
- 5 = truncate fastigiate.
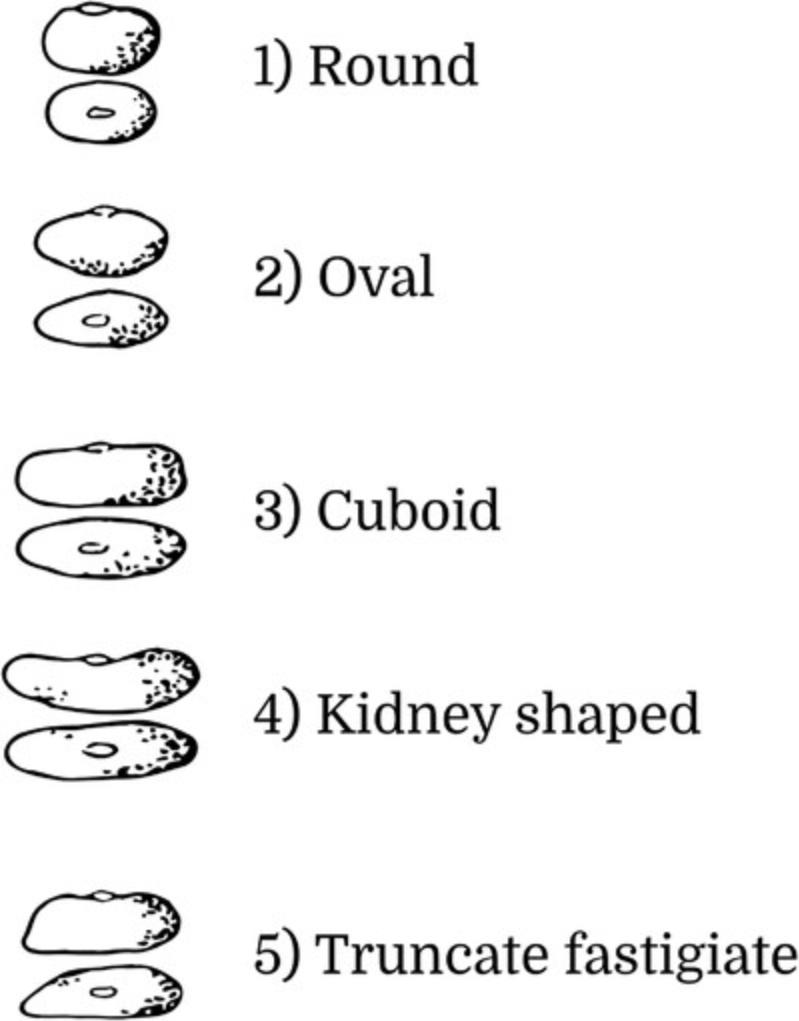
11.Measure the 100-Seed Weight, preferably by measuring the seed weight of at least two samples of 100 seeds (if not possible, then the seed weight should be measured on 10 seeds, as three different samples). Immature and/or infected seeds should be excluded.
Basic Protocol 2: BEAN SEED IMAGING
The aim of SEED bean imaging is to document the beans during their respective propagation steps in regard to the following traits:
1.Visual quality control of the propagation result; 2.Reference images for shape, color, and size; 3.Picture material for presenting the SSD line in various media; 4.Basis for automated image analysis.
A comprehensive discussion on the digitization of collections based on Nelson, Paul, Riccardi, and Mast (2012) is given in de la Hidalga, van Walsun, Rosin, Sun, and Wijers (2019). Accordingly, the following requirements should be fulfilled as the best-practice standard.
NOTE : Image capture should be done using a defined process under comparable conditions, and any institution and/or research group involved in seed increase of shared SSD lines should implement a standardized system to take images of seeds.
Important considerations for photography are listed below.
- To obtain good separation of the beans from the background, it is advisable to place them on a background of a color not present in skins of the beans. Light gray is ideal here for a neutral picture impression; if this is too close to the color of the beans, a black or white background can be used. The background should be flat and should not have any structural pattern.
- Include a color chart in the photographs for quality control and postprocessing. This allows the lighting, white balance, and color accuracy of the image to be verified.
- Include a scale bar to allow measurement of the dimensions of the objects.
Materials
- Uniform background (see above)
- Color chart: e.g., ColorGauge Micro Target (Image Science Associates) or ColorChecker Classic Mini or Nano (X-Rite)
- Scale bar
- Photo lighting studio made of a lighting box (40 × 40 cm) with brightness-adjustable LED lamps (allowing gradual adjustment of light level)
- Microscope camera of at least 20 megapixels and an appropriate macro lens mounted on a stable tripod above the box
- Image-editing software for post-processing images that allows image normalization and resizing, as well as the subsequent addition of text or QR codes to the image
Imaging process
1.Select at least five beans to be photographed. Selected bean seeds should be typical of the respective line in terms of color and size, although any variability should also be captured.
2.Place the bean seeds, color chart, and scale bar on the background. Arrange the beans so that they are separated from each other, and position one bean near the rule, at scale position zero of the scale. Be sure to leave enough space for the subsequent insertion of the label information and barcode.
3.Begin photography, processing only one bean line per pass to avoid mixing or confusion of the material. Before recording, verify the identity of the material.
4.Within the project space, the name of the image should be set to start with the unique IncreaseID followed by the sample ID or plant ID, and a unique image number separated by underscores, as:
5.Set the image resolution and size. The resolution and size of an image depends on the intended use. The higher the initial values, the more versatile the application is, as smaller versions can be easily created. The following values in Table 1 provide the guidelines for the resolution. The color bit depth must always be 24-bit color.
Expected use | Resolution (ppi) |
---|---|
Research and preservation | 600 |
Printing | 300 |
Web publishing | 72 |
6.Process the images (e.g., image enhancement, normalization, addition of label information, barcode). For the barcode, different variants are possible.
- a.
Two-dimensional QR Code: This is preferred because further information can be stored in addition to the identifier. The data record for the examples given here is structured as follows:
1. Full Image-ID: <IncreaseID>_<SampleID>_<ImageID>
2. Image of:
3. DOI: <DOI of the SSD line that is shown>
4. Project-ID: <IncreaseID>
5.Sample-ID: <SampleID>
6.Species: _P. vulgaris_ L.
7.Plant part: <Type of plant parts shown>
8.ITPGRFA Annex1 crop: Beans
9. Creation date: <yyyy-mm-dd> (e.g., 2020-10-26)
- b.
Line codes (Code 128): These should only be used for the internal identification of image documents. They are the simplest form of identifier and allow the coding of an identifier for the image. The recommended format is as follows:
7.Transfer the finalized images to a central repository for the project and reference them in a management system.
8.Integrate the image identification and description information as a label applied subsequent to the image processing. The human-readable information is limited to the identifiers of the depicted object, and the reference to the image rights license (here, the Creative Commons license CC BY-SA, https://creativecommons.org). Optionally, a logo can be included to boost brand recognizability.
