Influence of Admixture on Phenotypes
Steven W. Brugger, Steven W. Brugger, Mary F. Davis, Mary F. Davis
Abstract
Individuals of European descent have historically been the focus of genetic studies and possess relatively homogenous genomes. As a result, analytical methods have been developed and optimized with such genomes in mind. African-descent and Latino individuals generally possess genomes of greater architectural complexity due to mosaic genomic ancestry, which can extensively and intricately impact phenotypic expression. As such, genetic analyses of admixed individuals require that genetic admixture be quantified to accurately model the impact of genetic variation on phenotypic expression. In this overview, we explore how fundamental genetic concepts such as linkage disequilibrium and differential allele frequency interact with genetic admixture to uniquely influence phenotypes in admixed individuals. © 2023 The Authors. Current Protocols published by Wiley Periodicals LLC.
INTRODUCTION
With genome sequencing technologies continuing to mature and access to them becoming widespread, human research and healthcare-based initiatives that seek to utilize these data are becoming commonplace. As our capacity for data generation and acquisition grows, so does the complexity of the analytical systems required to interpret the data. Genome-wide association (GWA) studies for genetically homogenous populations have seen explosive success in identifying single nucleotide polymorphisms (SNPs) associated with an extensive and varied list of diseases and phenotypes. However, such studies have experienced dramatically reduced success in African-descent and Latino populations, partly due to their substantially more nuanced genetic architecture resulting from genetic admixture (Marchini et al., 2004; Peterson et al., 2019). This makes studies on admixed individuals particularly difficult in practice. Heredity of genetic risk factors, as well as the population from which they originate, can vastly differ, even between individuals descended from the same admixed populations. This overview aims to give the reader insights into genetic mechanisms and characteristics that drive the differential disease risk or altered phenotypic expression observed in genetically admixed populations compared to populations with comparatively lower genetic variability. Said characteristics and mechanisms include global ancestry, effects of linkage disequilibrium, distinct genomic architecture, differential ancestral allele frequency between ancestral populations, and locus-locus or locus-environment interaction.
KEY CONCEPTS
Genetic admixture
Admixture is a well-documented phenomenon in population genetics in which genomically distinct or divergent populations intermix and introduce new lineages (Shriner, 2023). The result is that, over time, individuals that descend from the same group of admixing ancestors possess mosaics of genetic identity unique to each individual due to the imprecise nature of inheritance and recombination (see Fig. 1). Individuals of one particular genomic ancestry can possess unique phenotypes or disease risk when compared to individuals of an alternative genomic ancestry, which has prompted investigations into the genetic determinants of differential risk by ancestry.
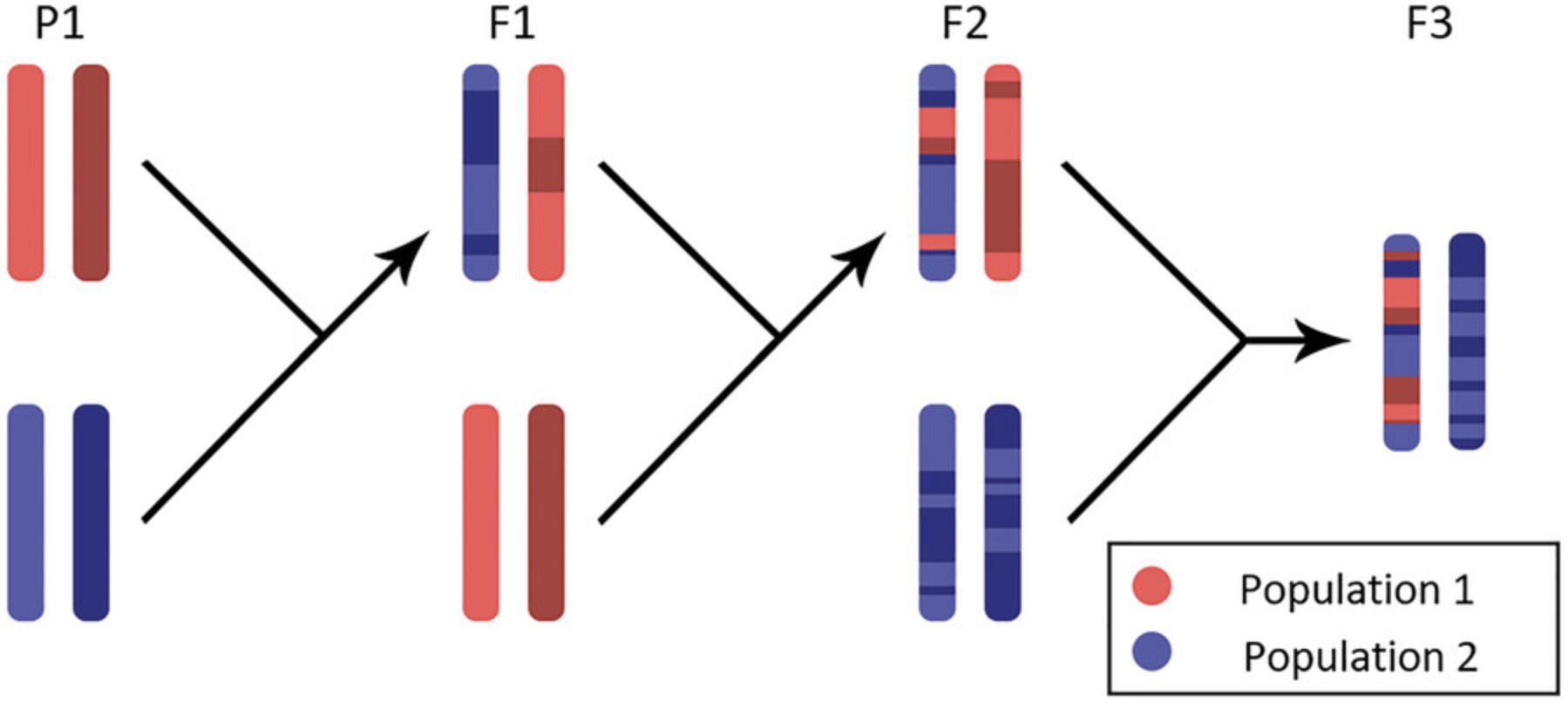
Admixture mapping
Admixture mapping was developed to facilitate genetic analysis of admixed individuals by matching such cohorts against genetically homogenous reference populations to quantify and map the contributions of each to the individual's genome. Admixture mapping estimates the proportional contribution of the reference populations to the overall genome of the individual of all ancestral populations (global ancestry) and/or locus-specific ancestral identity (local ancestry) (Alexander et al., 2009). Admixture mapping provides critical context for statistical analyses and has proven useful in identifying trait-locus relationships that modify phenotypic expression or disease risk solely by virtue of locus ancestry. It offers advantages over strategies used historically to control for genetic ancestry in allelic association tests such as principal components analysis—the results of which are commonly introduced into statistical models as covariates during GWA studies—by allowing researchers to identify traits that statistically associate with genetic regions, the origins of which can be traced to specific ancestral populations. Whereas principal component analyses and their use in contemporary GWA studies attempt to mitigate the effect of genetic ancestry, admixture mapping-informed analyses seek to identify sections of genetic ancestry influencing phenotypes. Approaches to admixture mapping vary widely depending on the populations and phenotypes of interest and have been described in detail elsewhere (Shriner, 2023).
