Multiplexed Proximity Biotinylation Coupled to Mass Spectrometry for Defining Integrin Adhesion Complexes
Megan R. Chastney, Megan R. Chastney, Craig Lawless, Craig Lawless, Martin J. Humphries, Martin J. Humphries
BioID
cell-extracellular matrix adhesion
integrin adhesion complexes
mass spectrometry
proximity-dependent labeling
Abstract
BioID, a proximity biotinylation technique, offers a valuable approach to examine the interactions occurring within protein complexes that complements traditional protein biochemical methods. BioID has various advantages that are beneficial to the study of complexes, including an ability to detect insoluble and transient proteins. We have applied BioID to the study of integrin adhesion complexes (IACs), which are located at the junction between the plasma membrane and actin cytoskeleton. The use of multiple BioID baits enables a complex-wide, spatial annotation of IACs, which in turn facilitates the detection of novel proximal interactors and provides insights into IAC architecture. This article describes the labeling and affinity purification of IAC-proximal proteins and their analysis by label-free quantitative mass spectrometry. The article also outlines steps to identify high-confidence proximity interactors, and to interrogate the topology and functional relevance of proximity interaction networks through bioinformatic analyses. © 2020 The Authors.
Basic Protocol 1 : Proximity biotinylation of integrin adhesion complex components
Basic Protocol 2 : Mass spectrometry data processing by MaxQuant and detection of high-confidence proximal interactors
Basic Protocol 3 : Bioinformatic analysis and data visualization
INTRODUCTION
IACs are multi-protein complexes that mediate cellular attachment to the extracellular matrix (ECM) and transduce biochemical and biomechanical signals to govern cell behavior (Humphries, Chastney, Askari, & Humphries, 2019). Although proteomic cataloguing of isolated IACs has revealed hundreds of putative IAC-associated proteins (the adhesome), such approaches cannot provide direct evidence for protein-protein interactions (PPIs) (Horton et al., 2015; Kuo, Han, & Waterman, 2012). The proximity-dependent labeling technique BioID is an effective method to examine in situ proximal relationships between proteins in IACs, as it circumvents many of the experimental difficulties typically associated with the membrane-associated, labile nature of IACs (Roux, Kim, Raida, & Burke, 2012). Through multiplexing BioID (i.e., using multiple baits), a network of proximal interactions within IACs can be built. Such networks not only facilitate the identification of novel interactors, but can be used to infer sub-complex architecture of IACs through the interrogation of network topology (Chastney et al., 2020; Gupta et al., 2015; Youn et al., 2018).
This article describes a pipeline to generate and interrogate proximity interaction networks in IACs using multiplexed BioID. The first protocol (Basic Protocol 1) describes the multiplexed labeling and affinity capture of biotinylated proteins from cells stably expressing BioID adhesome constructs, which can then be analyzed by high-resolution mass spectrometry. Basic Protocol 2 outlines the detection of proteins from mass spectrometry data and the identification of high-confidence, proximal interactions. Finally, Basic Protocol 3 describes bioinformatic approaches for analyzing multiplexed BioID datasets and visualizing proximal protein interaction networks. Although this protocol describes the use of multiplexed proximity biotinylation specifically for the study of IACs, the steps could be easily adapted for other multi-protein complexes.
Strategic Planning
Selection of BioID baits for multiplexed BioID
When examining multi-protein complexes, such as IACs, the identification of components is significantly improved with an increasing number of baits. This is likely due to the relatively narrow labeling radius of BioID (∼10 nm) and the limited number of PPIs associated with each component. Even proteins in smaller tightly associated complexes identify different subsets of proximity interactors. Increasing the number of baits increases the number of prey proteins and overlapping interactions detected, which therefore improves the ability to interrogate the network topology and creates a more complete view. As such, to improve coverage of larger structures and subcellular compartments, such as IACs, the maximal number of baits should be used. However, because increasing the number of baits increases time and cost, full coverage may not be feasible, and a compromise must be made.
BioID bait design
As with any experiment involving expression of a fusion protein, it is important to consider the effect that tagging may have on the function and folding of the protein of interest. The BirA*-tagged adhesome protein should exhibit the same subcellular targeting as the endogenous protein, and should capture physiologically relevant proximal interactors, with minimal disruption by the BirA* tag. It is therefore important to take into consideration C- or N-terminal post-translational modifications, signal peptides, or protein interactions that may affect protein function or localization, and design the fusion protein accordingly. If little is known about the effects of C- and N-terminal tagging of specific proteins, it may be beneficial to generate both constructs. Generating both N- and C-terminally tagged proteins may also be of benefit to maximize the detection of proximal interactions, particularly for larger proteins, due to the relatively small labeling radius of BirA*. Subcellular targeting of fusion proteins may also be improved by using BioID2 or miniTurbo, which use smaller biotin ligases (Branon et al., 2018; Kim et al., 2016). Plasmids containing BirA* can be obtained from Addgene (https://www.addgene.org/Kyle_Roux).
Appropriate controls
The use of appropriate controls is necessary to identify high-confidence bait-prey interactions. For most applications, and for cytosolic proteins in particular, BirA* only is a suitable control. Expression of BirA* enables detection of nonspecific interactions that may occur throughout the cell during labeling, and during affinity purification and sample processing. It also enables subtraction of endogenously biotinylated proteins. However, additional negative controls can prove beneficial depending on context and baits used. For example, a membrane-targeted BirA* control could be used for membrane-targeted BirA* baits to control for nonspecific interactions focused at the cell membrane. If examining proximal interactors of mutant baits, the wild-type bait should also be used alongside the BirA*-only control. Certain statistical platforms used to identify high-confidence bait-prey interactions, such as Significance Analysis of INTeractome (SAINT) and SAINTexpress, enable multiple baits to be used as controls in the absence of a negative control such as BirA* (Teo et al., 2014). However, care should be taken if using baits from a single complex, as this may lead to false negatives if a single protein is identified by multiple baits.
Generation and validation of stably expressing cell lines
It is recommended to first validate expression and localization in cells transiently expressing the construct by western blotting and imaging. However, transient expression may lead to increased levels of nonspecific biotinylation due to large variations in expression levels commonly observed. As a result, stable expression is preferred. The specific approach to induce stable expression may need to be tailored to individual cell lines. In stably expressing cell lines, where possible, select for differentially expressing cells, as overexpression of the construct could lead to increased nonspecific biotinylation as a result of construct mis-localization. Co-expression of non-covalently attached fluorescent proteins (e.g., TagBFP) facilitates identification of positively expressing cells and sorting into different expression levels, and enables comparisons of protein expression levels across different baits and control. Expression levels close to those of endogenous levels are typically recommended, to provide sufficient biotinylated material while minimizing potential effects resulting from over-expression. However, this may vary between baits, and it is important to also consider expression levels across different baits. Although not always possible, the user may wish to aim for comparable expression levels across different baits and controls. Expression of full-length fusion protein at appropriate levels and subcellular targeting should be confirmed through western blotting and immunofluorescence (Fig. 1).