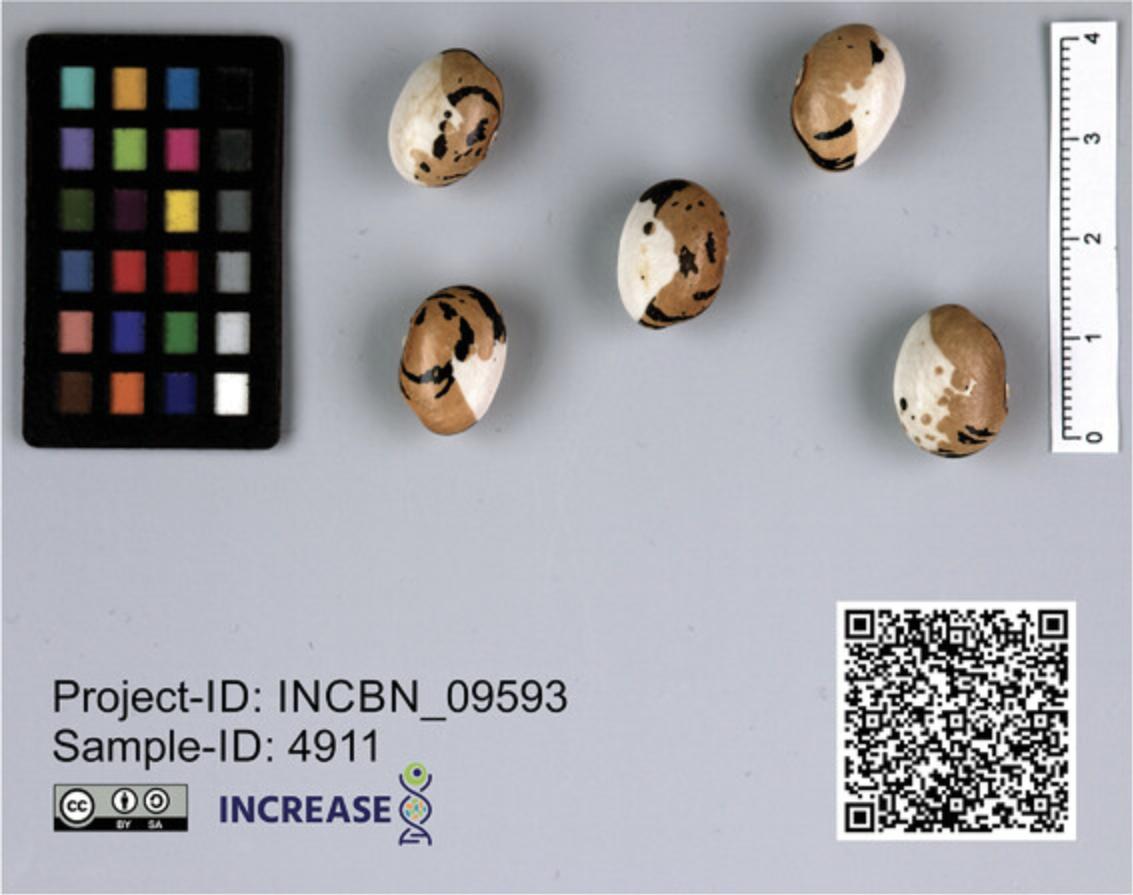
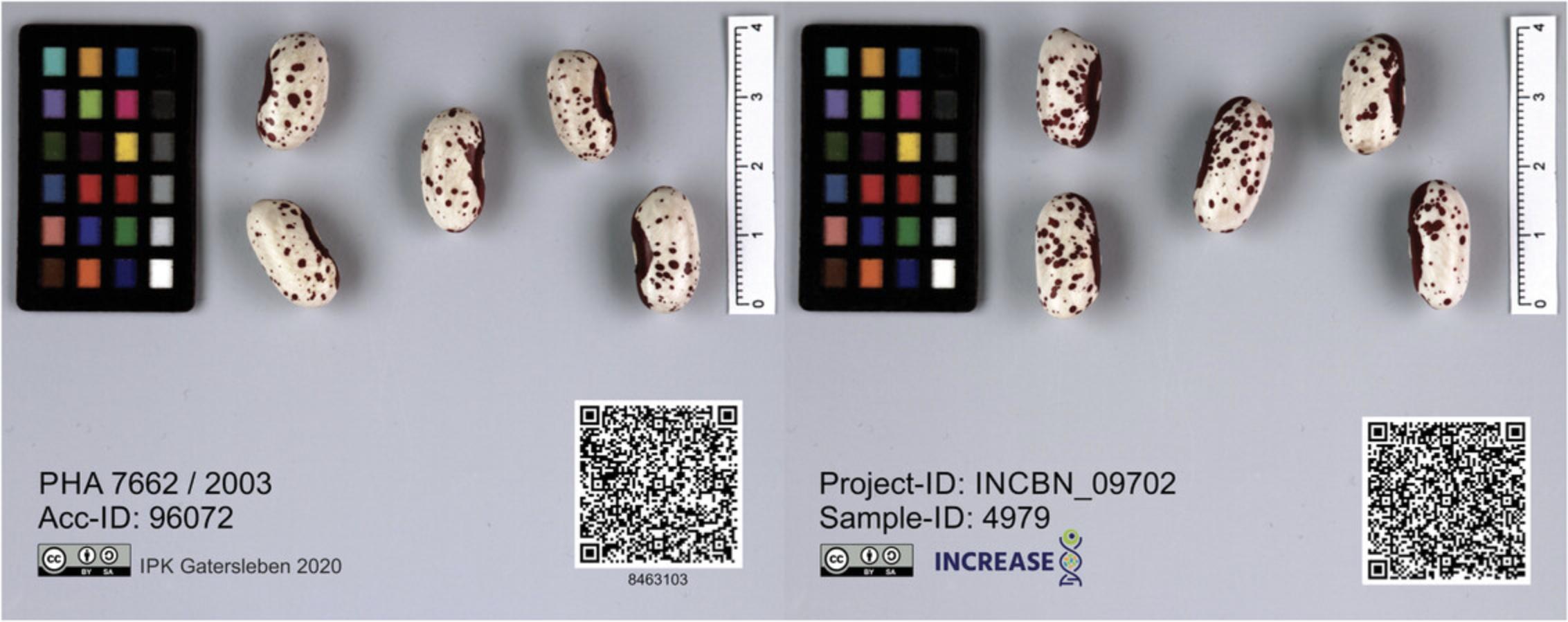
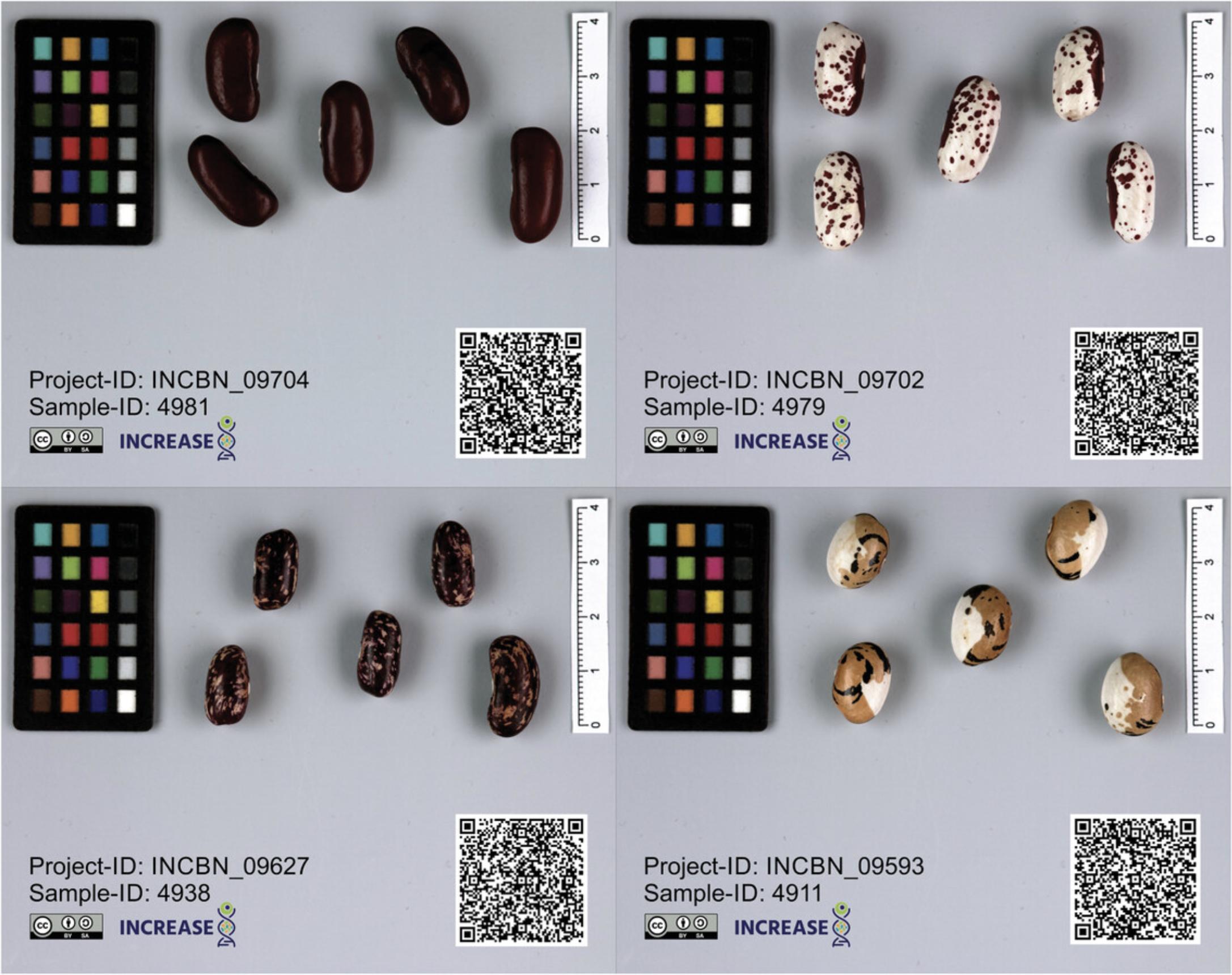
Basic Protocol 3: CHARACTERIZING BEAN LINES FOR PLANT TRAIT DESCRIPTORS SPECIFIC FOR COMMON BEAN PRIMARY SEED INCREASE
The main aim of this protocol is the phenotypic evaluation of the different lines grown in controlled conditions during the cycles of seed multiplication. These phenotypic data, along with those obtained by applying Basic Protocols 1 and 2, will be uploaded into the project database and integrated with those obtained for the same lines in other experiments (i.e., field trials). All of these data will represent precious information for future users, such as researchers, breeders, and so on.
Users should record the following Mandatory Traits (Priority 1, steps 1-27), which are considered as essential within INCREASE and must be recorded for each selfing cycle, along with Non-Mandatory Traits (Priority 2, steps 28-33). Non-Mandatory Traits are considered within INCREASE as non-essential, but preferable. The collection of Non-Mandatory Traits overlaps with the collection of Mandatory Traits, and thus the step numbers are not fully sequential in time.