Differential allele frequency
Admixture mapping operates under the limiting assumption that differential allele frequencies (DAF) among ancestral populations drive the observed differences in phenotype or disease risk. Admixture mapping, therefore, cannot detect disease-causing variants whose frequencies are comparable in all ancestral populations relevant to the analysis of interest (Rybicki et al., 2011). This does not imply that DAFs are exclusively responsible for phenotypic discrepancies between populations. Rather, it highlights that admixture mapping is limited in its analytical scope, which must be considered when drawing conclusions.
Linkage disequilibrium
The idea that DAF drives differential risk is underscored by linkage disequilibrium (LD), a phenomenon in which alleles associate non-randomly and may be co-inherited due to close physical proximity within the chromosomes. The identification of admixture signals, particularly in studies designed to test local ancestry estimates directly against a phenotype, is possible due to regional, non-random clustering of alleles whose frequencies similarly follow or deviate from those of provided reference populations, thus creating an associative signal due to LD-based clustering. The extent of LD (Reich et al., 2001) and its patterns at common haplotypes differ among most ancestral populations (Gabriel et al., 2002; Sawyer et al., 2005). An ancestry-associated locus in affected individuals is expected to display an overall greater proportion of alleles originating from the high-risk ancestral population than the unaffected individuals (Palmer et al., 2016). LD serves as the foundation of admixture mapping, specifically that LD arising from recent admixture is more impactful than any potential background admixture of the founding populations (Chakraborty & Weiss, 1988; Rife, 1954; Shriner et al., 2011).
LD is an especially important principle in admixture mapping, given that genotyping data are generally more practical and widely available than whole-genome data. Using genotyping data introduces the possibility that researchers identify a “tagging” variant as significant rather than the true causal variant, as genotyped SNPs are taken to be representative of other SNPs in the region (Carlson et al., 2004). While this does not automatically diminish the significance of a result, it does require that researchers be conscious of the local LD landscape when interpreting results.
INFLUENCE OF ADMIXTURE ON PHENOTYPES
Admixture mapping-based association studies report associations of phenotypes with SNPs, genes, or genomic regions unique to a distinct ancestral population, whose likelihood of association is ultimately determined by overall genomic characteristics and the forces that shape them. In the context of admixture mapping, these consist of 1) global ancestry, 2) effects of LD, 3) identification of distinct genomic architecture, 4) DAF, and 5) locus-locus or locus-environment interaction. Ascribing results to one of these categories is not straightforward, as it depends on the depth and breadth of published knowledge on the phenotype from which conclusions may be drawn. In some instances, authors report variant associations and draw credible conclusions regarding verification of DAF between study populations, effects on introns, exons, or gene regulatory elements, locus-locus or locus-environment interactions, effects on gene pathways or interacting gene networks, etc. Others may only speculate on the existence of causal variants in a relatively broad genomic region. Some report results relevant to each of these categories, while others are limited to one, which may be attributed as much to the nature of the phenotype as to the study's design.
Global ancestry
Studies focusing specifically on global ancestry proportions highlight future research candidates by performing analyses designed to probe for associations between global ancestry proportions and a phenotype. While these do not investigate direct trait-locus relationships, they have demonstrated their utility in screening phenotypes for evidence of a link to genomic ancestry and help establish a justification for further investigation. Many of the more detailed studies performed such foundational analyses before admixture mapping.
For example, a psychiatric study of antidepressant medication efficacy reported that increasing proportions of African genomic ancestry (AGA) are associated with poor treatment response in a sample of African American patients (Murphy et al., 2013). The effect of genomic ancestry on treatment response was speculated to be driven by allele frequency differences in common variants, though this was not specifically investigated. Other investigations, however, successfully identified a variant in HTR2A that was associated with better treatment response but over six times less prevalent in African Americans compared to European Americans (McMahon et al., 2006). The results show that 1) AGA is associated with antidepressant response, and 2) an identified variant with a substantial ancestry-associated disparity in allele frequency influences differential treatment outcomes, highlighting the iterative and evolutionary nature of admixture-informed genetic studies as they advance from a hypothesis to a potential association with clinical applications.
Effects of linkage disequilibrium
LD is an important genomic characteristic to consider, conceptually as it relates to admixture mapping and practically as it influences the interpretation of results. As stated previously, analysis of genotyping data requires careful consideration of results to avoid misattributing significance to a SNP that tags a potentially causal variant that was not genotyped. It is also possible that an association at a singular genotyped locus obfuscates the existence of multiple contributing SNPs in the region. While it is certainly possible that an identified SNP is a causal variant, care must be taken to ensure that any suspicions of its identity as a tagging or proxy SNP are appropriately disclosed if not thoroughly investigated.