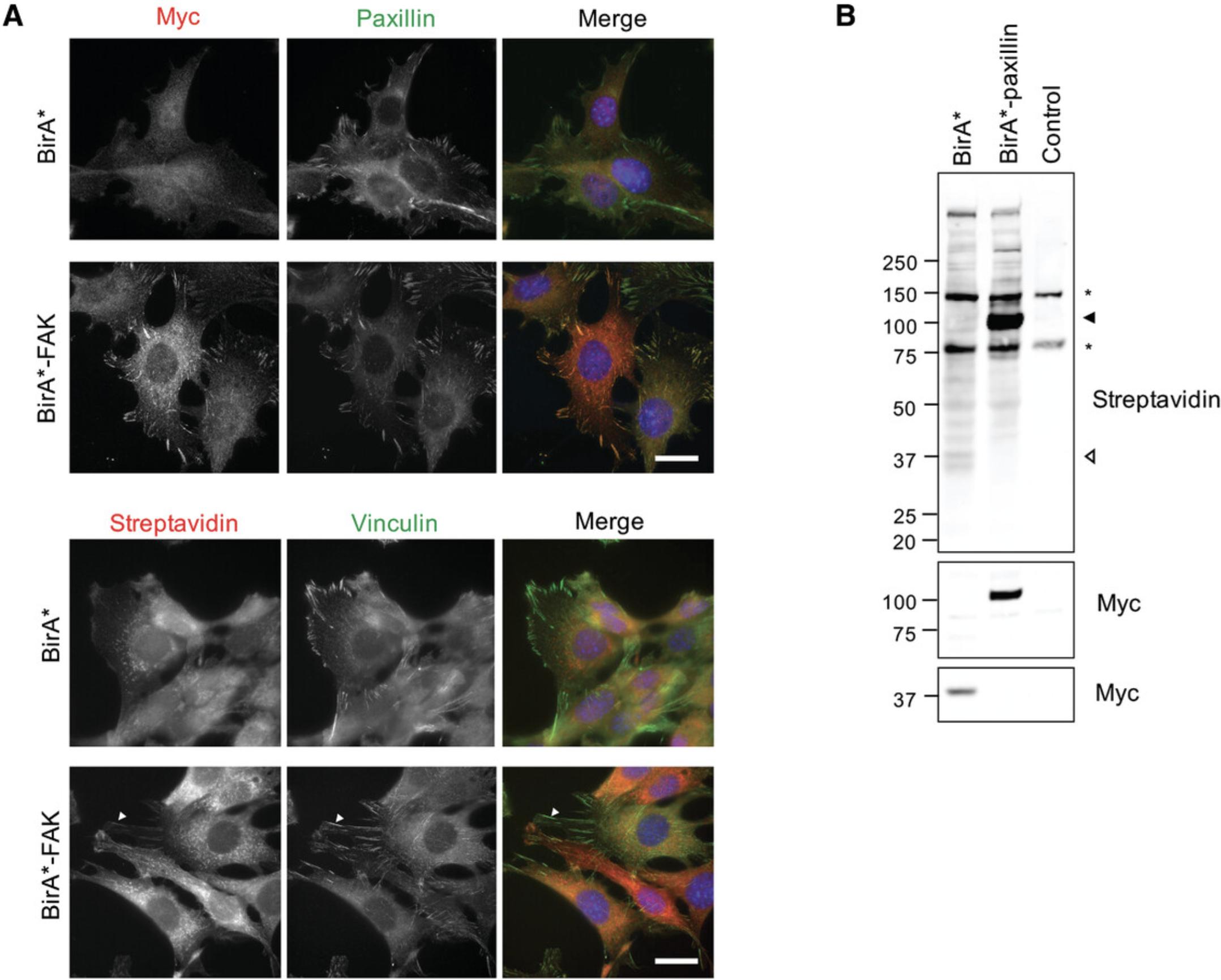
Basic Protocol 1: PROXIMITY BIOTINYLATION OF INTEGRIN ADHESION COMPLEX COMPONENTS
This protocol describes the generation and validation of cells expressing BirA*-tagged adhesome constructs, followed by the proximity labeling and isolation of biotinylated proteins to be analyzed by label-free quantitative mass spectrometry. The affinity purification of biotinylated proteins outlined here closely follows the protocol described in Current Protocols in Protein Science by Roux, Kim, & Burke (2013), with some minor modifications.
Materials
-
Target cell lines stably expressing BirA*-tagged IAC components and BirA* (or other control), with appropriate culture medium
- An additional tag for detection by immunofluorescence and western blotting is recommended (e.g., myc).
-
20× (1 mM) biotin stock solution (see recipe)
-
Phosphate-buffered saline (PBS) without calcium and magnesium (PBS; Sigma-Aldrich, cat. no. D8537)
-
Lysis buffer (see recipe)
-
20% (w/v) Triton X-100
-
50 mM Tris·Cl, pH 7.4
-
MagReSyn streptavidin magnetic beads (ReSyn Biosciences, cat. no. MR-STV010)
-
Equilibration buffer: lysis buffer with 0.1 volumes of 20% (v/v) Triton X-100 and 0.9 volumes of 50 mM Tris·Cl, pH 7.4, in addition to the Tris already present in the lysis buffer
-
5× Reducing sample buffer (RSB; see recipe)
-
Wash buffer A (see recipe)
-
Wash buffer B (see recipe)
-
Wash buffer C (see recipe)
-
2× RSB (see recipe for 5× RSB) with 100 μM biotin
-
10-cm-diameter plastic tissue culture plates
-
Cell scrapers
-
15-ml conical tubes
-
1-ml syringes (BD Plastipak, cat. no. SYR6000)
-
BD MicrolanceTM stainless steel needles (19-G, 21-G, and 25-G; Fisher Scientific, cat. no. 10234154, 10472204, and 10442204, respectively)
-
1.5-ml microcentrifuge tubes
-
Benchtop microcentrifuge at 4°C
-
Magnetic separation rack
-
End-over-end rotator
-
Shaker capable of 1000 rpm
-
Heat block
-
Additional reagents and equipment for western blotting (see Current Protocols article: Ni, Xu, & Gallagher, 2017) and mass spectrometry (see Current Protocols article: Zhang, Annan, Carr, & Neubert, 2014)
Labeling and affinity purification of proximal proteins
1.Seed cells stably expressing BirA* adhesome constructs and appropriate BioID controls on to three 10-cm-diameter tissue culture plates, and incubate for 8-24 hr. Aim for cells to be sub-confluent on day 2.
2.Add biotin (from 20× solution) to a final concentration of 50 μM, and incubate for 24 hr to initiate biotinylation of proximal proteins.
3.Wash cells three times with PBS at room temperature, draining the last wash fully to ensure minimal residual PBS.
4.Add 400 μl lysis buffer to each plate.
5.Detach cells with a cell scraper, and transfer lysate from the three plates to a 15-ml conical tube containing 120 μl 20% (v/v) Triton X-100 (40 μl/plate). Mix by pipetting. For subsequent steps, keep lysate on ice.
6.Pass cell lysates four times through a 25-G needle using a 1-ml syringe, followed by four times through a 21-G needle.
7.Add 1.08 ml of ice-cold 50 mM Tris·Cl, pH 7.4 (360 μl/plate), and pass four times through a 19-G needle.
8.Transfer cell lysate to two 1.5-ml microcentrifuge tubes and microcentrifuge 10 min at top speed (∼16,000 × g), 4°C.
9.Aliquot 45 μl of resuspended magnetic streptavidin beads per condition into duplicate 1.5-ml tubes (15 μl per plate), and place on a magnetic rack.
10.Wait for the beads to accumulate on the tube wall, and remove the bead buffer.
11.Wash twice in 100 μl equilibration buffer (lysis buffer with with 0.1 volumes 20% (v/v) Triton X-100 and 0.9 volumes 50 mM Tris·Cl, pH 7.4), ensuring that beads are fully resuspended between wash steps.
12.Remove the equilibration buffer and add the supernatant from step 8, taking care not to disturb the pellet at the bottom of the tube.
13.Resuspend the beads by inverting the tube, and rotate at 4°C overnight on an end-over-end rotator.
14.Briefly centrifuge tubes to remove residual sample from the tube caps, and place the tubes on a magnetic rack to collect the beads on the walls of the tubes.
15.Without disturbing the beads, remove the supernatant.
16.To pool beads from each sample, add 1 ml wash buffer A to the first of the two tubes, resuspend, and add to the second tube.
17.Rotate the tubes for 5 min at room temperature.
18.Repeat steps 14 and 15 to remove supernatant, and add 1 ml wash buffer A.
19.Rotate the tubes for 5 min at room temperature.
20.Repeat steps 14 and 15 to remove supernatant, and add 1 ml wash buffer B.
21.Rotate the tubes for 5 min at room temperature.
22.Repeat steps 14 and 15 to remove supernatant, and add 1 ml wash buffer C.
23.Rotate the tubes for 5 min at room temperature.
24.Repeat steps 14 and 15 to remove supernatant, ensuring that no residual buffer is remaining. Add 100 μl of 2× RSB with 100 μM biotin to each tube, and gently resuspend beads.
25.Incubate samples at 70°C for 10 min, with shaking (∼1000 rpm).
26.Repeat step 14, and transfer the supernatant containing the eluted biotinylated proteins to a new tube.
Confirm results and perform analyses
27.Confirm the presence of biotinylated proteins in eluted samples through western blotting (see Current Protocols article: Ni et al., 2017).
28.Successful experiments can then be prepared for and analyzed by high-resolution label-free quantitative mass spectrometry (see Current Protocols article: Zhang et al., 2014).
Basic Protocol 2: MASS SPECTROMETRY DATA PROCESSING BY MaxQuant AND DETECTION OF HIGH-CONFIDENCE PROXIMAL INTERACTORS
The raw files generated from the mass spectrometry analysis need to be processed to identify proteins and produce relative abundance measurements between control and bait experiments in order to estimate proximal interactions. Here we describe how to produce MS1 intensity values using MaxQuant software (Cox & Mann, 2008), but it is feasible to use other protein quantitation software applications such as Proteome Discover or Progenesis QI. Protein intensities can then be used to estimate proximal interactions. Here, the Significance Analysis of INTeractome (SAINT) software is used (Teo et al., 2014).
Materials
- MaxQuant version 1.6.3.4 (requires registration) available from https://maxquant.org/
- MaxQuant minimum hardware requirements are:
- Intel Pentium III/800 MHz or higher (or compatible), although one should probably not go below a dual-core processor
- 2 GB RAM minimum
- 2 GB RAM per thread that is executed in parallel is required
- There is no upper limit on the number of cores. Whatever you can fit into a shared memory machine will work as long as the disk performance scales up with it.
- SAINTexpress available from https://sourceforge.net/projects/saint-apms/files/
- SAINTexpress requires g++ version 4.4 or above for compilation of a complete install. Pre-compiled versions are also bundled with the download.
1.Using MaxQuant version 1.6.3.4, process the raw files to obtain protein intensities for each sample.