NOTE : This protocol assumes that plants and seeds are evaluated by visual inspection and manual measurement.
NOTE : During Primary Seed Increase, one plant is grown and characterized for each line, for at least the first two selfing cycles (development of SSD seeds); in subsequent cycles it may be possible to grow more than one plant per line, depending on the available space in controlled conditions (see Fig. 3). If more than three plants are grown per line, traits can be recorded on a subsample (at least three randomly chosen plants).
NOTE : Before starting the protocol, collect the following information on the experimental site: data collector name, location of experimental trial, latitude of experimental trial, longitude of experimental trial, altitude of experimental trial, and controlled-condition/insect-free measures applied (e.g., tunnel, greenhouse, grow chamber).
Materials
- Seeds
- Ruler
- Analytical balance
- Spreadsheet software
Recording Mandatory Traits (Priority 1)
1.Record the Sowing Date (i.e., date on which the seeds were sown).
2.Record the Days to Emergence (i.e., number of days after which the seedlings emerged, starting from the sowing day).
3.Through visual observation, evaluate and classify the Hypocotyl Pigmentation (i.e., color of the hypocotyl) according to one of the following three categories:
1.1 = purple 2.2 = green 3.3 = other (specify in the Notes).
4.Through visual observation, evaluate and classify the Leaf Color (chlorophyll) according to one of the following three categories of green:
- 1 = pale green
- 2 = medium green
- 3 = dark green.
5.Through visual observation, evaluate the presence/absence of the Leaf Color (anthocyanin pigmentation, red-purplish or red color) according to one of the following categories:
- 0 = absent
- 1 = present.
6.Record the Days to Beginning of Flowering (i.e., number of days from sowing to the appearance of the first open flower). Record this based on the presence of one open flower at any node.
7.Through visual observation, evaluate and classify the Flower Color—i.e., the color of the standard for freshly opened flowers—according to the following 14 categories:
- 1 = white
- 2 = greenish
- 3 = pink 4.4 = light purple 5.5 = purple 6.6 = dark purple 7.7 = white with purple spots 8.8 = white with red veins 9.9 = white with green spots 10.10 = pink with green spots 11.11 = light purple with green spots 12.12 = red 13.13 = greenish with purple spots 14.14 = other (specify in the Notes).
It is important to evaluate a freshly opened flower, as flower colors are highly changeable after opening.
8.Through visual observation, evaluate and classify the Flower Color of Wings for freshly opened flowers according to the following nine categories:
- 1 = white
- 2 = greenish
- 3 = pink
- 4 = light purple
- 5 = purple
- 6 = dark purple
- 7 = white or light purple with dark purple edges
- 8 = white with red veins
- 9 = other (specify in the Notes).
Again, be sure to evaluate freshly opened flowers, as flower colors are highly changeable after opening.
9.Record the Days to Pod Formation (i.e., the number of days after planting until the plants have at least one visible pod).
10.Through visual observation, evaluate and classify the Pod Cross-Section from fully expanded immature pods according to one of the following five categories (Fig. 10):
- 1 = very flat
- 2 = pear shaped
- 3 = round elliptic
- 4 = figure of eight
- 5 = other (specify in the Notes).
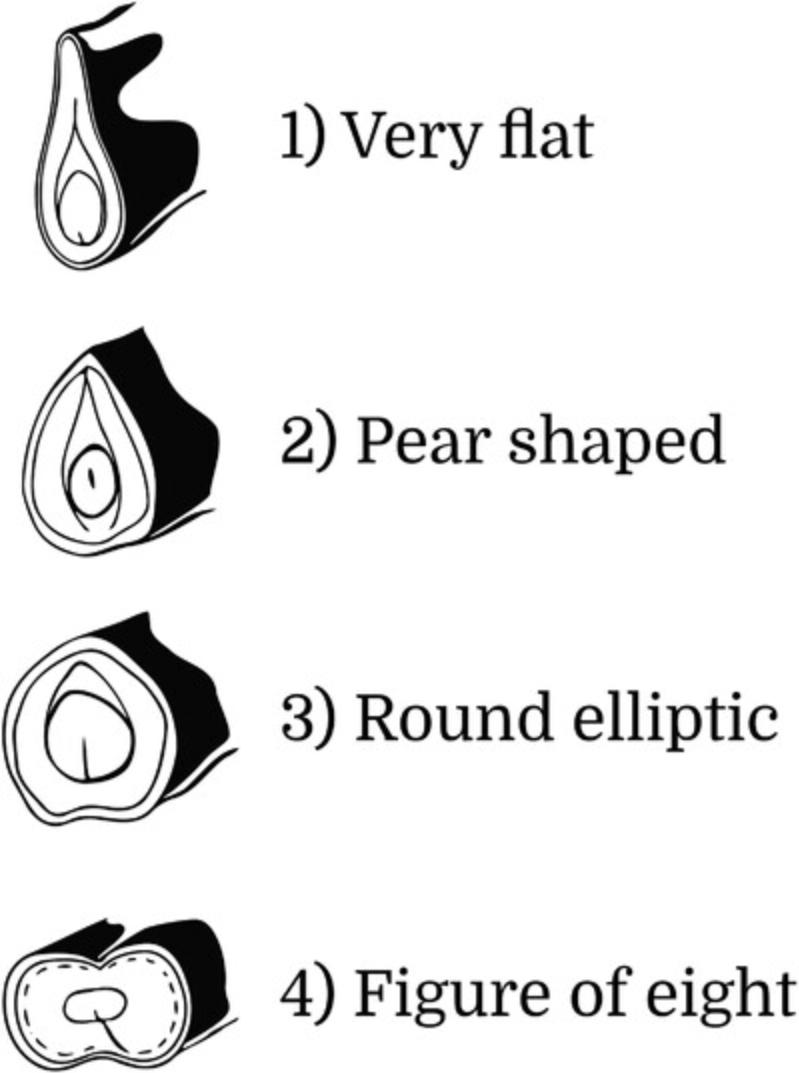
11.Through visual observation, evaluate and classify the Pod Curvature for fully expanded immature pods according to one of the following four categories (Fig. 11):
- 1 = straight
- 2 = slightly curved
- 3 = curved
- 4 = recurving.
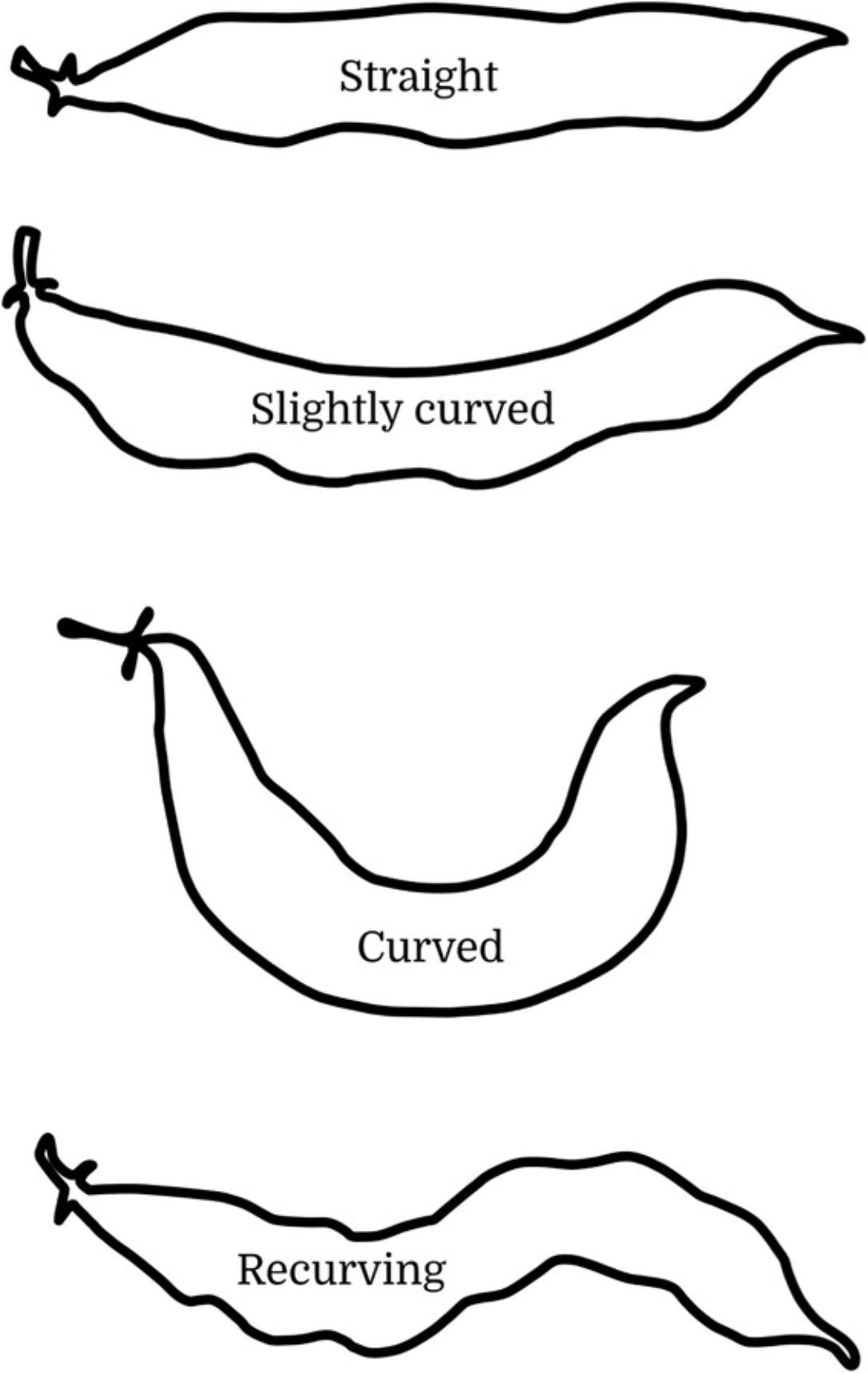
12.Through visual observation, evaluate and classify the Pod Color at Physiological Maturity (PM) according to one of the following seven categories (see Fig. 12 for an example of bean pods at physiological maturity):
- 1 = light yellow
- 2 = gold yellow/dark yellow
- 3 = light green/gray-green
- 4 = green/dark green
- 5 = red
- 6 = purple
- 7 = other (specify in the Notes).