Sifting proxy from causal SNPs may not be straightforward in complex phenotypes with multiple contributing genetic risk alleles. For example, a recent study on glioma risk in African American and Latino patients reported two risk-imparting genomic regions associated with increased European genomic ancestry (EGA) and one with decreased EGA (Ostrom et al., 2020). A deeper investigation into these and other known regions implied the contribution of multiple population-specific SNPs tagging at least one causal variant that increases glioma risk. The direction of effect of one identified SNP (rs730437) was opposite of that previously reported, leaving the authors to conclude that the SNP, which was reported as causal in previous GWA studies, may not truly be causal but tag other causal variants that vary according to ancestral group due to LD and allele frequency patterns. As stated previously, the extent of LD depends on the population (Reich et al., 2001); as such, the range of genomic regions may be refined for future analysis. For example, nicotine dependence in African American individuals is associated with SNP rs910083, which is in high LD with other SNPs in the region [15]. The LD window for participants with local EGA spanned three genes (COMM7 , DNMT3B , and MAPRE1), while the LD window for participants with local AGA spanned a single gene (DNMT3B) (Hancock et al., 2018).
One of the drawbacks to admixture mapping with genotyping panels is the possibility that causal variants are not directly assayed by particular arrays, precluding researchers from drawing firm conclusions. In a cohort of African American individuals, associations between asthma status and chromosomes 6p21 and 17q21 were reportedly driven by untyped variation, likely because 6p21 maps to the highly variable major histocompatibility complex (MHC) region of chromosome 6. In another study, admixture- and fine-mapping analyses showed associations of seven SNPs within CHKA with type 2 diabetes. The authors advised careful interpretation of the results, as fine-mapping relied on genotyping a limited number of tagSNPs, increasing the likelihood that rare variants were not captured in the data, as well as the possibility that causal variants may not be tagged by the genotyped tagSNPs (Jeff et al., 2014).
Distinct genomic architecture
Bridging the concepts of LD and DAF is that of distinct or unique genomic architecture at phenotypic loci. While most admixture mapping studies report results consistent with the method's intended use, many have identified examples of genomic characteristics observed only in groups of a particular ancestry. Through the random process of recombination and the occurrence of novel mutative events due to specific environmental or other selective pressures, unique genetic traits are expected to arise within distinct populations to deliver measurable effects on differential phenotype risk.
An increased proportion of global AGA is argued to be protective against the neuropathology of Alzheimer's disease (Schlesinger et al., 2013). Additional studies have corroborated this claim and added that local AGA of the ApoE ε4 allele confers a lower risk than is typical for those of EGA of the same locus across all tested populations (Rajabli et al., 2018). Similarly, associations at chromosome 17p13.2 indicate that EGA in African American individuals confers greater risk, while AGA reduces risk (Rajabli et al., 2022). The effect is proposed to stem from regulatory differences arising from mutations in non-protein-coding regions. This same phenomenon was discussed in a study of Caribbean Hispanics, for whom AGA at several ApoE loci was associated with a 39% reduction in risk compared to participants with EGA at the same loci; further, controlling for ApoE ε2/ε3/ε4 genotypes did not sufficiently explain this result and implies that other genetic factors underpin this observation (Blue et al., 2019). Sequence context around ApoE is variable by population (Babenko et al., 2018; Fullerton et al., 2000) and ancestry-specific coding changes exist within ApoE (Fullerton et al., 2000; Radwan et al., 2014), substantiating the claim that ApoE genotype alone is insufficient to explain Alzheimer's disease risk. Lastly, in a cohort of Peruvian participants, local Indigenous American genomic ancestry at the ApoE ε4 allele is associated with an increased risk of Alzheimer's disease. However, the authors note that this is contrary to previous studies that found a reduction in risk (Marca-Ysabel et al., 2021). Whatever the true effect of Indigenous American genomic ancestry on Alzheimer's risk is, it is clear that elements of the genetic architecture surrounding ApoE exert substantial influence on differential risk that is not attributable to DAF alone.
Interestingly, SNP associations with phenotypes have been identified in which genomic architecture is profoundly divergent, specifically in the form of missing alleles. One such SNP, rs148966337, was associated with breast cancer, but the risk allele was only present in African American women and was absent in all other women of non-African genetic ancestries in the study (Ruiz-Narvaez et al., 2016).
An intriguing report detected an association between ocular refraction and local EGA at the 7p5 locus in a cohort of 96 African American families (Ciner et al., 2008). The signal was found to have derived from families with high levels of European ancestry, and it was speculated that this genomic region may harbor variants that variably modify risk in different populations. The association was not population-specific, suggesting that the causal allele(s) originated uniquely in European lineages.
Differential ancestral allele frequency
DAFs between ancestral populations suspected to drive alternative phenotypic expression have been described for many of the phenotypes mentioned above. A fine-mapping analysis found that SNP rs13030825 within GALNT13 explains the majority of the identified admixture signal in chromosome 2q23.3–24.2, tying local AGA to longer bone length in African Americans (Tekola-Ayele et al., 2021). The identified SNP is nearly ubiquitous in the 1000 Genomes African-representative population (0.99), but less than half as prevalent in the European-representative population (0.42) and presents strong evidence for the influence of differing ancestral allele frequencies on a phenotype. An admixture mapping analysis in prostate cancer identified a single SNP near RACGAP1 that disrupts the recombination signal binding protein with the immunoglobulin kappa J binding site, which may contribute to unique prostate cancer risk in African American men (Wilson et al., 2018). Although the authors did not report a DAF for this SNP, another study reported a substantial difference in allele frequency between African and European reference populations (0.67 vs. 0.06, respectively) (Ricks-Santi et al., 2012). It was suggested that this SNP, along with seven others, may explain the increased susceptibility to a more aggressive type of prostate cancer at an earlier age in African American compared to EGA men.