2.Prepare three input files required for SAINTexpress using the LFQ intensities from the MaxQuant proteinGroups.txt output file (as illustrated in Fig. 2).
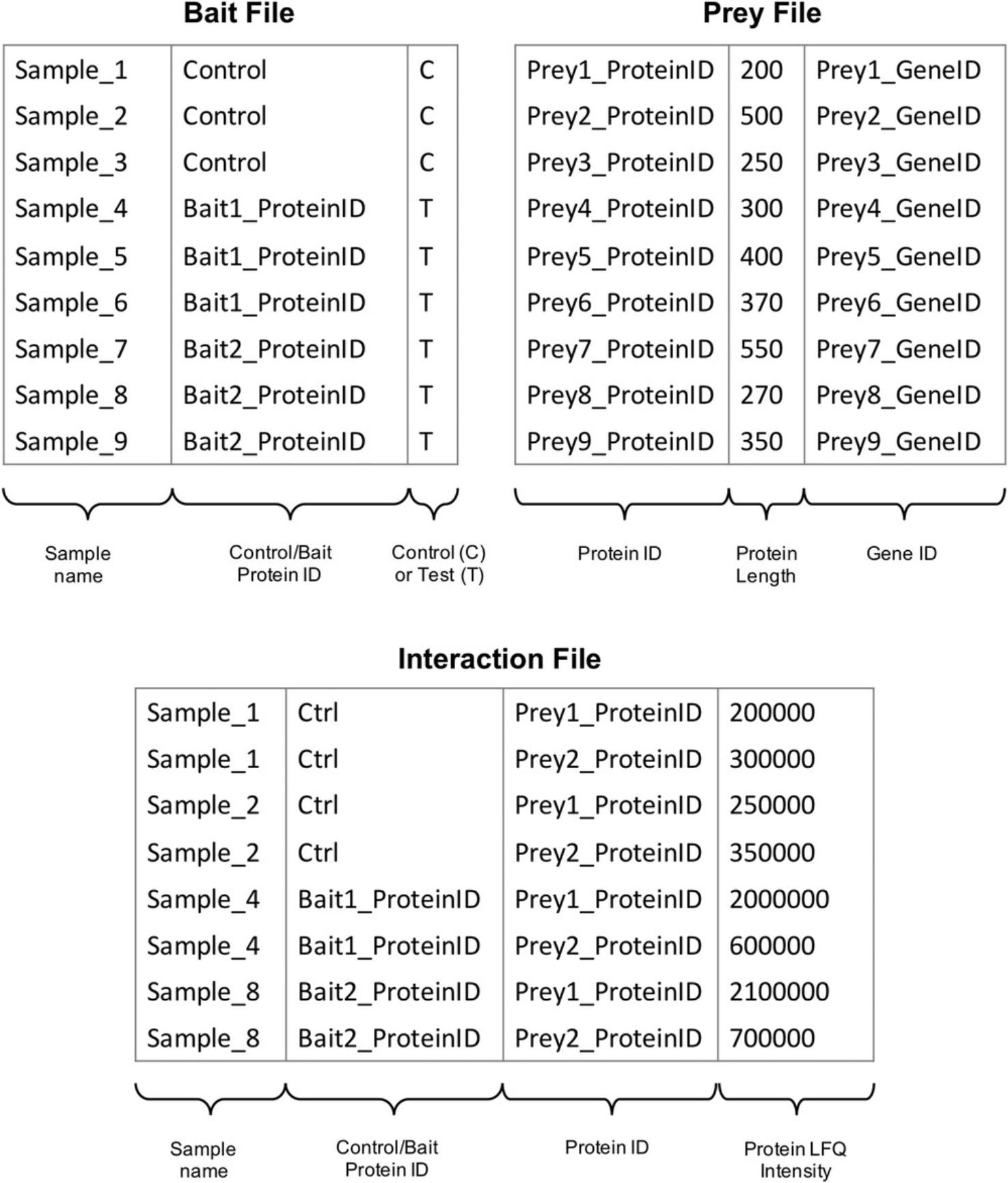
3.Run SAINTexpress using the three correctly formatted input files generated from the MaxQuant proteinGroups.txt.
-
SAINTexpress-int interaction.txt prey.txt bait.txt
4.Filter SAINTexpress results for significant proximal interactions (as illustrated in Fig. 3).
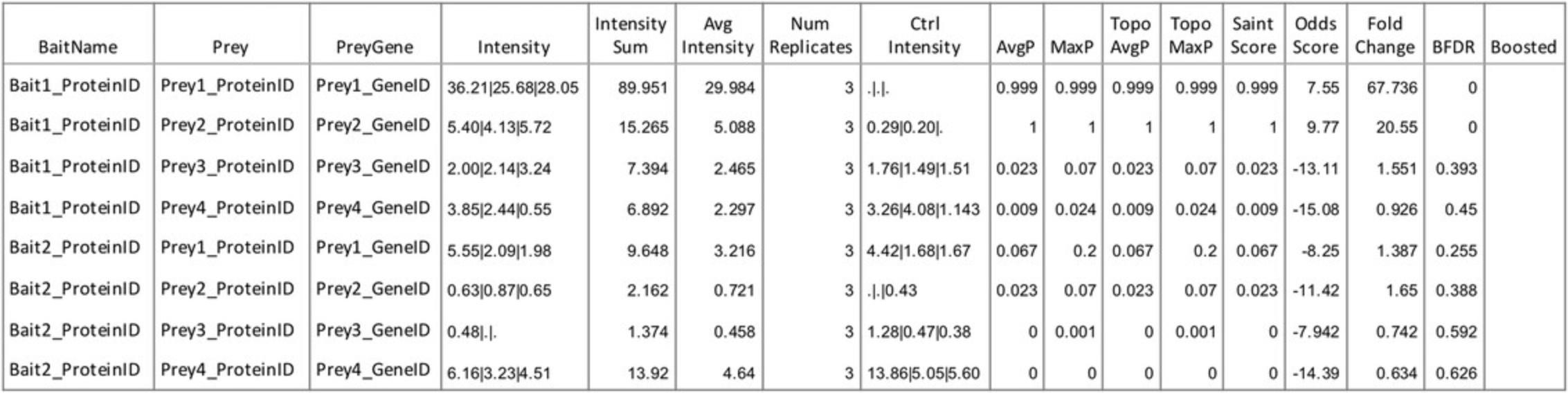
Basic Protocol 3: BIOINFORMATIC ANALYSIS AND DATA VISUALIZATION
This protocol outlines bioinformatic approaches that can be used to interrogate the topology of proximity interaction networks generated from multiplexed BioID experiments. Unbiased hierarchical cluster analysis can be used to determine relationships between baits and prey, which can then be analyzed for over-represented functional terms using gene ontology. Finally, this protocol describes the visualization of proximity interaction networks using Cytoscape. Although Cytoscape has many useful features that can interrogate various features of networks, this protocol describes the basic steps required to visualize the network.
Materials
- Computer with access to the internet
- R (version 3.5+, available from https://www.r-project.org) with the following R packages:
- Pheatmap (version 1.0.12+, available via CRAN)
- Reshape2 (version 1.4.4+, available via CRAN)
- Bioconductor (version 3.8+, available from http://bioconductor.org/)
- clusterProfiler (3.10.1+, available via Bioconductor) (Yu, Wang, Han, & He, 2012)
- Genome-wide annotation for specific species used. For mouse, use “org.Mm.eg.db” (version 3.7.0+, available via Bioconductor).
- Cytoscape (version v.3.7.2+; available from https://cytoscape.org)
Cluster analysis in R
1a. Import SAINTexpress results file into R, filter for significant interacting preys in at least one bait experiment and format for clustering analysis.
-
#import results into a data frame
-
results <- read.table(
- file = "saint_results.txt",
- sep="\t",
- quote="",
- stringsAsFactors=FALSE,
- header=TRUE,
- fill = TRUE)
-
#transform fold changes using log2
-
results
FoldChange) -
#create 3 data frames for prey clustering, bait clustering #and heatmap value overlay.
-
#create data frame for prey presence/absence clustering at 5% Bayesian false discovery rate (BFDR) threshold.
-
prey <- results[results$BFDR<= 0.05,]
-
prey <- dcast(prey[, c("Bait", "Prey", "log2FC")],
- Prey ∼ Bait,
- value.var = "logFC")
-
prey[,2:ncol(prey)] <- ifelse(
- is.na(prey[,2:ncol(prey)]),0, 1)
-
#create data frame for heatmap value overlay, keeping #log2FC of respective preys across all baits
-
sigInts <- unique(results[results
Prey) -
bait_overlay <- results[results$Prey %in% sigInts,]
-
bait_overlay <- dcast(bait[, c("Bait", "Prey", "log2FC")],
- Prey ∼ Bait,
- value.var = "logFC")
-
#create data frame for bait clustering, removing bait-bait #log2FC to avoid any bias
-
bait <- bait_overlay
-
for(i in colnames(bait)[2:ncol(bait)]){
-
bait[bait$Prey==i,i] <- NA
-
}
2a. Define row names, then perform hierarchical clustering on bait using Jaccard's distance (binary) and prey using Euclidean distance.