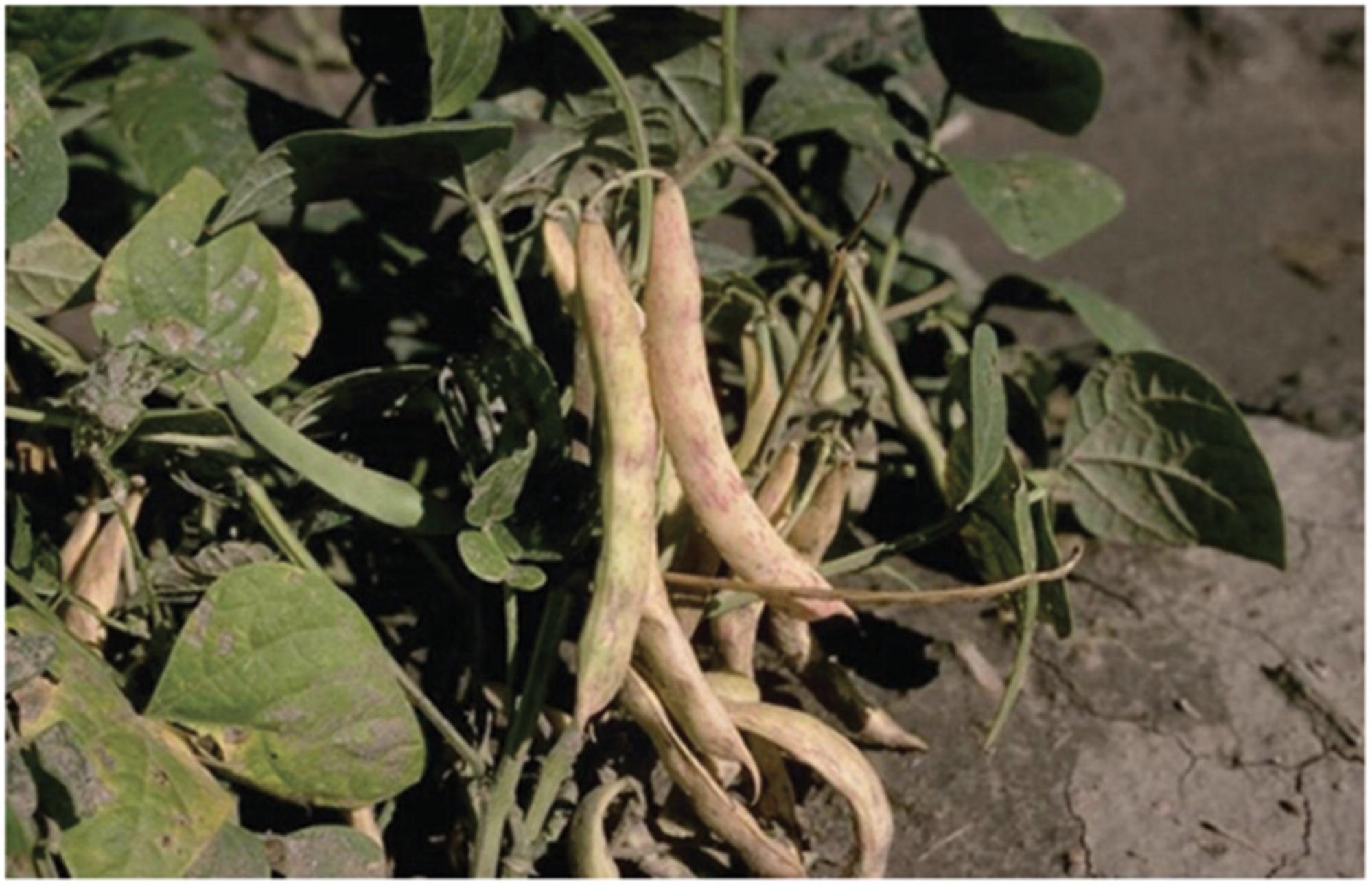
13.Through visual observation, evaluate and classify the Pattern of Pod Pigmentation at Physiological Maturity (PM) according to one of the following six categories:
- 0 = none
- 1 = speckled
- 2 = mottled
- 3 = striped
- 4 = covered, coated
- 5 = other (specify in the Notes).
14.Through visual observation, evaluate and classify the Pod Wall Fiber of the mature pods according to one of the following three categories (Fig. 13):
- 1 = strongly contracting (at dry maturity, adhering around seed)
- 2 = leathery podded (dry pods will not spontaneously open)
- 3 = excessive shattering (with strong twisting of dry pods).
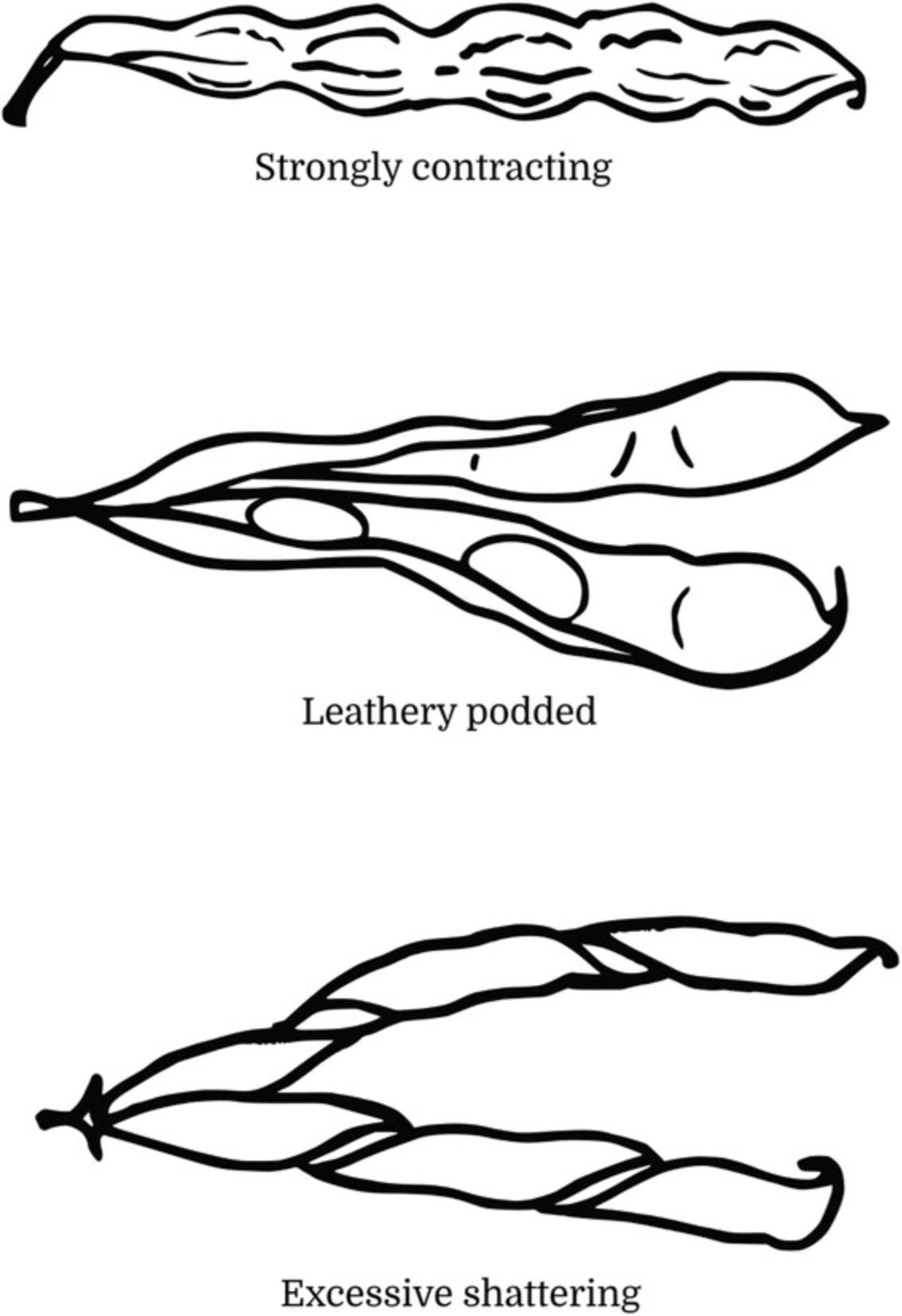
15.Record the Leaf Persistence at the time when 90% of the pods are dry according to one of the three categories:
- 1 = all leaves dropped
- 2 = intermediate
- 3 = all leaves persistent.