One of the strongest examples of differential risk by ancestry is found in multiple sclerosis. Investigating the MHC region of chromosome 6, as it relates to multiple sclerosis symptomatology and severity, established that at least one copy of the human leukocyte antigen (HLA) allele HLA-DRB1*15 is present in 53.3% of European patients and 37.6% of African American patients (Cree et al., 2009). Participants with this allele were demonstrably more likely to exhibit a classical multiple sclerosis disease course—as opposed to the opticospinal subtype—which is consistent with observations that African American patients with multiple sclerosis present with the latter at disproportionately increased rates compared to patients of European descent (Cree et al., 2004). In their analyses, the authors determined that African ancestry is not independently associated with the opticospinal subtype and concluded that the increased prevalence of opticospinal multiple sclerosis in African Americans is the result of the reduced frequency of the HLA-DRB1*15 allele in this population. AGA at HLA alleles was further tested against clinical outcomes, revealing that having alleles of African origin is positively associated with 1) multiple sclerosis-induced disability as measured by the Multiple Sclerosis Severity Score and 2) risk of cane dependency for ambulation. Taken together, these results indicate that differential frequencies at key MHC alleles likely account for the distinct phenotypic expression and augmented disease severity for African American patients with multiple sclerosis compared to patients of European descent.
Locus-locus or locus-environment interaction
Once alleles have been verifiably associated with a disease or phenotype with demonstrably different frequencies between distinct genetic ancestry groups, admixture mapping may be considered successful. While identifying these alleles is beneficial and informative in numerous cases, it is not always sufficient to explain how ancestry influences risk. Many phenotypes at the center of admixture mapping analyses are genetically complex and commonly influenced by other loci or external agents that can have a substantial direct and indirect effect on overall risk. Given that admixture and ancestral allele frequencies contribute to risk, it is conceivable that some implicated SNP, haplotype, or gene interacts with other factors to modify risk beyond what genetics control directly.
Neurological disorders exemplify this concept well. The HLA-DRB1*15:01 allele is associated with multiple sclerosis risk, but the degree of risk is dependent on ancestry, with the European haplotype conferring three times greater risk than the African haplotype, and additional—albeit lesser—risk is conferred in the same manner for HLA-B*07:02 and HLA-A*03:01 (Chi et al., 2019). It was suggested that disease-causing variants within these alleles might originate in only one ancestral population. However, this may not always be the case, as the African haplotype for HLA-DRB1*15:03 confers more risk than its European counterpart. Specific gene sets within the MHC, interpreted as a mosaic of ancestral origins for several HLA alleles, may dramatically alter risk as a host of variants within them likely work synergistically and antagonistically to shape overall risk for an individual. This is also observed in Alzheimer's disease. Researchers identified a novel locus of AGA (19q13.31) that reduces risk by approximately 75% for individuals with an ε4/ε4 ApoE genotype (Rajabli et al., 2022). This claim is supported by a recent publication demonstrating through single-nucleus RNA analysis that individuals of EGA express ApoE more highly than individuals of AGA and likely indicates that regulation of ApoE expression is ancestrally mediated (Griswold et al., 2021). Combined with the results discussed above, these lend credibility to the idea that complex SNP and/or gene interactions (including regulation thereof) somewhat depend on genomic ancestry.
SNPs rs1800796 and rs8192284 are associated with bronchodilator response in Puerto Rican and African American patients with asthma, but the risk alleles (C, both variants) interact variably with local ancestry to substantially impact bronchodilator efficacy. Study participants with local Indigenous American genomic ancestry experience increased efficacy, while participants with local EGA experience decreased efficacy (Corvol et al., 2009). Analyses of both gene- and SNP-based interactions in patients with asthma revealed that genotype combinations may be useful in identifying individuals at a higher risk for asthma and that the true effect of some genes on risk may not be clear unless considered in tandem with the effect of other genes (Baye et al., 2011). Despite the identification of several novel, ancestry-linked asthma risk loci, it has also been proposed that decreased lung function in African American individuals is subject to gene-environment interactions to shape an individual's overall risk (Parker et al., 2014). To speak more to environmental interactions, a range of SNPs across immune genes IL12RB1 , IL4 , IL15 , and TGFB1 have been proposed to function in tandem to influence breast cancer risk in African American women (Quan et al., 2014). Inquiries into epidemiological or evolutionary influences on genetics-based breast cancer risk revealed that allele frequencies within immune genes might exhibit differences between AGA versus EGA individuals due to pressure from malaria (Barreiro & Quintana-Murci, 2010; Kwiatkowski, 2005) and differences in immune function between these two groups is related to the historical exposure of African populations to parasitic worms (Anthony et al., 2007). Taken together, these studies suggest that the interaction of genes and the DAF of key variants substantially modifies breast cancer risk for African American women.
CONCLUDING REMARKS
Given the popularity and ubiquity of GWA studies in contemporary genetics research, much of the available genetic information exists as genotypes rather than entire sequences, as previously discussed. While genotyping data has certainly demonstrated its value and power in detecting common variation, it is inherently limited in its research applications, given that it represents a snapshot, rather than a complete picture, of an individual's genome. One of the major pitfalls of admixture mapping as a technique is that contemporary studies frequently use this limited genotyping data to infer ancestry and identify disease or phenotype associations. Recall that it is not rare for a study to report that a lead SNP is in high LD with other SNPs and may not be causal, concluding that the causal variant is not yet known and should be the focus of future research. To remedy this, many studies employ imputation to fill in the gaps between genotyping results and produce pseudo-whole-genome data. While this practice can be beneficial in most genetic association studies, special considerations must be made in the case of admixture, as admixture mapping operates on the principle of LD and identifiable patterns of clustering and frequency of variants within certain ancestral genomes. Special attention must be paid to the reference populations used to maximize the odds of high-fidelity imputation (Liu et al., 2013). As most studies describe a fine-mapping endeavor following admixture mapping analyses to pinpoint all relevant variants, high-quality genotyping or imputation is imperative. Poorly genotyped or imputed data may preclude researchers from conclusively identifying causal variation despite indications of biological, statistical, or other evidence for the variant's association. As whole-genome sequence data becomes increasingly available and substantially less expensive, future analyses may consider targeted sequencing of associative peaks identified by admixture mapping to overcome biases inherent to genotyped or imputed data.