-
Define row names
-
rownames(prey)<-prey$Prey
-
rownames(bait)<-bait$Prey
-
rownames(bait_overlay)<-bait_overlay$Prey
-
Cluster bait
-
exp_euc_dist<-dist(t(bait[, 2:ncol(bait)]),
- method="euclidean")
-
exp_euc_hclust<-hclust(exp_euc_dist)
-
Cluster prey
-
prot_bin_dist<-dist(prey[, 2:ncol(prey)],
- method="binary")
-
prot_bin_hclust<-hclust(prot_bin_dist)
3a. Generate a hierarchically clustered heatmap, using log2 fold change as an overlay display (see Fig. 4 for an example).
- pheatmap(bait_overlay[, 2:ncol(bait_overlay)],
- cluster_rows = prot_bin_hclust,
- cluster_cols = exp_euc_hclust)
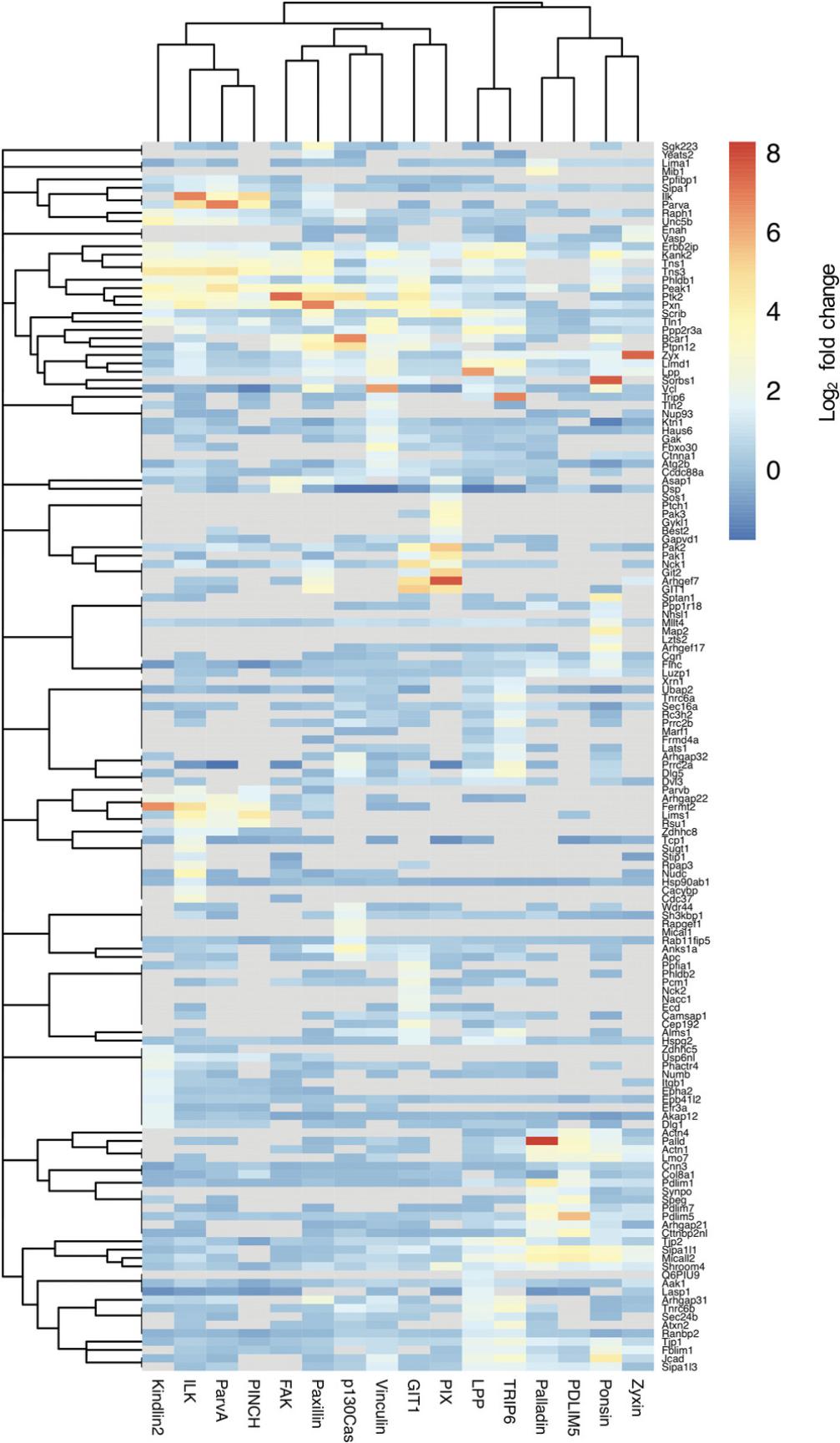
Functional enrichment analysis of bait and prey clusters using clusterProfiler
1b. Assemble a tab-delimited text file containing lists of high-confidence proximity interactions (with bait or prey cluster as column headings, e.g., BaitA, BaitB, BaitC, etc.), and import into R.
- genelists <- read.table(
- file = "gene_lists.txt",
- sep="\t",
- quote="",
- stringsAsFactors=FALSE,
- header=TRUE,
- fill = TRUE)
2b. Convert gene lists (from "SYMBOL", for example) into EntrezIDs.
-
Generate lists of EntrezIDs
-
idBaitA<- c(bitr(genelists$BaitA,
- fromType = "SYMBOL",
- toType = "ENTREZID",
- OrgDb = "org.Mm.eg.db"))
-
idBaitB<- c(bitr(genelists$BaitB,
- fromType = "SYMBOL",
- toType = "ENTREZID",
- OrgDb = "org.Mm.eg.db"))
-
idBaitC<- c(bitr(genelists$BaitC,
- fromType = "SYMBOL",
- toType = "ENTREZID",
- OrgDb = "org.Mm.eg.db"))
-
Assemble converted BaitID vectors into a list object
-
entrezlist<- list(idBaitA$ENTREZID,
- idBaitB$ENTREZID,
- idBaitC$ENTREZID)
-
names(entrezlist)<- c("BaitA", "BaitB", "BaitC")
3b. Perform functional enrichment analysis using compareCluster, and display as a dotplot (see Fig. 5 for an example). The “Molecular function” ("MF") ontological category is shown below. “Biological process” and “cellular compartment” are also available (ont = "BP" and "CC", respectively). Other functions are available to visualize results, including cnetplot and enrichMap.
-
GOCC <- compareCluster(geneClusters = entrezlist, fun = "enrichGO",
- OrgDb= org.Mm.eg.db,
- pvalueCutoff=0.05,
- ont="CC",
- readable=T)
-
Display as dotplot
-
dotplot(GOCC, showCategory= 10)
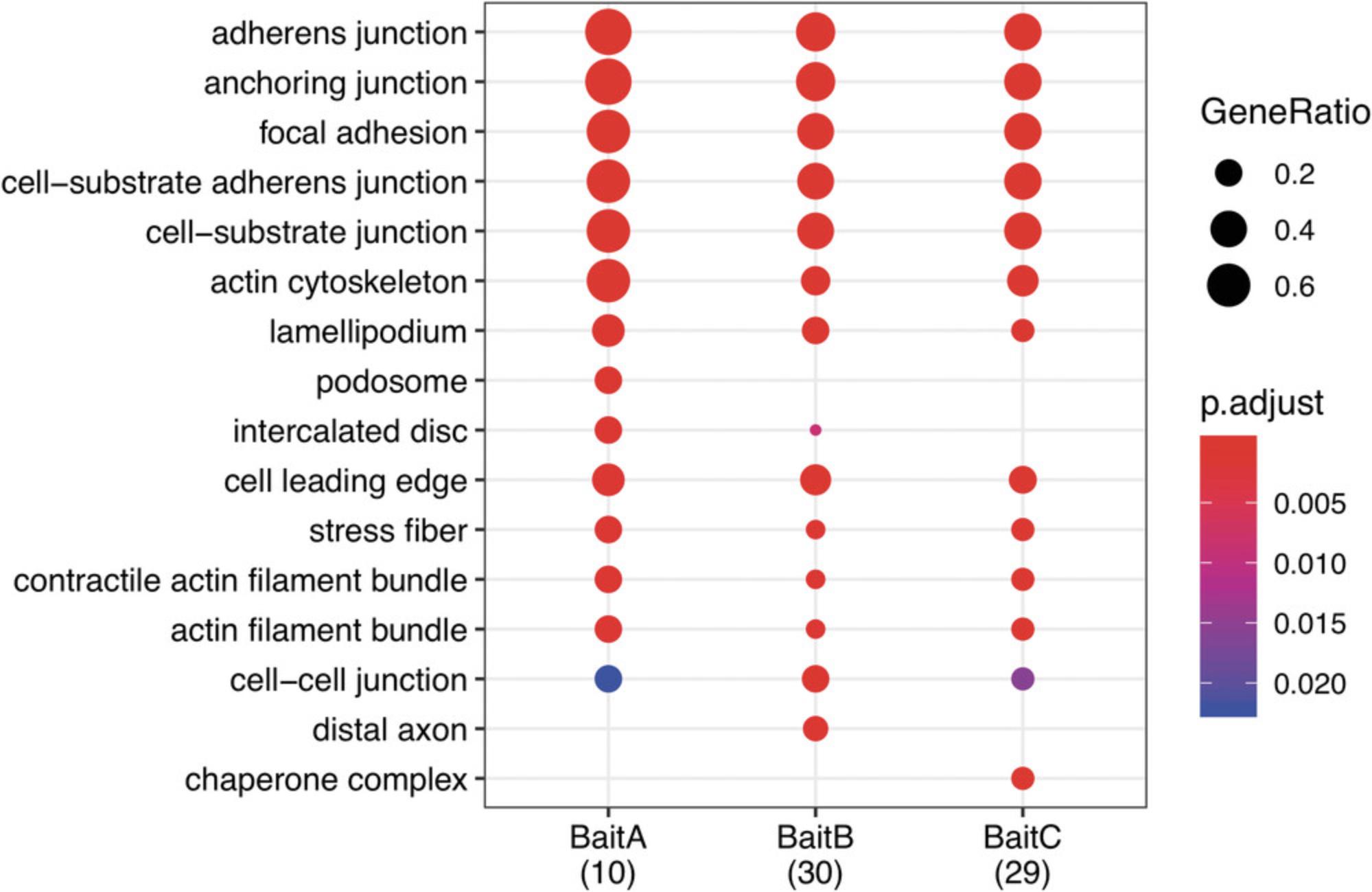
Visualization of interaction networks in Cytoscape
1c. Assemble a .xls or .txt file containing columns with all relevant information. The minimal information required is bait, prey, and BFDR (or SAINT score). Other information that could be included are fold change over control (log2), abundance (or intensity), presence/absence of prey in other datasets (e.g., published adhesomes), bait and prey cluster, and functional relevance (see Fig. 6 for an example). Ensure that there are no formulas, blank spaces, or duplicated headings.