16.Record the Plant Determinacy (i.e., determinate/indeterminate character) during flowering as follow:
- 1 = determinate
- 2 = indeterminate.
17.Record the Plant Length as the distance (cm) from the soil surface to the top of the plant, at a time when the plants have at least one open flower.
18.Measure the Stem Diameter (cm) just above the soil surface at plant maturity.
19.Record the time of Full Maturity (i.e., the number of days after planting after which 90% of the pods on the plant are golden-brown).
20.Record the Days to Harvest (i.e., the number of days from planting to harvest).
21.Measure the Pod Length (cm) at dry harvest maturity.
22.Measure the Pod Width (cm).
23.Record the Total Seed Mass (g) of all of the seeds harvested individually from each plant.
24.Record the Total Number of Seeds (count) harvested individually from each plant.
25.Count and record the 100-Seed Mass (g).
26.Record Diseases, and if possible, describe or make notes regarding disease status.
- 0 = no disease present
- 1 = disease present
- 2 = unsure.
There is a “Disease-specific comments” column for making any notes related to diseases, including but not limited to the observation that many or specific diseases are present.
27.Record the Stress Susceptibility, and if possible, describe or make notes about this.
- 0 = no stress present
- 1 = stress present
- 2 = unsure.
There is a “Stress-specific comments” column for making any notes related to stress.
Recording Non-Mandatory Traits (Priority 2)
28.Through visual observation, evaluate and classify the Emerging Cotyledon Color according to the following six categories:
- 1 = purple
- 2 = red
- 3 = green
- 4 = very pale green
- 5 = white
- 6 = other (specify in the Notes).
29.Record the Leaf Shape of the terminal leaflet of the third trifoliate leaf according to the following three categories (Fig. 14):
- 1 = triangular
- 2 = quadrangular
- 3 = round.
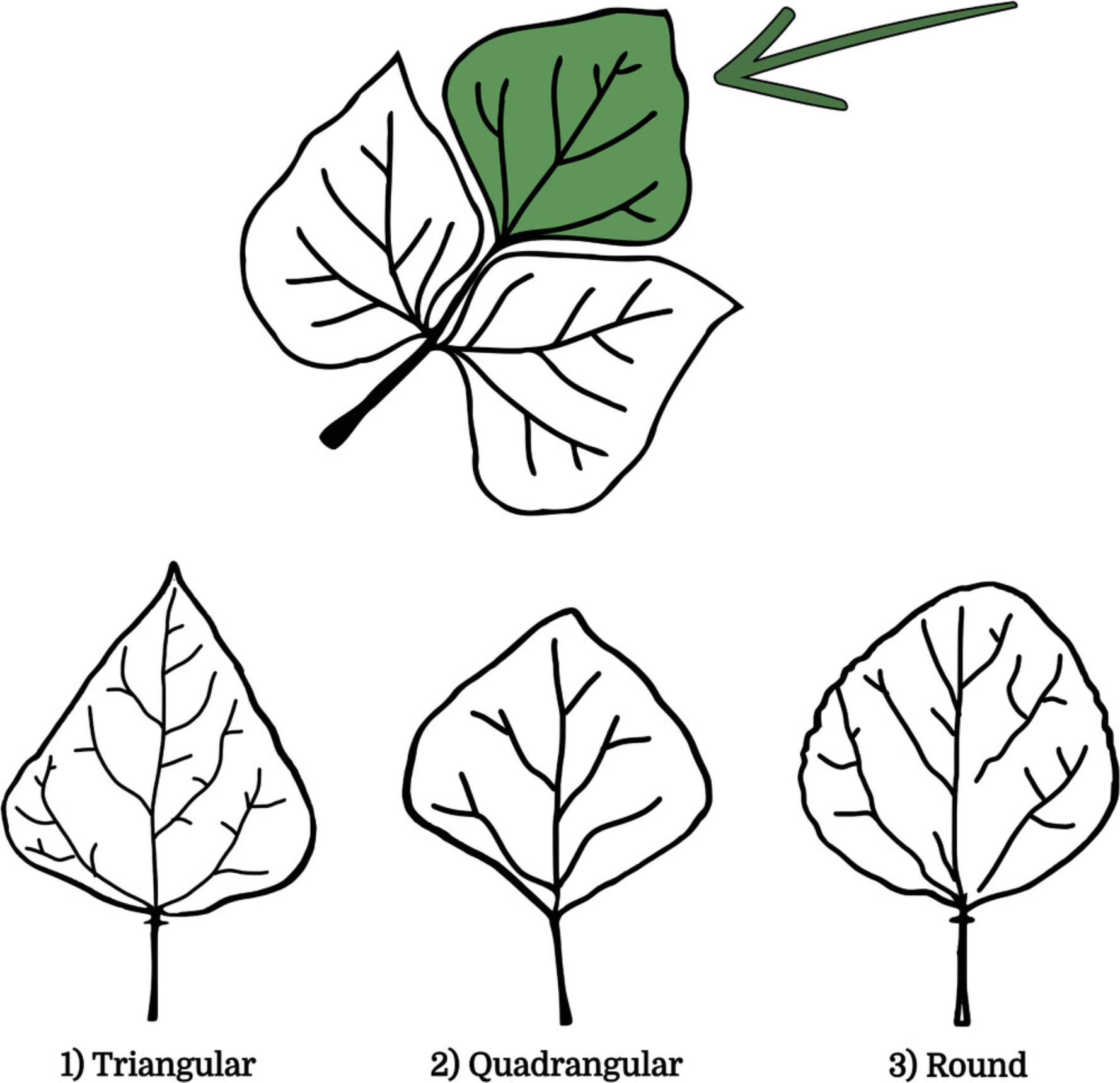
30.Record the Pod Color for fully expanded immature pods according to the following seven categories:
- 1 = light yellow
- 2 = golden yellow/dark yellow
- 3 = green/dark green
- 4 = light green/gray-green
- 5 = red
- 6 = purple
- 7 = other (specify in the Notes).
31.Record the Pod Length (cm) of the largest fully expanded immature pod, from three randomly chosen pods.
32.Record the Pod Suture String status on fully expanded immature pods from three randomly chosen pods, but only if the seed multiplication will be not compromised. Classify into one of the following four categories:
- 0 = stringless
- 1 = few strings
- 2 = moderately stringy
- 3 = very stringy.
33.Through visual observation, evaluate and classify, if present, the Stress Susceptibility according to the following three categories:
- 1 = low susceptibility
- 2 = medium susceptibility
- 3 = high susceptibility.
34.If a stress is present, record possible source of damage caused to aerial plant parts.
- Low temperature
- High temperature
- Drought
- High soil moisture
- Salinity
- Soil acidity
- Pests:
- ○
White fly
* ○
Thrips
* ○
Aphid
* ○
Red spider
* ○
Weevil
* ○
Other
- Fungi:
- ○
White mold
* ○
Anthracnose
* ○
Root rot
* ○
Powdery mildew
* ○
Ascochyta
* ○
Other
- Bacteria and viruses:
- ○
Bean common mosaic virus
* ○
Bean common necrotic mosaic virus
* ○
Halo blight.
COMMENTARY
Background Information
Here, we report an established system (i.e., phenotyping procedures and protocols) for the development of SSD lines starting from common bean genetic resources, for their maintenance and for the production of seeds for distribution. This system is based on a set of phenotyping protocols that can be applied by gene banks and research institutes that also want to use such resources. The strength of this system is that it is designed to produce both the genomic data associated with each pure line and an “open” platform for integration of data produced within INCREASE. This will also include data that are produced after the conclusion of INCREASE for the same materials. The idea is to set up a centralized system that can be used by gene banks and research institutes that can also integrate new data, and at the same time, be freely accessible by any user.
Acknowledgments
This study was conducted as part of the INCREASE project, funded from the European Union Horizon 2020 Research and Innovation program under grant agreement no. 862862.