In some—perhaps most—cases, positively identifying a causal variant with verification that its allele frequency differs between contributing ancestral groups signifies the culmination of an admixture mapping endeavor. With the field of genetics constantly maturing and its scope encompassing a staggering quantity of diseases and phenotypes, validating the biological relevance of significant results and verifying observed allele frequencies across various ancestral populations is becoming increasingly trivial. As a result, it is common for admixture mapping studies to report ancestral allele frequencies for a discovered variant and implicate the role of said discrepancy in shaping differential risk. This information is shared as it relates to the observed intra-cohort or reference population allele frequencies—no instances of reference to outside databases, such as dbSNP (Sherry et al., 2001), to verify potential discrepancies were noted. As admixture mapping analyses become more commonplace, such references might be encouraged to highlight cases in which observed allele frequency differences in the study population are (or are not) congruent with frequencies reported generally, which may serve to strengthen or weaken the proposition of differential phenotype or disease risk as the outcome of ancestry-specific allele frequencies in each specific case. Nevertheless, the abundance of publications claiming associations between phenotypes and SNPs/haplotypes with corresponding differences in ancestral allele frequencies suggests that publicly accessible databases would uphold a discrepancy discovered in a well-designed and carefully executed admixture mapping analysis, even if the precise frequencies reported vary. Finally, future studies may consider performing admixture mapping even on European-descent individuals. Subcontinental admixture in European and European American individuals has recently been shown to influence height, LDL cholesterol, and body mass index associations with LCT , the gene responsible for human lactase production (Gouveia et al., 2023). It is clear that our current understanding of the influence of genetic admixture on phenotypes is incomplete, and further exploration of its effects in any population is warranted.
In summary (see Fig. 2), many of the studies described above suggest that variants significantly associated with the phenotypes in question are in LD with other SNPs, some of which may contribute to the observed phenotypes or possibly be causal variants that the association simply tags. This is to say that studies reporting the tagging of a suspected causal variant through the identified association may be viewed as a “first step” toward classifying the role of the variant(s) in the studied phenotype. Once a potential locus is identified, fine mapping of the region can proceed in an effort to identify ancestry-specific genetics that may contribute to the phenotype. This usually generates a list of differentially observed candidate SNPs that map to genes that, in turn, may be differentially expressed. Differential expression within one gene can itself be enough to alter phenotypes or disease risk, but it may also influence the expression of other genes in a related pathway, depending on the nature of the variation and the disease. It is not likely that differing ancestral origins of identical alleles are sufficient to produce divergent phenotypes; of course, other extra-genomic risk factors can modify risk and may be incorporated into admixture mapping-based inquiries. However, the influence of ancestry on phenotypes or diseases specifically is narrow. It is expressed within the observation that allele frequencies of a single allele differ between ancestral populations or that an allele associated with a disease or phenotype is in high LD with the true causal variant(s). Other effects or interactions are observed but are derivative of the fundamental concepts of LD and DAF that enable admixture mapping-based analyses.

AUTHOR CONTRIBUTIONS
Steven W. Brugger : Conceptualization; investigation; writing original draft; writing review and editing. Mary F. Davis : Conceptualization; resources; supervision; writing review and editing.
CONFLICT OF INTEREST
The authors declare no conflict of interest.
Open Research
DATA AVAILABILITY STATEMENT
Data sharing not applicable to this article as no datasets were generated or analyzed during the current study.
LITERATURE CITED
- Alexander, D. H., Novembre, J., & Lange, K. (2009). Fast model-based estimation of ancestry in unrelated individuals. Genome Research , 19(9), 1655–1664. https://doi.org/10.1101/gr.094052.109
- Anthony, R. M., Rutitzky, L. I., Urban, J. F., Stadecker, M. J., & Gause, W. C. (2007). Protective immune mechanisms in helminth infection. Nature Reviews Immunology , 7(12), 975–987. https://doi.org/10.1038/nri2199
- Babenko, V. N., Afonnikov, D. A., Ignatieva, E. V., Klimov, A. V., Gusev, F. E., & Rogaev, E. I. (2018). Haplotype analysis of APOE intragenic SNPs. BMC Neuroscience , 19, 16. https://doi.org/10.1186/s12868-018-0413-4