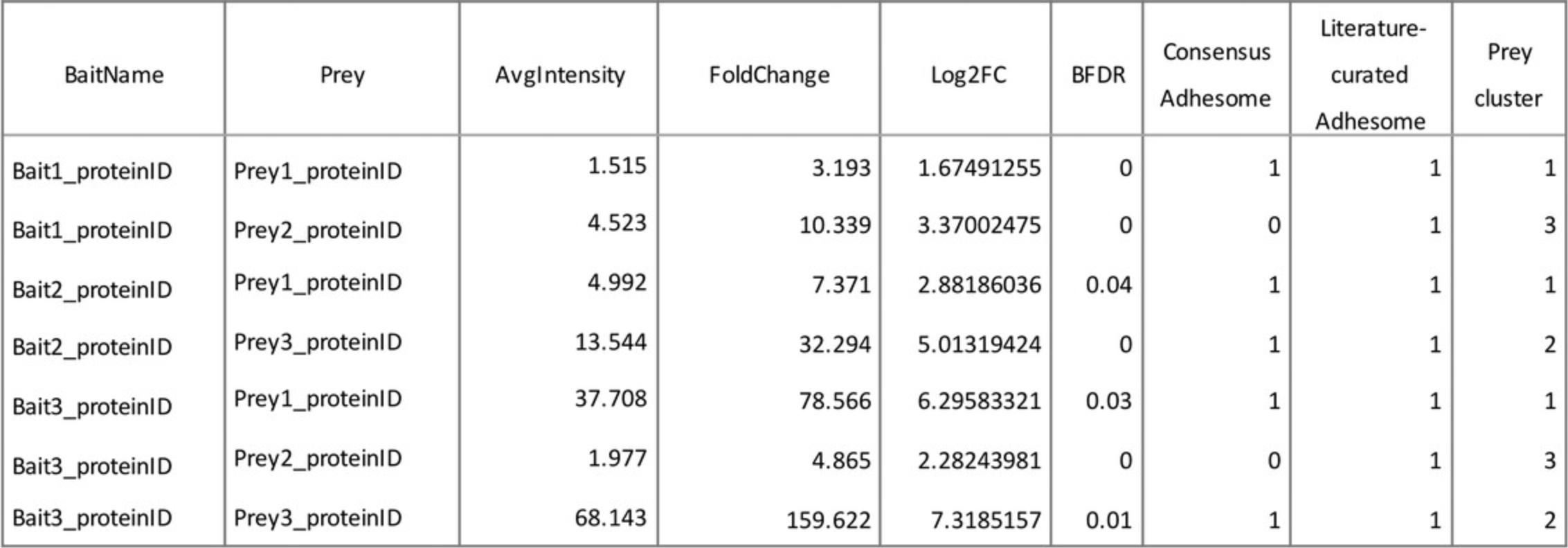
2c. Open Cytoscape. To import your network, go to menu File → Import → Network from File… and select the file assembled in step 1.
3c. In the “Import Network from Table” box that appears, assign a column “meaning” to each column and assign data type from the drop-down box that appears by clicking on each column heading.
4c. Click “OK.” A network will appear, consisting of all the bait and prey (nodes) and interactions (edges) from the input file.
5c. Use column filters to select for high-confidence proximity interactions (e.g., BFDR ≤ 0.05) to create a sub-network of relevant interactions. Choose “Select” in the Control Panel, and click on the “+” icon to add “Column Filter.” Choose selection criteria (e.g., BFDR) from the drop-down menu, select filter value (enter manually or use sliding bar), and then click “Apply.”
6c. Create a new network from the selected nodes and edges by clicking the “New Network from Selection” icon, or by going to menu File → New Network → From Selected Nodes, Selected Edges.
7c. Alter the network layout by manually dragging nodes, or by selecting options from the drop-down Layout menu (e.g., Layout → Perfuse Force Directed layout).
8c. Change the appearance of the network, and map values (e.g., BFDR, fold change) onto nodes and edges in the “Style” menu on the Control Panel.
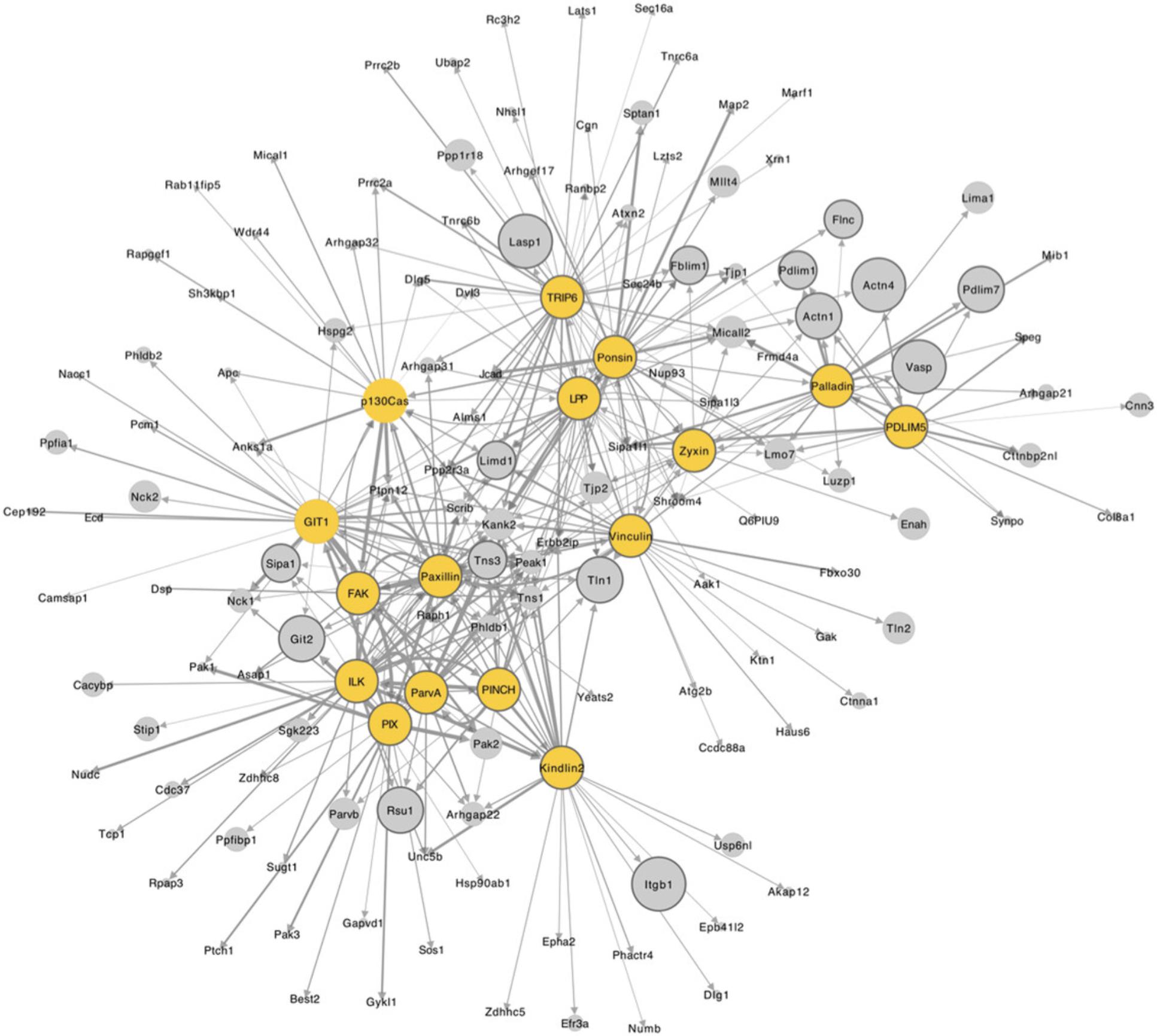
REAGENTS AND SOLUTIONS
In all recipes, use double-deionized water (e.g., using Milli-Q water purification system, MerckMillipore). All reagents were purchased from Sigma-Aldrich, unless specified.