Author Contributions
Gaia Cortinovis : Writing-original draft, writing-review and editing. Markus Oppermann : Validation, writing-original draft, writing-review and editing. Kerstin Neumann : Validation, writing-original draft, writing-review and editing. Andreas Graner : Validation, writing-original draft, writing-review and editing. Tania Gioia : Validation, writing-original draft, writing–review and editing. Marco Marsella : Validation, writing-original draft, writing-review and editing. Saleh Alseekh : Validation, writing-original draft, writing-review and editing. Alisdair R. Fernie : Validation, writing-original draft, writing-review and editing. Roberto Papa : Validation, writing-original draft, writing-review and editing. Elisa Bellucci : Supervision, writing-original draft, writing-review and editing. Elena Bitocchi : Supervision, writing-original draft, writing-review and editing.
Conflict of Interest
The authors declare no conflict of interest.
Open Research
Data Availability Statement
Data sharing not applicable—no new data generated.
Literature Cited
- Almeida, C. P., Paulino, J. F. C., Morais Carbonell, S. A., Chiorato, A. F., Song, Q., Di Vittori, V., … Benchimol-Reis, L. L. (2020). Genetic diversity, population structure, and Andean introgression in Brazilian common bean cultivars after half a century of genetic breeding. Genes , 11, 1298. doi: 10.3390/genes11111298.
- Angioi, S. A., Rau, D., Attene, G., Nanni, L., Bellucci, E., Logozzo, G., … Papa, R. (2010). Beans in Europe: Origin and structure of the European landraces of Phaseolus vulgaris L. Theoretical and Applied Genetics , 121, 829–843. doi: 10.1007/s00122-010-1353-2.
- Ariani, A., & Gepts, P. (2019). Signatures of environmental adaptation during range expansion of wild common bean (Phaseolus vulgaris) [Preprint]. BioRxiv , 571042. Retrieved from https://www.biorxiv.org/content/10.1101/571042v1. doi: 10.1101/571042.
- Ariani, A., Mier y Teran, J. C., & Gepts, P. (2017). Spatial and temporal scales of range expansion in wild Phaseolus vulgaris. Molecular Biology and Evolution , 35, 119–131. doi: 10.1093/molbev/msx273.
- Azani, N., Babineau, M., Bailey, C. D., Banks, H., Barbosa, A. R., Pinto, R. B., … Zimmerman, E. (2017). A new subfamily classification of the Leguminosae based on a taxonomically comprehensive phylogeny. The Legume Phylogeny Working Group (LPWG). Taxon , 66(1), 44–77.
- Bellucci, E., Aguilar, M., Alseekh, S., Bett, K., Brezeanu, C., Cook, D., … Papa, R. (submitted). The INCREASE project: Intelligent collections of food-legume genetic resources for European agrofood systems. Plant Journal , submitted.
- Bellucci, E., Bitocchi, E., Ferrarini, A., Benazzo, A., Biagetti, E., Klie, S., … Papa, R. (2014). Decreased nucleotide and expression diversity and modified co-expression patterns characterize domestication in the common bean. The Plant Cell , 26, 1901–1912. doi: 10.1105/tpc.114.124040.
- Bellucci, E., Bitocchi, E., Rau, D., Nanni, L., Ferradini, N., Giardini, A., … Papa, R. (2013). Population structure of barley landrace populations and gene-flow with modern varieties. PLoS One , 8(12), e83891. doi: 10.1371/journal.pone.0083891.
- Bellucci, E., Bitocchi, E., Rau, D., Rodriguez, M., Biagetti, E., Giardini, A., … Papa, R. (2014). Genomics of origin, domestication and evolution of Phaseolus vulgaris. In R. Tuberosa, A. Graner, & E. Frison (Eds.), Genomics of plant genetic resources (pp. 483–507). Berlin, Germany: Springer.
- Bioversity International. (2011). Crop ontology curation and annotation tool. Generation Challenge Programme, Bioversity International (Rome) as project-implementing agency. Retrieved from https://www.cropontology.org/.
- Bitocchi, E., Nanni, L., Bellucci, E., Rossi, M., Giardini, A., Spagnoletti Zeuli, P., … Papa, R. (2012). Mesoamerican origin of the common bean (Phaseolus vulgaris L.) is revealed by sequence data. Proceedings of the National Academy of Sciences of the United States of America , 109, E788–E796. doi: 10.1073/pnas.1108973109.
- Bitocchi, E., Nanni, L., Rossi, M., Rau, D., Bellucci, E., Giardini, A., … Papa, R. (2009). Introgression from modern hybrid varieties into landrace populations of maize (Zea mays ssp. mays L.) in central Italy. Molecular Ecology , 18, 603–621. doi: 10.1111/j.1365-294X.2008.04064.x.
- Bitocchi, E., Bellucci, E., Rau, D., Albertini, E., Rodriguez, M., Veronesi, F., … Nanni, L. (2015). European flint landraces grown in situ reveal adaptive introgression from modern maize. PLoS ONE , 10(4), e0121381. doi: 10.1371/journal.pone.0121381.
- Bitocchi, E., Rau, D., Bellucci, E., Rodriguez, M., Murgia, M. L., Gioia, T., … Papa, R. (2017). Beans (Phaseolus ssp.) as a model for understanding crop evolution. Frontiers in Plant Science , 8, 722. doi: 10.3389/fpls.2017.00722.
- Bitocchi, E., Rau, D., Benazzo, A., Bellucci, E., Goretti, D., Biagetti, E., … Papa, R. (2017). High level of nonsynonymous changes in common bean suggests that selection under domestication increased functional diversity at target traits. Frontiers in Plant Science , 7, 2005. doi: 10.3389/fpls.2016.02005.
- Broughton, W. J., Hernández, G., Blair, M., Beebe, S., Gepts, P., & Vanderleyden, J. (2003). Beans (Phaseolus spp.) — Model food legumes. Plant and Soil , 252, 55–128. doi: 10.1023/A:1024146710611.
- Cichy, K. A., Porch, T., Beaver, J. S., Cregan, P., Fourie, D., Glahn, R. P., … Miklas, P. N. (2015). A Phaseolus vulgaris diversity panel for Andean bean improvement. Crop Science , 55, 2149–2160. doi: 10.2135/cropsci2014.09.0653.
- Cichy, K. A., Wiesinger, J. A., & Mendoza, F. A. (2015). Genetic diversity and genome-wide association analysis of cooking time in dry bean (Phaseolus vulgaris L.). Theoretical and Applied Genetics , 128, 1555–1567. doi: 10.1007/s00122-015-2531-z.
- Cortinovis, G., Di Vittori, V., Bellucci, E., Bitocchi, E., & Papa, R. (2020). Adaptation to novel environments during crop diversification. Current Opinion in Plant Biology , 13, 1–15. doi: 10.1016/j.pbi.2019.12.011.
- Cortinovis, G., Frascarelli, G., Di Vittori, V., & Papa, R. (2020). Current state and perspectives in population genomics of the common bean. Plants , 9, 330. doi: 10.3390/plants9030330.
- de la Hidalga, N. A., van Walsun, M., Rosin, P., Sun, X., & Wijers, A. (2019). Quality management methodologies for digitisation operations. Retrieved from https://zenodo.org/record/3469521.
- Desiderio, F., Bitocchi, E., Bellucci, E., Rau, D., Rodriguez, M., Attene, G., … Nanni, L. (2013). Chloroplast microsatellite diversity in Phaseolus vulgaris. Frontiers in Plant Science , 3, 312. doi: 10.3389/fpls.2012.00312.
- Di Vittori, V., Bitocchi, E., Rodriguez, M., Alseekh, S., Bellucci, E., Nanni, L., … Papa, R. (2020). Pod indehiscence in common bean is associated to the fine regulation of PvMYB26 and a non-functional abscission layer. Journal of Experimental Botany , 72(5), 1617–1633. doi: 10.1093/jxb/eraa553.
- Diamond, J. (2002). Evolution, consequences and future of plant and animal domestication. Nature , 418, 700–707. doi: 10.1038/nature01019.
- Dwivedi, S. L., Salvatore, C., Blair, M. W., Upadhyaya, H. D., Are, A. K., & Ortiz, R. (2016). Landrace germplasm for improving yield and abiotic stress adaptation. Trends Plant Science , 21, 31–41. doi: 10.1016/j.tplants.2015.10.012.
- Elshire, R. J., Glaubitz, J. C., Sun, Q., Poland, J. A., Kawamoto, K., Buckler, E. S., & Mitchell, S. E. (2011). A robust, simple genotyping-by-sequencing (GBS) approach for high diversity species. PLoS One , 6(5), e19379. doi: 10.1371/journal.pone.0019379.
- Gepts, P. (2010). Crop domestication as a long-term selection experiment. Plant Breeding Reviews , 24, 1–44.
- Gioia, T., Logozzo, G., Attene, G., Bellucci, E., Benedettelli, S., & Negri, V. (2013). Evidence for introduction bottleneck and extensive inter-gene pool (Mesoamerica × Andes) hybridization in the European common bean (Phaseolus vulgaris L.) germplasm. PLoS One , 8, e75974. doi: 10.1371/journal.pone.0075974.
- Glémin, S., & Bataillon, T. (2009). A comparative view of the evolution of grasses under domestication. The New Phytologist , 183, 273–290. doi: 10.1111/j.1469-8137.2009.02884.x.