- Barreiro, L. B., & Quintana-Murci, L. (2010). From evolutionary genetics to human immunology: How selection shapes host defence genes. Nature Reviews Genetics , 11(1), 17–30. https://doi.org/10.1038/nrg2698
- Baye, T. M., Kovacic, M. B., Myers, J. M. B., Martin, L. J., Lindsey, M., Patterson, T. L., He, H., Ericksen, M. B., Gupta, J., Tsoras, A. M., Lindsley, A., Rothenberg, M. E., Wills-Karp, M., Eissa, N. T., Borish, L., & Hershey, G. K. K. (2011). Differences in candidate gene association between European Ancestry and African American asthmatic children. PLoS ONE , 6(2), e16522. https://doi.org/10.1371/journal.pone.0016522
- Blue, E. E., Horimoto, A. R. V. R., Mukherjee, S., Wijsman, E. M., & Thornton, T. A. (2019). Local ancestry at APOE modifies Alzheimer's disease risk in Caribbean Hispanics. Alzheimers & Dementia, 15(12), 1524–1532. https://doi.org/10.1016/j.jalz.2019.07.016
- Carlson, C. S., Eberle, M. A., Rieder, M. J., Yi, Q., Kruglyak, L., & Nickerson, D. A. (2004). Selecting a maximally informative set of single-nucleotide polymorphisms for association analyses using linkage disequilibrium. American Journal of Human Genetics , 74(1), 106–120. https://doi.org/10.1086/381000
- Chakraborty, R., & Weiss, K. M. (1988). Admixture as a tool for finding linked genes and detecting that difference from allelic association between loci. Proceedings of the National Academy of Sciences of the United States of America , 85(23), 9119–9123. https://doi.org/10.1073/pnas.85.23.9119
- Chi, C., Shao, X., Rhead, B., Gonzales, E., Smith, J. B., Xiang, A. H., Graves, J., Waldman, A., Lotze, T., Schreiner, T., Weinstock-Guttman, B., Aaen, G., Tillema, J. M., Ness, J., Candee, M., Krupp, L., Gorman, M., Benson, L., Chitnis, T., … Barcellos, L. F. (2019). Admixture mapping reveals evidence of differential multiple sclerosis risk by genetic ancestry. Plos Genetics , 15(1), e1007808. https://doi.org/10.1371/journal.pgen.1007808
- Ciner, E., Wojciechowski, R., Ibay, G., Bailey-Wilson, J. E., & Stambolian, D. (2008). Genomewide scan of ocular refraction in African-American families shows significant linkage to chromosome 7p15. Genetic Epidemiology , 32(5), 454–463. https://doi.org/10.1002/gepi.20318
- Corvol, H., De Giacomo, A., Eng, C., Seibold, M., Ziv, E., Chapela, R., Rodriguez-Santana, J. R., Rodriguez-Cintron, W., Thyne, S., Watson, H. G., Meade, K., LeNoir, M., Avila, P. C., Choudhry, S., Burchard, E. G., Study, G., & En, S. A. A. A. G. (2009). Genetic ancestry modifies pharmacogenetic gene-gene interaction for asthma. Pharmacogenetics and Genomics , 19(7), 489–496. https://doi.org/10.1097/FPC.0b013e32832c440e
- Cree, B. A., Khan, O., Bourdette, D., Goodin, D. S., Cohen, J. A., Marrie, R. A., Glidden, D., Weinstock-Guttman, B., Reich, D., Patterson, N., Haines, J. L., Pericak-Vance, M., DeLoa, C., Oksenberg, J. R., & Hauser, S. L. (2004). Clinical characteristics of African Americans vs Caucasian Americans with multiple sclerosis. Neurology , 63(11), 2039–2045. https://doi.org/10.1212/01.wnl.0000145762.60562.5d
- Cree, B. A. C., Reich, D. E., Khan, O., De Jager, P. L., Nakashima, I., Takahashi, T., Bar-Or, A., Tong, C., Hauser, S. L., & Oksenberg, J. R. (2009). Modification of Multiple Sclerosis Phenotypes by African Ancestry at HLA. Archives of Neurology , 66(2), 226–233. https://doi.org/10.1001/archneurol.2008.541
- Fullerton, S. M., Clark, A. G., Weiss, K. M., Nickerson, D. A., Taylor, S. L., Stengard, J. H., Salomaa, V., Vartiainen, E., Perola, M., Boerwinkle, E., & Sing, C. F. (2000). Apolipoprotein E variation at the sequence haplotype level: Implications for the origin and maintenance of a major human polymorphism. American Journal of Human Genetics , 67(4), 881–900. https://doi.org/10.1086/303070
- Gabriel, S. B., Schaffner, S. F., Nguyen, H., Moore, J. M., Roy, J., Blumenstiel, B., Higgins, J., DeFelice, M., Lochner, A., Faggart, M., Liu-Cordero, S. N., Rotimi, C., Adeyemo, A., Cooper, R., Ward, R., Lander, E. S., Daly, M. J., & Altshuler, D. (2002). The structure of haplotype blocks in the human genome. Science , 296(5576), 2225–2229. https://doi.org/10.1126/science.1069424
- Gouveia, M. H., Bentley, A. R., Leal, T. P., Tarazona-Santos, E., Bustamante, C. D., Adeyemo, A. A., Rotimi, C. N., & Shriner, D. (2023). Unappreciated subcontinental admixture in Europeans and European Americans and implications for genetic epidemiology studies. Nature Communications , 14(1), 6802. https://doi.org/10.1038/s41467-023-42491-0
- Griswold, A. J., Celis, K., Bussies, P. L., Rajabli, F., Whitehead, P. L., Hamilton-Nelson, K. L., Beecham, G. W., Dykxhoorn, D. M., Nuytemans, K., Wang, L. Y., Gardner, O. K., Dorfsman, D. A., Bigio, E. H., Mesulam, M. M., Weintraub, S., Geula, C., Gearing, M., McGrath-Martinez, E., Dalgard, C. L., … Vance, J. M. (2021). Increased APOE epsilon 4 expression is associated with the difference in Alzheimer's disease risk from diverse ancestral backgrounds. Alzheimers & Dementia, 17(7), 1179–1188. https://doi.org/10.1002/alz.12287