Biotin stock solution (1 mM, 20×)
Add 12.2 mg biotin (Sigma-Aldrich, cat. no. B4501) to 50 ml serum-free medium or Opti-MEM (ThermoFisher Scientific, cat. no. 31985062). When the biotin has dissolved, filter sterilize by passing through a 0.22-μm filter. Store at 4°C for up to 8 weeks.
Lysis buffer
- 0.5 M NaCl
- 50 mM Tris·Cl, pH 7.4
- 0.2% (w/v) SDS
- Store with above ingredients up to 2 weeks at room temperature.
- Just before use, add the following:
- 1× cOmpleteTM Protease Inhibitor Cocktail (Roche, cat. no. 11697498001)
- 1 mM DTT
Reducing sample buffer (RSB), 5×
- 10% (w/v) SDS
- 125 mM Tris·Cl, pH 6.8
- 25% (w/v) glycerol
- 0.01% (w/v) bromophenol blue
- 0.1% (v/v) β-mercaptoethanol
- Store with the above ingredients up to 1 year at 4°C
Wash buffer 1
- 2% (w/v) SDS
- Store up to 2 weeks at room temperature
Ensure that SDS is fully dissolved before use.
Wash buffer 2
- 0.5 M NaCl
- 1 mM EDTA
- 1% (w/v) Triton X-100
- 50 mM HEPES, pH 7.5
- 0.1% (w/v) deoxycholic acid
- Store for up to 2 weeks at room temperature
Keep stock solutions of deoxycholic protected from light.
Wash buffer 3
- 10 mM Tris·Cl, pH 7.4
- 1 mM EDTA
- 0.5% (w/v) NP-40
- 0.5% (w/v) deoxycholic acid
- Store for up to 2 weeks at room temperature
Keep stock solutions of deoxycholic protected from light.
COMMENTARY
Background Information
Since its development, BioID has become a popular method to screen for proximal interactors, on scales ranging from individual proteins and complexes to large-scale network mapping initiatives (Chastney et al., 2020; Dong et al., 2016; Gupta et al., 2015; Roux et al., 2012; Youn et al., 2018). BioID uses a promiscuous mutant biotin ligase (BirA R118G; BirA*) from E. coli , fused to a protein of interest, to label proximal proteins (Roux et al., 2012). Upon the addition of biotin, BirA* generates biotinoyl-5′-AMP, a highly reactive moiety that covalently attaches to primary amines of available lysines on proteins within a ∼10 nm radius (Kim et al., 2014). These proteins can then be affinity purified and analyzed by mass spectrometry.
BioID has many advantages that make it a valuable method to examine proximity interactions, and complements existing techniques to examine IAC composition and architecture. For example, traditional antibody-based affinity purification approaches rely on PPIs being maintained throughout processing, which is likely to lead to poor detection of weakly and transiently interacting proteins. Furthermore, the mild lysis and wash conditions required to maximize retention of PPIs typically result in fewer poorly soluble and membrane-associated proteins being detected (a particular issue for many IAC components). Conversely, BioID labeling occurs in situ and does not rely on PPIs being maintained throughout processing. BioID is therefore able to detect transient and weakly interacting proteins, and allows for stringent lysis and wash conditions to facilitate capture of poorly soluble and membrane-localized proteins, resulting in fewer nonspecific proteins. In situ labeling also leads to the capture of endogenous interactions, while conventional affinity purification approaches may detect PPIs that occur in vitro. Importantly, as BioID has a limited labeling radius, it provides a means to annotate whole complexes with spatial information.
However, there are a number of limitations that must be kept in mind while interpreting BioID data. For example, BioID requires the exogenous expression of the BirA*-tagged protein of interest, while antibody-based affinity capture can be performed on endogenous proteins (if antibodies are available). Exogenous tagging of a protein of interest with BirA* may lead to poor subcellular targeting or steric hindrance of PPIs, leading to false negatives. BioID2, which uses a smaller biotin ligase, was developed to minimize steric hindrance and improve targeting (Kim et al., 2016). False negatives may also arise if proximal proteins lack accessible lysines. The long labeling time required to induce sufficient biotinylation (typically 8-24 hr) is also unsuitable for time-sensitive experiments (e.g., cell-cycle time points or growth factor receptor stimulation). In such cases, alternative proximity-labeling approaches may be more suitable, such as turboID or APEX and APEX2 (Branon et al., 2018; Lam et al., 2015; Martell et al., 2012). Furthermore, although no significant effects have been reported thus far, the long-term consequences of biotinylation on proteins are currently unknown, and it is possible that post-translational modifications or PPIs could be altered. Importantly, absolute intensity values or differences in abundance between prey and bait types does not necessarily directly correlate with degree of proximity, as these values can also result from other variables such as changes in abundance, interaction frequency, and duration of interaction. Further validation is required to determine the nature of such interactions.
Nevertheless, BioID has proven effective for examining proximal interactors of individual proteins and larger complexes that are typically difficult to study, such as cell-cell contacts, the centrosome, and IACs (Chastney et al., 2020; Dong et al., 2016; Gupta et al., 2015; Van Itallie et al., 2013). In larger-scale network mapping studies, multiplexed BioID has been used to reveal the spatial relationships between components of large complexes, and even between multiple organelles (Chastney et al., 2020; Go et al., 2019; Gupta et al., 2015; Youn et al., 2018). In such initiatives, careful analysis is required to interpret the data and to infer potential biological insight. First, high-confidence proximity interactions must be identified. Among the most commonly used statistical models to identify high-confidence proximity interactions from BioID experiments are SAINT and SAINTexpress (Choi et al., 2010; Teo et al., 2014), which use probabilistic scoring to objectively identify true bait-prey interactions over background nonspecific noise. Other probability scoring methods, including MiST, ComPASS, and HGScore, have also been developed to predict high-confidence interactors from affinity purification experiments (Guruharsha et al., 2011; Jäger et al., 2012; Sowa, Bennett, Gygi, & Harper, 2009). Once high-confidence proximity interactions are identified, bioinformatic approaches such as hierarchical clustering and functional enrichment analyses are often used to identify groups of commonly detected proteins and determine putative functional roles. While these can be performed in R (as described in Basic Protocol 3), a number of online tools are also available that may be preferable for those with limited experience in R. For example, Prohits-Viz is a useful tool for visualizing PPI datasets such as those generated from multiplexed BioID, and supports outputs from SAINT and SAINTexpress (Knight et al., 2017). In addition to visualizing networks, Cytoscape contains various plugins to further interrogate networks, including those for gene ontology and network analysis (e.g., BiNGO and NetworkAnalyzer, respectively) (Assenov, Ramírez, Schelhorn, Lengauer, & Albrecht, 2007; Maere, Heymans, & Kuiper, 2005; also see Current Protocols article: Su, Morris, Demchak, & Bader, 2014).
Critical Parameters and Troubleshooting
Troubleshooting may be required for a number of steps throughout this protocol. First, generation of the BirA*-tagged proteins by molecular cloning can take some time if setbacks are encountered, with troubleshooting dependent on the specific molecular cloning approach used. Similarly, difficulties that arise from generating stable cell lines require troubleshooting specific to the approach used. The use of a self-cleaving fluorescent tag (e.g., TagBFP) co-expressed alongside the BirA*-tagged adhesome protein at a 1:1 ratio facilitates sorting of positively expressing cells into different populations (i.e., high, medium, and low), and can overcome some of the issues associated with subcloning and screening for expressing cells. These differentially expressing cells can then be examined to select populations with optimal bait targeting (minimal over-expression and mis-localization) and to examine expression levels between cells expressing different baits. If baits show poor co-localization with the target subcellular compartment (e.g., IACs), it may be necessary to consider tagging a different terminus (N- or C-terminus) or adding a linker between BirA* and the bait.