- González, M. Y., Philipp, N., Schulthess, A. W., Weise, S., Zhao, Y., Börner, A., … Reif, J. C. (2018). Unlocking historical phenotypic data from an ex situ collection to enhance the informed utilization of genetic resources of barley (Hordeum sp.). Theoretical and Applied Genetics , 131, 2009–2019. doi: 10.1007/s00122-018-3129-z.
- Graham, P. H., & Vance, C. P. (2003). Legumes: Importance and constraints to greater use. Plant Physiology , 131, 872–877. doi: 10.1104/pp.017004.
- Guerra-García, A., Gioia, T., von Wettberg, E., Logozzo, G., & Bett, K. E. Increasing lentil genetic resources: Evolutionary history, recent genomic characterization of germplasm, and the need for well characterized collections. Current Protocols in Plant Biology , in preparation.
- Harlan, J., & de Wet, J. (1971). Towards a rational classification of cultivated plants. Taxon , 20, 509–517. doi: 10.2307/1218252.
- International Board for Plant Genetic Resources (IPGRI). (1982). Phaseolus vulgaris descriptors. Rome, Italy: International Board for Plant Genetic Resources.
- Kamfwa, K., Cichy, K. A., & Kelly, J. D. (2015a). Genome-wide association analysis of symbiotic nitrogen fixation in common bean. Theoretical and Applied Genetics , 128, 1999–2017. doi: 10.1007/s00122-015-2562-5.
- Kamfwa, K., Cichy, K. A., & Kelly, J. D. (2015b). Genome-wide association study of agronomic traits in common bean. Plant Genome , 8, 1–12. doi: 10.3835/plantgenome2014.09.0059.
- Kleintop, A. E., Myers, J. R., Echeverria, D., Thompson, H. J., & Brick, M. A. (2016). Total phenolic content and associated phenotypic traits in a diverse collection of snap bean cultivars. Journal of the American Society for Horticultural Science , 141(1), 3–11. doi: 10.21273/JASHS.141.1.3.
- Kroc, M. et al. (submitted). Lupin INCREASE Intelligent Collections: Characterization and development of single seed descent genetic resources. Current Protocols , submitted.
- Kumar, S. et al. (submitted). Chickpea INCREASE Intelligent Collections: Characterization and development of single seed descent genetic resources. Current Protocols , in preparation.
- Lewis, G. P., Schrire, B. D., Mackinder, B. A., Rico, L., & Clark, R. (2013). A linear sequence of legume genera set in a phylogenetic context: A tool for collections management and taxon sampling. South African Journal of Botany , 89, 76–84. doi: 10.1016/j.sajb.2013.06.005.
- Luikart, G., Kardos, M., Hand, B. K., Rajora, O. P., Aitken, S. N., & Hohenlohe, P. A. (2018). Population genomics: Advancing understanding of nature. In O. P. Rajora (Ed.), Population genomics: Concepts, approaches and applications (pp. 3–79). Cham, Switzerland: Springer International Publishing.
- McClean, P. E., Bett, K. E., Stonehouse, R., Lee, R., Pflieger, S., Moghaddam, S. M., … Mamidi, S. (2018). White seed colour in common bean (Phaseolus vulgaris) results from convergent evolution in the P (pigment) gene. New Phytologist , 219, 1112–1123. doi: 10.1111/nph.15259.
- McClean, P. E., Moghaddam, S. M., Lopéz-Millán, A. F., Brick, M. A., Kelly, J. D., Miklas, P. N., … Grusak, M. A. (2017). Phenotypic diversity for seed mineral concentration in North American dry bean germplasm of MA ancestry. Crop Science , 57, 3129–3144. doi: 10.2135/cropsci2017.04.0244.
- McCouch, S., Navabi, K., Abberton, M., Anglin, N. L., Barbieri, R. L., Baum, M., … Rieseberg, L. H. (2020). Mobilizing crop biodiversity. Molecular Plant , 13, 1341–1344. doi: 10.1016/j.molp.2020.08.011.
- Milner, S. G., Jost, M., Taketa, S., Mazon, E. R., Himmelbach, A., Oppermann, M., … Stein, N. (2019). Genebank genomics highlights the diversity of a global barley collection. Nature Genetics , 51, 319–326. doi: 10.1038/s41588-018-0266-x.
- Mir, R. A., Sharma, A., & Mahajan, R. (2020). Crop landraces: Present threats and opportunities for conservation. In R. Salgotra & S. Zargar (Eds.), Rediscovery of genetic and genomic resources for future food security (pp. 335–350). Singapore: Springer.
- Moghaddam, S. M., Brick, M. A., Echeverria, D., Thompson, H. J., Brich, L. A., Lee, R., … McClean, P. E. (2017). The genetic architecture of dietary fiber and oligosaccharide content in a MA panel of edible dry bean (Phaseolus vulgaris L.). Plant Genome , 11, 1. doi: 10.3835/plantgenome2017.08.0074.
- Moghaddam, S. M., Mamidi, S., Osorno, J. M., Lee, R., Brick, M., Kelly, J., … McClean, P. E. (2016). Genome-wide association study identifies candidate loci underlying agronomic traits in a Middle American diversity panel of common bean. Plant Genome , 9, 1–21. doi: 10.3835/plantgenome2016.02.0012.
- Mousavi-Derazmahalleh, M., Bayer, P. E., Hane, J. K., Valliyodan, B., Nguyen, H. T., Nelson, M. N., … Edwards, D. (2019). Adapting legume crops to climate change using genomic approaches. Plant, Cell & Environment, 42, 6–19. doi: 10.1111/pce.13203.
- Murgia, M. L., Attene, G., Rodriguez, M., Bitocchi, E., Bellucci, E., Fois, D., … Rau, D. (2017). A comprehensive phenotypic investigation of the ‘pod-shattering syndrome’ in common bean. Frontiers in Plant Science , 8, 251. doi: 10.3389/fpls.2017.00251.
- Myers, J. R., Wallace, L. T., Moghaddam, S. M., Kleintop, A. E., Echeverria, D., Thompson, H. J., … McClean, P. E. (2019). Improving the health benefits of snap bean: Genome-wide association studies of total phenolic content. Nutrients , 11, 2509. doi: 10.3390/nu11102509.
- Nelson, G., Paul, D., Riccardi, G., & Mast, A. (2012). Five task clusters that enable efficient and effective digitization of biological collections. ZooKeys , 209, 19–45. doi: 10.3897/zookeys.209.3135.
- Oladzad, A., Porch, T., Rosas, J. C., Moghaddam, S. M., Beaver, J., Beebe, S. E., … McClean, P. E. (2019). Single and multi-trait GWAS identify genetic factors associated with production traits in common bean under abiotic stress environments. G3: Genes, Genomes, Genetics , 9(6), 1881–1892. doi: 10.1534/g3.119.400072.
- Oladzad, A., Zitnick-Anderson, K., Jain, S., Simons, K., Osorno, J. M., McClean, P. E., & Pasche, J. S. (2019). Genotypes and genomic regions associated with Rhizoctonia solani resistance in common bean. Frontiers in Plant Science , 10, 956. doi: 10.3389/fpls.2019.00956.
- Parker, T. A., Berny Mier, Y., Teran, J. C., Palkovic, A., Jernstedt, J., & Gepts, P. (2020). Pod indehiscence is a domestication and aridity resilience trait in common bean. New Phytologist , 225, 558–570. doi: 10.1111/nph.16164.
- Rau, D., Murgia, M. L., Rodriguez, M., Bitocchi, E., Bellucci, E., Fois, D., … Papa, R. (2019). Genomic dissection of pod shattering in common bean: Mutations at non-orthologous loci at the basis of convergent phenotypic evolution under domestication of leguminous species. The Plant Journal , 97, 693–714. doi: 10.1111/tpj.14155.
- Rendón-Anaya, M., Montero-Vargas, J. M., Saburido-Álvarez, S., Vlasova, A., Capella-Gutierrez, S., Ordaz-Ortiz, J. J., … Herrera-Estrella, A. (2017). Genomic history of the origin and domestication of common bean unveils its closest sister species. Genome Biology , 18, 60. doi: 10.1186/s13059-017-1190-6.
- Rodriguez, M., Rau, D., Bitocchi, E., Bellucci, E., Biagetti, E., Carboni, A., … Attene, G. (2016). Landscape genetics, adaptive diversity, and population structure in Phaseolus vulgaris. New Phytologist , 209, 1781–1794. doi: 10.1111/nph.13713.
- Schmutz, J., McClean, P. E., Mamidi, S., Wu, G. A., Cannon, S. B., Grimwood, J., … Jackson, S. A. (2014). A reference genome for common bean and genome-wide analysis of dual domestications. Nature Genetics , 46, 707–713. doi: 10.1038/ng.3008.
- Schröder, S., Mamidi, S., Lee, R., McKain, M. R., McClean, P. E., & Osorno, J. M. (2016). Optimization of genotyping by sequencing (GBS) data in common bean (Phaseolus vulgaris L.). Molecular Breeding , 36, 6. doi: 10.1007/s11032-015-0431-1.