- Hancock, D. B., Guo, Y., Reginsson, G. W., Gaddis, N. C., Lutz, S. M., Sherva, R., Loukola, A., Minica, C. C., Markunas, C. A., Han, Y., Young, K. A., Gudbjartsson, D. F., Gu, F., McNeil, D. W., Qaiser, B., Glasheen, C., Olson, S., Landi, M. T., Madden, P. A. F., … Johnson, E. O. (2018). Genome-wide association study across European and African American ancestries identifies a SNP in DNMT3B contributing to nicotine dependence. Molecular Psychiatry , 23(9), 1911–1919. https://doi.org/10.1038/mp.2017.193
- Jeff, J. M., Armstrong, L. L., Ritchie, M. D., Denny, J. C., Kho, A. N., Basford, M. A., Wolf, W. A., Pacheco, J. A., Li, R., Chisholm, R. L., Roden, D. M., Hayes, M. G., & Crawford, D. C. (2014). Admixture mapping and subsequent fine-mapping suggests a biologically relevant and novel association on chromosome 11 for type 2 diabetes in African Americans. PLoS ONE , 9(3), e86931. https://doi.org/10.1371/journal.pone.0086931
- Kwiatkowski, D. P. (2005). How malaria has affected the human genome and what human genetics can teach us about malaria. American Journal of Human Genetics , 77(2), 171–192. https://doi.org/10.1086/432519
- Liu, E. Y., Li, M. Y., Wang, W., & Li, Y. (2013). MaCH-Admix: Genotype imputation for admixed populations. Genetic Epidemiology , 37(1), 25–37. https://doi.org/10.1002/gepi.21690
- Marca-Ysabel, M. V., Rajabli, F., Cornejo-Olivas, M., Whitehead, P. G., Hofmann, N. K., Manrique, M. Z. I., Otani, D. M. V., Neyra, A. K. M., Castro-Souarez, S., Meza-Vega, M., Adams, L. D., Mena, P. R., Rosario, I., Cuccaro, M. L., Vance, J. M., Beecham, G. W., Custodio, N., Montesinos, R., Soler, P. E. M., & Pericak-Vance, M. A. (2021). Dissecting the role of Amerindian genetic ancestry and the ApoE epsilon 4 allele on Alzheimer disease in an admixed Peruvian population. Neurobiology of Aging , 101, 298.e11–298.e15. https://doi.org/10.1016/j.neurobiolaging.2020.10.003
- Marchini, J., Cardon, L. R., Phillips, M. S., & Donnelly, P. (2004). The effects of human population structure on large genetic association studies. Nature Genetics , 36(5), 512–517. https://doi.org/10.1038/ng1337
- McMahon, F. J., Buervenich, S., Charney, D., Lipsky, R., Rush, A. J., Wilson, A. F., Sorant, A. J. M., Papanicolaou, G. J., Laje, G., Fava, M., Trivedi, M. H., Wisniewski, S. R., & Manji, H. (2006). Variation in the gene encoding the serotonin 2A receptor is associated with outcome of antidepressant treatment. American Journal of Human Genetics , 78(5), 804–814. https://doi.org/10.1086/503820
- Murphy, E., Hou, L. P., Maher, B. S., Woldehawariat, G., Kassem, L., Akula, N., Laje, G., & McMahon, F. J. (2013). Race, genetic ancestry and response to antidepressant treatment for major depression. Neuropsychopharmacology , 38(13), 2598–2606. https://doi.org/10.1038/npp.2013.166
- Ostrom, Q. T., Egan, K. M., Nabors, L. B., Gerke, T., Thompson, R. C., Olson, J. J., LaRocca, R., Chowdhary, S., Eckel-Passow, J. E., Armstrong, G., Wiencke, J. K., Bernstein, J. L., Claus, E. B., Il'yasova, D., Johansen, C., Lachance, D. H., Lai, R. K., Merrell, R. T., Olson, S. H., … Bondy, M. L. (2020). Glioma risk associated with extent of estimated European genetic ancestry in African Americans and Hispanics. International Journal of Cancer , 146(3), 739–748. https://doi.org/10.1002/ijc.32318
- Palmer, N. D., Divers, J., Lu, L. Y., Register, T. C., Carr, J. J., Hicks, P. J., Smith, S. C., Xu, J. Z., Judd, S. E., Irvin, M. R., Gutierrez, O. M., Bowden, D. W., Wagenknecht, L. E., Langefeld, C. D., & Freedman, B. I. (2016). Admixture mapping of serum vitamin D and parathyroid hormone concentrations in the African American-Diabetes Heart Study. Bone , 87, 71–77. https://doi.org/10.1016/j.bone.2016.01.028
- Parker, M. M., Foreman, M. G., Abel, H. J., Mathias, R. A., Hetmanski, J. B., Crapo, J. D., Silverman, E. K., Beaty, T. H., & Investigators, C. O. (2014). Admixture mapping identifies a quantitative trait locus associated with FEV1/FVC in the COPDGene Study. Genetic Epidemiology , 38(7), 652–659. https://doi.org/10.1002/gepi.21847
- Peterson, R. E., Kuchenbaecker, K., Walters, R. K., Chen, C.-Y., Popejoy, A. B., Periyasamy, S., Lam, M., Iyegbe, C., Strawbridge, R. J., Brick, L., Carey, C. E., Martin, A. R., Meyers, J. L., Su, J., Chen, J., Edwards, A. C., Kalungi, A., Koen, N., Majara, L., … Duncan, L. E. (2019). Genome-wide Association Studies in ancestrally diverse populations: Opportunities, methods, pitfalls, and recommendations. Cell , 179(3), 589–603. https://doi.org/10.1016/j.cell.2019.08.051
- Quan, L., Gong, Z. H., Yao, S., Bandera, E. V., Zirpoli, G., Hwang, H., Roberts, M., Ciupak, G., Davis, W., Sucheston, L., Pawlish, K., Bovbjerg, D. H., Jandorf, L., Cabasag, C., Coignet, J. G., Ambrosone, C. B., & Hong, C. C. (2014). Cytokine and cytokine receptor genes of the adaptive immune response are differentially associated with breast cancer risk in American women of African and European ancestry. International Journal of Cancer , 134(6), 1408–1421. https://doi.org/10.1002/ijc.28458
- Radwan, Z. H., Wang, X. B., Waqar, F., Pirim, D., Niemsiri, V., Hokanson, J. E., Hamman, R. F., Bunker, C. H., Barmada, M. M., Demirci, F. Y., & Kamboh, M. I. (2014). Comprehensive evaluation of the association of APOE genetic variation with plasma lipoprotein traits in US Whites and African Blacks. PLoS ONE , 9(12), e114618. https://doi.org/10.1371/journal.pone.0114618