Multiplexed BioID generates a relatively large number of samples, which can take considerable time to prepare and analyze by mass spectrometry. While it is preferable to process and analyze the maximal number of baits in parallel to avoid batch-to-batch variation (e.g., tryptic digestion, mass spectrometry, run conditions), it is not always feasible to prepare samples from all baits in a single batch. Given that the appropriate negative controls are used and processed in parallel with other baits, it is possible to combine datasets taken at different time points, as the control should allow for subtraction of differences in mass spectrometry sample preparation and analysis. Care should also be taken to minimize the risk of carry-over during mass spectrometry runs (e.g., use pooled samples, run replicates together).
The processing of raw file files within MaxQuant requires the correct fasta file to search the spectra against. It should contain the sequences of all non-endogenous baits used, across all experiments, in order to calculate the correct intensity measurement for subsequent interaction analysis using SAINT or SAINTexpress. Absence of such information can lead to reduction in significant proximal interactions and increase false-positive interactions due to an inferred lower quantification.
The number of high-confidence interactions identified is likely to vary in a bait-dependent manner (typically between 10 and 50). However, a number of experimental and bioinformatic differences can also contribute to the number of high-confidence bait-prey interactions ultimately identified. If very few high-confidence proximity interactions are identified despite significant biotinylation in samples observed by western blotting, it may be necessary to examine the quality of the mass spectrometry data. The mass spectrometry spectra should be of high quality, with consistency across repeats (minimum of three biological replicates), and analyses performed, such as pair-wise comparisons and PCA, should show distinction between the BirA* control and BirA*-tagged baits and similarity across repeats.
Understanding Results
The first step required for multiplexed BioID is the generation of stably expressing cell populations which express full-length BioID baits that target to the sub-cellular region of interest (e.g., IACs). Immunofluorescence imaging should show co-localization of BirA*-tagged adhesome components (using antibodies against the BirA* tag, e.g., myc) with IAC markers such as paxillin or vinculin (Fig 1A). Meanwhile, the BirA* control should show no specific co-localization with IACs, and should be observed throughout the cytosol and the nucleus (Fig 1A). Streptavidin-conjugated fluorophores can be used to visualize the subcellular localization of biotinylated proteins. Structures resembling mitochondria may be observed in cells stained for biotinylated proteins (including control cells with no BirA* expression), which are likely to result from the presence of endogenously biotinylated proteins in this organelle. The localization of biotinylated proteins detected by streptavidin at IACs may be less clear than that observed for BirA*-tagged adhesome proteins, due to the detection of endogenously biotinylated proteins and the labeling of proximal interactors that may subsequently translocate to the cytosol and other subcellular compartments. Endogenously biotinylated proteins can also be observed by western blotting (Fig. 1B), which should be used to confirm expression of full-length fusion protein and biotinylation of proteins (e.g., using streptavidin-conjugated fluorophores). Often, the bait protein itself is among the most prominent bands detected by streptavidin, though this varies between baits. Where possible, cell populations with optimal targeting should be selected to maximize identification of candidate proteins. Over-expression can lead to poor subcellular targeting and elevated nonspecific interactions, while minimal expression increases the relative proportion of background endogenously biotinylated proteins over proximal interactions.
Prior to interaction analysis using SAINT or SAINTexpress, it is essential that the underlying LFQ intensities be checked. At the simplest level, the LFQ correlations in an all-versus-all comparison can highlight issues with any samples. Correlations should be higher within sample replicates than against other samples, the caveat being that different baits that interact with same or a similar subset of proteins may produce high correlations between them. Lower within-replicate correlation is cause for concern, and would require further inspection. Progressing regardless of this to SAINT or SAINTexpress would incorporate higher variation of protein measurements within the bait replicates, and thus raise the level of noise.
SAINT and SAINTexpress enable the identification of high-confidence proximity interactions (e.g., BFDR ≤ 0.05) that are consistently detected with a higher abundance in bait over the BirA* control. The list of proteins that meet required thresholds varies between baits and analytical methods, and is also dependent upon the experimental quality. For example, large variations across experimental repeats are likely to result in fewer high-confidence proximity interactions. Typically, for good-quality data when following Basic Protocol 2, between 10 and 50 proximity interactors can be identified per bait. These lists are likely to contain well-established direct interactors of the bait protein (if known) and other adhesome components. Functional enrichment analysis of proximity interactors should identify terms relating to cell-ECM adhesion as being over-represented (Fig. 5). Functional enrichment analysis may also be useful to identify potentially under-appreciated roles for baits.
When using multiple baits within a given complex, such as an IAC, it is likely that a number of proteins will be identified by multiple baits, while others are uniquely identified by a single bait. This will be reflected in the hierarchical cluster analysis. Proteins that cluster together (both baits and prey) are likely to represent groups of proteins that share common interactors. For example, it is probable that baits known to closely associate will be identified within the same cluster (e.g., the IPP complex components ILK, PINCH, and parvin are found to cluster together in Fig. 4). However, commonly detected interactors may not interact within the same space and time (i.e., within the same complex). As proximity labeling is unable to differentiate between abundance, proximity, and interaction frequency, further validation is required to determine the nature of the relationship between baits and candidate prey. Furthermore, as many proteins locate to multiple subcellular compartments, it is important to note that proximity interactions may occur outside of the complex of interest (i.e., IACs).
This article outlines only a few basic bioinformatic tools to interrogate proximity interaction networks. Various plugins are available in Cytoscape to interrogate networks, and datasets can be further examined through comparisons with PPI databases (e.g., BioGRID, STRING), published adhesomes (Horton et al., 2015; Winograd-Katz, Fässler, Geiger, & Legate, 2014), and other proximity interaction studies (Chastney et al., 2020; Dong et al., 2016).
Time Considerations
Generation of constructs and their validation by transient transfection can be achieved in as little as a week, though it is possible that issues may arise. The generation of stably expressing cells by lentiviral transduction and their sorting into differentially expressing cell lines can take 3-4 weeks with validation by immunofluorescence imaging and western blotting. A BioID experiment can be performed in less than 1 week. Depending on how many BioID baits are being used, it may be beneficial to perform more than one batch of BioID experiments (each with a relevant negative control). Preparation and analysis of samples by mass spectrometry can take less than a week, although this depends on the number of samples. Processing data using MaxQuant and SAINT/SAINTexpress analysis can take one to several days, depending on the number of baits used and computational capacity. Although bioinformatic analysis can in theory be performed in as little as one day, it may take considerably longer to fully interrogate the data. From start to finish, a multiplexed BioID experiment (∼16 baits) can be performed in less than 2 months.
Acknowledgments
We thank Jonathan Humphries for helpful discussions and feedback on the manuscript, and Beverley Wilson for contributions regarding the affinity purification of biotinylated proteins. The support of Stacey Warwood and David Knight of the Bio-MS mass spectrometry facility in the Faculty of Biology, Medicine and Health at the University of Manchester is gratefully acknowledged. This work was supported by a Cancer Research UK Program Grant (C13329/A21671 to M.J. Humphries), and M.R. Chastney was supported by a PhD studentship from Biotechnology and Biological Sciences Research Council. The work was conducted within the Wellcome Centre for Cell-Matrix Research (core award 203128/Z/16/Z).
Author Contributions
Megan R. Chastney : Conceptualization; formal analysis; investigation; methodology; visualization; writing-original draft; writing-review & editing. Craig Lawless : Data curation; formal analysis; methodology; software; visualization; writing-original draft; writing-review & editing. Martin J. Humphries : Conceptualization; funding acquisition; project administration; supervision; writing-review & editing.
Literature Cited
- Assenov, Y., Ramírez, F., Schelhorn, S.-E., Lengauer, T., & Albrecht, M. (2007). Computing topological parameters of biological networks. Bioinformatics , 24(2), 282–284. doi: 10.1093/bioinformatics/btm554.
- Branon, T. C., Bosch, J. A., Sanchez, A. D., Udeshi, N. D., Svinkina, T., Carr, S. A., … Ting, A. Y. (2018). Efficient proximity labeling in living cells and organisms with TurboID. Nature Biotechnology , 36, 880. doi: 10.1038/nbt.4201.
- Chastney, M. R., Lawless, C., Humphries, J. D., Warwood, S., Jones, M. C., Knight, D., … Humphries, M. J. (2020). Topological features of integrin adhesion complexes revealed by multiplexed proximity biotinylation. Journal of Cell Biology , 219(8), e202003038. doi: 10.1083/jcb.202003038.
- Choi, H., Larsen, B., Lin, Z.-Y., Breitkreutz, A., Mellacheruvu, D., Fermin, D., … Nesvizhskii, A. I. (2010). SAINT: Probabilistic scoring of affinity purification−mass spectrometry data. Nature Methods , 8, 70. doi: 10.1038/nmeth.1541.
- Cox, J., & Mann, M. (2008). MaxQuant enables high peptide identification rates, individualized p.p.b.-range mass accuracies and proteome-wide protein quantification. Nature Biotechnology , 26(12), 1367–1372. doi: 10.1038/nbt.1511.
- Dong, J.-M., Tay, F. P.-L., Swa, H. L.-F., Gunaratne, J., Leung, T., Burke, B., & Manser, E. (2016). Proximity biotinylation provides insight into the molecular composition of focal adhesions at the nanometer scale. Science Signaling , 9(432), rs4 LP–rs4. doi: 10.1126/scisignal.aaf3572.