- Singh, N., Wu, S., Raupp, W. J., Sehgal, S., Arora, A., Tiwari, V., … Poland, J. (2019). Efficient curation of genebanks using next generation sequencing reveals substantial duplication of germplasm accessions. Scientific Reports , 9, 650. doi: 10.1038/s41598-018-37269-0.
- Soltani, A., Moghaddam, S. M., Walter, K., Restrepo-Montoya, D., Mamidi, S., Schroder, S., … Osorno, J. M. (2017). Genetic architecture of flooding tolerance in the dry bean Middle-American diversity panel. Frontiers in Plant Science , 8, 1183. doi: 10.3389/fpls.2017.01.
- Song, Q., Jia, G., Hyten, D. L., Jenkins, J., Hwang, E. Y., Schroeder, S. G., … Cregan, P. B. (2015). SNP assay development for linkage map construction, anchoring whole-genome sequence, and other genetic and genomic applications in common bean. G3: Genes, Genomes, Genetics , 5, 2285–2290. doi: 10.1534/g3.115.020594.
- Tock, A. J., Fourie, D., Walley, P. G., Holub, E. B., Soler, A., Cichy, K. A., … Miklas, P. N. (2017). Genome-wide linkage and association mapping of halo blight resistance in common bean to race 6 of the globally important bacterial pathogen. Frontiers in Plant Science , 8, 1170. doi: 10.3389/fpls.2017.01170.
- Toro, O., Tohme, J., & Debouck, D. G. (1990). Wild bean (Phaseolus vulgaris L.): Description and distribution. In Centro Internacional de Agricultura Tropical (CIAT) Cali, Colombia: International Board for Plant Genetic Resources (IBPGR).
- Vavilov, N. (1920). The law of homologous series in variation. Lecture at the 3rd All-Russian Breeding Conference, Saratov.
- Vavilov, N. (1922). The law of homologous series in variation. Journal of Genetics , 12, 47–89. doi: 10.1007/BF02983073.
- Vlasova, A., Capella-Gutiérrez, S., Rendón-Anaya, M., Hernández-Oñate, M., Minoche, A. E., Erb, I., … Guigó, R. (2016). Genome and transcriptome analysis of the Mesoamerican common bean and the role of gene duplications in establishing tissue and temporal specialization of genes. Genome Biology , 17, 32. doi: 10.1186/s13059-016-0883-6.
- Wallace, L., Arkwazee, H., Vining, K., & Myers, J. R. (2018). Genetic diversity within snap beans and their relation to dry beans. Genes , 9(12), 587. doi: 10.3390/genes9120587.
- Wu, J., Wang, L., Fu, J., Chen, J., Wei, S., Zhang, S., … Wang, S. (2020). Resequencing of 683 common bean genotypes identifies yield component trait associations across a north–south cline. Nature Genetics , 52, 118–125. doi: 10.1038/s41588-019-0546-0.
- Zeven, A. C. (1998). Landraces: A review of definitions and classifications. Euphytica , 104, 127–139.
- Zhu, Y., Chen, H., Fan, J., Wang, Y., Li, Y., Chen, J., … Mundt, C. C. (2000). Genetic diversity and disease control in rice. Nature , 406, 718–722.
- Zuiderveen, G. H., Padder, B. A., Kamfwa, K., Song, Q., & Kelly, J. D. (2016). Genome-wide association study of anthracnose resistance in Andean beans (Phaseolus vulgaris). PLoS One , 11, e0156391. doi: 10.1371/journal.pone.0156391.
Internet Resources
Global Information System of the International Treaty on Plant Genetic Resources for Food and Agriculture. This service assigns Digital Object Identifiers (dois) to Plant Genetic Resources for Food and Agriculture (PGRFA) for reference in third-party systems and the scientific literature.
Online platform hosting information about Plant Genetic Resources for Food and Agriculture (PGRFA) conserved in gene banks worldwide.
Plant Comparative Genomics portal of the U.S. Department of Energy's Joint Genome Institute.
INCREASE Project website.
The Creative Commons license CC BY-SA.
Crop ontology curation and annotation tool.
X-Rite, Inc. (2020) ColorChecker Classic Mini (retrieved 26 October 2020).
Citing Literature
Number of times cited according to CrossRef: 8
- Vijay Rani Rajpal, Apekshita Singh, Renu Kathpalia, Rakesh Kr. Thakur, Mohd. Kamran Khan, Anamika Pandey, Mehmet Hamurcu, Soom Nath Raina, The Prospects of gene introgression from crop wild relatives into cultivated lentil for climate change mitigation, Frontiers in Plant Science, 10.3389/fpls.2023.1127239, 14 , (2023).
- Mustafa Bulut, Regina Wendenburg, Elena Bitocchi, Elisa Bellucci, Magdalena Kroc, Tania Gioia, Karolina Susek, Roberto Papa, Alisdair R. Fernie, Saleh Alseekh, A comprehensive metabolomics and lipidomics atlas for the legumes common bean, chickpea, lentil and lupin, The Plant Journal, 10.1111/tpj.16329, 116 , 4, (1152-1171), (2023).
- Lorenzo Rocchetti, Tania Gioia, Giuseppina Logozzo, Creola Brezeanu, Luis Guasch Pereira, Lucía De Rosa, Stefania Marzario, Alice Pieri, Alisdair R. Fernie, Saleh Alseekh, Karolina Susek, Douglas R. Cook, Rajeev K. Varshney, Shiv Kumar Agrawal, Aladdin Hamwieh, Elena Bitocchi, Roberto Papa, Towards the Development, Maintenance and Standardized Phenotypic Characterization of Single‐Seed‐Descent Genetic Resources for Chickpea, Current Protocols, 10.1002/cpz1.371, 2 , 2, (2022).
- Travis A. Parker, Jorge Acosta Gallegos, James Beaver, Mark Brick, Judith K. Brown, Karen Cichy, Daniel G. Debouck, Alfonso Delgado‐Salinas, Sarah Dohle, Emmalea Ernest, Consuelo Estevez Jensen, Francisco Gomez, Barbara Hellier, Alexander V. Karasev, James D. Kelly, Phillip McClean, Phillip Miklas, James R. Myers, Juan M. Osorno, Julie S. Pasche, Marcial A. Pastor‐Corrales, Timothy Porch, James R. Steadman, Carlos Urrea, Lyle Wallace, Christine H. Diepenbrock, Paul Gepts, Genetic Resources and Breeding Priorities in Phaseolus Beans, Plant Breeding Reviews, 10.1002/9781119874157.ch6, (289-420), (2022).
- Antonella Vitti, Vincenzo Bevilacqua, Giuseppina Logozzo, Stefania Marzario, Tania Gioia, Maria Nuzzaci, Response of Two Local Common Bean Ecotypes of “Fagioli di Sarconi” PGI (Phaseolus vulgaris L.) to Seed-Borne Pathogens and Environmental Change, Agronomy, 10.3390/agronomy11101924, 11 , 10, (1924), (2021).
- Elisa Bellucci, Orlando Mario Aguilar, Saleh Alseekh, Kirstin Bett, Creola Brezeanu, Douglas Cook, Lucía De la Rosa, Massimo Delledonne, Denise F. Dostatny, Juan J. Ferreira, Valérie Geffroy, Sofia Ghitarrini, Magdalena Kroc, Shiv Kumar Agrawal, Giuseppina Logozzo, Mario Marino, Tristan Mary‐Huard, Phil McClean, Vladimir Meglič, Tamara Messer, Frédéric Muel, Laura Nanni, Kerstin Neumann, Filippo Servalli, Silvia Străjeru, Rajeev K. Varshney, Marta W. Vasconcelos, Massimo Zaccardelli, Aleksei Zavarzin, Elena Bitocchi, Emanuele Frontoni, Alisdair R. Fernie, Tania Gioia, Andreas Graner, Luis Guasch, Lena Prochnow, Markus Oppermann, Karolina Susek, Maud Tenaillon, Roberto Papa, The INCREASE project: Intelligent Collections of food‐legume genetic resources for European agrofood systems, The Plant Journal, 10.1111/tpj.15472, 108 , 3, (646-660), (2021).
- Magdalena Kroc, Magdalena Tomaszewska, Katarzyna Czepiel, Elena Bitocchi, Markus Oppermann, Kerstin Neumann, Luis Guasch, Elisa Bellucci, Saleh Alseekh, Andreas Graner, Alisdair R. Fernie, Roberto Papa, Karolina Susek, Towards Development, Maintenance, and Standardized Phenotypic Characterization of Single‐Seed‐Descent Genetic Resources for Lupins, Current Protocols, 10.1002/cpz1.191, 1 , 7, (2021).
- Azalea Guerra‐García, Tania Gioia, Eric Wettberg, Giuseppina Logozzo, Roberto Papa, Elena Bitocchi, Kirstin E Bett, Intelligent Characterization of Lentil Genetic Resources: Evolutionary History, Genetic Diversity of Germplasm, and the Need for Well‐Represented Collections, Current Protocols, 10.1002/cpz1.134, 1 , 5, (2021).