- Rajabli, F., Beecham, G. W., Hendrie, H. C., Baiyewu, O., Ogunniyi, A., Gao, S. J., Kushch, N. A., Lipkin-Vasquez, M., Hamilton-Nelson, K. L., Young, J. I., Dykxhoorn, D. M., Nuytemans, K., Kunkle, B. W., Wang, L. Y., Jin, F., Liu, X. X., Feliciano-Astacio, B. E., Schellenberg, G. D., Dalgard, C. L., … Consortium, A. D. G. (2022). A locus at 19q13.31 significantly reduces the ApoE epsilon 4 risk for Alzheimer's Disease in African Ancestry. Plos Genetics , 18(7), e1009977. https://doi.org/10.1371/journal.pgen.1009977
- Rajabli, F., Feliciano, B. E., Celis, K., Hamilton-Nelson, K. L., Whitehead, P. L., Adams, L. D., Bussies, P. L., Manrique, C. P., Rodriguez, A., Rodriguez, V., Starks, T., Byfield, G. E., Lopez, C. B. S., McCauley, J. L., Acosta, H., Chineaid, A., Kunkle, B. W., Reitz, C., Farrer, L. A., … Pericak-Vance, M. A. (2018). Ancestral origin of ApoE epsilon 4 Alzheimer disease risk in Puerto Rican and African American populations. Plos Genetics , 14(12), e1007791. https://doi.org/10.1371/journal.pgen.1007791
- Rajabli, F., Tosto, G., Hamilton-Nelson, K. L., Kunkle, B. W., Vardarajan, B. N., Naj, A., Whitehead, P. G., Gardner, O. K., Bush, W. S., Sariya, S., Mayeux, R. P., Farrer, L. A., Cuccaro, M. L., Vance, J. M., Griswold, A. J., Schellenberg, G. D., Haines, J. L., Byrd, G. S., Reitz, C., … Martin, E. R. (2022). Admixture mapping identifies novel Alzheimer's disease risk regions in African Americans. Alzheimers & Dementia, 19, 2538–2548. https://doi.org/10.1002/alz.12865
- Reich, D. E., Cargill, M., Bolk, S., Ireland, J., Sabeti, P. C., Richter, D. J., Lavery, T., Kouyoumjian, R., Farhadian, S. F., Ward, R., & Lander, E. S. (2001). Linkage disequilibrium in the human genome. Nature , 411(6834), 199–204. https://doi.org/10.1038/35075590
- Ricks-Santi, L. J., Apprey, V., Mason, T., Wilson, B., Abbas, M., Hernandez, W., Hooker, S., Doura, M., Bonney, G., Dunston, G., Kittles, R., & Ahaghotu, C. (2012). Identification of genetic risk associated with prostate cancer using ancestry informative markers. Prostate Cancer and Prostatic Diseases , 15(4), 359–364. https://doi.org/10.1038/pcan.2012.19
- Rife, D. C. (1954). Populations of hybrid origin as source material for the detection of linkage. American Journal of Human Genetics , 6(1), 26–33.
- Ruiz-Narvaez, E. A., Sucheston-Campbell, L., Bensen, J. T., Yao, S., Haddad, S., Haiman, C. A., Bandera, E. V., John, E. M., Bernstein, L., Hu, J. J., Ziegler, R. G., Deming, S. L., Olshan, A. F., Ambrosone, C. B., Palmer, J. R., & Lunetta, K. L. (2016). Admixture mapping of African-American Women in the AMBER Consortium Identifies New Loci for Breast Cancer and Estrogen-Receptor Subtypes. Frontiers in Genetics , 7, 170. https://doi.org/10.3389/fgene.2016.00170
- Rybicki, B. A., Levin, A. M., McKeigue, P., Datta, I., Gray-McGuire, C., Colombo, M., Reich, D., Burke, R. R., & Iannuzzi, M. C. (2011). A genome-wide admixture scan for ancestry-linked genes predisposing to sarcoidosis in African-Americans. Genes and Immunity , 12(2), 67–77. https://doi.org/10.1038/gene.2010.56
- Sawyer, S. L., Mukherjee, N., Pakstis, A. J., Feuk, L., Kidd, J. R., Brookes, A. J., & Kidd, K. K. (2005). Linkage disequilibrium patterns vary substantially among populations. European Journal of Human Genetics , 13(5), 677–686. https://doi.org/10.1038/sj.ejhg.5201368
- Schlesinger, D., Grinberg, L. T., Alba, J. G., Naslavsky, M. S., Licinio, L., Farfel, J. M., Suemoto, C. K., Ferretti, R. E. D., Leite, R. E. P., de Andrade, M. P., dos Santos, A. C. F., Brentani, H., Pasqualucci, C. A., Nitrini, R., Jacob, W., Zatz, M., & Grp, B. A. B. S. (2013). African ancestry protects against Alzheimer's disease-related neuropathology. Molecular Psychiatry , 18(1), 79–85. https://doi.org/10.1038/mp.2011.136
- Sherry, S. T., Ward, M. H., Kholodov, M., Baker, J., Phan, L., Smigielski, E. M., & Sirotkin, K. (2001). dbSNP: The NCBI database of genetic variation. Nucleic Acids Research , 29(1), 308–311. https://doi.org/10.1093/nar/29.1.308
- Shriner, D. (2023). Overview of Admixture Mapping. Current Protocols , 3(2), e677. https://doi.org/10.1002/cpz1.677
- Shriner, D., Adeyemo, A., Ramos, E., Chen, G., & Rotimi, C. N. (2011). Mapping of disease-associated variants in admixed populations. Genome Biology , 12(5), 223. https://doi.org/10.1186/gb-2011-12-5-223
- Tekola-Ayele, F., Ouidir, M., Shrestha, D., Workalemahu, T., Rahman, M. L., Mendola, P., Grantz, K. L., Hinkle, S. N., Wu, J., & Zhang, C. (2021). Admixture mapping identifies African and Amerindigenous local ancestry loci associated with fetal growth. Human Genetics , 140(7), 985–997. https://doi.org/10.1007/s00439-021-02265-4
- Wilson, B. D., Ricks-Santi, L. J., Mason, T. E., Abbas, M., Kittles, R. A., Dunston, G. M., & Kanaan, Y. M. (2018). Admixture mapping links RACGAP1 regulation to prostate cancer in African Americans. Cancer Genomics & Proteomics, 15(3), 185–191. https://doi.org/10.21873/cgp.20076