- Go, C. D., Knight, J. D. R., Rajasekharan, A., Rathod, B., Hesketh, G. G., Abe, K. T., … Gingras, A.-C. (2019). A proximity biotinylation map of a human cell. BioRxiv , 796391. doi: 10.1101/796391.
- Gupta, G. D., Coyaud, É., Gonçalves, J., Mojarad, B. A., Liu, Y., Wu, Q., … Pelletier, L. (2015). A dynamic protein interaction landscape of the human centrosome-cilium interface. Cell , 163(6), 1484–1499. doi: 10.1016/j.cell.2015.10.065.
- Guruharsha, K. G., Rual, J.-F., Zhai, B., Mintseris, J., Vaidya, P., Vaidya, N., … Artavanis-Tsakonas, S. (2011). A protein complex network of Drosophila melanogaster. Cell , 147(3), 690–703. doi: 10.1016/j.cell.2011.08.047.
- Horton, E. R., Byron, A., Askari, J. A., Ng, D. H. J., Millon-Frémillon, A., Robertson, J., … Humphries, M. J. (2015). Definition of a consensus integrin adhesome and its dynamics during adhesion complex assembly and disassembly. Nature Cell Biology , 17, 1577. doi: 10.1038/ncb3257.
- Humphries, J. D., Chastney, M. R., Askari, J. A., & Humphries, M. J. (2019). Signal transduction via integrin adhesion complexes. Current Opinion in Cell Biology , 56, 14–21. doi: 10.1016/j.ceb.2018.08.004.
- Jäger, S., Cimermancic, P., Gulbahce, N., Johnson, J. R., McGovern, K. E., Clarke, S. C., … Krogan, N. J. (2012). Global landscape of HIV-human protein complexes. Nature , 481(7381), 365–370. doi: 10.1038/nature10719.
- Kim, D. I., Jensen, S. C., Noble, K. A., Kc, B., Roux, K. H., Motamedchaboki, K., & Roux, K. J. (2016). An improved smaller biotin ligase for BioID proximity labeling. Molecular Biology of the Cell , 27(8), 1188–1196. doi: 10.1091/mbc.E15-12-0844.
- Kim, D. I., Birendra, K. C, Zhu, W., Motamedchaboki, K., Doye, V., & Roux, K. J. (2014). Probing nuclear pore complex architecture with proximity-dependent biotinylation. Proceedings of the National Academy of Sciences USA , 111(24), E2453–61. doi: 10.1073/pnas.1406459111.
- Knight, J. D. R., Choi, H., Gupta, G. D., Pelletier, L., Raught, B., Nesvizhskii, A. I., & Gingras, A.-C. (2017). ProHits-viz: A suite of web tools for visualizing interaction proteomics data. Nature Methods , 14, 645–646. doi: 10.1038/nmeth.4330.
- Kuo, J.-C., Han, X., 3rd, Yates, J. R., & Waterman, C. M. (2012). Isolation of focal adhesion proteins for biochemical and proteomic analysis. Methods in Molecular Biology , 757, 297–323. doi: 10.1007/978-1-61779-166-6_19.
- Lam, S. S., Martell, J. D., Kamer, K. J., Deerinck, T. J., Ellisman, M. H., Mootha, V. K., & Ting, A. Y. (2015). Directed evolution of APEX2 for electron microscopy and proximity labeling. Nature Methods , 12(1), 51–54. doi: 10.1038/nmeth.3179.
- Maere, S., Heymans, K., & Kuiper, M. (2005). BiNGO: A Cytoscape plugin to assess overrepresentation of gene ontology categories in biological networks. Bioinformatics , 21(16), 3448–3449. doi: 10.1093/bioinformatics/bti551.
- Martell, J. D., Deerinck, T. J., Sancak, Y., Poulos, T. L., Mootha, V. K., Sosinsky, G. E., … Ting, A. Y. (2012). Engineered ascorbate peroxidase as a genetically encoded reporter for electron microscopy. Nature Biotechnology , 30(11), 1143–1148. doi: 10.1038/nbt.2375.
- Ni, D., Xu, P. & Gallagher, S. (2017). Immunoblotting and immunodetection. Current Protocols in Cell Biology , 74, 6.21–6.2.37. doi: 10.1002/cpcb.18.
- Roux, K. J., Kim, D. I., & Burke, B. (2013). BioID: A screen for protein-protein interactions. Current Protocols in Protein Science , 74(1), 19.23.1–19.23.14. doi: 10.1002/0471140864.ps1923s74.
- Roux, K. J., Kim, D. I., Raida, M., & Burke, B. (2012). A promiscuous biotin ligase fusion protein identifies proximal and interacting proteins in mammalian cells. The Journal of Cell Biology , 196(6), 801–810. doi: 10.1083/jcb.201112098.
- Sowa, M. E., Bennett, E. J., Gygi, S. P., & Harper, J. W. (2009). Defining the human deubiquitinating enzyme interaction landscape. Cell , 138(2), 389–403. doi: 10.1016/j.cell.2009.04.042.
- Su, G., Morris, J. H., Demchak, B., & Bader, G. D. (2014). Biological network exploration with cytoscape 3. Current Protocols in Bioinformatics , 47(1), 8.13.1–8.13.24. doi: 10.1002/0471250953.bi0813s47.
- Teo, G., Liu, G., Zhang, J., Nesvizhskii, A. I., Gingras, A.-C., & Choi, H. (2014). SAINTexpress: Improvements and additional features in Significance Analysis of INTeractome software. Journal of Proteomics , 100, 37–43. doi: 10.1016/j.jprot.2013.10.023.
- Van Itallie, C. M., Aponte, A., Tietgens, A. J., Gucek, M., Fredriksson, K., & Anderson, J. M. (2013). The N and C termini of ZO-1 are surrounded by distinct proteins and functional protein networks. The Journal of Biological Chemistry , 288(19), 13775–13788. doi: 10.1074/jbc.M113.466193.
- Winograd-Katz, S. E., Fässler, R., Geiger, B., & Legate, K. R. (2014). The integrin adhesome: From genes and proteins to human disease. Nature Reviews. Molecular Cell Biology , 15(4), 273–288. doi: 10.1038/nrm3769.
- Youn, J.-Y., Dunham, W. H., Hong, S. J., Knight, J. D. R., Bashkurov, M., Chen, G. I., … Gingras, A.-C. (2018). High-density proximity mapping reveals the subcellular organization of mRNA-associated granules and bodies. Molecular Cell , 69(3), 517–532.e11. doi: 10.1016/j.molcel.2017.12.020.
- Yu, G., Wang, L.-G., Han, Y., & He, Q.-Y. (2012). clusterProfiler: An R package for comparing biological themes among gene clusters. OMICS: A Journal of Integrative Biology , 16(5), 284–287. doi: 10.1089/omi.2011.0118.
- Zhang, G., Annan, R. S., Carr, S. A., & Neubert, T. A. (2014). Overview of peptide and protein analysis by mass spectrometry. Current Protocols in Molecular Biology , 108, 10.21.1–10.21.30. doi: 10.1002/0471142727.mb1021s108.
Citing Literature
Number of times cited according to CrossRef: 4
- Frederic Li Mow Chee, Bruno Beernaert, Billie G. C. Griffith, Alexander E. P. Loftus, Yatendra Kumar, Jimi C. Wills, Martin Lee, Jessica Valli, Ann P. Wheeler, J. Douglas Armstrong, Maddy Parsons, Irene M. Leigh, Charlotte M. Proby, Alex von Kriegsheim, Wendy A. Bickmore, Margaret C. Frame, Adam Byron, Mena regulates nesprin-2 to control actin–nuclear lamina associations, trans-nuclear membrane signalling and gene expression, Nature Communications, 10.1038/s41467-023-37021-x, 14 , 1, (2023).
- Grace A. Schaack, Owen M. Sullivan, Andrew Mehle, Identifying Protein‐Protein Interactions by Proximity Biotinylation with AirID and splitAirID, Current Protocols, 10.1002/cpz1.702, 3 , 3, (2023).
- Chengbo Ji, Mili Zhang, Junjie Hu, Can Cao, Qisheng Gu, Youdong Liu, Xu Li, Duogang Xu, Le Ying, Yuqin Yang, Hugh Gao, Jikun Li, Liang Yu, The kinase activity of integrin-linked kinase regulates cellular senescence in gastric cancer, Cell Death & Disease, 10.1038/s41419-022-05020-3, 13 , 7, (2022).
- Everardo Hegewisch-Solloa, Seungmae Seo, Bethany L. Mundy-Bosse, Anjali Mishra, Erik H. Waldman, Sarah Maurrasse, Eli Grunstein, Thomas J. Connors, Aharon G. Freud, Emily M. Mace, Differential Integrin Adhesome Expression Defines Human NK Cell Residency and Developmental Stage, The Journal of Immunology, 10.4049/jimmunol.2100162, 207 , 3, (950-965), (2021).